筛选
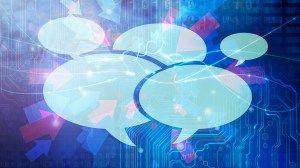
Managed File Transfer: Share & Process Documents Securely via Cloud, AS2, HTTP, and Email
Effective and secure file transfer plays a critical role in maintaining uninterrupted workflows. Whether you want to share sensitive financial data, transmit large media files, or synchronize information across systems, a managed file transfer (MFT) solution is a required for secure data exchange. MTF ensures control, efficiency, and performance across various protocols. What is Managed File Transfer? Managed File Transfer allows businesses to securely exchange and transfer digital files of any size or format with partners, customers, and employees. MFT goes beyond basic file transfer protocols by providing advanced security features such as encryption, authentication, and data integrity checks. These features mitigate the risk of data breaches and ensure compliance with industry regulations. Benefits of Implementing Managed File Transfer Solutions MFT solutions can significantly enhance the efficiency and performance of organizations of all sizes. Here are some key benefits of MFT: Enhanced Data Security MFT solutions employ robust encryption measures to safeguard files, minimizing the risk of data breaches. By utilizing strong encryption algorithms and secure protocols, MFT solutions ensure that sensitive information remains confidential and protected throughout the transfer process. Automation of File Transfer Processes One of the primary benefits of MFT solutions is the automation of file transfer procedures. Automation eliminates the need for manual intervention, reducing errors and saving valuable time. Predefined workflows can be established within MFT systems, enabling files to be automatically transferred to designated recipients. This enhances efficiency and allows you to focus on more strategic tasks. Improved Visibility and Control MFT solutions provide higher visibility and control over your file transfers. They facilitate tracking and monitoring of file activities, offering insights into who sent the file, who received it, who accessed certain files, when they were accessed, and any modifications made. This feature helps in streamlining files from the different business partners and track their transfer. It also helps to monitor server security for any possible breaches and enhances visibility which helps maintain compliance with industry regulations and internal policies and minimizes the risk of incurring non-compliance penalties. How Managed File Transfer Works MFT involves a series of steps to transfer files between systems securely. The typical process includes: Authentication: Users verify their identity and establish necessary permissions to access and transfer files, ensuring the file transfer process’s integrity. Encryption: Encryption protects files during transit by converting the data into a format only decipherable by the appropriate decryption key. Transfer: Encrypted files move from the source system to the destination system through various MFT protocols and communication channels, like the Internet, private networks, or dedicated connections. Decryption: Upon arrival, files undergo decryption, using the appropriate decryption key to return the data to its original format for further processing. Notification: Notifications are sent to parties involved in the file transfer. These notifications convey information about transfer status, errors or issues encountered, and confirmation of successful delivery. Understanding MFT Protocols MFT protocols are a set of rules and standards that govern the secure and efficient exchange of files between systems, both within and across organizations. These protocols are essential in facilitating seamless communication between systems via MFT. Here are the some of the MFT protocols: FTP (File Transfer Protocol) is one of the oldest and most straightforward methods for transferring files over a network. FTP allows files to be transferred between systems using a client-server architecture and operates on two channels: the control channel for sending commands and the data channel for transferring files. However, it lacks built-in security, making it less suitable for sensitive data transfer. SFTP (Secure File Transfer Protocol) adds an extra layer of security by using encryption. It combines FTP features with SSH (Secure Shell) security to protect file transfers from unauthorized access. AS2 is a specific protocol designed for secure business-to-business (B2B) data exchange between trading partners. It employs digital signatures and encryption to guarantee data integrity and confidentiality during transfer. AS2 is widely used in industries like healthcare and retail for secure EDI (Electronic Data Interchange) transactions and other B2B communications. HTTP (Hypertext Transfer Protocol) and its secure variant, HTTPS (HTTP Secure), are commonly used for web-based file transfers. While not exclusive to MFT, they serve as the backbone for many MFT solutions. HTTPS uses SSL/TLS to secure data transfers over the internet, making it suitable for both public and private network environments. Manage and Process All Incoming Files with LIKE.TG LIKE.TG’s Intelligent Document Processing feature is a pivotal component within LIKE.TG’s comprehensive no-code data management platform. It has strong capabilities designed to facilitate secure and efficient file transfers while seamlessly integrating with LIKE.TG’s broader data management ecosystem. With a focus on encryption, automation, and compliance, LIKE.TG MFT ensures seamless data flow, enabling you to achieve your data integration, extraction, and warehousing goals with unparalleled ease and reliability. Key Features of LIKE.TG’s MFT Functionality LIKE.TG IDP solution offers a comprehensive suite of features and functionalities tailored to meet the diverse needs of every user. These features go beyond basic file transfer capabilities, providing every user with enhanced control, security, and efficiency. Multi-protocol support: LIKE.TG supports various transfer protocols, including SFTP, FTP, email, cloud, HTTP, and AS2 for EDI. This versatility lets you connect with all your partners and systems using your preferred methods. Secure file transfer: Secure file transfer features include encryption/decryption using predefined algorithms or certificates and control over file size and type limits, enhancing data security. Automated workflows: LIKE.TG streamlines file transfer processes by automating repetitive tasks. This saves time and reduces the risk of human error, ensuring reliable and consistent transfers. Job triggering and ETL capabilities: LIKE.TG enables triggering jobs, including ETL and data extraction processes, upon receiving files. This ensures seamless data processing and integration with other systems. Advanced scheduling and notification capabilities: The MFT solution offers flexible scheduling options, allowing you to optimize file transfers based on your specific requirements. Additionally, LIKE.TG also provides real-time notifications to keep stakeholders informed about the status of transfers. Compliance adherence: LIKE.TG helps you meet industry-specific regulations such as GDPR and HIPAA. It provides built-in features and configurations that align with these regulations, ensuring data privacy and compliance. File monitoring and tracking: Users can monitor all incoming and outgoing file transfers in real-time through a dedicated window, providing transparency and control over data flow within the organization. File journey tracking: LIKE.TG allows senders to trace the journey of their files within the system, providing visibility into the status and location of transferred data. Role-based access management: Role-based access control ensures that only authorized individuals can access specific functionalities, enhancing security and compliance within the organization. Final Words Managed File Transfer is a powerful solution that helps businesses securely exchange data, streamline operations, and ensure compliance with data protection regulations. Adopting the right MFT solution can optimize your file transfer processes and pave the way for seamless digital transformation. LIKE.TG’s Intelligent Document Processing is your gateway to efficient data handling. With multi-protocol support, robust security, automation, and compliance features, LIKE.TG seamlessly integrates with your data workflows. Take control of your data with LIKE.TG MFT. Ready to see it in action? Request a free demo for your use case today! SECURELY MANAGE SENSITIVE DATA LIKE.TG's Managed File Transfer streamlines secure and efficient file transfers with multi-protocol support, automated workflows, and advanced scheduling, ensuring compliance and data integrity. View Demo
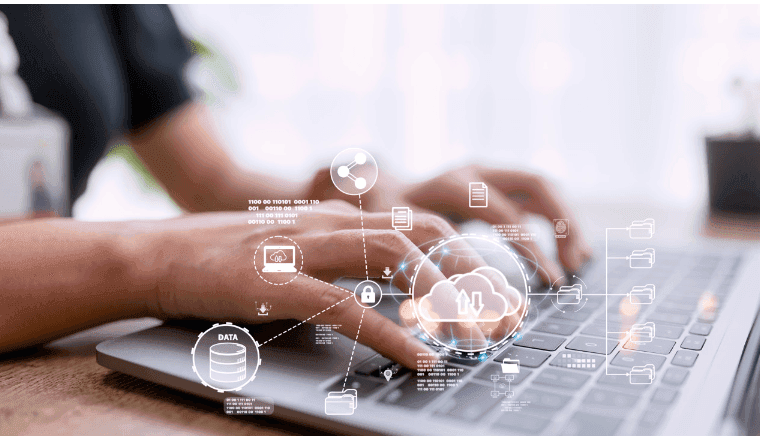
Data Warehouse vs. Database: Understanding the Differences
Businesses rely heavily on various technologies to manage and analyze their growing amounts of data. Data warehouses and databases are two key technologies that play a crucial role in data management. While both are meant for storing and retrieving data, they serve different purposes and have distinct characteristics. This comprehensive guide will explore the differences between data warehouses vs databases, their unique features and how to choose between them. What is a Data Warehouse? A data warehouse is not just a simple storage facility; it is a powerful tool that enables organizations to gain valuable insights from their data. Imagine a data warehouse as a vast, well-organized warehouse where you store and manage your data for easy access and analysis. One of the primary purposes of a data warehouse is to consolidate data from various sources into a single, unified view. Businesses use this single source of truth to analyze their data holistically, uncover patterns, trends, and correlations that may not be apparent when data sources are examined individually. Data warehouses employ a process called Extract, Transform, Load (ETL), whereby data is extracted from different operational systems, such as customer relationship management (CRM) platforms, enterprise resource planning (ERP) systems and more and then it undergoes a transformation process to ensure consistency and compatibility. Finally, the transformed data is loaded into the data warehouse for easy accessibility and analysis. A data warehouse enhances the reliability and accuracy of its information through data cleansing, integration, and standardization. Ensuring reliability is crucial for organizations to make informed decisions based on historical data and gain valuable insights into their operations. Unique Features of a Data Warehouse Data Integration: Data integration is a critical aspect of data warehousing. It involves gathering and transforming data from various sources into a unified format. This process requires careful planning and implementation to ensure the integrated data is accurate, consistent, and reliable. Subject-Oriented: The subject-oriented nature of data warehouses allows organizations to focus on specific business areas. For example, a retail company may have separate sales, inventory, and customer data marts. Analysts and decision-makers can easily access and analyze the information they need by organizing data around these subjects. This subject-oriented approach enables targeted analysis and facilitates the identification of trends, patterns, and opportunities within specific business domains. Time-Variant: Time-variant data warehousing enables organizations to analyze historical data and track changes over time. Data warehouses are capable of capturing and storing data at regular intervals, which provides a comprehensive historical record that can be used for trend analysis, forecasting, and performance evaluation. For example, a data warehouse for a manufacturing company may store production data from the past five years, so analysts can identify seasonal patterns, production trends, and areas for improvement. Non-Volatile: The non-volatile nature of data warehouses ensures that the stored data remains unchanged and consistent over time. Unlike operational databases that are frequently updated, data warehouses are designed to be read-only, which ensures a stable and reliable source of information for analysis. Why Use a Data Warehouse? Data warehouses offer numerous advantages for organizations that need to manage and analyze large volumes of data. Here are some of the key advantages of using a data warehouse: Business intelligence and analytics: Data warehouses consolidate diverse data sources and enable in-depth analysis, reporting, and decision-making. Data transformation and normalization: The ETL process within data warehouses cleans, transforms, and standardizes data and ensures data suitability for analysis and reduces data preparation time. Scalability: Data warehouses can expand their storage and processing capacity as data volumes grow, so they can easily accommodate the increasing demands of an organization. What is a Database? In contrast to a data warehouse, a database is a structured collection of data designed to support transactional operations. Think of a database as a digital filing cabinet that allows users to store, retrieve, and manipulate data efficiently. Databases are optimized for fast read and write operations, which makes them ideal for applications that require real-time data processing and quick access to specific information. They are commonly used in scenarios where speed and accuracy are paramount, such as online transaction processing (OLTP) systems. Traditional databases adhere to the ACID (Atomicity, Consistency, Isolation, Durability) properties, which ensure data integrity and reliability. These properties guarantee that each transaction is treated as a single, indivisible unit, so the consistency of the database is not compromised even in the face of failures or concurrent operations. Characteristics of a Database Transactional Support: Databases provide transactional support, which means that data modifications occur reliably and consistently. Transactions groups multiple operations, so either all changes are committed or none at all, which guarantees data integrity and helps maintain the database’s ACID properties. Concurrent Access: They are designed to handle concurrent access through through locking mechanisms and transaction isolation levels. So, multiple users and applications can simultaneously read and modify data without conflicts or inconsistencies. Normalized Data Structures: Databases employ normalization techniques to eliminate data redundancy and improve data consistency. Normalization breaks down data into smaller, more manageable tables and establishes relationships between them, which reduces data duplication. Normalization also simplifies data maintenance and enhances data integrity. High-Speed Performance: Databases are optimized for fast read and write operations. Database systems use performance optimization techniques, such as indexing, query optimization, and caching. This makes them suitable for applications that require real-time responsiveness, such as e-commerce platforms, financial systems, and online reservation systems. Why Use a Database? Databases offer a structured and efficient approach to storing, organizing, and retrieving data. Using a database offers several advantages for individuals and organizations, such as: Data persistence: Databases ensure data remains accessible and secure, preventing loss or degradation over time. Data integrity and consistency: Databases enforce constraints and validation rules to maintain data accuracy and quality. Data sharing and collaboration: Database promotes teamwork and efficiency since it lets multiple users access, modify, and interact with data simultaneously. Data retrieval capabilities: Databases support complex querying, sorting, and filtering options. This allows users to extract specific information quickly and effectively. Database Vs Data Warehouse: Key Differences On the surface, data warehouses are designed for optimized analytical processing. They support complex queries and historical analysis, while databases are more general-purpose and focus on transactional data management and application support. Here are some more differences between the two: Aspect Database Data Warehouse Data Structure Stores structured data Stores both structured and semi-structured data Data Volume Designed for smaller data volumes Built for large volumes of historical data Data Transformation Minimal data transformation Often includes ETL (Extract, Transform, Load) processes Query Complexity Suited for simple, transactional queries Designed for complex analytical queries Usage Used for day-to-day operations Employed for business intelligence, analysis, and reporting Latency Provides low-latency access to data May have higher latency due to complex queries Scalability Typically vertically scalable Horizontally scalable to accommodate data growth Schema Changes Complex schema changes may require downtime More flexible in adapting to schema changes Data Retention Stores recent and frequently changing data Retains historical data for trend analysis Database Vs Data Warehouse: Similarities While databases and data warehouses are meant for different purposes, they do have some underlying similarities as well: Aspect Data Warehouse and Database Data Retrieval Supports querying for data retrieval Data Management Organizes and manages data efficiently Data Security Implements security measures to protect data Data Integrity Ensures data integrity through constraints Data Backup and Recovery Provides backup and recovery mechanisms Data Indexing Uses indexing for faster data access Structured Query Language Utilizes SQL for querying and data manipulation User Access Control Offers user access control and permissions Data Warehouse Vs Database: Factors to Consider Deciding between a data warehouse and a database requires careful consideration of several factors: Business Requirements:Organizations should assess their specific needs and priorities as well as define the purpose of the data management system and the type of data analysis required. It is important to understand the goals and objectives of the data management system. Is the organization looking to improve operational efficiency or make strategic decisions based on comprehensive data analysis? By defining the purpose of the system, organizations can better assess whether a data warehouse or a database is the right fit. Volume and Complexity of Data:Consider the organization’s data volume, variety, and velocity. A data warehouse may be the better choice if the business has vast amounts of data that require complex analysis. Data warehouses are designed to handle large volumes of data and support advanced analytics, which is why they are ideal for organizations with extensive historical data requiring in-depth analysis. Budget and Resources:Evaluate the budget and available resources to determine the feasibility of implementing and maintaining a data warehouse. A database may be a more feasible if the organization has limited resources and budget constraints. Databases generally require less upfront investment and technical expertise compared to data warehouses. They are suitable for organizations that need a reliable and efficient data management system without extensive historical data analysis. Assessing Your Business Needs Analyze the organization’s specific business needs to determine the best fit: Operational Efficiency: Databases are designed to handle transactional data efficiently and provide quick access to real-time information, so they are best for organizations prioritizing operational efficiency. Strategic Decision-Making: Data warehouses are optimized for complex analysis, historical trend analysis, and predictive analytics. They allow organizations to derive valuable insights from large volumes of data, enabling informed decision-making and strategic planning. Hybrid Approach:A combination of both technologies may be appropriate in some cases. A hybrid approach allows organizations to leverage the benefits of real-time data processing and sophisticated analysis in a single integrated solution. By integrating data from both sources, organizations can have a comprehensive view of their data. The Future of Data Warehouses and Databases Emerging Trends in Data Warehousing Data warehousing continues to evolve to meet the ever-increasing demands of businesses. Some emerging trends include: Cloud-Based Data Warehouses:Cloud computing offers greater scalability, flexibility, and cost-effectiveness for storing and analyzing vast amounts of data. Big Data Integration:Data warehouses are adapting to incorporate big data technologies, enabling organizations to analyze large and diverse data sets, including unstructured and semi-structured data. Data Virtualization:Virtualization allows for real-time data access and integration from multiple sources without physically moving the data. Data Governance and Compliance: With the increasing focus on data privacy and regulations like GDPR, data warehouses are incorporating robust data governance and compliance features to ensure data’s responsible and legal handling. Real-time Data Processing: Data warehouses handle streaming data through instant data processing and real-time analytics that are essential in financial trading and IoT applications. Innovations in Database Technology Database technology is also advancing to keep pace with evolving business needs. Some notable innovations include: In-Memory Databases:These databases store data in memory, and provide lightning-fast access for applications that require real-time responsiveness. Distributed Databases:Distributed databases distribute data across multiple machines, improving scalability, fault tolerance, and performance. NoSQL Databases:These non-relational databases handle unstructured and semi-structured data, offering flexible schemas and horizontal scalability. Database as a Service (DBaaS): The DBaaS model allows businesses to access and manage databases in the cloud, providing scalability, reduced administrative overhead, and cost savings. Edge Databases: As edge computing gains prominence, databases designed for edge locations are emerging. These databases enable real-time data processing and analytics at the edge of the network, reducing latency and enhancing performance for applications like autonomous vehicles and IoT devices. Final Words There is no clear winner in the data warehouses vs database debate. They both serve distinct purposes and cater to different business needs. While databases excel in real-time transactional operations, data warehouses are particularly effective for analytical queries and historical analysis. Understanding their differences, unique features, and the organization’s specific requirements is essential to make an informed decision. LIKE.TG offers an end-to-end data warehousing solution that helps businesses create data warehouses from scratch. UsingLIKE.TG DW builder,organizations can use the meta-data-driven approach to build a data warehouse and deploy it on the cloud, on-premise, or in a hybrid environment. Sign up for a free demo or free 14-day trial to get started with LIKE.TG DW Builder today!
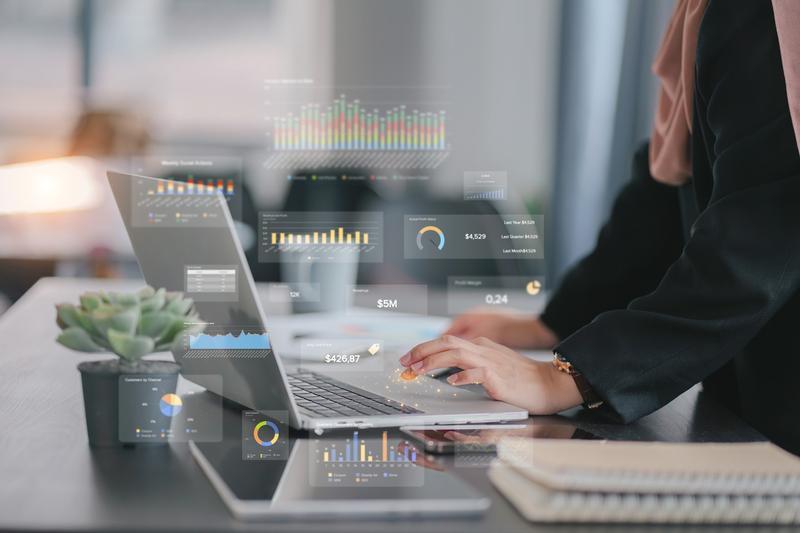
The Ultimate Guide to Enterprise Data Warehouse
Data has permeated all aspects of our lives today. Whether it’s Netflix offering personalized recommendations, Facebook and Instagram curating targeted ads, or Google Maps providing real-time navigation, each instance is driven by the strategic use of data. But have you ever wondered how data informs the decision-making process? The key to leveraging data lies in how well it is organized and how reliable it is, something that an Enterprise Data Warehouse (EDW) can help with. An enterprise data warehouse allows organizations to integrate, store, and analyze information from diverse sources into a single source of truth (SSOT) for enterprise-wide reporting and analytics. Let’s delve deeper to get a comprehensive understanding of the EDW, particularly its key components, types, architecture, and benefits. What is an Enterprise Data Warehouse (EDW)? An Enterprise Data Warehouse is a centralized repository that consolidates data from various sources within an organization for business intelligence, reporting, and analysis. It is designed to provide a long-range view of data over time, allowing organizations to analyze historical trends, make informed predictions, and respond to changing market conditions promptly. The architecture of an EDW ensures high data integrity, reliability, and accessibility. It supports a wide range of business applications, including customer relationship management (CRM), supply chain management, and financial management. An EDW enhances operational efficiency and strategic decision-making by offering a single, comprehensive view of business data, thereby providing a competitive edge in the market. Key Components of an Enterprise Data Warehouse (EDW) Data Sources: There are diverse data sources in an enterprise data warehouse (EDW), including relational databases, external data feeds, and flat files. Data from these sources is extracted through SQL queries, batch processing, and real-time streaming. Staging Area: The staging area temporarily holds raw data before further processing through the extract, transform, and load (ETL) process. ETL typically involves data transformation before loading into the data warehouse. On the other hand, the modern extract, load, and transform (ELT) approach first loads raw data and then performs transformations within the data warehouse. Storage Layer: The EDW’s core usually utilizes a relational database or specialized data warehousing platforms like Snowflake or Amazon Redshift. The storage layer acts as the foundational repository for the enterprise’s data assets, enabling efficient data storage, retrieval, and analysis. Presentation Layer: Finally, the presentation layer connects users to the EDW using BI tools and reporting platforms such as Tableau, Power BI, or Looker. Users can create reports, dashboards, and visualizations to extract meaningful insights. Data Warehouse vs. Enterprise Data Warehouse The primary difference between a data warehouse and an enterprise data warehouse lies in their scope and scale. A Data Warehouse is typically designed to serve a specific business function or department within an organization. It collects, organizes, and stores data related to that particular function or department and is used for reporting and data analysis within that context. On the other hand, an Enterprise Data Warehouse is designed to serve the entire organization rather than a specific department or function. It integrates data from various sources into a unified business view that supports cross-functional analysis, reporting, and strategic decision-making at the enterprise level. Benefits of an EDW Having gained a clear understanding of what comprises an enterprise data warehouse and its key components, let’s now discuss its benefits: Immediate Data Access: EDWs offer instant access to data, so users can retrieve critical information swiftly. The agility facilitates faster decision-making and analysis. Organizations can, therefore, respond promptly to evolving business conditions and gain a competitive edge. EDWs optimize data storage and retrieval mechanisms and ensure that data is readily available for real-time queries. Efficient Collaboration: By centralizing data, EDWs foster cross-departmental collaboration. Teams can seamlessly access, share, and jointly analyze data, facilitating better alignment, problem-solving, and innovation throughout the organization. EDWs often come with collaborative features and permissions that allow teams to engage with shared datasets in real-time, which enhances teamwork and productivity. Holistic Data View: Enterprise data warehouses integrate data from disparate sources and create a single source of truth for the organization. They provide a consolidated and comprehensive data view, encompassing information from various sources and departments. A complete view of organizational data empowers users to recognize hidden correlations, trends, and opportunities that can drive informed decision-making and strategic planning. Empowering Non-Technical Users: EDWs offer user-friendly tools that enable non-technical personnel in departments such as marketing, finance, and HR to access and interpret data. By doing so, enterprises foster a data-driven culture, promote collaboration and facilitate effective decision-making. For instance, marketing teams can use data from EDWs to analyze customer behavior and optimize campaigns, while finance can monitor financial performance and HR can track workforce metrics, all contributing to informed, cross-functional decision-making. Data Governance and Compliance: Data security and compliance with data privacy regulations, such as GDPR or HIPAA, are paramount for businesses handling sensitive information. To protect data, enterprise data warehouses offer robust security features, which include encryption, access controls, and auditing capabilities. They also help organizations enforce data governance practices, ensuring that data is handled consistently and complies with relevant laws. EDWs minimize the risk of data breaches and help organizations avoid legal and financial penalties associated with non-compliance. Business Needs that Require an Enterprise Data Warehouse From increasing data volumes to the demand for real-time insights, businesses are continually redefining their requirements. In this dynamic landscape, enterprise data warehouses enable organizations to stay agile and competitive. Let’s explore the key drivers that make EDW vital for organizations navigating changing business requirements. Improved Decision-Making: An EDW provides decision-makers with a single source of truth. It ensures that the data is accurate, up-to-date, and consistent, which is critical for making informed decisions. With data readily available, decision-makers can quickly access the information they need to respond to business challenges, seize opportunities, and address critical issues. EDWs also enable sophisticated data analysis and reporting, allowing organizations to derive valuable insights that drive strategic planning and operational improvements. Historical Analysis: An enterprise data warehouse’s ability to store historical data is vital for historical analysis and trend identification. By retaining historical records, businesses can evaluate past performance, track changes over time, and predict future trends. In industries like finance, where historical data can inform investment decisions, or retail, where it helps with inventory management and demand forecasting, the ability to monitor past data records is crucial. Real-Time Data Access: Some businesses require real-time data access to respond to events as they unfold, particularly enterprises operating in the e-commerce, finance, or manufacturing domain, where decisions must be made immediately. An EDW can support real-time data integration, analysis, and reporting, ensuring decision-makers can access the most current information. Businesses can, therefore, respond rapidly to market changes, customer preferences, or supply chain disruptions and stay agile and competitive in the fast-paced environment. Types of Enterprise Data Warehouses Enterprise data warehouses come in several types, depending on their architecture and an organization’s specific needs. Here are some common types: On-Premises Data Warehouses: On-premises data warehouses are located within an organization’s own data center or facility. They offer a high degree of control over hardware, software, and data security, which makes them ideal for organizations with stringent data privacy and compliance requirements. They provide an environment where businesses can retain complete control over their data assets, ensuring data remains within their physical boundaries. This setup promotes trust and confidence, especially when dealing with sensitive information. Cloud-Based Data Warehouses: Cloud-based data warehouses are hosted on cloud service provider platforms such as Amazon Web Services (AWS), Google Cloud Platform (GCP), or Microsoft Azure. They provide scalability and cost-efficiency through pay-as-you-go pricing, reducing initial capital expenses, and transferring maintenance to the cloud provider. Cloud platforms enable data accessibility from anywhere with an internet connection and offer flexibility for a modern, dispersed workforce. It’s particularly beneficial for organizations seeking to quickly adapt to changing work dynamics, embrace remote work, and harness the power of the cloud for data analysis and reporting. Hybrid Data Warehouses: Hybrid data warehouses combine on-premises and cloud components, allowing organizations to maintain control of sensitive information while outsourcing other parts to the cloud. The hybrid approach offers flexibility by using the cloud for scalable workloads and on-premises infrastructure for steady-state requirements. Data management across these environments may require advanced strategies, but the advantages include cost control and adaptability to dynamic business needs. Many organizations opt for hybrid solutions to balance on-premises control over sensitive data and cloud flexibility while managing expenses. Enterprise Data Warehouse Schemas EDW schemas define how data is structured and stored within data warehousing systems. Let’s explore the three main types of schemas: 1. Star Schema: The star schema is a simple data organization structure used in data warehousing. It consists of a central fact table with core data and dimension tables that provide context. The design simplifies data retrieval and analysis because it allows for easy and quick querying. It’s well-suited for organizations with straightforward data structures where data analysis must be straightforward and efficient. For example, a retail data warehouse uses a star schema with a central fact table for sales transactions and dimension tables for products, customers, time, and stores. 2. Snowflake Schema: The snowflake schema extends the star schema by further normalizing the dimension tables. Normalization involves breaking down dimension tables into sub-dimensions, reducing data redundancy. This schema is particularly useful for data warehouses with substantial data volumes. It optimizes storage by minimizing redundancy and enhancing data quality, making it a strong choice for organizations with diverse yet highly structured data. For example, an e-commerce platform employs a snowflake schema for user orders, with normalized dimension tables for products, customers, addresses, and further tables for cities and states. 3. Galaxy Schema: The galaxy schema, also known as a Fact Constellation Schema, is a complex yet versatile architecture, ideal for organizations with diverse data needs. It handles multiple fact tables that share dimension tables, streamlining data integration across different domains. Since it enhances analytical capabilities and reporting flexibility across data domains, this schema is invaluable for large enterprises with intricate reporting requirements. For example, a multinational conglomerate’s data warehouse uses a galaxy schema with multiple fact tables for sales, production, and customer support requests, all sharing common dimension tables for time, geography, and customer data. Enterprise Data Warehouse Architecture The architecture of Enterprise Data Warehouse (EDW) is a critical aspect of efficient data handling and analysis. Here, we’ll examine three core EDW architecture models, each with its specific attributes. These models impact how data is accessed and analyzed, providing tailored solutions for diverse business needs. Let’s explore these architectures in detail. One-tier Architecture: This architecture establishes a direct connection between the user interface and the data source. It simplifies data queries and analysis by eliminating intermediary layers. The importance of this architecture lies in its simplicity and directness, making data access and analysis straightforward and efficient. Two-tier Architecture: This model introduces an intermediary layer, known as the Data Mart Layer, between the user interface and the EDW. Data marts, specialized subsets of the data warehouse, cater to specific business areas such as sales, marketing, and HR, enhancing the relevance and efficiency of data retrieval and analysis. The significance of this architecture is its ability to provide targeted, relevant data to specific user groups, improving the accuracy and efficiency of data analysis. Three-tier architecture: This structure introduces an Online Analytical Processing (OLAP) Layer to the dual-tier model. Positioned between the Data Mart Layer and the user interface, the OLAP layer employs OLAP cubes for multi-dimensional data analysis. The importance of this architecture is its capacity to handle complex, multi-dimensional queries, enhancing the system’s adaptability and scalability. The OLAP cubes support various operations, each with its unique importance: Roll-up: Aggregates data by ascending a concept hierarchy, aiding in trend analysis. Drill-down: Descends the concept hierarchy, providing detailed data for root cause analysis. Slice: Applies a filter on a single dimension, enabling focused analysis on a specific data point. Dice: Applies filters on two or more dimensions, facilitating detailed comparative analysis. How To Select a Warehouse for Your Organization? Selecting an Enterprise Data Warehouse (EDW) for your organization is a pivotal decision that can significantly impact business operations and analytical capabilities. Here are some key factors to consider: Business Requirements: Start by understanding the specific business goals and objectives that your organization aims to achieve with the EDW. Defining your business goals provides a strategic foundation for selecting an EDW that aligns with your organization’s needs. These could include improving decision-making, enhancing customer insights, or streamlining operations. Data Needs and Compatibility: Identify the data types you work with, such as structured, semi-structured, or unstructured, and ensure that the chosen enterprise data warehouse can handle them effectively. Additionally, evaluate how well it can integrate with your existing data sources, ETL processes, and business intelligence tools. Data compatibility ensures that your EDW can efficiently work with your existing systems and provide accurate and valuable insights. Scalability and Performance: Ensure that the chosen solution can scale as your data volumes and analytical demands grow. A scalable enterprise data warehouse can accommodate increased data without compromising performance, allowing your organization to consistently process, analyze, and extract valuable insights from the data. The performance of the EDW should meet or exceed your organization’s expectations for query and data processing speed. Data Security and Compliance: Assess the security features of the EDW, including data encryption, access controls, and compliance with relevant regulations. A robust data security infrastructure helps safeguard sensitive information and ensures compliance with data protection laws, such as GDPR or HIPAA, which can prevent costly breaches and legal consequences. Cost and Budget Considerations: Analyze the budget available for implementing and maintaining the enterprise data warehouse, including both upfront expenses and ongoing operational costs. Understanding the licensing and pricing structure of the EDW is critical to avoid unexpected expenses. A well-planned budget ensures your organization can sustain the EDW over time without financial strain. Vendor Reputation and Support: Research the vendor’s track record, read reviews, and examine case studies and customer testimonials. A reputable vendor is more likely to provide a reliable and well-supported solution, reducing the risk of technical issues and ensuring you receive assistance when needed. Vendor support can be crucial for troubleshooting, updates, and addressing any concerns or questions your organization may have. Conclusion Looking ahead, the future of enterprise data warehouses appears promising. With technological advancements, we can expect EDWs to become more sophisticated, offering enhanced capabilities such as real-time data processing, advanced analytics, and seamless integration with various data sources. Furthermore, as businesses increasingly adopt artificial intelligence and machine learning, EDWs will provide the necessary data infrastructure for these advanced technologies. The significance of EDWs lies in their capacity to turn raw data into actionable insights. With every piece of information, they empower organizations to pivot, adapt, and thrive in an ever-changing marketplace. So, it is important to consider your specific needs and objectives before choosing an enterprise data warehouse. Make sure it’s a system that can support your data management and analysis needs now and grow with you in the future. Ready to take the next step? Download LIKE.TG Data Warehouse Builder’s 14-day free trial today and explore how our EDW solution can cater to your business’s unique needs. Build Your Enterprise Data Warehouse in Minutes Leverage LIKE.TG Data Warehouse Builder to seamlessly design, construct, and optimize your enterprise data warehouse for unparalleled insights and performance. Download Free Trial
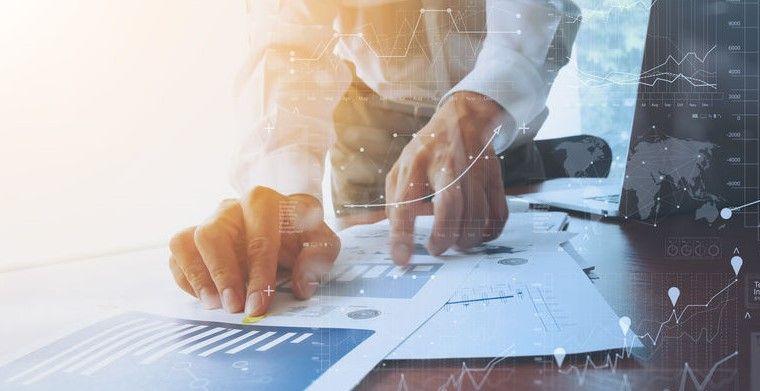
API Developer Portal: Importance and Best Practices
As businesses grow, their interactions with a variety of internal, external, and third-party APIs become more prevalent. According to the Developers Economic Survey 2023, over 90% of developers use APIs to accelerate digital interactions, with 74% of developers using third-party APIs. Establishing a central hub that simplifies resource access for everyone becomes essential to facilitate the consumption of APIs and create a more efficient workflow. That’s where the API developer portal comes into play– a valuable portal designed to make API usage user-friendly for developers, resulting in faster API integration and consumption. What is an API Developer Portal? An API developer portal is a platform that serves as a comprehensive resource for developers looking to integrate APIs into their applications. It’s a central hub with all the tools, resources, and information required to consume APIs effectively. Let’s take a fictional example of a company called “WeatherNow” that offers weather data through an API. To facilitate developers in accessing and using their weather data in applications, they can publish an API Developer Portal. This portal will contain essential resources like API documentation, key management, code samples, and developer support. The WeatherNow API Developer Portal will serve as a core resource for developers looking to integrate WeatherNow’s weather data into their applications. It will provide them with the necessary information for successful API utilization and foster a community for collaboration and assistance. Components of a Good Developer Portal An API developer portal is a gateway for developers to access documentation, sample code, tutorials, and support forums, enabling them to understand and utilize APIs easily. Within a good API developer portal, developers can find detailed documentation that outlines the data resources, endpoints, and expected parameters inputs or outputs of the available APIs. This documentation clearly explains how to interact with the APIs and guides developers through the API integration process. The documentation is made available in well-known standard formats such as Swagger Open API, WSDL, Async API, etc. to enable quick import and utility. In addition to documentation, an effective developer portal also offers sample code snippets in various languages that developers can use as a starting point for their projects. These code snippets demonstrate how to utilize the API’s capabilities effectively. The portal accelerates the development process by providing ready-to-use code examples, enabling developers to prototype and test their ideas quickly. API developer portals also often include tutorials and step-by-step guides that walk developers through common use cases and scenarios. These tutorials provide practical examples and offer insights into leveraging the API’s features to solve real-world problems. Developer Portals also include user management and subscription flows to request access for various APIs. There is also a “try out” test environment to make test calls to APIs and ensure their access. The Importance of an API Developer Portal An API developer portal plays a crucial role in software development. Some of the various aspects that make it so important are: Enhancing Developer Experience An API developer portal goes beyond just providing documentation and code snippets. It aims to create a user-friendly environment for developers to explore, experiment, and integrate APIs into their applications. Imagine a developer trying to integrate an API without any guidance or support. It will become a complex, resource-intensive task. However, a well-designed API developer portal will offer comprehensive documentation, step-by-step tutorials, and even interactive examples to guide developers through the integration process. Facilitating API Adoption APIs are powerful tools that allow developers to leverage existing functionalities and services to enhance their applications. However, without proper documentation and guidance, developers may struggle to understand the intricacies of the API and how to utilize it effectively. Besides documentation, tutorials, and use cases, an API developer portal often includes a community forum or support channel where developers can ask questions, share their experiences, and seek expert guidance. This fosters a collaborative environment and encourages developers to explore the full potential of the APIs. Streamlining API Management An API developer portal also contributes to streamlining API management. It serves as a self-service platform for developers, empowering them to control their integrations and dependencies more with respect to version updates. Developers can generate API keys or tokens, monitor usage, and manage their applications through an API developer portal. This eliminates the need for manual intervention from API providers, reducing the workload and allowing them to focus on other critical tasks. Furthermore, an API Developer Portal often provides reporting features, allowing developers to gain insights into how their applications utilize the APIs. This data-driven approach enables developers to optimize their integrations, identify potential bottlenecks, and make informed decisions to improve the performance and efficiency of their applications. Best Practices for API Developer Portal There are several best practices for developing an API Developer Portal. From user-friendly design to high-quality documentation and robust security measures, each aspect plays an important role in providing developers with a seamless experience. These best practices include: Ensuring User-Friendly Design A good API Developer Portal prioritizes user experience. It goes beyond just providing the necessary information and focuses on creating an intuitive and visually appealing interface. Clear navigation menus, search functionality, and organized content are key elements that contribute to a user-friendly design. Imagine a developer visiting an API Developer Portal with a cluttered and unsystematic layout. They would have difficulty finding the information they need. On the other hand, a well-structured and organized interface will enable developers to easily navigate the portal, finding the resources they need efficiently. Furthermore, a clean and visually rich interface can also enhance the overall experience. Incorporating modern design principles, such as clean typography, consistent color schemes, and intuitive icons, makes the API developer portal functional and easy to navigate. Prioritizing Documentation Quality High-quality documentation is the backbone of any API Developer Portal. It serves as a comprehensive guide for developers, providing them with the necessary information to understand and utilize the API effectively. Here are some key factors to consider: Comprehensiveness:The documentation should cover all aspects of the API, including its endpoints, request/response formats, authentication methods, and error handling. Leaving any gaps in the documentation can lead to confusion and hinder developers’ progress. Accuracy:Ensuring the documentation is accurate and up to date is essential. Any discrepancies between the documentation and the actual API behavior can waste time and effort. Code Examples:Including code examples can significantly enhance the clarity and usefulness of the documentation. Developers often rely on these examples to understand how to interact with the API and to jumpstart their implementation. Implementing Robust Security Measures Security is a critical aspect of API development. An API Developer Portal should prioritize implementing robust security measures to protect sensitive information and prevent unauthorized access. Here are some key considerations: Secure Authentication Mechanisms:The API Developer Portal should support users to avail supported authentication mechanisms, such as OAuth, API keys, or JSON Web Tokens (JWT). These mechanisms ensure that only authorized developers can access the API and its resources. Role-Based Access Control:Implementing role-based access control allows developers to have different levels of access based on their roles and responsibilities. This ensures that sensitive operations and data are only accessible to authorized individuals. Thorough Vulnerability Testing:Regular vulnerability testing should be conducted to identify and address any potential security vulnerabilities. This includes penetration testing, code reviews, and security audits to ensure the API and the Developer Portal are resilient against attacks. Manage All Your APIs Access In One Platform An API developer portal is vital to modern software development. It serves as a platform for developers to access documentation, tools, and community support, ultimately enhancing their experience and facilitating the adoption of APIs. By following best practices and incorporating key characteristics, a well-designed API developer portal can make a significant difference in the success of API integrations and collaborations. LIKE.TG is a no-code API building and implementing solution with a self-service API developer portal that enables API consumers to discover, explore, and subscribe to public and private APIs. LIKE.TG empowers developers to make the most out of the APIs, reducing the learning curve and enabling them to build innovative applications more efficiently. Some of LIKE.TG’s developer portal features include: Easy-to-use portal so developers can subscribe and start their API integration process in minutes. Detailed documentation outlining the APIs’ functionalities, endpoints, and parameters. Ready to integrate Swagger documentations for the deployed APIs. Access token management and authorization. Ready to build, access, and consume APIs effortlessly? LIKE.TG makes it simple! Sign up for the 14-day free trial or a free demo today Ready to build, access, and consume APIs effortlessly? Discover, explore, and subscribe to public and private APIs with LIKE.TG's self-service API developer portal. View Demo
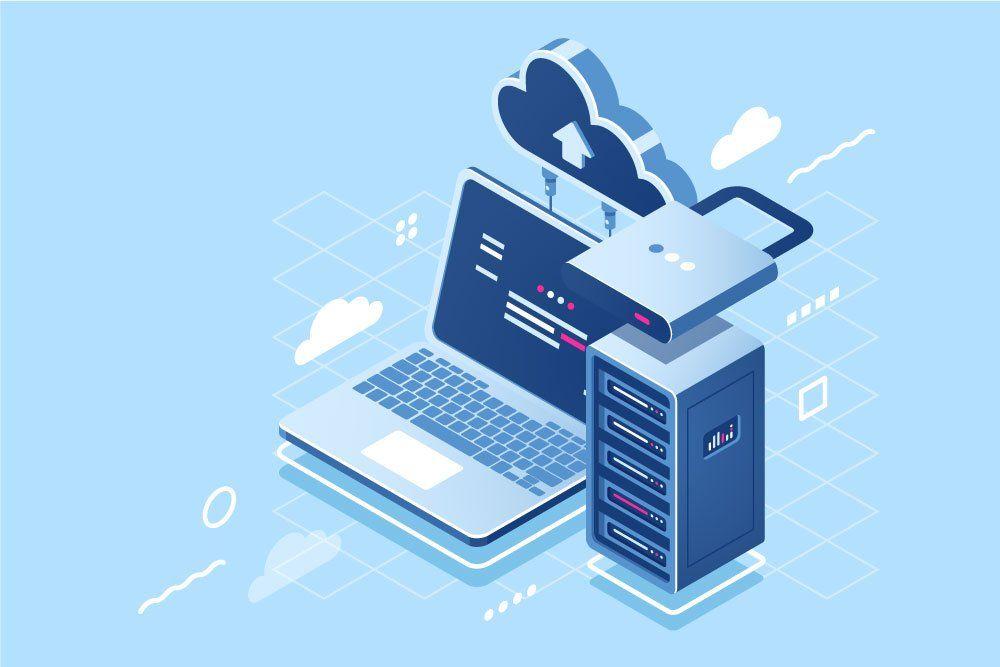
Finance Data Warehouse for Reporting and Analytics
The role of data warehousing in finance is indispensable. It serves as the foundation of modern finance operations and enables data-driven analysis and efficient processes to enhance customer service and investment strategies. Data warehouses have risen to prominence as fundamental tools that empower financial institutions to capitalize on the vast volumes of data for streamlined reporting and business intelligence. Banks, credit unions, insurance companies, investment companies, and various types of modern financial institutions rely on a finance data warehouse to make informed business decisions. A centralized repository of accurate, complete, and timely data allows financial institutions to gather actionable insights to make informed choices across strategic, tactical, and operational fronts. The North American data warehousing market is projected to dominate the global industry with a share of above 40% by 2025 (SDC Exec) Strategic Insights: The Finance Data Warehouse Advantage Financial institutions deal with a lot of data from various departments and subsidiaries. This data about customers, financial products, transactions, and market trends often comes in different formats and is stored in separate systems. To understand the vast amount of information available, they organize it and store it in a centralized repository. This consolidated repository helps analysts assess risks, predict future trends, and create effective strategies. And this is where a data warehouse becomes important. A data warehouse is the heart of this operation. It takes all the different data sources and puts them into one place, which makes it easier to report on and analyze. The key benefits of a finance data warehouse include: Centralized Data: A data warehouse contains data from various sources across different locations and systems. This consolidated repository ensures that financial institutions have a reliable, up-to-date, and accurate data pool for business intelligence. Efficient Reporting: Standardized data within a data warehouse simplifies the reporting process. This enables analysts to generate consistent reports swiftly, which are essential to evaluate performance, monitor financial health, and make informed strategic decisions. Enhanced Analytics: A financial data warehouse allows businesses to delve into advanced analytics to unlock a host of valuable benefits. These include improved loan portfolio management, more accurate credit risk assessment, and refined fraud detection, which results in better decision-making, cost reduction, and increased profitability. Regulatory Compliance: Data warehouses assist financial institutions adhere to regulatory requirements by centralizing and organizing data in a way that facilitates audits, regulatory reporting, and compliance monitoring. Customer Insights: Integrating data from various customer touchpoints to a data warehouse allows financial institutions to gain a 360-degree view of customers’ payment behavior, transaction history, and overall financial health. This facilitates targeted marketing, personalized services such as credit terms, and improved customer satisfaction. Faster Decision-Making: Quick access to comprehensive and reliable data in a data warehouse streamlines decision-making processes, which enables financial organizations to respond rapidly to market changes and customer needs. In addition, a finance data warehouse enhances various aspects of data management, such as: Data Security: Consolidating data in a data warehouse facilitates the implementation of robust security measures to protect sensitive financial information, including personally identifiable information. Data Integration: A data warehouse enables seamless integration of data from various systems and eliminates data silos and promotes interoperability and overall performance. Data Quality and Consistency: Data warehouses allow financial institutes to enforce rigorous data quality standards, which leads to improved data accuracy, completeness, and consistency. Data-driven Finance with LIKE.TG Download Now Who Can Benefit from a Finance Data Warehouse? Finance Executives and Decision-Makers Senior leaders in financial organizations, including banks and credit unions, rely on data to drive strategic decisions. Data warehousing empowers them with centralized data repositories, robust data quality management, and efficient querying tools. This facilitates data-driven decision-making, enhances operational efficiency, and provides a competitive advantage in the financial sector. Data Analysts and Technologists Data analysts and technology professionals within financial institutions benefit from data warehousing by automating repetitive tasks like data extraction and transformation. This automation allows them to focus on higher-value activities such as data analysis, modeling, and innovation. It not only enhances their job satisfaction but also enables them to contribute to the development of innovative financial products and solutions that drive business growth. Business Users and Operations Teams Business users within financial organizations often encounter challenges related to data accessibility and timely insights. With a data warehouse, they gain self-service reporting and analytics capabilities. This empowers them to generate reports on demand and reduce their reliance on IT or data teams. Such agility accelerates their ability to respond swiftly to market fluctuations, customer demands, and emerging financial opportunities, which ultimately strengthens the organization’s agility and competitiveness. Read More: The Cost of Building a Data Warehouse How a Finance Data Warehouse can Help with Risk Management The biggest functional area benefit of a Data Warehouse (DW) in finance is typically related to risk management. Data Warehousing enables financial organizations to aggregate and analyze vast amounts of historical and real-time data from various sources, which helps them assess and effectively manage various risks, including credit risk, market risk, operational risk, and compliance risk. The ability to centralize and standardize data within a finance data warehouse allows for more accurate risk modeling, early risk detection, and improved decision-making. Additionally, it enhances the organization’s ability to meet regulatory requirements, which is crucial in the highly regulated financial industry. A data warehouse architecture facilitates comprehensive insights into risk factors, which allows financial institutions to proactively identify potential issues, make informed risk assessments, and take the necessary actions to minimize financial losses and protect their stability and reputation. Building Credit Scoring Models with a Finance Data Warehouse Comprehensive customer data can be used to develop advanced credit scoring models. These models take into account a wide range of factors, including income, employment history, debt-to-income ratio, and behavioral data. Financial institutions can then use these scores to make decisions about interest rates, credit limits, and loan approvals. This enables them to tailor credit terms to each borrower’s risk profile. For example, customers with a strong credit history and stable income may be offered lower interest rates and higher credit limits, while those with higher risk profiles may receive more stringent terms. This leads to more accurate credit decisions and helps devise appropriate terms for different customer segments. Finance Data Warehouse: A Strategic, Future-Proof Investment An enterprise-grade data warehouse automation solution can give financial institutions significant returns. It streamlines data processes, reduces manual efforts, and enhances data accuracy, which ultimately leads to improved operational efficiency and cost savings. The return on investment varies based on a company’s size and objectives, but in the majority of cases, financial organizations typically recover their initial investment within the first two to three years. Building a finance data warehouse demands a substantial allocation of organizational resources, which raises concerns about both its initial justification and ongoing assessment. But despite this commitment, data warehouses consistently prove to be highly valuable and lucrative investments in the financial sector. Building a Finance Data Warehouse? Try LIKE.TG DW Builder! LIKE.TG DW Builderis a no-code data warehouse automation solution that empowers you todesign, develop, and deploy high-volume finance data warehouses in a matter of weeks. Trusted by Fortune 1000 companies, the flexible and scalable data warehousing solution comes with automation capabilities to fast-track design, development, and implementation phases. Equipped with LIKE.TG’s industrial-strength ETL engine, the solution allows you to build data models from scratch or reverse engineer your existing data models—all in a unified, zero-code environment. An All-Round Platform for Finance Data Warehouse LIKE.TG DW Builder is a part of LIKE.TG’s data ecosystem that has parallel processing ETL/ELT engine and data transformation, validation, and monitoring features. It provides a tailored set of data warehouse automation features to meet your specific data requirements. It also simplifies the resource-intensive task of consolidating data from various sources, including both on-premises and cloud platforms, to ensure you have a unified, high-quality data foundation that greatly enhances your financial reporting and analytics. For financial organizations, LIKE.TG’s capabilities facilitate the efficient management of historical financial data, which makes it easier to connect to diverse data sources and destinations. The push-down optimization feature significantly improves query performance, which enables finance professionals to focus on value-added activities, such as financial analysis, to identify emerging opportunities, optimize financial operations, and align their strategies with changing market dynamics. LIKE.TG has a library of built-in connectors that allow financial institutes to ingest and load data to reporting and analytics dashboards. Agile connectivity minimizes manual interventions and improves data accessibility. Coupled with LIKE.TG’s data lineage and scalability, it empowers them to seamlessly handle their data management processes as they grow while ensuring security and transparency throughout the process. In summary, LIKE.TG empowers financial professionals with the solution to make data more accessible for reporting and analytics, which results in more transparency, visibility, and informed decision-making. LIKE.TG Advantage LIKE.TG’s data warehousing automation tool simplifies and accelerates the development process. The metadata-driven, no-code solution allows financial organizations to design, develop, and deploy enterprise-grade data warehouses without requiring complex infrastructure or coding. This means that enterprise-grade data warehousing projects that would require months or even years to complete can be completed in a matter of a few weeks. Advanced Data Modeling and ETL/ELT Capabilities: LIKE.TG enables users to build automated data pipelines, which streamlines data processing with minimal manual intervention. Pre-built Transformations and Connectors: LIKE.TG provides a library of pre-built transformations and connectors that optimize data warehouse connectivity, which allows financial institutions to handle vast amounts of data from various channels. User-Friendly Interface: LIKE.TG’s drag-and-drop, intuitive user interface makes it easy for both technical and non-technical users to manage and maintain data and adapt to evolving business requirements. LIKE.TG’s unified solution also offers significant resource savings by eliminating the need for separate licenses, maintenance, and support for various tools and vendors, consequently optimizing cost-effectiveness and resource management. As a result, building a finance data warehouse with LIKE.TG provides a substantially lower total cost of ownership. With LIKE.TG as your partner, you can build a finance data warehouse that caters to your data management needs, both now and in the future. To get started, reach us at[emailprotected]or request afree trialtoday. Build Finance Data Warehouse with LIKE.TG Contact Us
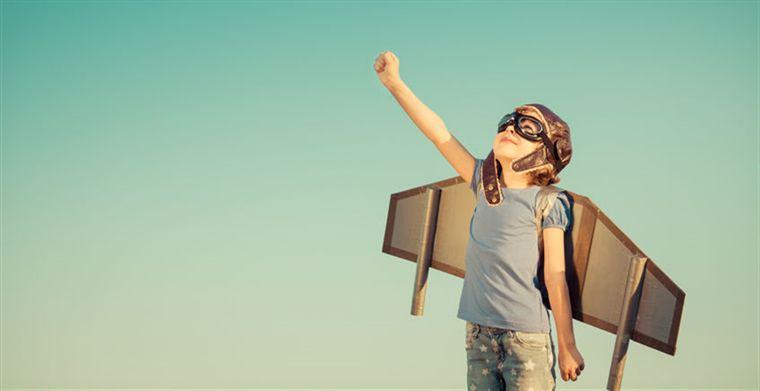
Data Profiling: Types, Techniques and Best Practices
APIs, or Application Programming Interfaces, serve as a set of rules and protocols that enable different software applications to communicate with one another. They play a pivotal role in modern software development by allowing developers to access and leverage the functionality and data of other applications or services. The concept is pretty simple, but what goes on behind the scenes? In short, the back and forth amongst applications occurs through API calls. What are API Calls? API calls are specific requests made by one software application to another. These requests serve as a means for the requesting application to access and utilize the functionality and data offered by the target application or service. API calls are essential for enabling communication and interaction between different software systems, allowing them to exchange information, execute tasks, and integrate seamlessly. These calls typically consist of a structured set of instructions, parameters, and data, which are processed by the target API to perform a particular operation, retrieve information, or trigger a specific response. Read More: How to Build an API Technicalities of an API Call Before we move on to how you can make an API call, let’s understand the technicalities of API calls: Client Application: The first component of an API call is the client application, which is the software that intends to make use of an API. It can be a web application, a mobile app, or any program that requires data or services from another source. API Endpoint: The client application needs to know the API’s endpoint(s). An endpoint is a specific URL (Uniform Resource Locator) that represents a particular resource or action provided by the API. For example, an API for weather data might have an endpoint like https://api.weather.com/current-weather. HTTP Request: To communicate with the API, the client application sends an HTTP request to the API endpoint. The request consists of several parts: HTTP Method: This specifies the type of action the client wants to perform. Common methods include: GET (retrieve data) POST (create data) PUT (update data) DELETE (remove data). Headers: These contain additional information about the request, such as the content type, authentication details, and more. Query Parameters or Request Body: Depending on the API and the specific endpoint, data can be sent as query parameters in the URL or as a JSON/XML payload in the request body. API Server: The API server is the software that listens for incoming requests at the specified endpoints. When a request is received, the server processes it based on the endpoint, method, and data provided. Request Processing: The API server processes the request by carrying out the intended action such as retrieving data from a database, performing calculations, or interacting with other services. Business Logic: The API often contains business logic, which defines how the data or service should be provided. For example, if you’re using a payment processing API, it might validate the payment data, charge the user, and return a response. Response Generation: After processing the request, the API server generates an HTTP response, which includes several components: Headers: These contain additional information about the response, such as content type and caching directives. Response Body: This is where the data or result of the request is included. It’s typically formatted as JSON, XML, HTML, or some other structured format. HTTP Status Code: It indicates the outcome of the request. HTTP Response: The API server sends the HTTP response back to the client application. Client Processing: The client application receives the response and processes it based on the HTTP status code and the data provided in the response body. Error Handling: If an error occurs during the API call (e.g., due to invalid input or server issues), the API server will return an appropriate status code (e.g., 400 for bad request or 500 for internal server error). The client application should handle and report these errors gracefully. Subsequent Actions: Depending on the nature of the client application, it may take further actions based on the API response, such as displaying data to the user or using the retrieved data in its own processes. Experience the Power of Well-Designed APIs Design efficient, secure, and developer-friendly APIs in LIKE.TG's no-code environment View Demo How to Make an API Call? Now that you have a basic understanding of the terms and how API call works, let’s see how you can make one: Read API documentation thoroughly When preparing to make API calls, it’s essential to thoroughly review the API documentation to ensure that you understand how to use the API effectively and adhere to any requirements or limitations. Here are the key pointers you should read in the API documentation before making the call: Understand the authentication method required to access the API. The documentation explaina whether you need API keys, OAuth tokens, or another form of authentication. Learn how to obtain and use these credentials. Identify the available API endpoints and their purposes. The documentation should provide the base URL and specific endpoint paths for each resource or action you want to access. Check for any rate limits or usage quotas imposed by the API. Understand how many requests you are allowed to make within specific time intervals. Be aware of any cost implications or the consequences of exceeding these limits. Check if the API documentation mentions versioning. Understand the API version you are working with and how to specify the version in your requests. Be aware of any upcoming changes or deprecated features. Determine if the API provider offers a sandbox or testing environment where you can experiment with the API without affecting production data. Choose the Right HTTP Method As explained earlier, the HTTP method you will choose for your API call will bebased on the action you want to perform. These HTTP methods, often referred to as CRUD operations (Create, Read, Update, Delete), provide a standardized way for clients to interact with APIs. What method you choose, depends on the action you want to perform. When working with RESTful APIs, these methods map to the basic operations that can be performed on resources, contributing to a clear and consistent API structure. Construct the API URL Build the URL for the API call by combining the base API URL and the specific endpoint you want to access. Here’s a breakdown of the considerations when making an API URL: The base URL is the starting point for the API. It typically includes the protocol (http or https) and the domain or server where the API is hosted. For example, the base URL is “https://api.example.com.” Next, you need to specify the endpoint, which comes right after the base URL and is often represented by a path. For example, if you want to retrieve user profiles, the endpoint might be “/user-profiles.” Then you need to add the query parameters that provide additional information to the API request. They are included in the URL after a question mark “?” and separated by “”. For instance, if you want to retrieve a specific user profile with an ID of 123, you might include a query parameter like “?user_id=123.” The final URL would look like: https://api.example.com/user-profiles?user_id=123 If the API requires authentication, you might need to include an authentication token or API key in the request, you can do it through headers or as part of the URL (e.g., “?api_key=your_api_key”). Putting it all together, your URL might look like: https://api.example.com/user-profiles?user_id=123. Set Up the Request Create an HTTP request in your programming language or API client. Set the following elements in your request: HTTP Method: Set the method corresponding to your intended action (e.g., GET, POST, PUT, DELETE). Headers: Include headers like “Content-Type” (specifying the data format of your request) and any required authorization headers. Query Parameters or Request Body: Include any data or parameters necessary for the API call. Depending on the API, data may be sent as query parameters in the URL or in the request body as JSON or another format.Receive the Response Here are some common types of response codes that you should know about: 2xx Success 200 OK: The request was successful. 201 Created: The request resulted in the creation of a new resource. 204 No Content: The request was successful, but there is no new information to send back (often used for DELETE requests). 3xx Redirection 301 Moved Permanently: The requested resource has been permanently moved to a new location. 302 Found (or 307 Temporary Redirect): The requested resource has been temporarily moved to a new location. 304 Not Modified: Used for caching purposes; the requested resource has not been modified since the last request. 4xx Client Errors 400 Bad Request: The server could not understand the request. 401 Unauthorized: The request lacks proper authentication credentials. 403 Forbidden: The server understood the request, but it refuses to authorize it. 404 Not Found: The requested resource could not be found on the server. 405 Method Not Allowed: The method specified in the request is not allowed for the resource identified by the request. 5xx Server Errors 500 Internal Server Error: A generic error message indicating that the server encountered an unexpected condition. 501 Not Implemented: The server does not support the functionality required to fulfill the request. 502 Bad Gateway: The server, while acting as a gateway or proxy, received an invalid response from an upstream server. 503 Service Unavailable: The server is currently unable to handle the request due to temporary overloading or maintenance. 429 Too Many Requests: The user has sent too many requests in a given amount of time. Ready to build, access, and consume APIs effortlessly? Discover, explore, and subscribe to public and private APIs with LIKE.TG's self-service API developer portal. View Demo How to Secure APIs from Invalid Calls? So, what happens when you are on the other end that is you are receiving API calls? You need to protect your APIs from unnecessary calls as Invalid calls may be attempts by malicious actors to exploit vulnerabilities in your system. Plus, they consume system resources, leading to degradation in performance or even denial of service. Here is how you can protect your APIs from unwanted API calls: Authentication: Use strong authentication mechanisms such as API keys, OAuth tokens, or JWT (JSON Web Tokens). Implement multi-factor authentication for additional security. Authorization: Enforce proper access controls to ensure that authenticated users only have access to the resources they are authorized to use. Implement role-based access control (RBAC) to manage permissions effectively. HTTPS (SSL/TLS): Always use HTTPS to encrypt data in transit and prevent eavesdropping. Use the latest and most secure versions of SSL/TLS protocols. Input Validation: Validate and sanitize all input data to prevent injection attacks such as SQL injection, cross-site scripting (XSS), and other common exploits. Rate Limiting: Implement rate limiting to prevent abuse and protect against brute force attacks. Limit the number of requests a client can make within a specific timeframe. API Keys: Use API keys to control access and track API usage. Rotate keys regularly and revoke access for compromised keys. Token Expiration: Set expiration times for tokens to limit their validity period. Refresh tokens should be used to obtain new access tokens. Logging and Monitoring: Implement robust logging to record API requests and responses. Monitor logs for suspicious activity and unauthorized access. Set up alerts for unusual patterns or security incidents. CORS (Cross-Origin Resource Sharing): Configure CORS settings to control which domains are allowed to access your API. This helps prevent cross-site request forgery (CSRF) attacks. API Gateway: Use an API gateway for centralized management of API security, including authentication, authorization, and monitoring. Web Application Firewall (WAF): Implement a WAF to protect against common web application attacks, such as SQL injection, XSS, and other security threats. Security Headers: Utilize security headers like Content Security Policy (CSP), Strict-Transport-Security (HSTS), and others to enhance the security of your API. Regular Security Audits and Penetration Testing: Conduct regular security audits and testing to identify vulnerabilities and address them proactively. API Versioning: Implement versioning for your APIs to ensure backward compatibility. This allows you to to deprecate and retire outdated versions with security vulnerabilities. Parting Words As technology continues to evolve, the role of APIs becomes increasingly pivotal in shaping the interconnected digital landscape. Whether you’re designing APIs for internal use or exposing them to external developers, a thoughtful and well-documented approach is key. If you want to design robust, powerful APIs in a code-free drag and drop environment, then try LIKE.TG API Management. The solution comes with a powerful API designer and integration capabilities all in one platform. Download free trial today.
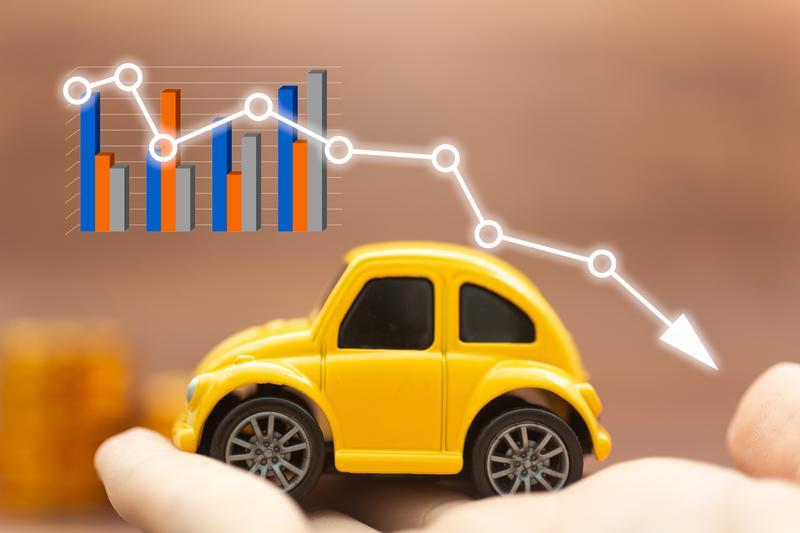
API Calls and How do They Work? A Complete Guide
APIs, or Application Programming Interfaces, serve as a set of rules and protocols that enable different software applications to communicate with one another. They play a pivotal role in modern software development by allowing developers to access and leverage the functionality and data of other applications or services. The concept is pretty simple, but what goes on behind the scenes? In short, the back and forth amongst applications occurs through API calls. What are API Calls? API calls are specific requests made by one software application to another. These requests serve as a means for the requesting application to access and utilize the functionality and data offered by the target application or service. API calls are essential for enabling communication and interaction between different software systems, allowing them to exchange information, execute tasks, and integrate seamlessly. These calls typically consist of a structured set of instructions, parameters, and data, which are processed by the target API to perform a particular operation, retrieve information, or trigger a specific response. Read More: How to Build an API Technicalities of an API Call Before we move on to how you can make an API call, let’s understand the technicalities of API calls: Client Application: The first component of an API call is the client application, which is the software that intends to make use of an API. It can be a web application, a mobile app, or any program that requires data or services from another source. API Endpoint: The client application needs to know the API’s endpoint(s). An endpoint is a specific URL (Uniform Resource Locator) that represents a particular resource or action provided by the API. For example, an API for weather data might have an endpoint like https://api.weather.com/current-weather. HTTP Request: To communicate with the API, the client application sends an HTTP request to the API endpoint. The request consists of several parts: HTTP Method: This specifies the type of action the client wants to perform. Common methods include: GET (retrieve data) POST (create data) PUT (update data) DELETE (remove data). Headers: These contain additional information about the request, such as the content type, authentication details, and more. Query Parameters or Request Body: Depending on the API and the specific endpoint, data can be sent as query parameters in the URL or as a JSON/XML payload in the request body. API Server: The API server is the software that listens for incoming requests at the specified endpoints. When a request is received, the server processes it based on the endpoint, method, and data provided. Request Processing: The API server processes the request by carrying out the intended action such as retrieving data from a database, performing calculations, or interacting with other services. Business Logic: The API often contains business logic, which defines how the data or service should be provided. For example, if you’re using a payment processing API, it might validate the payment data, charge the user, and return a response. Response Generation: After processing the request, the API server generates an HTTP response, which includes several components: Headers: These contain additional information about the response, such as content type and caching directives. Response Body: This is where the data or result of the request is included. It’s typically formatted as JSON, XML, HTML, or some other structured format. HTTP Status Code: It indicates the outcome of the request. HTTP Response: The API server sends the HTTP response back to the client application. Client Processing: The client application receives the response and processes it based on the HTTP status code and the data provided in the response body. Error Handling: If an error occurs during the API call (e.g., due to invalid input or server issues), the API server will return an appropriate status code (e.g., 400 for bad request or 500 for internal server error). The client application should handle and report these errors gracefully. Subsequent Actions: Depending on the nature of the client application, it may take further actions based on the API response, such as displaying data to the user or using the retrieved data in its own processes. Experience the Power of Well-Designed APIs Design efficient, secure, and developer-friendly APIs in LIKE.TG's no-code environment View Demo How to Make an API Call? Now that you have a basic understanding of the terms and how API call works, let’s see how you can make one: Read API documentation thoroughly When preparing to make API calls, it’s essential to thoroughly review the API documentation to ensure that you understand how to use the API effectively and adhere to any requirements or limitations. Here are the key pointers you should read in the API documentation before making the call: Understand the authentication method required to access the API. The documentation explaina whether you need API keys, OAuth tokens, or another form of authentication. Learn how to obtain and use these credentials. Identify the available API endpoints and their purposes. The documentation should provide the base URL and specific endpoint paths for each resource or action you want to access. Check for any rate limits or usage quotas imposed by the API. Understand how many requests you are allowed to make within specific time intervals. Be aware of any cost implications or the consequences of exceeding these limits. Check if the API documentation mentions versioning. Understand the API version you are working with and how to specify the version in your requests. Be aware of any upcoming changes or deprecated features. Determine if the API provider offers a sandbox or testing environment where you can experiment with the API without affecting production data. Choose the Right HTTP Method As explained earlier, the HTTP method you will choose for your API call will bebased on the action you want to perform. These HTTP methods, often referred to as CRUD operations (Create, Read, Update, Delete), provide a standardized way for clients to interact with APIs. What method you choose, depends on the action you want to perform. When working with RESTful APIs, these methods map to the basic operations that can be performed on resources, contributing to a clear and consistent API structure. Construct the API URL Build the URL for the API call by combining the base API URL and the specific endpoint you want to access. Here’s a breakdown of the considerations when making an API URL: The base URL is the starting point for the API. It typically includes the protocol (http or https) and the domain or server where the API is hosted. For example, the base URL is “https://api.example.com.” Next, you need to specify the endpoint, which comes right after the base URL and is often represented by a path. For example, if you want to retrieve user profiles, the endpoint might be “/user-profiles.” Then you need to add the query parameters that provide additional information to the API request. They are included in the URL after a question mark “?” and separated by “”. For instance, if you want to retrieve a specific user profile with an ID of 123, you might include a query parameter like “?user_id=123.” The final URL would look like: https://api.example.com/user-profiles?user_id=123 If the API requires authentication, you might need to include an authentication token or API key in the request, you can do it through headers or as part of the URL (e.g., “?api_key=your_api_key”). Putting it all together, your URL might look like: https://api.example.com/user-profiles?user_id=123. Set Up the Request Create an HTTP request in your programming language or API client. Set the following elements in your request: HTTP Method: Set the method corresponding to your intended action (e.g., GET, POST, PUT, DELETE). Headers: Include headers like “Content-Type” (specifying the data format of your request) and any required authorization headers. Query Parameters or Request Body: Include any data or parameters necessary for the API call. Depending on the API, data may be sent as query parameters in the URL or in the request body as JSON or another format.Receive the Response Here are some common types of response codes that you should know about: 2xx Success 200 OK: The request was successful. 201 Created: The request resulted in the creation of a new resource. 204 No Content: The request was successful, but there is no new information to send back (often used for DELETE requests). 3xx Redirection 301 Moved Permanently: The requested resource has been permanently moved to a new location. 302 Found (or 307 Temporary Redirect): The requested resource has been temporarily moved to a new location. 304 Not Modified: Used for caching purposes; the requested resource has not been modified since the last request. 4xx Client Errors 400 Bad Request: The server could not understand the request. 401 Unauthorized: The request lacks proper authentication credentials. 403 Forbidden: The server understood the request, but it refuses to authorize it. 404 Not Found: The requested resource could not be found on the server. 405 Method Not Allowed: The method specified in the request is not allowed for the resource identified by the request. 5xx Server Errors 500 Internal Server Error: A generic error message indicating that the server encountered an unexpected condition. 501 Not Implemented: The server does not support the functionality required to fulfill the request. 502 Bad Gateway: The server, while acting as a gateway or proxy, received an invalid response from an upstream server. 503 Service Unavailable: The server is currently unable to handle the request due to temporary overloading or maintenance. 429 Too Many Requests: The user has sent too many requests in a given amount of time. Ready to build, access, and consume APIs effortlessly? Discover, explore, and subscribe to public and private APIs with LIKE.TG's self-service API developer portal. View Demo How to Secure APIs from Invalid Calls? So, what happens when you are on the other end that is you are receiving API calls? You need to protect your APIs from unnecessary calls as Invalid calls may be attempts by malicious actors to exploit vulnerabilities in your system. Plus, they consume system resources, leading to degradation in performance or even denial of service. Here is how you can protect your APIs from unwanted API calls: Authentication: Use strong authentication mechanisms such as API keys, OAuth tokens, or JWT (JSON Web Tokens). Implement multi-factor authentication for additional security. Authorization: Enforce proper access controls to ensure that authenticated users only have access to the resources they are authorized to use. Implement role-based access control (RBAC) to manage permissions effectively. HTTPS (SSL/TLS): Always use HTTPS to encrypt data in transit and prevent eavesdropping. Use the latest and most secure versions of SSL/TLS protocols. Input Validation: Validate and sanitize all input data to prevent injection attacks such as SQL injection, cross-site scripting (XSS), and other common exploits. Rate Limiting: Implement rate limiting to prevent abuse and protect against brute force attacks. Limit the number of requests a client can make within a specific timeframe. API Keys: Use API keys to control access and track API usage. Rotate keys regularly and revoke access for compromised keys. Token Expiration: Set expiration times for tokens to limit their validity period. Refresh tokens should be used to obtain new access tokens. Logging and Monitoring: Implement robust logging to record API requests and responses. Monitor logs for suspicious activity and unauthorized access. Set up alerts for unusual patterns or security incidents. CORS (Cross-Origin Resource Sharing): Configure CORS settings to control which domains are allowed to access your API. This helps prevent cross-site request forgery (CSRF) attacks. API Gateway: Use an API gateway for centralized management of API security, including authentication, authorization, and monitoring. Web Application Firewall (WAF): Implement a WAF to protect against common web application attacks, such as SQL injection, XSS, and other security threats. Security Headers: Utilize security headers like Content Security Policy (CSP), Strict-Transport-Security (HSTS), and others to enhance the security of your API. Regular Security Audits and Penetration Testing: Conduct regular security audits and testing to identify vulnerabilities and address them proactively. API Versioning: Implement versioning for your APIs to ensure backward compatibility. This allows you to to deprecate and retire outdated versions with security vulnerabilities. Parting Words As technology continues to evolve, the role of APIs becomes increasingly pivotal in shaping the interconnected digital landscape. Whether you’re designing APIs for internal use or exposing them to external developers, a thoughtful and well-documented approach is key. If you want to design robust, powerful APIs in a code-free drag and drop environment, then try LIKE.TG API Management. The solution comes with a powerful API designer and integration capabilities all in one platform. Download free trial today.
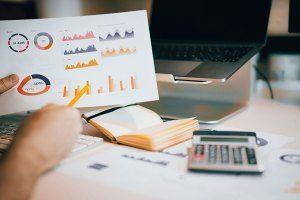
CRUD APIs: Benefits & Data Handling Techniques
CRUD APIs form the backbone of modern connectivity between digital systems. CRUD stands for Create, Read, Update, and Delete, and it’s a cycle meant to represent the fundamental operations you perform on your data. These APIs empower users to interact with software, serving as an important component for digital systems. If you’re using an application that allows you to read information and update it, it’s a CRUD app. Key Benefits of CRUD APIs CRUD operations are the cornerstone of many applications and systems, and they are often accessed through HTTP requests in RESTful APIs. The key benefits of CRUD APIs are: Simplicity: CRUD APIs are intuitive and straightforward to understand. They mirror the common operations you would perform on data, making them user-friendly for developers and clients. Consistency: CRUD APIs provide a consistent way to interact with data, reducing the learning curve and improving efficiency in development. Flexibility: These APIs are adaptable and can be used with various programming languages and platforms, promoting interoperability. These APIs are vital for data reliability and user satisfaction. Companies employ various CRUD API data handling techniques to ensure seamless interaction between systems and users. CRUD API Data Handling Techniques CRUD API data handling techniques are a set of practices and processes imperative to ensuring the efficiency, reliability, and usability of your APIs. These techniques include custom validation to maintain data integrity, data enrichment to enhance provided information , data transformations for seamless data exchange, and data pipelines for processing data efficiently. Let’s look at each of these techniques in more detail: Performing Custom Validation When designing CRUD APIs, custom validation plays a significant role. It involves checking data input and ensuring it adheres to your specific business rules. By implementing custom validation, you can prevent invalid or potentially harmful data from entering your system. This step ensures data integrity and enhances the reliability of your API. An e-commerce company may develop a CRUD API for processing product orders. They implement custom validation to check that the quantity of items ordered is within a reasonable range, ensure that the shipping address is complete and valid, and verify that the payment method used is supported by the system. This prevents orders with incorrect or potentially fraudulent data from being processed, maintaining data integrity and trust in your system. Enriching Your Data Data enrichment is all about enhancing the information you provide through your API. This can include adding related data, transforming data formats, or enriching data with additional details. The goal is to deliver comprehensive and valuable information to the end-users, simplifying their tasks and reducing the need for multiple API calls. For example, a weather information API provides current temperature data for a given location. To enhance the usability of this API, you could add additional information, such as weather forecasts for the next few days, historical weather data for that location, and even local points of interest. This enrichment provides users with a comprehensive weather-related dataset, reducing the need for users to make multiple API calls to gather related information. Performing Transformations Transformation means converting data from one format to another. For instance, you may need to transform data into JSON, XML, or other formats that best suit your clients. Proper data transformations can significantly reduce the complexity of client-side code and improve usability. A financial CRUD API might store data in a proprietary format within a system, while clients may prefer to interact with JSON data. To accommodate their preferences, you can implement transformations that convert your internal data into JSON format when responding to client requests. This conversion makes it easier for your clients to consume the data without needing to write complex code to interpret your proprietary format. Creating Data Pipelines Data pipelines are a sequence of data processing steps applied to incoming data. These pipelines can include data validation, transformations, and data enrichment. By creating efficient data pipelines, you ensure that the data you serve is of high quality and consistent. For a social media platform with a real-time feed API, data pipelines can be employed to process incoming posts before they are presented to users. In this case, the data pipeline might include steps like custom validation to check for inappropriate content, data transformations to format the post data for different user devices, and data enrichment to attach user avatars and relevant hashtags. By applying these steps in a sequence, you ensure that the data displayed in the feed is of high quality and consistency, enhancing the user experience. Why Are These Techniques Important for CRUD APIs? These data handling techniques are essential for CRUD APIs for several reasons: Data Quality: Custom validation and data enrichment help maintain data quality, ensuring that your API delivers reliable and accurate information. Usability: Handling data effectively makes your API more user-friendly. Clients can work with your API with ease, saving time and effort. Scalability: Proper data handling techniques can significantly improve the scalability of your CRUD API. It ensures that your API can handle increasing loads without sacrificing performance. Interoperability: These techniques make your API more adaptable to different programming languages and platforms, enhancing its versatility. Conclusion CRUD APIs are crucial because they provide a logical and efficient way to create, retrieve, update, and delete data, which is fundamental for managing and scaling operations effectively. The simplicity and standardization of CRUD APIs streamline development and integration processes, reducing complexity and costs. Speaking of API development, this area is evolving, and new solutions are emerging to simplify the process further. No-code API development solutions are becoming increasingly popular. These solutions enable users to design and deploy APIs with zero coding, making API development accessible to a broader audience, including those without extensive technical backgrounds. Simplify Developing CRUD APIs with LIKE.TG LIKE.TG offers a no-code API development solution that simplifies the CRUD API operations. Using its intuitive, drag-and-drop interface, users can auto-generate CRUD API endpoints for any database. Are you interested in building CRUD APIs? Contact us for a free demo or a free 14-day trial today.
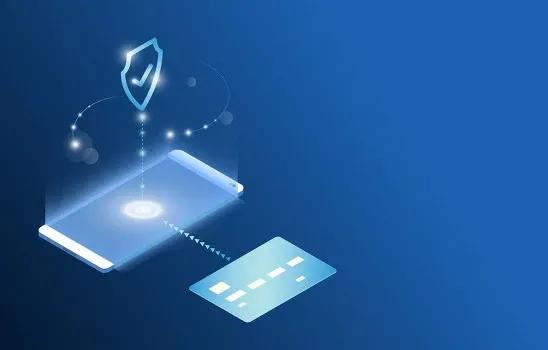
Modern Data Warehouse: Why Should You Migrate?
Data is the lifeblood of informed decision-making, and a modern data warehouse is its beating heart, where insights are born. The emphasis here is not on any data warehouse but a “modern” data warehouse that can keep up with the demands of today’s data challenges. The unprecedented rise in data volume and complexity has shown that the traditional infrastructure cannot suffice, which is why data warehouse modernization is an essential requirement. So, what do we mean by a modern data warehouse? You might be thinking it’s on the cloud. Yes, it is, but there is much more to it than just being on the cloud. In this blog, we will discuss everything about a modern data warehouse including why you should invest in one and how you can migrate your traditional infrastructure to a modern data warehouse. What is a Modern Data Warehouse? Simply put, a modern data warehouse is hosted over cloud as compared to traditional data warehouses, which are on-premises. So, what does the cloud have to offer? The most obvious benefit is scalability. The cloud data warehouse is highly scalable, which makes it ideal for processing an insane amount of data and performing extremely complex queries within minutes. The interesting part about a cloud data warehouse is the pay-as-you-go model. So, it is not only powerful and capable, but affordable as well. On top of it, many modern data warehouses separate storage from compute resources, so organizations can scale each component independently, which further improves cost efficiency and performance optimization. As far as the technical aspects are concerned, modern data warehouses do not follow a rigid schema, which means they can easily handle semi-structured and unstructured data. The best part about a modern data warehouse is that it comes with user-friendly tools and interfaces that enable business analysts and data scientists to explore and analyze data without requiring deep technical expertise. Modern Data Warehouse Architecture If you talk about traditional data warehouse architecture, they usually have a three-tier architecture, with data base server as the bottom layer, data storage layer as the middle layer and data processing as the final layer. The traditional architecture is hence limited to ETL/ELT and mostly star schema. Modern data warehouses, on the other hand, don’t have a particular structure. Each cloud data warehouse platform supports a different architecture. However, to generalize, a modern data warehouse can support: Massively Parallel Processing (MPP): It is a distributed computing architecture designed to process and analyze large volumes of data through parallelism. MPP architecture achieves parallelism by dividing data and tasks into smaller, manageable units that can be processed simultaneously across multiple nodes. Each node is equipped with its own processing power and memory. MPP systems often employ load balancing to distribute query workloads evenly across nodes to prevent any single node from becoming a performance bottleneck. Due to the scalability, MPP systems can adapt to the evolving data needs of businesses, which is why they are well-suited for dynamic environments where data is constantly growing and evolving. Lambda Architecture: The Lambda Architecture aims to provide a robust and fault-tolerant solution for processing both batch and real-time data in a scalable way. The architecture is divided into different layers including: Batch Layer: This layer is responsible for handling historical or batch data processing. Speed Layer: The Speed Layer deals with real-time data processing and is responsible for handling data as it arrives. It is designed to process data with very low latency for real-time insights. Serving Layer: The Serving Layer is where the results from both the Batch and Speed Layers are stored and made available for querying. This layer provides a consolidated view of processed data. The Presentation Layer represents the top layer of the Lambda Architecture. It is where data is presented to end-users through various applications, including dashboards, reports, and APIs. Hybrid Architecture: A hybrid architecture in the context of a modern data warehouse refers to a design that combines both on-premises and cloud-based components to meet an organization’s data processing and analytics needs. The core of the hybrid architecture is a cloud data warehouse, which provides scalability, flexibility, and the ability to efficiently process and store large volumes of data. However, organizations maintain some of their data sources and systems on their own physical infrastructure. These on-premises data sources can include legacy databases, proprietary systems, and sensitive data that needs to be kept in-house for compliance or security reasons. Migrate to a Modern Data Warehouse With Zero Coding 14-Day Free Trial Traditional Vs Modern Data Warehouse When it comes to the differences between a traditional and a modern data warehouse, it is not just about being on cloud or on-premises. Here are some more differences between the two: 1. Data Processing Paradigm Traditional data warehouses are typically batch-oriented. They process and load data in scheduled, periodic batches, so data is not available for analysis until after it has been processed and loaded, leading to a latency in data availability. Modern data warehouses, on the other hand, emphasize real-time or near-real-time data processing. They enable continuous data streaming and support both batch and stream processing, which provide faster access to up-to-the-minute insights. 2. Scalability Scaling traditional data warehouses can be a complex and expensive process because they often require significant upfront investments in hardware and infrastructure. Cloud data warehouses are are highly scalable and allow you to easily scale up or down based on their needs, which is particularly valuable for handling variable workloads and accommodating growing data volumes. 3. Data Integration Data integration in traditional data warehouses can be complex and time-consuming, as it involves extensive ETL (Extract, Transform, Load) processes to prepare data for analysis. Data from different sources often needs to be transformed to fit into a common schema. Modern data warehouses often provide built-in data integration and transformation capabilities, which simplifies the process of ingesting, cleansing, and harmonizing data from various sources. 4. Data Storage and Cost: Traditional data warehouses rely on specialized, expensive hardware and on-premises infrastructure. Organizations are responsible for managing and maintaining the hardware, which results in high operational costs. Modern ata warehouses operate on a pay-as-you-go model, where you only pay for the resources you consume, resulting in cost savings and more predictable expenses. 5. Schema Flexibility One of the most important differences between the two is that traditional data warehouses often use fixed and rigid schemas. Any changes in the data structure or schema require significant effort and can disrupt ongoing operations. In contrast, modern data warehouses offer more schema flexibility, and can support semi-structured and unstructured data. Aspect Traditional Data Warehouse Modern Data Warehouse Data Processing Paradigm Batch processing Real-time and batch processing Scalability Often difficult and expensive to scale Easily scalable, pay-as-you-go model Data Integration Complex ETL processes for data integration Built-in data integration and transformation Data Storage and Cost On-premises hardware with high operational costs Cloud-based, pay-as-you-go model, cost-effective Schema Flexibility Rigid, fixed schemas More flexible, supports semi-structured and unstructured data Security and Compliance Organization’s responsibility Cloud providers often offer robust security and compliance features User Accessibility and Self-Service Typically requires IT involvement Promotes self-service analytics, greater user accessibility Now that you know the different architectures, let’s look at some of the specific modern cloud data warehouses in the market and their architectures. Read More: Data warehouse Cost Estimation New Data Warehouses Architecture Amazon Redshift Source Amazon Redshift harnesses the power of a massively parallel processing (MPP) architecture, where data is stored in slices using a columnar format. In this architecture, each node is equipped with its dedicated storage, RAM, and computational resources. Redshift primarily operates through two types of nodes: leader nodes and compute nodes. The leader node takes charge of accepting queries and then delegates their execution to the compute nodes, which carry out the data processing tasks. One of the key advantages of this MPP setup is its ability to allow compute nodes to concurrently process data across various slices, resulting in robust query performance. These compute nodes subsequently return the query results to the leader nodes, which consolidate and prepare the data for client-side applications to utilize. What’s especially convenient is that you can seamlessly connect with Redshift using a variety of business intelligence (BI) or analytics tools. Read More: 3 Ways to Transfer Data from Amazon S3 to Redshift Google Big Query Google BigQuery operates on a serverless architecture that lets Google dynamically manage the allocation of machine resources and keep resource management decisions hidden from you. One of the standout features of BigQuery is its versatility in data handling. You can load data from various sources, including Google Cloud Storage and other readable data repositories. Alternatively, you can opt for real-time data streaming, which enables the incremental addition of data row-by-row as it becomes available. At the heart of BigQuery’s architecture is Dremel, a high-performance, massively parallel query engine Dremel employs a columnar data structure, akin to Redshift, and leverages a tree architecture to efficiently dispatch queries among thousands of machines, resulting in rapid response times. Read More: BigQuery vs. Redshift: Which One Should You Choose? Snowflake Source Snowflake’s architecture also follows a cluster and node-based approach. However, the major difference in the architecture is that it that separates compute capabilities from storage. So, when data is not actively in use, Snowflake effectively relocates it to a more cost-efficient storage area. This approach optimizes resource utilization as it avoids the unnecessary use of storage areas during computation. Moreover, the decoupling of storage from compute offers the ability to efficiently scale resources up or down as needed, surpassing the capacities of traditional on-premises data warehouses. "Building a data warehouse is no longer exclusively for IT coders and coders." Take the lead now! Benefits of Modern Data Warehousing Up till now, we have established the fact that modern data warehouses are on the cloud and they are way better and capable that traditional data warehouses. But is modern data warehousing even worth it? Should you even consider disrupting your entire architecture to migrate to a modern one? Here are some benefits that you should consider if you are stuck with the dilemma: Cost Efficiency: While migrating to a new architecture would be a time consuming and cost intensive initially, but you need to think long term. Cloud-based modern data warehouses often operate on a pay-as-you-go model, so there is no need for upfront investments in hardware and infrastructure. This model reduces total cost of ownership and predictable expenses. Global Reach: Traditional data warehouses cannot beat this benefit at any cost. Cloud providers have data centers in various regions, so you can store and process data closer to their target audience, which Improves user experience and ultimately leads to potential revenue gains High Performance: Many cloud data warehouses are designed for high-speed querying and analytics, offering impressive performance for complex analytical workloads. Faster analytics mean quicker insights and quicker decision making. Agility: Modern data warehouses offer rapid provisioning, so you can set up and start using the environment quickly. The agility is especially important for organizations that need to respond to changing data and analytics demands promptly. Security: Now this is a debatable benefit. Often the argument is that on-premises architecture is safer for sensitive data. However, cloud providers invest heavily in security measures, including data encryption, access controls, and compliance certifications. Your data is stored in highly secure data centers, which reduces the risk of data breaches. How to Migrate? Migrating to a modern data warehouse is a strategic process that requires careful planning and execution. Here’s a more detailed step-by-step guide for a successful migration: Assessment and Planning:Conduct a comprehensive assessment of your current data infrastructure, including databases, data sources, and processing systems. Identify pain points, scalability limitations, and areas that need improvement.Define clear objectives for the migration. Determine how the modern data warehouse aligns with your organization’s business goals. Select the Right Modern Data Warehouse:Research and select a modern data warehouse solution that best suits your organization’s needs. Consider factors such as scalability, integration capabilities, pricing, and data processing features. Data Profiling and Cleansing:Before migration, perform data profiling and cleansing to ensure data quality. Address issues such as duplicates, inconsistencies, and inaccuracies in your data. Data Mapping and Transformation:Map your existing data structures to the schema of the modern data warehouse. You may need to transform data to match the new schema requirements. Data Migration:Execute the data migration plan by extracting data from current systems, transforming it as needed, and loading it into the modern data warehouse. Use ETL (Extract, Transform, Load) tools where necessary. Integration with Data Sources:Configure data integration pipelines to establish smooth data flow from diverse sources into the modern data warehouse. Implement connectors and pipelines for automated data ingestion. Testing and Validation:Conduct rigorous testing to ensure the accuracy and completeness of the migrated data. Verify that the data warehouse processes queries and analytics correctly and maintains data integrity. Performance Optimization:Optimize the performance of the modern data warehouse by adjusting configurations, indexing, and query optimization. Ensure efficient query execution. Security and Access Control:Implement robust security measures, including role-based access controls, encryption, and compliance with data privacy regulations. Safeguard data in transit and at rest Build Your Data Warehouse with LIKE.TG Data Warehouse Builder Want to migrate to a modern data warehouse? LIKE.TG DW Builder can help. It is a comprehensive data warehousing solution that streamlines the creation and implementation of data warehouses without the need for coding. It employs a meta-driven method that allows you to work with data through an extensive range of pre-built transformations, eliminating the need for intricate ETL or SQL scripting. Download 14-day free trial today and move to a cloud data warehouse without any hassle!.

The 10 Best API Management Tools for 2024
The reliance on APIs is increasing for businesses striving to stay digitally competitive. However, creating and managing APIs is not a simple task. As your business evolves, the demand for scalable, secure, and well-documented APIs intensifies, adding to the already high pressure on your development team. The consequences of ineffective API management are tangible—delays in project timelines, security vulnerabilities, and missed opportunities for innovation that can propel your business forward. Enter API management tools. This blog covers the top API management software in the market. It also provides guidelines to help you select the best API solution based on your use case. First, let’s quickly cover the basics of API management tools and their benefits for data teams. What is API Management? API management is the systematic process of creating, overseeing, and securing APIs to ensure efficient and secure communication between different software applications. It involves a set of tools and practices that facilitate the development, deployment, and monitoring of APIs throughout their lifecycle. API management encompasses tasks such as defining API specifications, handling authentication and authorization, managing traffic and usage, and monitoring API performance. Effective API management is crucial for businesses that seek to optimize data accessibility and interoperability. What are API Management Tools? API management tools help users design, create, test, monitor, and deploy APIs. They help data teams configure the entire API lifecycle within a single platform. Some key functionalities of API management solutions include: API Design and Creation: API tools assist in designing API specifications, defining endpoints, and creating the necessary documentation for developers to understand how to interact with the API. Security and Authentication: API management tools provide mechanisms for securing APIs, implementing authentication, and controlling access through methods such as API keys, OAuth, or other authentication protocols. Traffic Management: These tools enable you to monitor and control the flow of data between APIs and applications, preventing overload or misuse, and ensuring optimal performance. Monitoring and Analytics: API management solutions offer real-time monitoring and analytics capabilities, allowing you to track API usage, identify performance bottlenecks, and gain insights into user behavior. Benefits of Using API Management Tools API Management tools greatly simplify API creation, deployment, and administration for businesses. They provide an array of benefits, such as secure data sharing, faster time-to-insight, and increased scalability. Secure Data Sharing APIs allow you to share critical enterprise data with internal and external partners, so maintaining security is one of the most important activities of any API management initiative. According to a Postman survey involving 37,000 developers and API professionals, 20% of respondents reported that API security incidents or breaches happen at least once every month at their organizations. API management solutions help ensure that your APIs and data remain protected. An API management tool also gives insights into real-time API activity, which allows you to identify potential security vulnerabilities in networks, operating systems, and API components beforehand. An Agile Approach to Development API management enables you to design, test, publish, manage, and analyze all APIs in a single platform. Many API management solutions are fully automated, saving you time with manual tasks such as testing, deployment, or generating documentation. Additionally, API management tools improve API usability so you can rapidly launch new initiatives to support changing business requirements. Automation Equals to Time Saving Developers spend a considerable amount of their time managing APIs. According to the 2022 Postman State of API Report, over 40% of developers spend more than 20 hours a week working with APIs — almost a third of which is spent creating APIs through coding and programming. The manual work can be an enormous burden on IT resources, especially when managing APIs on a large scale. Automated API management solutions, such as LIKE.TG’s, accelerate API creation and publishing by automating most activities, like testing, error handling, pagination, etc. Insight-Driven API Development A crucial part of API management is API monitoring. A modern tool API tool comes with dashboards and logging mechanisms that give you an overview of key API metrics such as runtime statistics, API traffic, response times, and spikes. Additionally, it simplifies debugging by giving you detailed logs of processes and errors. You can use this data and insights to troubleshoot issues and plan for future API development. Maintain API History with Versioning As trends and requirements change, APIs need to evolve. An API management solution ensures that you can make changes to your APIs without disrupting the underlying system. API management tools also have governance features that make it easier to keep a tab on different versions of APIs. Now that we’ve covered the benefits, let’s jump straight into the top future-proof API tools in the market. The 10 Best API Management Tools for 2024 LIKE.TG LIKE.TG API Management is the complete solution for full API lifecycle management. With its robust built-in functions and an intuitive code-free interface, LIKE.TG API Management makes building and managing APIs a breeze. Here is how you can take control of the entire API lifecycle with LIKE.TG API Management: API Consumption: You can easily mash and consume APIs in LIKE.TG API Management. The API consumption component supports multiple authentication types, HTTP methods, and Open API metadata support. API Designer: The API Designer allows you to design and deploy API in minutes within a drag-and-drop interface. You can also test and validate results in real-time with Instant Preview and deploy APIs with one click. Leverage LIKE.TG’s wide array of pre-made components like connectors, transformations, data quality checks, and input/output settings to swiftly build and automate API Pipelines for applications dealing with large volumes of data. API Testing: You can test APIs at every stage of the process with the Instant Preview feature. You can also use the automatic documentation capability to export your API collection to an outside tool for testing. API Security: LIKE.TG API Management provides Oauth2 and bearer token authorization to ensure your API services are protected properly. You can define access roles. Authorization can be defined at any level of the endpoint hierarchy, up to and including the individual endpoint. Leverage LIKE.TG’s built-in security framework to ensure the protection of your API services and enable secure data sharing with stakeholders. API Publishing: APIs can be published as single endpoints or en masse for product deployment. With LIKE.TG API Management, you can publish your APIs in just a few clicks. All deployed APIs automatically generate Open API documentation which can be viewed in browser or exported as a collection to any API testing or consuming platform. API Monitoring: You can effortlessly visualize APIs and gain valuable business insights from your API data with the live dashboard in LIKE.TG API Management. Additionally, access a complete overview of your APIs via detailed API reports and logs. Postman Postman is another API development and management platform that provides a comprehensive set of tools for designing, building, testing, and deploying APIs. It offers a user-friendly graphical interface, support for various API formats, and a wide range of features that cater to both individual developers and enterprise teams. Key API Management features of Postman are: Centralized API repository: Postman serves as a central hub for managing your API collection, documentation, tests, and other related artifacts. It allows you to organize your APIs into workspaces, making it easy to share and collaborate with team members. API design and documentation: Postman provides tools for designing and documenting your APIs in a structured and clear manner. You can use the built-in editor to create OpenAPI specifications (OAS), which define the structure and capabilities of your APIs. API testing and monitoring: Postman offers a powerful testing framework for validating the functionality and performance of your APIs. You can create automated tests using JavaScript or the Postman Sandbox, a mock API server that simulates responses to your API requests. API deployment and monitoring: Once your APIs are developed and tested, you can deploy them to a production environment using Postman’s deployment tools. Postman also provides monitoring capabilities to track API usage and performance metrics. Apigee Apigee is a comprehensive API management platform that offers a wide range of features for designing, building, deploying, and securing APIs. It is a cloud-based platform that is part of Google Cloud Platform (GCP). Key features of Apigee include: A visual API design tool that allows you to easily create and configure API proxies. API proxies act as intermediaries between your backend services and API consumers, allowing you to add security, transformation, and other policies to your APIs. Automatically deploy your APIs to multiple environments, including production, staging, and development. It can also scale your APIs up or down automatically based on traffic demand. Multiple security features, including authentication, authorization, rate limiting, and data encryption. You can also use Apigee to protect your APIs from common web application attacks, such as SQL injection and cross-site scripting (XSS). Real-time analytics and monitoring for your APIs. You can track API usage, performance, and errors. You can also use Apigee to identify and troubleshoot API issues. Mulesoft MuleSoft is a software company that provides a platform for connecting applications, data, and devices. It is best known for its Anypoint Platform, a cloud-based integration platform. Key API Management features of MuleSoft Anypoint Platform: A visual API design tool that allows you to easily create and configure API proxies. Ability to automatically deploy your APIs to multiple environments, including production, staging, and development. It can also scale your APIs up or down automatically based on traffic demand. A wide range of security features, including authentication, authorization, rate limiting, and data encryption. You can also use MuleSoft Anypoint Platform to protect your APIs from common web application attacks, such as SQL injection and cross-site scripting (XSS). A developer portal that allows you to provide a self-service portal for your API consumers. Governance features that allow you to control access to your APIs and enforce usage policies. You can define access control lists (ACLs), set usage quotas, and monitor API usage to ensure that your APIs are being used in a compliant manner. SwaggerHub SwaggerHub is a cloud-based API development and management platform that provides a comprehensive set of tools for managing APIs. SwaggerHub provides: A central hub for managing your API collection, documentation, tests, and other related artifacts. It allows you to organize your APIs into workspaces, making it easy to share and collaborate with team members. Tools for designing and documenting your APIs in a structured and clear manner. You can use the built-in editor to create OpenAPI specifications (OAS). A powerful testing framework for validating the functionality and performance of your APIs. You can create automated tests using JavaScript or the SwaggerHub Explore tool, which allows you to interact with your APIs directly in the browser. Once your APIs are developed and tested, you can deploy them to a production environment using SwaggerHub’s deployment tools. SwaggerHub also provides monitoring capabilities to track API usage and performance metrics. Apidog Apidog is a free API development tool. It is a cloud-based platform that offers a user-friendly interface and a wide range of features that cater to both individual developers and enterprise teams. Apidog streamlines the API lifecycle through: Options for designing and documenting your APIs in a structured and clear manner. You can use the built-in editor to create and maintain your API documentation, which can be exported to various formats, such as OpenAPI, Markdown, and HTML. A testing framework for validating the functionality and performance of your APIs. You can create automated tests using JavaScript or Python. API deployment and monitoring. Once your APIs are developed and tested, you can deploy them to a production environment using Apidog’s deployment tools. Apidog also provides monitoring capabilities to track API usage, performance, and errors. Streamlined collaboration and sharing among API developers and consumers by enabling real-time editing, commenting, and version control for API collections. Integration with various third-party tools and services, such as CI/CD pipelines, testing frameworks, and version control systems. Kong Kong is an open-source API gateway that is a popular choice for managing APIs due to its flexibility, scalability, and ease of use. It is a cloud-native platform that can be deployed on-premises, in the cloud, or in a hybrid environment. Key API Management features of Kong are: Efficient routing of incoming API requests to the appropriate backend services based on various criteria, such as URLs, headers, and methods. It also provides features for load balancing, rate limiting, and circuit breaking to ensure the availability and performance of your APIs. Data transformation and manipulation using plugins. This enables you to perform tasks such as sanitization, validation, and encryption, ensuring the integrity and security of your data. Comprehensive monitoring and observability features to track API usage, performance, and errors. You can collect metrics, generate logs, and configure alerts to gain insights into the health and behavior of your APIs. A rich ecosystem of plugins that extend its functionality and enable you to integrate it with various third-party tools and services. This makes Kong highly customizable and adaptable to diverse use cases. Amazon API Gateway Amazon API Gateway is a fully managed service that makes it easy for developers to manage APIs at any scale. Amazon API Gateway allows users to: Create and configure REST APIs and WebSocket APIs. Secure APIs with authentication and authorization. API Gateway provides a range of authentication mechanisms to secure your APIs, including API keys, IAM policies, and Amazon Cognito user pools. Real-time monitoring of API usage, including metrics for request volume, latency, and errors. You can also use CloudTrail to track API changes and CloudWatch to log API requests and responses. Automatically scale your APIs up or down based on traffic demand. This ensures that your APIs are always available and responsive. IBM API Connect IBM API Connect provides a wide range of features for designing, building, testing, deploying, and securing APIs across multiple environments. It is a hybrid platform that can be deployed on-premises, in the cloud, or hybrid. Key features include: API deployment and scaling. IBM API Connect can automatically deploy your APIs to multiple environments, including production, staging, and development. It can also scale your APIs up or down automatically based on traffic demand. Security features, including authentication, authorization, rate limiting, and data encryption. You can also use IBM API Connect to protect your APIs from common web application attacks, such as SQL injection and cross-site scripting (XSS). A developer portal that allows you to provide a self-service portal for your API consumers. The developer portal can include documentation, tutorials, and sandboxes for testing your APIs. API monetization features, such as usage-based pricing and subscription billing. You can use IBM API Connect to charge your API consumers for access to your APIs. Data integration and transformation capabilities to connect your APIs to various data sources and transform data formats before sending it to your API consumers. Support for microservices architectures, allowing you to manage and deploy APIs for microservices-based applications. How to Select the Best API Management Tool for Your Organization? You know the best API tools in the market and their benefits now. However, before opting for a tool you should consider how the tools match your specific requirements, in addition to their product features. Here are some points to help streamline the decision to invest in API software: Define Your Requirements: Clearly outline your business goals and the specific requirements for API management. Consider aspects such as the number and complexity of APIs, security needs, scalability requirements, integration capabilities, and the level of analytics and monitoring you require. Evaluate Features: Compare the features offered by different API management tools. Look for essential capabilities such as API design and creation, security and authentication options, and traffic management. Scalability: Assess the scalability of the API management tool. Consider whether it can handle your current API demands and easily scale to accommodate future growth in terms of API volume, users, and data. Cost Considerations: Understand the pricing model of each API management tool. Consider factors such as licensing fees, subscription models, and any additional costs for features or usage beyond certain thresholds. Community and Support: Assess the community and support options associated with each tool. A strong user community and reliable support services can be valuable resources when facing challenges or seeking guidance. Trial and Testing: Whenever possible, take advantage of trial versions or free tiers to test the API management tools. This hands-on experience will give you a better understanding of how well the tool meets your requirements in a real-world scenario. Vendor Reputation: Consider the reputation and track record of the API management tool vendor. Reviews, testimonials, and case studies can provide insights into the experiences of other organizations using the tool. You can check out reviews for major providers on websites like G2, Capterra, and Softwaretestinghelp. LIKE.TG Simplifies Full-Cycle API Management LIKE.TG API Management offers a comprehensive suite of features to streamline the entire API lifecycle—powered by a no-code approach. It an intuitive interface, real-time monitoring, no-code building, one-click testing and data visualization capabilities to make API management a breeze. Plus, LIKE.TG’s excellent support will enable you to set up and push APIs in no time at all! Streamline your API initiatives now – with a free 14-day trial!

Introducing Cloud File Drop Automation with LIKE.TG
Cloud adoption has risen significantly in the past few years due to digital transformation initiatives. Businesses are migrating their operations to the cloud due to its enhanced scalability, flexibility, and agility. Gartner predicts that by 2025, over 85% of organizations will adopt a cloud-first principle. The Shift to Cloud Computing The global business landscape is witnessing a significant shift towards cloud computing, driven by its myriad benefits. Let’s examine some key factors that make cloud computing an ideal solution for your business. Scalability: Cloud computing offers you the ability to scale resources up or down based on demand, which reduces the need for upfront investments, and accommodates fluctuating workloads. Accessibility: Cloud services can be accessed from anywhere with an internet connection, promoting remote work, global collaboration, and expansion. Disaster Recovery: Cloud providers offer robust data backup and disaster recovery solutions, reducing the risk of data loss and ensuring business continuity. Understanding Cloud File Drop Automation File Drop Automation is a process that automates workflows based on the addition of new files to a specific folder. This means that when a file is dropped into a designated folder, a predefined workflow is triggered automatically, eliminating the need for manual intervention. As the adoption of popular cloud services such as Amazon S3 and Microsoft Azure Blob Storage is on the rise, this functionality plays an even more important role. It involves the automation of processes when files are added to these cloud-based folders. This includes data processing, data integration, and even data analysis tasks, ensuring that the data is readily available for use across various platforms and applications. For instance, your business might set up a workflow that automatically processes sales data whenever a new sales report is added to a specific Amazon S3 bucket. Or you might use Microsoft Azure Blob Storage to store customer feedback forms, with a workflow set up to automatically analyze and categorize this feedback whenever a new form is added. By automating these processes, your business can ensure that its data is processed quickly and accurately, allowing you to make timely, data-driven decisions. Cloud File Drop Automation can benefit your organization through: Enhanced Efficiency: File Drop Automation significantly reduces the time and effort required to initiate and execute workflows. By automatically triggering processes when a new file is added, it frees up resources for other critical tasks. Improved Accuracy: With automation, the risk of errors associated with manual processes is significantly reduced, ensuring that the right processes are triggered by the right files at the right time. Cost-Effective: By reducing the need for manual intervention and minimizing errors, File Drop Automation can lead to significant cost savings in the long run. Real-Time Processing: File Drop Automation allows for real-time processing of data as soon as it is dropped into the cloud-based folder, enabling businesses to make timely decisions based on the most recent data. Enhanced Collaboration: With files automatically processed and available in the cloud, collaboration among team members located in different geographical locations becomes easier and more efficient. Cloud File Drop Automation with LIKE.TG LIKE.TG makes it possible for you to ingest data from the cloud without spending hours in manual coding and other processes. Simply leverage the file drop automation feature to automate the processing of files in cloud-based sources such as FTP, Amazon S3, and Microsoft Azure Blob Storage. This streamlined automation enhances efficiency and reduces the risk of errors, which makes data management more accurate and reliable. How LIKE.TG File Drop Automation Works LIKE.TG allows you to set up a system that automatically detects when a file is dropped into a specified cloud directory, processes the data, and transfers it to a designated destination. This not only simplifies data management but also ensures that your data is always up-to-date and ready for analysis. To utilize the cloud browser support in File Drop, you need to initially deploy the shared connection via the catalog. Once this is done, you can schedule the process to automate the workflow. This enhancement streamlines the process and makes it more efficient, further empowering businesses to leverage the power of the cloud. Here is a step-by-step guide on how to effectively use this feature: As a first step in this process, create a Shared Action containing a Cloud Connection to deploy it via the catalog. Next, click on enable for catalog option and proceed to deploy the Shared Action. Once the connection is successfully deployed and added to the catalog, check if the shared action is visible in the Connections section of the Resource Catalog to ensure it has been saved. Next, open your dataflow. Here, we are writing data from a delimited source to an Excel destination. The file in the source is extracted from the variable where the FilePath is defined. The variable here comes from the workflow, where the ContextInfo is typically used with the “Dropped File Path” frequency. Now close the project and open the Scheduler. Here, amongst the Frequency options choose “When File is Dropped” and then select Browse Cloud Folders. A dialog box will appear displaying your deployed Cloud Connection. Select any folder and set it as the directory to watch. Enable polling and set the time interval to 30 seconds. This will ensure that the directory is checked every 30 seconds to see if a file has been dropped. Finally, save the scheduler settings and drop a file in the designated folder to verify its functionality. Final Words As businesses increasingly rely on data for informed decision-making, the importance of file drop automation in the cloud becomes evident. This feature equips you with a competitive edge in managing the rising volume of information while promoting agility, adaptability, and efficiency in the dynamic business landscape. LIKE.TG’s file drop automation of cloud-based sources is an efficient and user-friendly feature that can significantly enhance your data management and transfer processes. By utilizing it, you can not only save valuable time but also reduce the likelihood of errors, ultimately leading to a boost in productivity. To experience the benefits of this feature firsthand, download LIKE.TG’s 14-day free trial today! Ready for a Workflow Upgrade? Leverage LIKE.TG's new Cloud File Drop Automation to seamlessly process files dropped into your cloud-based folders. Effortlessly manage files and enhance workflow efficiency in just a few clicks. Try it Now!
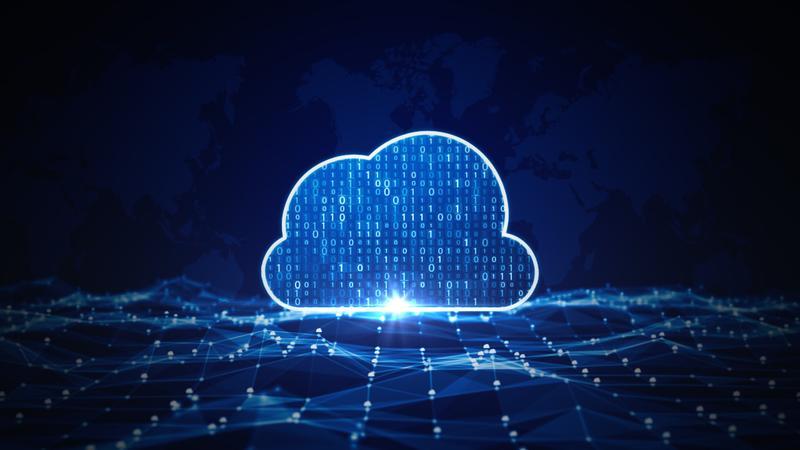
API Development: Best Practices and Strategies
API development facilitates efficient data sharing, enhances interoperability between systems, and drives digital ecosystem growth. APIs are at the core of modern applications and systems. In fact, 56% of enterprise leaders agree that APIs help them build better digital experiences and products. So, how do you develop APIs correctly, ensuring they seamlessly connect to the digital world and drive the growth of digital ecosystems? The answer lies in a series of best practices and strategies that pave the way for effective and user-friendly API development. Understanding API Development An API, or Application Programming Interface, serves as a bridge between different software systems, enabling them to communicate and share data seamlessly. APIs come in many shapes and sizes, from RESTful web services to SOAP endpoints, but the principles for creating effective APIs remain fairly consistent. Best Practices and Strategies for API Development 1. Define Clear API Objectives and Goals Every successful API starts with a clear purpose. Define the objectives and goals of your API project before writing a single line of code. What problem does your API solve, and who are the intended users? Having a well-defined scope helps you avoid feature creep, i.e., excessive features that make an API too complicated or difficult to consume, and ensures your API serves its intended purpose effectively. 2. Use Meaningful and Consistent Naming Conventions Choosing meaningful and consistent naming conventions for your API endpoints, methods, and data structures is essential. It makes your API more intuitive and user-friendly, reducing the learning curve for developers interacting with it. Be concise and maintain a standardized approach to naming. 3. Version Your APIs to Maintain Backward Compatibility As your API evolves, it’s critical to maintain backward compatibility with existing clients. Versioning your API allows you to make changes and improvements without breaking the functionality for users of older versions. Follow a clear versioning strategy (e.g., using URLs or headers) to manage these changes effectively. 4. Implement Proper Authentication and Authorization Mechanisms Security should be a top priority in API development. Implement robust authentication and authorization mechanisms to ensure that only authorized users can access your API’s endpoints. Options include OAuth, API keys, and JWT tokens, depending on your application’s requirements. 5. Document Your APIs Comprehensively Comprehensive and well-maintained documentation is the lifeblood of any API. It serves as a reference guide for developers, making it easier for them to understand how to use your API effectively. Include clear examples, use cases, and details about endpoints, requests, and response formats. 6. Provide Descriptive Error Messages and Status Codes Users should receive informative error messages and status codes when something goes wrong in an API request. Well-documented error responses help developers quickly identify and resolve issues, improving the overall user experience. 7. Validate Input Data and Handle Validation Errors Input data validation is essential for the security and reliability of your API. Validate user input to prevent common security vulnerabilities like SQL injection and cross-site scripting. Ensure that you handle validation errors and provide meaningful feedback to users. 8. Optimize API Endpoints for Performance and Scalability API performance can significantly impact the user experience. Optimize your API endpoints for speed and scalability. Use techniques like caching, pagination, and asynchronous processing to enhance performance while ensuring your API can handle increasing loads of user requests. 9. Implement Rate Limiting and Throttling to Prevent Abuse To prevent abuse or overuse of your API, implement rate limiting and throttling. Set limits on the number of API requests a user or client can make within a specific time frame. This helps maintain fair usage and protects your API from abuse. 10. Use Appropriate HTTP Methods (GET, POST, PUT, DELETE) for CRUD Operations Follow the HTTP methods (GET, POST, PUT, DELETE) convention for CRUD (Create, Read, Update, Delete) operations. This aligns your API with standard practices, making it more intuitive for other developers to work with. Understanding the Limitations Technical Complexity: API development demands expertise in various programming languages and protocols. Resource Intensive: Building and maintaining APIs can require significant resources, including development time, server capacity, and operational costs. Learning Curve: For those new to API development, there is a learning curve. It may take time to grasp the intricacies of APIs fully. Security Concerns: Ensuring robust security measures is essential. APIs can be vulnerable to attacks if not adequately protected. Versioning Challenges: Managing different versions of an API can become complex, necessitating careful planning and execution. Coding Expertise: Traditional API development often requires coding skills, which may not be the preferred approach for all projects. Consider no-code or low-code solutions to bypass this limitation. Following the best practices and strategies outlined above and acknowledging the limitations, you can create robust and user-friendly APIs that contribute to the growth and connectivity of modern enterprises and provide a seamless experience for users and developers alike. Simplify API Development with LIKE.TG Now, let’s explore how LIKE.TG’s no-code API development solution can assist you in implementing these guidelines effectively. Streamlined Development LIKE.TG API Management simplifies the API development process with its no-code environment. You can define clear API objectives and goals effortlessly. Developers can focus on business logic while the platform takes care of the technical intricacies. This ensures that your APIs are aligned with your organization’s objectives and reduces development time. Automated Documentation LIKE.TG API Management includes built-in features for creating comprehensive API documentation. You can generate clear and up-to-date documentation with ease, detailing endpoints, request-response formats, and authentication methods. This ensures that your APIs are well-documented, reducing confusion and friction for developers. Security and Versioning The platform offers robust security features, including authentication and authorization mechanisms. It also simplifies versioning, making it easy to maintain backward compatibility as your APIs evolve. LIKE.TG API Management allows you to implement these critical best practices without diving into complex coding, reducing the risk of security vulnerabilities and ensuring smooth transitions between API versions. Performance Optimization LIKE.TG API Management simplifies the process of optimizing API endpoints for performance and scalability. Its built-in features allow you to manage caching, fine-tune database queries, and integrate content delivery networks (CDNs) with ease. This ensures that your APIs are designed for efficiency and high performance, meeting the demands of large-scale enterprise applications without the need for complex coding. LIKE.TG simplifies the development process, automates documentation, and ensures robust security. With features that streamline performance optimization, rate limiting, and versioning, the no-code API development tool empowers you to create APIs that align seamlessly with your enterprise objectives. Ready to see LIKE.TG API Management in action? We’d love to show you how it works! Reach out to us at [emailprotected] to take the first step towards efficiency, security, and innovation today. Reduce API Development Time by Up To 80% With LIKE.TG Simplify API development through an intuitive, drag-and-drop interface, enabling seamless API consumption, designing, and deployment for both technical and non-technical users. View Demo
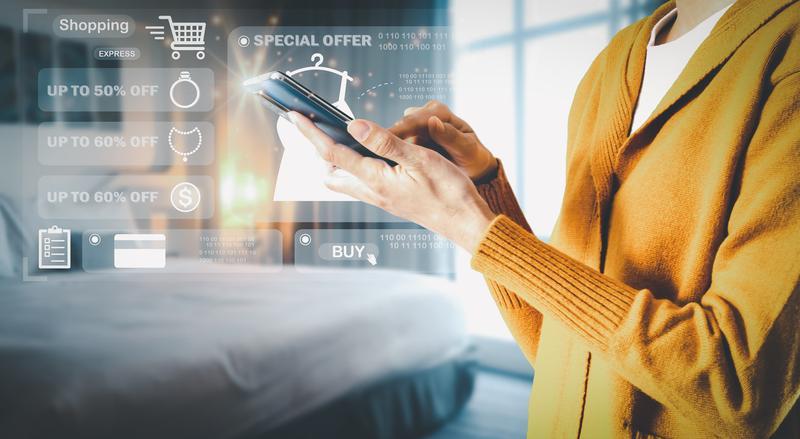
Introducing Cloud File Drop Automation with LIKE.TG
Cloud adoption has risen significantly in the past few years due to digital transformation initiatives. Businesses are migrating their operations to the cloud due to its enhanced scalability, flexibility, and agility. Gartner predicts that by 2025, over 85% of organizations will adopt a cloud-first principle.
The Shift to Cloud Computing
The global business landscape is witnessing a significant shift towards cloud computing, driven by its myriad benefits. Let’s examine some key factors that make cloud computing an ideal solution for your business.
Scalability: Cloud computing offers you the ability to scale resources up or down based on demand, which reduces the need for upfront investments, and accommodates fluctuating workloads.
Accessibility: Cloud services can be accessed from anywhere with an internet connection, promoting remote work, global collaboration, and expansion.
Disaster Recovery: Cloud providers offer robust data backup and disaster recovery solutions, reducing the risk of data loss and ensuring business continuity.
Understanding Cloud File Drop Automation
File Drop Automation is a process that automates workflows based on the addition of new files to a specific folder. This means that when a file is dropped into a designated folder, a predefined workflow is triggered automatically, eliminating the need for manual intervention. As the adoption of popular cloud services such as Amazon S3 and Microsoft Azure Blob Storage is on the rise, this functionality plays an even more important role. It involves the automation of processes when files are added to these cloud-based folders. This includes data processing, data integration, and even data analysis tasks, ensuring that the data is readily available for use across various platforms and applications.
For instance, your business might set up a workflow that automatically processes sales data whenever a new sales report is added to a specific Amazon S3 bucket. Or you might use Microsoft Azure Blob Storage to store customer feedback forms, with a workflow set up to automatically analyze and categorize this feedback whenever a new form is added. By automating these processes, your business can ensure that its data is processed quickly and accurately, allowing you to make timely, data-driven decisions.
Cloud File Drop Automation can benefit your organization through:
Enhanced Efficiency: File Drop Automation significantly reduces the time and effort required to initiate and execute workflows. By automatically triggering processes when a new file is added, it frees up resources for other critical tasks.
Improved Accuracy: With automation, the risk of errors associated with manual processes is significantly reduced, ensuring that the right processes are triggered by the right files at the right time.
Cost-Effective: By reducing the need for manual intervention and minimizing errors, File Drop Automation can lead to significant cost savings in the long run.
Real-Time Processing: File Drop Automation allows for real-time processing of data as soon as it is dropped into the cloud-based folder, enabling businesses to make timely decisions based on the most recent data.
Enhanced Collaboration: With files automatically processed and available in the cloud, collaboration among team members located in different geographical locations becomes easier and more efficient.
Cloud File Drop Automation with LIKE.TG
LIKE.TG makes it possible for you to ingest data from the cloud without spending hours in manual coding and other processes. Simply leverage the file drop automation feature to automate the processing of files in cloud-based sources such as FTP, Amazon S3, and Microsoft Azure Blob Storage. This streamlined automation enhances efficiency and reduces the risk of errors, which makes data management more accurate and reliable.
How LIKE.TG File Drop Automation Works
LIKE.TG allows you to set up a system that automatically detects when a file is dropped into a specified cloud directory, processes the data, and transfers it to a designated destination. This not only simplifies data management but also ensures that your data is always up-to-date and ready for analysis.
To utilize the cloud browser support in File Drop, you need to initially deploy the shared connection via the catalog. Once this is done, you can schedule the process to automate the workflow. This enhancement streamlines the process and makes it more efficient, further empowering businesses to leverage the power of the cloud.
Here is a step-by-step guide on how to effectively use this feature:
As a first step in this process, create a Shared Action containing a Cloud Connection to deploy it via the catalog.
Next, click on enable for catalog option and proceed to deploy the Shared Action.
Once the connection is successfully deployed and added to the catalog, check if the shared action is visible in the Connections section of the Resource Catalog to ensure it has been saved.
Next, open your dataflow. Here, we are writing data from a delimited source to an Excel destination. The file in the source is extracted from the variable where the FilePath is defined.
The variable here comes from the workflow, where the ContextInfo is typically used with the “Dropped File Path” frequency.
Now close the project and open the Scheduler. Here, amongst the Frequency options choose “When File is Dropped” and then select Browse Cloud Folders.
A dialog box will appear displaying your deployed Cloud Connection. Select any folder and set it as the directory to watch.
Enable polling and set the time interval to 30 seconds. This will ensure that the directory is checked every 30 seconds to see if a file has been dropped. Finally, save the scheduler settings and drop a file in the designated folder to verify its functionality.
Final Words
As businesses increasingly rely on data for informed decision-making, the importance of file drop automation in the cloud becomes evident. This feature equips you with a competitive edge in managing the rising volume of information while promoting agility, adaptability, and efficiency in the dynamic business landscape.
LIKE.TG’s file drop automation of cloud-based sources is an efficient and user-friendly feature that can significantly enhance your data management and transfer processes. By utilizing it, you can not only save valuable time but also reduce the likelihood of errors, ultimately leading to a boost in productivity.
To experience the benefits of this feature firsthand, download LIKE.TG’s 14-day free trial today!
Ready for a Workflow Upgrade?
Leverage LIKE.TG's new Cloud File Drop Automation to seamlessly process files dropped into your cloud-based folders. Effortlessly manage files and enhance workflow efficiency in just a few clicks.
Try it Now!
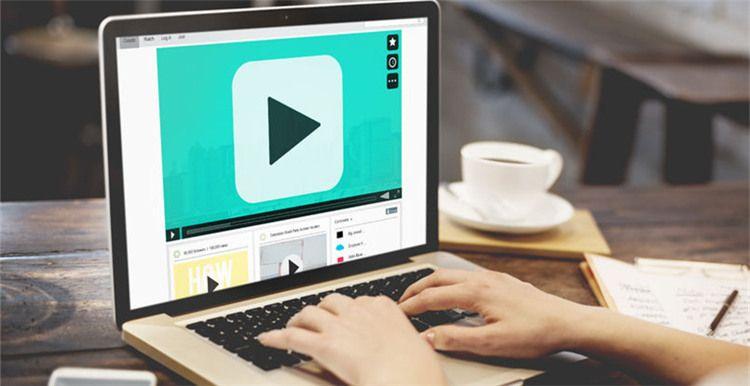
Build an Agile Data Warehouse with an Iterative Approach
If you have had a discussion with a data engineer or architect on building an agile data warehouse design or maintaining a data warehouse architecture, you’d probably hear them say that it is a continuous process and doesn’t really have a definite end. And that, in essence, is pretty much the truth. A successful data warehouse design generally uses an agile approach, iterative development methods that ensure delivering quality insights to end-users based on current business data. Fortunately, cloud data warehouse providers, like Microsoft Azure and Amazon Redshift, offer rapid flexibility and scalability options that make adopting this approach relatively easier than the rigid traditional frameworks. And consequently, having a constantly evolving architecture means you will have access to accurate, up-to-date data to fuel your analytics, allowing teams and departments to meet their respective goals. As Geoffrey Moore rightly said: “Without big data analytics, companies are blind and deaf, wandering out onto the web like deer on a freeway.” So, how can you build your own agile data warehouse design, how does this iterative data warehousing solution work, and what results can a data warehouse team of engineers and business analysts expect from it? Steps to Build a Data Warehouse Creating a More Adaptable, Responsive Data Warehouse Infrastructure In an iterative development model, the data warehouse is in a constant state of improvement and evolution. Instead of creating an architecture that answers all the queries on day one, your team focuses on the information that matters to your business users the most. You need to prioritize what’s important, put them into small manageable segments, and then take several rounds to modify your data warehouse architecture based on your business requirements. To achieve this, data teams work in multiple sprints and cycles, making changes to the ETL flows, dimensional models, and other data processes after taking feedback from business analysts and other stakeholders. After every cycle, continuous feedback ensures that these implementations provide a true picture of business performance and effectively address pain points and gaps. Build Your Data Warehouse From Scratch within a matter of days - 14-day Free Trial Try It Now! What do you need to build an agile data warehouse? Aside from a dedicated team of data architects and analysts, you’ll essentially need a data warehousing tool that offers a codeless development environment to facilitate rapid changes, allowing you to kick the rigidity out of the architecture. Main Components of LIKE.TG’s Data Warehouse Builder With that said, almost any organization can deploy an agile data warehousing solution, provided that it has the right technology stack fueling the initiative. In essence, a data warehousing toolshould provide: Source data modeling capabilities that can easily map and build relationships among your data sources Dimensional modeling functionality that can help determine facts and dimensions based on the source data and generate the schema and tables that would fit into your destination warehouse A high-performance ETL engine to simplify and accelerate data aggregation and offer an array of transformation options, like normalization, denormalization, merge, join, and more The option to switch to ELT or pushdown mode to reduce time to transform and access business data quickly Data quality modules to ensure each byte of data meets the quality standards of your organization A wide range of web applications, databases, and cloud connectors to connect and bring your business data to your data warehouse Slowly Changing Dimensions (Types 1-6) to control how different data fields are populated in database tables Support for OData service to provide secure ways to end-users to access data for reporting and analyses This is not an exhaustive list but just an overview of the major features and functionalities required. Now, you may find many solution providers that patch together several tools and build a customized package to close the functionality gaps. However, the recommended way is to opt for a unified platform that checks all these boxes to meet your data warehousing needs. Discover best practices to manage high volume data warehouses Download Whitepaper Okay, Sounds Great. But Why Should I Consider This Approach? Well, here is why: The main purpose of any agile data warehouse design implementation is to provide answers backed by trustable data based on the current business scenario. But the problem is that growing companies acquire new data sources and make changes to the information flow within apps and databases to match the pace of their increasing business needs. All these new sources and structural updates naturally have to be factored in the existing data models and reflected in the centralized repository to supply accurate, trusted data to end-users. As such, the iterative approach follows the agile methodology that allows you to design, develop, and deploy the most flexible, result-oriented architecture to build your enterprise data warehouse. You can easily include new data streams without having to revisit or rewrite ETL scripts, and that too in a matter of a few hours, if not minutes. That’s largely because modern data warehousing tools provide a code-free development environment and end-to-end process automation, allowing you to make quick changes to your flows and build better ETL processes. Not only that, you can enjoy the benefits in various scenarios as well. Whether it is planning and strategizing the data warehousing process, deploying prototypes to test its feasibility, or performing ETL testing to ensure consistent results, this agile methodology facilitates the development process at every stage. Perhaps, the real value of this approach is seen in the business benefits organizations can yield with its implementation. These include but not limited to: Faster results to meet end-user Data Warehouse business intelligence (DW BI) analytics requirements Better alignment with the evolving business needs Ability to speed up prototyping and feasibility analysis Lower TCO due to streamlined development processes Higher quality, up-to-date insights into robust decision making Reduced project complexity due to smaller, more manageable cycles The ability to identify shortcomings faster, leading to better risk management Greater transparency into the progress, performance, and challenges in each iteration Supercharge your BI initiatives and accelerate time-to-insight journey Get Started A Solution that Fits the Bill “The traditional architecture is much too slow. We no longer have the luxury of two to three months. We need to be able to tell users, ‘We can have that for you — in two to three days.'” – Rick van der Lans From source to insights – 7 steps And this is where modern data warehousing solutions, like LIKE.TG DW Builder, come in that are known to cut down development time significantly through end-to-end automation. It provides a unified data warehousing framework that enables a business to go from gathering requirements all the way to analytics via direct integration with data visualization software. Interested in creating your own data warehouse based on an agile, iterative development method? Take the first step and get a personalized live demo of LIKE.TG DW Builder.
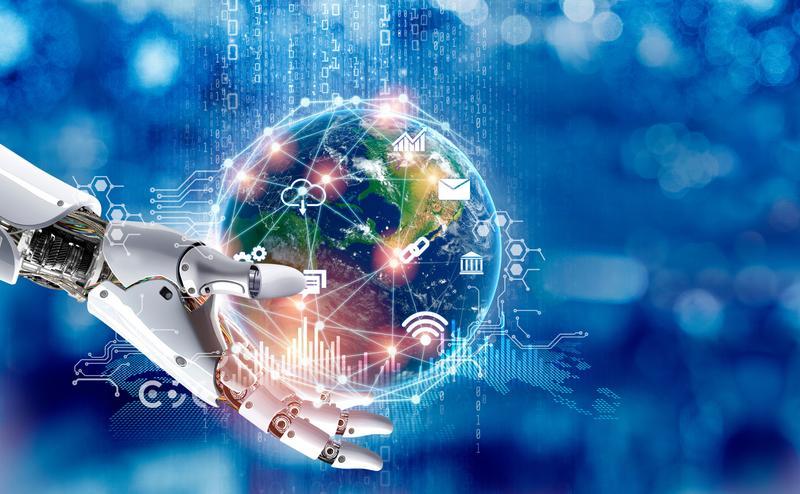
SOAP vs. REST: Which API Design is Right for Your Business?
According to Slashdata, nearly 90% of developers use APIs in some capacity. APIs enable developers to efficiently build software applications by abstracting away the complexity of low-level software layers—allowing developers to focus on core functionalities. Whether you’re a business or IT professional, understanding the nuances of API development is crucial for your organization’s success. There are two main API building methods: SOAP and REST. These methods differ significantly in their approaches and characteristics, each with its own set of advantages and considerations. What is SOAP? SOAP, or Simple Object Access Protocol, is a protocol-based approach to API development. It follows strict rules for communication, using XML as its message format. SOAP APIs are known for their structure, built-in error handling, security features, and stateful capabilities. What is REST? REST, which stands for Representational State Transfer, is an architectural style for building APIs. It’s all about simplicity and flexibility. REST APIs use various formats for message exchange, including JSON and XML. They are inherently stateless and rely on the underlying transport protocol, usually HTTP, for security and error handling. (Source: Seobility) SOAP vs. REST: Which API Suits Your Business? The way APIs handle communication, message formats, state management, error handling, and security can significantly impact your development process and the performance of your applications. SOAP, a protocol-driven approach, and REST, an architectural style, offer distinct features that are worth exploring. Communication: Protocol vs. Architectural Style SOAP is a protocol that mandates a set of rules for communication. It relies on request and response messages, typically transmitted over HTTP, SMTP, or TCP. In contrast, REST is an architectural style that doesn’t dictate a particular protocol. It takes advantage of existing protocols, primarily using HTTP methods like GET, POST, PUT, and DELETE. In an enterprise-level inventory management system real-time communication between servers and client applications is crucial. SOAP would be ideal, as it defines a clear communication protocol, ensuring that data integrity and consistency are maintained. On the other hand, if you are developing a public-facing e-commerce website, REST’s architectural style, which leverages standard HTTP methods like GET, POST, PUT, and DELETE, would provide the necessary flexibility to interact with different clients and platforms while taking advantage of existing web protocols. Message Format: XML vs. Multiple Formats SOAP exclusively uses XML for message formatting, which ensures strict structure and data typing. REST, on the other hand, allows for multiple formats, including JSON, XML, and HTML. This flexibility can be a game-changer, especially in diverse development environments. A financial application requiring accurate and strict data representation, would be best suited to SOAP. SOAP, with its reliance on XML, ensures that financial transactions are consistently formatted, reducing the chances of data interpretation errors. In contrast, if you’re developing a social media platform, REST’s support for multiple message formats like JSON, XML, and HTML allows you to cater to a wide variety of clients, including web browsers, mobile apps, and third-party integrations, making it a versatile choice. State Management: Stateless (with options) vs. Stateless SOAP can be either stateful or stateless, depending on how you configure your API. In contrast, REST is inherently stateless, which simplifies server and client communication. However, this means you may need to manage states manually if required. Consider a multi-step transactional process like booking a flight ticket. SOAP’s stateful capabilities can help maintain the session throughout the booking process, ensuring that user data is consistently available across multiple requests. If you’re building a content management system where each HTTP request is independent and doesn’t rely on previous requests, REST’s stateless nature simplifies both server and client interactions, making it suitable for systems where maintaining session states isn’t a primary concern. Error Handling: Built-in vs. Dependent on Implementation SOAP comes with built-in error handling through standardized fault messages, making it easier to pinpoint issues. In REST, error handling is dependent on the implementation, often utilizing HTTP status codes. This flexibility can be both a blessing and a curse. When developing a healthcare information exchange system, the built-in error handling of SOAP, with standardized fault messages, ensures that any errors in transmitting critical patient data are immediately and clearly addressed, enhancing patient safety. In the context of a public-facing news website, REST’s flexibility in error handling allows you to tailor error responses to suit the specific needs of various clients. While this flexibility can be advantageous, it also requires a more meticulous hand in implementation. Security: WS-Security vs. Dependent on Protocols like HTTPS SOAP provides robust security features through WS-Security, making it an excellent choice for sensitive data and regulated industries. REST relies on the underlying transport protocol, such as HTTPS, for security, which is suitable for most use cases. A banking application that deals with sensitive financial transactions would benefit from SOAP’s WS-Security’s strong encryption and authentication, ensuring that customer data is protected to the highest standards and complies with regulatory requirements. However, for a weather forecast service that provides publicly available information, relying on the underlying transport protocol’s security, such as HTTPS, is a cost-effective and suitable choice. This minimizes the complexity of security implementation for non-sensitive data. These distinct capabilities and features illustrate how the choice between SOAP vs. REST is as complex as the specific requirements and constraints of your project. Your choice should align with the goals, resources, and nature of your business. Factors to Consider When Choosing Between SOAP vs. REST When standing at the crossroads of API design decisions, i.e., SOAP vs. REST, several critical factors come into play. Your choice between SOAP and REST isn’t just a technical matter; it’s a strategic decision that impacts your project’s success. Here are some key factors to keep in mind: Nature of the Project It’s all about matching your API to your project. For example, if you’re building a big enterprise system with lots of complex processes that need to be just right, SOAP is a good pick. It’s the sturdy, reliable option. But if you’re creating a dynamic public web app or working on smaller connections, REST is a more flexible option. Required Level of Security From a data security POV, keep in mind that if your API handles processes with confidential data assets like financial transactions or personal medical records, SOAP has stronger security features that’ll keep your data safe. For non-sensitive data, REST is both more cost effective and has enough security. Expected Volume of Traffic and Scalability Needs If you’re expecting a huge crowd and loads of data, REST is the go-to choice. It’s good at handling a lot of requests without getting bogged down. But if you need to keep meticulous access records, SOAP’s is the better choice. Integration with Existing Systems Another important factor is how your new API fits in with your current systems. If your organization already uses mostly SOAP-based services, a SOAP-based API will make your life easier and vice versa with REST-based services. The Skillset of the Development Team If your development team is skilled with XML and structured data, SOAP aligns well with their existing skill set. If their expertise leans toward web technologies, REST is quicker and easier. A solution that works irrespective of technical skill is a no-code API development solution. Conclusion Your decision when evaluating SOAP vs. REST should be driven by your unique business needs, technical demands, and future aspirations. There’s no one-size-fits-all answer, and that’s perfectly okay. SOAP and REST are like different tools in a toolbox, each designed for specific tasks. So, whether you opt for SOAP or REST, it’s all about crafting an API that perfectly fits your mission, ensuring that your digital endeavors are primed for success. Contact us to learn more about how LIKE.TG, a self-service no-code API design tool, can support your API strategy.
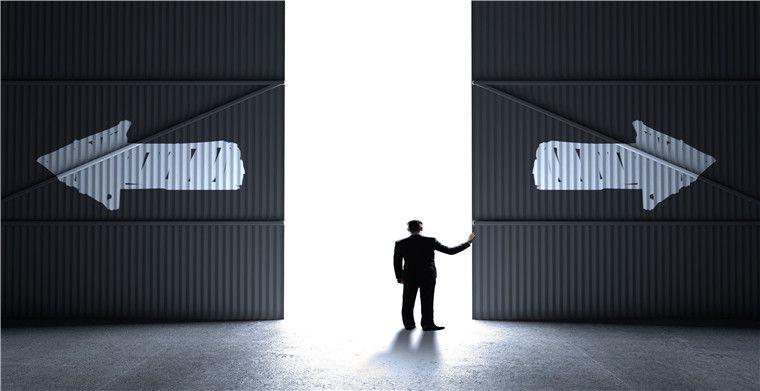
A Complete Guide to the Different Types of APIs
Although, APIs have a recent history, they have penetrated the digital ecosystem so quickly that everything today is dependent on them. From web development to mobile applications, APIs have become the building blocks of the digital world. Over the years, APIs have evolved significantly so today there are various types of APIs, each with its own purpose. Moreover, APIs are not only categorized based on their architecture but also based on their access policies. Therefore, it is crucial to understand the different nature of these APIs. If you’re confused about which API to use and when, this guide will help clear the confusion and will help you choose the right one! Let’s dive straight in. What are The Different Types of APIs? There are four main types of APIs: Public APIs Internal APIs Partner APIs Composite APIs Public APIs Public APIs, also known as external or open APIs, are APIs made available to developers, businesses, and the public by companies, organizations, or platforms. These APIs provide access to certain functionalities or data that the API provider exposes to external users or third-party developers. You can access these APIs via a form of authentication – usually an API key. Some public APIs are free while others require a payment based on the number of times the API is called. An example of a paid public API is the Google Translate API, which costs $20 for every 1 million characters translated. Internal APIs Internal APIs, also called private APIs or in-house APIs, are developed to be used within an organization. These APIs serve as the communication bridge between different components, services, or applications within a company and are not intended for external access or use by third parties. While internal APIs are flexible in their documentation and naming conventions, as they usually don’t follow the OpenAPI standard they are generally less secure than other types of APIs. Partner APIs Partner APIs are also called external APIs. These APIs facilitate communication and data exchange between different businesses, organizations, or entities and enable controlled access to certain functionalities, services, or data between partnering companies. Imagine a partnership between a cloud-based accounting software and a payment processing company. A partnership API allows the software to integrate with the payment processor’s services. The payment company provides the API to multiple companies in this way. Composite APIs Composite APIs allow you to execute multiple API requests into a single call. So, in short you can perform complex operations with a single call, reducing the number of round trips to the server and improving performance. Composite APIs are particularly useful for scenarios where multiple API calls are tightly related and need to be executed in a specific sequence. Think of an e-commerce website. There are multiple steps to making a purchase: Search for a product. Click on the result. Add to cart. Checkout. Rather than execute each call separately, it is more efficient to combine and execute them at once. API Protocols An API protocol refers to the set of rules, standards, and conventions through which communication occurs between different software components or systems. It outlines the structure and methods by which applications or services can interact and exchange data. It’s important to understand the different types of protocols as the way you create and manage your APIs is influenced by the protocol you use. The common API protocols are: REST SOAP GraphQL RPC REST APIs The full form of REST is Representational State Transfer. REST APIs use HTTP requests (GET, POST, PUT, DELETE) to interact with resources (data) via URIs. They follow stateless communication and generally use the XML format for data exchange. REST APIs are defined by: Resource-oriented:REST APIs treat data as resources,each with a unique identifier (URI or URL). Client-server architecture:REST APIs separate the client (application) from the server (API provider),allowing for independent development and deployment. Stateless:REST API interactions are stateless,meaning each request contains all the necessary information to be processed independently. Cacheable:Responses can be cached to improve performance and reduce server load. Read more about REST API When to use them: RESTful APIs are best suited for scenarios that require scalability, simplicity, and interoperability. They work well for web and mobile applications, where flexibility in data retrieval and ease of use are essential. Use them for CRUD operations (Create, Read, Update, Delete) on resources and when working in a distributed system environment. (Source: Seobility) SOAP APIs SOAP stands for Simple Object Access Protocol. These APIs use standardized XML for message formatting and typically operate over HTTP, TCP, SMTP, and other protocols. They employ a strict messaging format, including WSDL for defining the service interface. A SOAP API consists of the following components: SOAP Envelope:The outer layer of the SOAP message,containing the header and body sections. SOAP Header:Optional section that provides additional information about the message,such as routing or security information. SOAP Body:Mandatory section that contains the actual data being exchanged. SOAP Operation:A specific action that the API can perform,such as creating,retrieving,updating,or deleting data. When to Use: SOAP APIs are beneficial for complex enterprise-level applications that demand strong security measures, ACID transactions, and error handling. They’re suitable for scenarios where standards compliance and built-in mechanisms for data integrity and security are critical, such as financial transactions. They’re also protocol independent, unlike REST. Read more about SOAP APIs and how they compare to REST. GraphQL APIs GraphQL is not actually an API protocol rather it is a query language and runtime for APIs. It allows clients to request specific data structures using HTTP requests. GraphQL APIs use a single endpoint and a flexible query language with unlimited data schemas. Unlike REST APIs, which require multiple round trips to fetch the desired data from different endpoints, GraphQL allows clients to specify the exact data they need in a single request. With GraphQL, clients can specify the shape and structure of the data they require using a query, and the server responds with JSON data that matches the query, which help reduce over-fetching and under-fetching. When to Use: GraphQL is ideal when there’s a need for optimized data fetching tailored to client requirements. It suits scenarios where clients demand flexibility in data retrieval, such as in mobile applications or when dealing with complex data structures. RPC APIs RPC stands for Remote Procedure Call, and RPC APIs facilitate communication between different processes by allowing one program to execute procedures on another system. RPC protocol return a method instead of a resource. For example, an RPC protocol might return a server confirmation or an error, while a REST protocol might give a text document. There are various implementations of RPC, such as gRPC, XML-RPC, and JSON-RPC. Each has its own protocols and methodologies for handling remote procedure calls. These APIs are commonly used in building distributed systems, client-server architectures, and other scenarios where different components need to communicate over a network. When to use: RPC APIs are useful where developers need to invoke functions or procedures on remote servers. They are a suitable option for lightweight data packs and basic requests. Now that you understand the different API types, let’s look at more examples of APIs around us. APIs are All Around Us The digital world is filled with APIs of different types. They act as bridges between applications, allowing them to exchange data. Here are some examples of APIs commonly used in various aspects of our lives: Weather APIs: These APIs provide real-time and historical weather data, including temperature, precipitation, forecasts, and weather alerts. They are used by weather apps, news websites, and smart home devices to keep us informed about weather conditions. Maps and Navigation APIs: These APIs provide maps, directions, and traffic information, enabling navigation apps and location-based services. Google Maps and Apple Maps fall in this category. Social Media APIs: Social media platforms like Facebook, Twitter, and Instagram expose APIs that allow developers to integrate social media features into their applications. This enables sharing content, accessing user profiles, and managing social interactions. Payment APIs: APIs like PayPal, Stripe, and Square facilitate online and mobile payments. This allows merchants to accept payments securely and integrate payment processing into their websites or apps. E-commerce APIs: These APIs provide the building blocks for e-commerce platforms, like Amazon and eBay, enabling product catalog management, order processing, inventory tracking, and customer relationship management. Communication APIs: APIs like Twilio and Plivo enable developers to integrate SMS, voice, and messaging functionality into their applications, allowing for real-time communication and notifications. Machine Learning APIs: APIs like Google Cloud AI, Amazon Rekognition, and Microsoft Azure Cognitive Services provide machine learning capabilities for tasks like image recognition, natural language processing, and sentiment analysis. Open Data APIs: Governments and organizations around the world publish open data APIs that provide access to public datasets, enabling developers to create data-driven applications and visualizations. Choosing the Right API – Factors to Consider The type of API you choose will depend on your particular use case. However, there are certain universal principles that you need to keep in mind while picking an API such as: Documentation: Solid documentation is a big plus for both users and developers as it helps developers implement APIs efficiently and successfully. SOAP APIs have a strict set of rules, while REST are more flexible. Security: APIs should use robust encryption and authorization standards, such as OAuth 2 and SSL, to protect sensitive data. Some APIs, like RPC, are less secure than others and are only suitable for internal use. SOAP APIs provide better security. Ease of use and developer familiarity: APIs should be easy to use. Your developers should also be familiar with the chosen protocol as it simplifies development. REST is a good option as it is the most widely used protocol. API adoption: A good API is easy to acquire. The signup process should be simple with robust authentication. Another aspect of ensuring easy adoption is good chat and live support. This isn’t limited to the sign up – good support also extends to debugging errors. REST APIs are popular for being very user-friendly, being widely used in web services. Scalability:Consider the expected usage volume and traffic patterns of your API. If you anticipate high traffic or frequent requests, opt for a protocol that can handle large amounts of data efficiently. REST APIs are highly scalable, and a good option. Vendor ecosystem:Third party support is also important. Evaluate the vendor ecosystem surrounding the protocol, including the availability of third-party tools, libraries, and management platforms. Improve Your Knowledge of APIs This blog is just an introduction to some of the various types of APIs. Each API type is very complex and interesting and should be studied thoroughly. Now that you have a basic understanding, you can go ahead and learn more about each individual type in more detail. Check out our other API blogs for a more in-depth exploration: Web API vs. REST API SOAP vs. REST CRUD APIs Want to build robust APIs in a code-free environment? Check out our guide to creating APIs. Learn more about LIKE.TG’s API solution. LIKE.TG enables teams to easily and securely create, publish, and monitor APIs in a no-code way, simplifying the entire API management cycle.
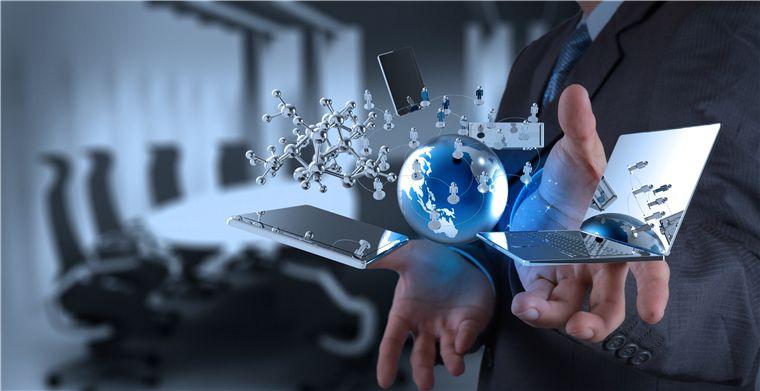
10 Best Informatica Alternatives & Competitors in 2024
Informatica, one of the key players in the data integration space, offers a comprehensive suite of tools for data management and governance. However, for reasons such as cost, complexity, or specific feature requirements, users often seek alternative solutions. In this article, we are going to explore the top 10 Informatica alternatives so you can select the best data integration solution for your organization. But before we get to it, let’s quickly get the basics out of the way and understand why there is a need for an alternative data integration solution to Informatica. What Is Informatica? Informatica is an enterprise-grade data management platform that caters to a wide range of data integration use cases, helping organizations handle data from end to end. The services it provides include data integration, quality, governance, and master data management, among others. The overall goal is to combine company-wide data into a single source of truth for BI, reporting, and analytics. Key Features of Informatica Informatica offers several features that help users with their data management and integration projects. Some of its features include: Database Management Like other data management and integration platforms, Informatica enables users to connect to and integrate data from diverse databases, for example, Oracle, SQL Server, etc. It uses the metadata-driven approach to optimize performance, streamlining the overall database operations. Data Transformation Putting aside the fact that it’s not 100% no-code, Informatica offers a rich set of transformations. These include aggregator, data cleanse, data masking, filter, to name a few. Orchestration Informatica’s Data Integration Hub makes the best use of its orchestration capabilities, coordinating data movement across different systems and applications. API Integration Besides ETL, Informatica also offers API integration features that facilitate interaction between applications and services. Access Control Informatica enables users to fine-tune access controls and manage permissions for data sets. They can also set permissions on database, domain, and security rule set nodes to authorize users to edit the nodes. Data Security As far as security is concerned, Informatica employs a range of measures tailored to its suite. These include target database credential management, encryption, access controls, and data masking. So, if the platform offers such a vast set of features, why should users then consider the different Informatica alternatives, or competitors for that matter? Let’s find out. LIKE.TG—One of the Best Alternatives to Informatica for Data Integration Looking for a powerful yet easy-to-use alternative to Informatica for data management and integration? Look no further. Try LIKE.TG. Learn More Why Consider Informatica Alternatives for Data Integration? Informatica has established itself as a key player in the data management and integration space. However, this feat is not enough for companies in today’s rapidly evolving business landscape, especially if we consider the increasing number of new SaaS startups. These businesses need dynamic solutions that are: The best fit for their use case Easy to implement, learn, and use Economical with transparent pricing Committed to exceptional customer support Additionally, with the global data integration market size set to reach USD 39 billion by 2032, numerous opportunities for innovation and growth exist, particularly in areas like real-time data integration, data quality and governance, and the integration of unstructured data and emerging technologies, making space for new players to enter the market. This means that the consumers now have a myriad of options to choose from. Here are some reasons why an Informatica alternative could make more sense for your business: Cost Considerations While informatica offers a breadth of products and services, sometimes it becomes a simple case of getting overwhelmed and paying for features that users will hardly ever use. Additionally, Informatica is relatively more expensive when compared to other options, such as LIKE.TG. The already complex pricing structure lacks transparency and their website does not offer full pricing information. Its implementation requires significant investments in hardware and infrastructure, making the overall total cost of ownership (TCO) much higher—even in the long run. High Complexity Another major challenge with Informatica is its complexity—this is more of an inherent issue with the platform. The platform is so vast and complex to use that at times even the Informatica-certified data professionals face challenges. This is primarily due to its different modules/services using vastly different user interfaces (UI). Specialized Use Cases An organization that has a highly specific use case might find that an alternative solution is better aligned with its needs. While Informatica is a comprehensive and versatile platform, its extensive feature set may include functionalities that might be unnecessary or less relevant to their unique use case. It’s like choosing a general-purpose tool with a wide range of features instead of a precision tool for a specialized job. Resource-Intensive Informatica, especially its PowerCenter, is very resource-intensive compared to its competitors, requiring significant processing power and memory. However, the performance is still not comparable. Based on reviews on popular channels, such as G2, Gartner, etc., users have frequently reported performance issues and slowdowns, raising several eyebrows. Top Informatica Alternatives to Consider in 2024 LIKE.TG LIKE.TG is an end-to-end, automated data management and integration platform powered by artificial intelligence (AI). It features a truly unified, 100% no-code UI that delivers consistent user experience across all its products. The tool enables users of all backgrounds to build their own data pipelines within minutes. LIKE.TG Data Stack The platform stands out by offering a flexible and cost-effective approach to meet the unique needs of every business. Unlike Informatica’s rigid pricing structures, LIKE.TG allows you to collaborate with their expert data solution team and cherry-pick the modules that align precisely with your use case. This ensures that you pay only for the functionalities that are essential to your operations. LIKE.TG includes: ReportMiner for unstructured data extraction in bulk Centerprise for data integration and building data pipelines Datawarehouse Builder for data warehousing EDIConnect for EDI management API management for designing, testing, publishing, and monitoring APIs The platform offers several features that easily make it one of the best Informatica alternatives. For example, with LIKE.TG, you can: Establish native connectivity to a range of data sources and destinations, both on-premises and cloud-based. Transform and shape your data the way your business needs it using pre-built transformations and functions. Build ETL and ELT pipelines without writing a single line of code. Ensure only healthy data makes it to your data warehouses via built-in data quality management. Automate and orchestrate your data integration workflows seamlessly. LIKE.TG—One of the Best Alternatives to Informatica for Data Integration Looking for a powerful yet easy-to-use alternative to Informatica for data management and integration? Look no further. Try LIKE.TG. Learn More Talend (acquired by Qlik) Talend is another data management solution that enables businesses to drive their BI and analytics. Talend’s Data Fabric is a cloud-independent platform covering the entire data lifecycle. It features data preparation, transformation, integration, as well as data quality and governance. Pros Enables users to build ETL pipelines and move data from a myriad of sources. Offers built-in transformations, including unions and joins. Offers an open-source version, making it accessible to a wide range of users and organizations. Cons Despite a visual interface, mastering all the features and capabilities takes considerable time. It’s not a completely no-code solution. Some users have reported performance issues with Talend, particularly when dealing with large datasets or complex transformations. Real-time processing capabilities are not as robust compared to some other data integration tools. Some important features, such as scheduling, are only available in the enterprise version that comes with a premium price tag. Given the limitations, and its recent acquisition, many are actively looking for Talend alternatives. Alteryx Alteryx offers a solution that allows users to access, manipulate, and analyze data without coding. It enables users to connect to a wide range of data sources and ETL data in a visual, drag-and-drop environment. Pros Its user-friendly interface makes it easy to use for most users. It excels in data blending and preparation, allowing users to clean, transform, and combine data from various sources. Multiple users can take advantage of the workflows by using the server instead of purchasing multiple individual licenses. Cons While Alteryx offers a capable reporting ability, it’s not as versatile as other competing solutions, such as Power BI. Applying logic is limited to columns—users have to work around when working at the record/cell level. Error-handling, while available, doesn’t provide greater visibility into dataflows. Additionally, users report that the error messages can be vague. Oracle Oracle Data Integrator (ODI) enables data movement and transformation in bulk. It’s primarily an ELT platform that is highly interoperable with other Oracle programs, making it a great Informatica alternative for businesses that have invested in other Oracle products and services. Businesses can use ODI with GoldenGate and unify data loading with synchronization, keeping their data warehouses up to date. Pros A great addition to technology stacks that already include other Oracle products as it integrates seamlessly within the Oracle ecosystem. Simple and straightforward UI makes it easy to use and navigate. Most use cases can be catered to in a single interface without having to switch between multiple interfaces. Cons While the tool is generally easy to use, it’s not particularly intuitive, especially when troubleshooting. The price can be prohibitive as the TCO in the long run is higher compared to competition. User review sites report frequent performance issues and suboptimal customer support, especially when it comes to Oracle Data Integrator. SnapLogic SnapLogic is an iPaaS that offers application, data, and cloud integration for enterprises. Like other tools, SnapLogic also relies on low-code/no-code approach to fast-track application and data integration. Pros Offers a dashboard to monitor configured pipelines and integrations easily. Powered by a visual UI that’s simple and easy to use and navigate. Provides support for real-time integration, as well as batch processing. Cons Users have reported that the resource utilization is not accurately displayed on the dashboard. The support for XML files is limited, especially the ones with mixed content. While it sports a visual UI, it’s not one of the most intuitive ones. Error-handling and available documentation lack depth. Ab Initio Ab Initio is an enterprise-level self-service data platform offering a range of capabilities, including batch and real-time data integration, BI and analytics, automation, as well as data quality and governance. Pros Ab Initio’s end-to-end graphical development makes it simple for users to easily process data. The ability to handle very large data sets—users can process billions of records in minutes. Overall, a feature rich ETL platform powered by parallel processing. Cons One of the biggest downsides of Ab Initio is the significant upfront licensing and infrastructure cost. While its graphical development environment is based on the low-code approach, it still has a steep learning curve for new users. Requires databases running in the background on the servers to function, adding to the already expensive solution. IBM InfoSphere DataStage IBM’s InfoSphere DataStage is a data integration platform used to design, develop, and run workflows that transform and move data. At its core, it’s an ETL tool that leverages graphical notation to build data integration solutions. Pros The solution offers high availability ands scalability—users can use multi-instance jobs that scale easily. Users can leverage complex transformations without having to write code. Metadata and data quality features are built into the solution. Cons Compared to its competitors, IBM InfoSphere DataStage is a more expensive solution. The initial setup being a complex process and the UI not being as user-friendly as the competition make it hard to justify the higher price point. Some processes can be time-consuming, for example it takes a lot of time to trigger jobs and then view logs. AWS Glue AWS Glue is another Informatica alternative, which is a serverless data integration solution offered by Amazon as part of Amazon Web Services (AWS). The overall goal with AWS Glue is to make data preparation faster and cheaper for machine learning (ML) and analytics. It does so by simplifying data integration by modernizing the ETL process using automatic provisioning and worker management. Pros AWS Glue offers a user-friendly UI that’s simple and intuitive, keeping the initial learning curve smooth. Pay-as-you-go pricing structure ensures businesses only pay for what they need. It also eliminates the need to provide capacity in advance, which simplifies scheduling. Offers advanced users the ability to create ETL scripts with custom endpoints. Cons While the UI is user-friendly, it’s not entirely beginner friendly. Some tasks will require Python and Scala expertise. While is offers support for creating ETL scripts, only two programming languages are supported: Python and Scala. AWS Glue works flawlessly as long as the user works within the AWS ecosystem. Integrating with platforms outside of Amazon is tricky. Microsoft SQL Server Integration Service As the name suggests, Microsoft SQL Server Integration Service (SSIS) is a service in Microsoft’s SQL Server software that can be used to integrate data from multiple sources. It allows users to build ETL-based enterprise-grade data integration and transformation solutions. Being Microsoft’s own service, SSIS offers superior interoperability for businesses that have invested in Microsoft’s other services, making it a great Informatica alternative. Pros Included with SQL Server licenses, making it a cost-effective option for smaller organizations already invested in the Microsoft ecosystem. Drag-and-drop interface and tight integration with SQL Server make it user-friendly for beginners and experienced developers alike. Can scale to handle large data volumes by utilizing multiple servers and parallel processing. Cons Due to limited availability of pre-built connectors, it requires scripting or custom development for some data sources and formats. Some cases require investment in additional tools due to its limited data transformation capabilities. The visual representation in the IDE sometimes does not adequately reflect the underlying code, making code review and collaboration challenging. Integrate.io Integrate.io is another Informatica alternative for businesses looking to integrate data via ETL, ELT, and CDC. It’s a cloud-based data integration platform that may be a fit for businesses of all sizes, depending on the use case. Like other visual data integration platforms, Integrate.io also offers similar features, such as pre-built connectors, transformations, etc., making it easy to use for users with varying technical expertise. Pros Offers transparent pricing based on the number of connectors used, eliminating the complex and often expensive licensing fees. Provides enterprise-grade transformation capabilities that are easy to work with. Responsive customer support that’s available 24/7 in all time zones. Cons Debugging can be a challenge, especially when fault records lack granularity and error logs lack specificity. While it offers a transparent pricing model based on flat rate pricing, the yearly costs can turn out to be higher than the alternatives. Even though the platform is low-code, the UI can be challenging for businesses without dedicated technical teams as the complexity of integrations increases. Simplify and Accelerate Data Integration With LIKE.TG Build a connected enterprise data architecture with a 100% no-code platform that simplifies and accelerates data integration. Download Free Trial Here’s Why LIKE.TG Is the Best Informatica Alternative The decision to opt for a data integration solution is not straightforward since most solutions offer similar features and cater to a wide range of use cases. Ultimately, it comes down to an organization’s unique requirements, including data volume and variety, integration complexity, desired level of data automation, security requirements, budget constraints, and future scalability, and how these requirements align with the capabilities and limitations of available solutions. In other words, it’s about finding the perfect fit. In this context, LIKE.TG stands out from the abovementioned Informatica alternatives as it offers a complete data integration and management suite with a unified user interface that delivers consistent experience throughout. This means that the platform can be used by business and technical users alike. Here are some more reasons to consider LIKE.TG as an alternative to Informatica: Simple and straightforward initial setup process significantly reduces the time required for solution deployment. 100% no-code, visual drag-and-drop UI keeps the learning curve short and smooth. Parallel processing ETL/ELT engine delivers superior performance even when dealing with high volume data sets. Pre-built connectors for a breadth of data source types, including file formats, databases, data warehouses, applications, cloud storages, web services, and file providers. The ability to instantly preview data at every step of the process with a couple of clicks. LIKE.TG offers the capability to build an entire data warehouse from scratch without writing a single line of code. The ability to build a custom connector for the required source or destination seamlessly. Proactive customer support that’s available round the clock regardless of geographic location. Don’t overpay for complexity. Get more for less with LIKE.TG. Download a 14-day free trial to get started today. Alternatively, you can get in touch with us directly at +1 888-77-LIKE.TG, where one of our data solutions experts will be happy to assist you with your use case.
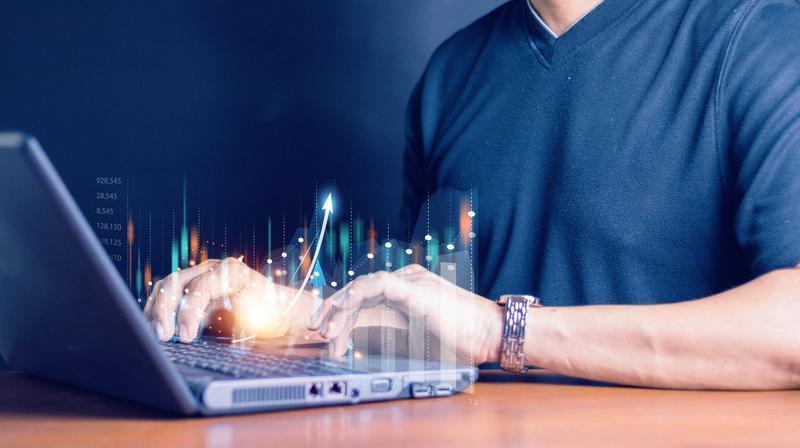
Data Pipeline Monitoring: Metrics and Best Practices
A report by KPMG indicates that only 35% of executives report a high level of trust in an organization’s use of data and analytics. The other 65% face difficulties transporting and using data. This highlights the need for effective data pipeline monitoring. Data pipeline monitoring enhances decision-making, elevates business performance, and increases trust in data-driven operations, contributing to organizational success. What is Data Pipeline Monitoring? In its simplest form, data pipeline monitoring is the ongoing process of overseeing and managing the flow of data from its source to its destination. It is a systematic process that involves tracking, validating, and ensuring the smooth operation of a data pipeline, which is a set of processes that move data from one system to another. The primary goal is to maintain the integrity and reliability of data as it moves across the pipeline. Importance of Data Pipeline Monitoring Data pipeline monitoring is crucial for several reasons: Data Quality: Data pipeline monitoring is crucial in maintaining data quality. By continuously tracking the data, errors and inconsistencies can be detected and rectified promptly. This ensures that the final data used for analysis and decision-making is accurate and reliable. Efficiency: Data pipeline monitoring identifies any bottlenecks or inefficiencies in data processing. Optimizing these processes allows data to be processed faster and more efficiently. Reliability: Regular monitoring ensures that the pipeline is functioning correctly and that data is delivered to its destination on time. Regulatory Compliance: In many industries, such as healthcare and finance, regulation govern data handling. Data pipeline monitoring ensures compliance with these regulations by providing a clear record of how data is processed and where it is stored. Benefits of Data Pipeline Monitoring Having established the significance of data pipeline monitoring, let’s explore the practical benefits it offers: Minimized Data Processing Latency Monitoring tools enable organizations to identify and address performance bottlenecks in real-time, leading to minimized data processing latency. This ensures that data moves efficiently through the pipeline, supporting timely analytics, reporting, and other critical business processes. Automation and Orchestration Monitoring can be integrated with automation and orchestration tools to trigger responses or corrective actions based on predefined conditions. This can help in automating routine tasks and ensuring the reliability of the pipeline. Enhanced Data Security Data pipeline monitoring plays a vital role in ensuring the security of sensitive information as it moves through the pipeline. By continuously monitoring access patterns, data flow, and user interactions, organizations can quickly identify and respond to any suspicious activities or potential security breaches. Data Monitoring Techniques Let’s explore some key data monitoring techniques, also known as the three pillars of data observability, for optimizing data pipeline performance: Metrics Metrics are numerical measurements that quantify various aspects of a system’s performance. They provide insights into aspects like data flow rate, error count, or processing time, offering a comprehensive understanding of the system’s efficiency, reliability, and overall health. Logs Logs are textual records that document events, errors, and activities within a system. They provide a detailed narrative of system behavior and can include information such as error messages, timestamps, and user interactions. They are essential for troubleshooting as they offer a historical record of events, helping identify and rectify any issues in the data pipeline. Traces Traces provide a detailed view of how data requests move through a specific application, helping identify any bottlenecks or issues. Their scope, however, is limited to that particular application. To gain a comprehensive view of the entire system, traces are often used in conjunction with other tools like system metrics and network monitoring solutions. Key Concepts in Data Pipeline Monitoring To effectively monitor these pipelines, it’s important to understand some key concepts that form the foundation of efficient data pipeline monitoring, helping businesses optimize their data flow and make informed decisions. These include: Data Freshness Data freshness ensures that the information used for analysis and decision-making is current, thereby enhancing the accuracy of insights. Data Distribution Data distribution describes how data is spread across different values or ranges. It involves identifying and handling missing information and unexpected spikes or deviations from the anticipated range of values to prevent skewed results. Data Volume Data volume refers to the quantity of data that is generated and processed. Efficient handling of data volume prevents data overload and underutilization, ensuring optimization of data storage and processing capabilities. Data Schema Data schema refers to the structure of data, including its organization, format, and the relationships between different data elements. A well-defined data schema is vital for accurate data analysis and plays a significant role in maintaining the integrity and quality of the data. Data Lineage Data lineage involves tracking data from its source to its destination, including all the transformations it undergoes in the process. It ensures transparency and accountability of the data, aiding in tracing any errors or inconsistencies back to their source and improving the overall quality of the data. It is also crucial for regulatory compliance and data governance. 6 Essential Metrics for Data Pipeline Monitoring Data pipeline monitoring involves six important metrics that help assess the performance, efficiency, and reliability of the data flow. These metrics are: Latency: This metric measures the time it takes for data to move from the point of entry to its destination in the pipeline. High latency can indicate bottlenecks or performance issues within the pipeline. Throughput: This measures the amount of data that can be transported through the pipeline in a given time frame. It helps in understanding the capacity of the pipeline and can indicate if scaling is required. Error Rate: This metric tracks the number of errors that occur during data processing. A high error rate can indicate issues with data quality or problems in the pipeline’s processing capabilities. Availability: This is a measure of the data pipeline’s reliability and accessibility. It quantifies the percentage of time the pipeline is functional and capable of processing data without interruptions or failures. Data Drift Detection: This metric monitors for unexpected changes in the data’s characteristics or structure over time. It helps in identifying significant shifts in the data’s statistical properties that could impact data analysis and decision-making. System Health: This involves monitoring the operational parameters of the system running the data pipeline, such as CPU usage, memory usage, and network bandwidth. It helps in identifying any system-related issues that could affect the performance of the data pipeline. How Data Pipeline Monitoring Works Data pipeline monitoring plays a crucial role in establishing the groundwork for maintaining data integrity. Its main steps include: Instrumentation Instrumentation involves embedding monitoring tools or agents at strategic points within the data pipeline architecture. These tools act as sensors, capturing relevant data and metrics as the data moves through various stages of the pipeline. Instrumentation is the first step to enable continuous monitoring. Data Collection Once the monitoring tools are in place, they continuously collect data on different aspects of the data pipeline’s operation. This includes metrics such as data throughput, latency, resource utilization, and error rates. The collected data serves as the basis for assessing the performance and health of the data pipeline. Real-time Monitoring Real-time monitoring ensures that insights into the data pipeline’s status are available immediately. Monitoring tools process and analyze incoming data in real-time, allowing for the prompt detection of issues or anomalies. This timely awareness is crucial for maintaining the efficiency and reliability of the data pipeline. Alerting System An alerting system is configured to generate notifications or alerts based on predefined criteria. For example, if the data throughput drops below a certain threshold or if an error rate surpasses a predefined limit, the alerting system triggers notifications. Alerts enable data engineers and operators to respond quickly to emerging issues. Logging Logging involves recording detailed information about events, errors, and activities within the data pipeline. Logs serve as a historical record that can be used for auditing, debugging, and understanding the sequence of events, which are essential for post-incident analysis and troubleshooting. Best Practices for Data Pipeline Monitoring Effective data pipeline monitoring requires a strategic approach to ensure the reliability, efficiency, and accuracy of data flow. Here are some best practices: Establish Clear Metrics: Identify and define the key metrics that will provide insights into the pipeline’s health and performance. These metrics will serve as the foundation of your monitoring strategy. Implement Real-Time Monitoring: Use tools and solutions that allow for real-time monitoring of your data pipelines. This enables immediate detection and resolution of issues. Automate Alerts: Set up automated alerts to be triggered when predefined thresholds are reached. This ensures prompt attention to potential issues, even when you’re not actively monitoring the dashboard. Maintain End-to-End Visibility: Ensure your monitoring strategy provides a comprehensive, end-to-end view of your data pipeline. This allows for precise pinpointing of issues and a better understanding of data flow and transformations. Prioritize Data Quality: Implement checks at various stages of the pipeline to ensure data quality. This includes validating data formats, checking for missing values, and identifying any inconsistencies. Monitor Regularly: Establish a routine practice of regularly assessing your data pipeline’s performance and seek to make incremental improvements. This will help ensure your data pipelines remain efficient, robust, and capable of handling your evolving data needs. Monitor your Data Pipelines Seamlessly LIKE.TG’s Centerprise helps you seamlessly monitor your data pipelines using a user-friendly and easy-to-use interface. Try it now! Book Free Trial Start Monitoring Your Data Effectively Managing data pipelines is integral as it provides insights into their performance, enabling organizations to promptly identify and rectify any issues that may arise. By implementing robust monitoring, organizations can enhance their data analysis and gain a competitive edge. Organizations must build a strong data monitoring strategy and foster a culture of data awareness and responsibility across all levels of the organization. This will ensure that monitoring data pipeliens is not just a one-off task but an ongoing commitment. Need help building and manage your data pipelines? Start your 14-day free trial with LIKE.TG’s Data Pipeline Builder now! Take Control of your Data Pipelines Discover how LIKE.TG's advanced features empower your team to proactively manage, optimize, and future-proof your data workflows. Download Free Trial
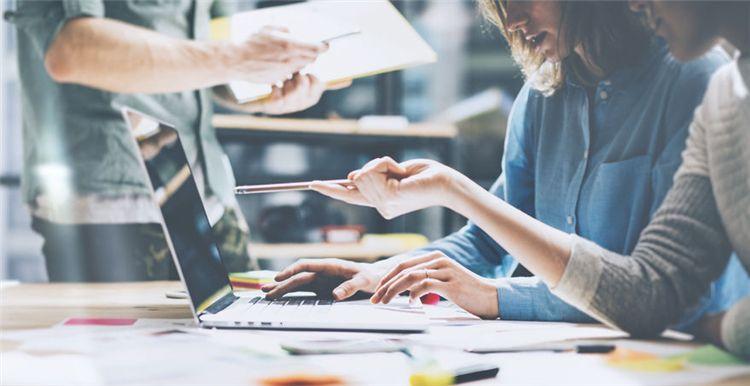
How to Build a Data Pipeline: A Step-by-Step Guide
Data plays a significant role in business growth and digital initiatives for approximately 94% of enterprises. However, the full potential of these data assets often remains untapped, primarily due to the scattered nature of the data. The solution lies in constructing a structured data pipeline, a task that can be resource-intensive and time-consuming. A simple pipeline may take weeks to build, while a more complex one could span months. Therefore, it is imperative for your organization to invest in appropriate tools and technologies to streamline the process of building a data pipeline. This blog details how to build a data pipeline effectively step by step, offering insights and best practices for a seamless and efficient development process. How To Build a Robust Data Pipeline Building a data pipeline is a multi-step process that requires careful planning and execution. Here are eight steps to build a data pipeline: Step 1: Define Your Goals The first step in building a data pipeline is to clearly outline your objectives. These could be to enable real-time analytics, facilitate machine learning models, or ensure data synchronization across systems. Your goals will guide the design, complexity, and scalability of your pipeline. Consider the specific data requirements, the frequency of data updates, and the desired speed of data processing and analysis. Step 2: Identify Data Sources Recognizing your data sources is a crucial step in building a data pipeline. Data sources can be broadly divided into six categories: Databases: Databases could be relational like MySQL, PostgreSQL, or non-relational like MongoDB, Cassandra. Cloud Storage: Data can also be stored in cloud platforms like AWS S3, Google Cloud Storage, or Azure Blob Storage. Real-time Data Streams: These are continuous data sources like IoT devices, social media feeds, or log files. File Sources: Data may originate from files, including formats such as CSV, Excel, JSON, or other file-based storage systems. APIs (Application Programming Interfaces): Data can be extracted from APIs that provide a direct connection to various web services and external platforms.” Understanding the nature, format, and volume of these data sources is essential as it impacts the subsequent stages of ingestion and transformation. Step 3: Determine the Data Ingestion Strategy Next, you need to extract, integrate and incorporate data from diverse sources into your pipeline. There are two primary methods of data ingestion: Batch Ingestion: This method involves collecting data over a specific period and processing it as a group. It’s suitable for large volumes of static data where real-time insights are not required. Real-time Ingestion: In this method, data is processed immediately as it arrives. It’s ideal for scenarios where real-time insights are crucial, such as fraud detection or system monitoring. Step 4: Design the Data Processing Plan You’ll need to clean, validate, and structure the ingested data into a format suitable for analysis. There are two main approaches to data transformation: ETL (Extract, Transform, Load): Here, you extract data from the source onto a staging server, transform it, and then load it into the destination. This approach is typically used when dealing with smaller volumes of data or when the transformed data needs to be used by multiple downstream applications. ELT (Extract, Load, Transform): In this approach, you extract data from the source, load it into the destination, and then transform it within the destination server. This eliminates the need to load data on a staging server, making it preferable for handling larger volumes of data in a big data environment. The data transformation process in ELT is similar to ETL but occurs after the data has been loaded into the destination. Step 5: Decide Where to Store the Information Next, you’ll store the processed data for further use. This could be a traditional data warehouse like Amazon Redshift for structured data, a data lake like Google Cloud Storage for semi-structured or unstructured data, or a database system like PostgreSQL for transactional data. The choice of destination depends on the nature of the data, the use case, and the specific data analytics requirements. Step 6: Establish the Workflow At this stage, it’s important to define the sequence of operations in the pipeline. This includes specifying the order of tasks, managing dependencies between tasks, handling errors, and setting up retries or notifications in case of failure. This step is crucial to ensure the smooth operation of the pipeline and to handle any issues that might arise promptly. It involves creating data validation checks and defining procedures for error handling and recovery. Step 7: Set a Monitoring Framework It’s crucial to monitor data pipeline performance to ensure it’s working as expected. This involves tracking the flow of data through the pipeline, checking for errors or bottlenecks that might slow down data processing, and monitoring resource usage to ensure the pipeline is operating efficiently. Monitoring helps detect and resolve issues before they affect the performance of the pipeline or data quality. Step 8: Implement Data Consumption Layer Finally, you’ll need to create a reliable interface through which end-users or applications access the processed data. This could be a business intelligence (BI) tool that provides data visualization capabilities, a reporting tool that generates detailed reports, or an API that allows other applications to interact with the data. The data consumption layer needs to be designed to easy access to the data. Best Practices for Building Data Pipelines Streamline Development and Deployment: Implementing Continuous Integration/Continuous Deployment (CI/CD) is key here. CI/CD automates the process of integrating code changes and deploying those changes to the production environment. This means that any updates or improvements to the pipeline can be implemented rapidly and reliably, ensuring the pipeline is always operating at its best. Maintain Consistency: Version control systems help achieve consistency in collaborative development environments. They track and manage changes to the codebase, enabling simultaneous work without overwriting changes and providing the ability to revert to previous code versions when necessary. Optimize Data Management: Data partitioning divides a large database into smaller, more manageable segments based on specific criteria, improving query performance and streamlining data management tasks. Leverage Modern Architectures: Modern software architectures like containers and microservices can significantly improve the scalability and reliability of your data pipeline. Containers encapsulate an application and its dependencies into a single unit that can run consistently across platforms. Microservices create an application as a collection of independent services, promoting better scalability and easier debugging. Ensure Data Security: As data moves through various stages of the pipeline, from extraction and transformation to loading, it’s crucial to protect it from unauthorized access and potential breaches. This involves implementing data encryption, access controls, and regular vulnerability testing to protect sensitive information. Compliance with relevant data safety regulations, such as GDPR and HIPAA, is essential, particularly in sectors dealing with personal data, such as healthcare and finance. Shifting from Manual to Automated Data Pipelines Building data pipelines manually (Python for data pipelines) is inherently a time-consuming and resource-intensive process, making it susceptible to delays, errors, and inefficiencies. The transition to automated data pipeline tools addresses these challenges, streamlining processes for greater efficiency and resource optimization. These advanced tools play a pivotal role in streamlining the entire process, offering advantages such as reduced development time, minimized errors, and enhanced scalability. By automating repetitive tasks, they allow organizations to expedite pipeline development while maintaining a high level of accuracy. Create Data Pipelines Easily with LIKE.TG LIKE.TG’s Data Pipeline Builder brings all of these automation capabilities together. It simplifies data integration, offers scalability, and comes equipped with features to maintain data security and compliance. With its user-friendly interface and powerful features, LIKE.TG’s solution reduces the effort and time needed to build a robust data pipeline, allowing businesses to focus more on leveraging their data for insights and decision-making. Building a data pipeline with LIKE.TG takes only five steps: Data Extraction: LIKE.TG allows you to extract data from a wide range of sources. This includes various databases like SQL Server, Oracle, and MySQL, file formats such as Excel, CSV, XML, and JSON, and cloud applications like Salesforce and Microsoft Dynamics 365, ensuring a comprehensive data collection process. Data Transformation: Once the data is extracted, LIKE.TG provides a variety of transformation functions that you can use to clean, format, and structure your data as per your requirements. For example, you can use these functions to remove duplicates, convert data types, or aggregate data based on specific criteria. Data Loading: After the data is transformed, LIKE.TG enables you to load it into your desired destination, be it a database, a data warehouse, or a file system. You could load the data into an SQL Server database, a cloud-based data warehouse like Amazon Redshift, or even export it as a CSV file. Automation and Scheduling: LIKE.TG also provides features for automating and scheduling your data pipeline tasks. This means that once you’ve set up your pipeline, you can have it run automatically at scheduled intervals or triggered by certain events, reducing manual effort and ensuring your data is always up-to-date. Monitoring and Error Handling: LIKE.TG provides real-time monitoring capabilities, allowing you to track the performance of your data pipeline and quickly identify and resolve any issues. Upgrade from manual to automated data pipelines today! Download LIKE.TG Data Pipeline Builder’s 14-day free trial and transform your data workflows. Build Automated Data Pipelines With Just a Few Clicks! With LIKE.TG's robust features and automation capabilities, you can effortlessly build and deploy data pipelines, saving time and enhancing efficiency. Download Free Trial
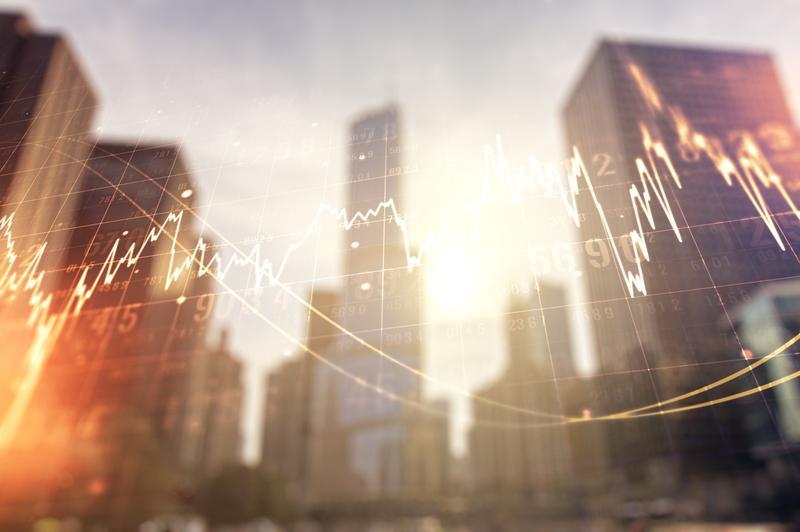
The Role of Data in Personalized Banking
With over 70% of customers emphasizing the importance of personalized offers in banking, it’s evident that people highly value tailored experiences from their financial institutions. However, despite this strong customer preference, only 14% of banks have embraced personalized banking. This reveals a significant gap between customer expectations and the services currently provided by the finance industry. Bridging this gap requires leveraging the role of data. Personalized banking experiences rely on utilizing customer information and insights derived from data. These insights are crucial in shaping services that align more precisely with individual preferences and needs. What is Personalized Banking? Personalized banking focuses on delivering unique experiences to customers based on their financial needs and preferences outlined by behaviors. This personalized approach goes beyond the one-size-fits-all approach of traditional banking services. Banks collect vast amounts of customer data, ranging from transaction history to online browsing behavior. By leveraging this data for analytics, banks can gain valuable insights into consumer behavior and provide customized recommendations and offers. Modern banks recognize the importance of personalization in every aspect of their operations—be it customer acquisition, service, onboarding, or overall engagement. Benefits of Personalized Banking Customer Satisfaction and Loyalty: Personalization enhances customer satisfaction by addressing specific financial needs, making customers feel valued and understood. This tailored approach fosters a deeper connection with the bank, leading to increased loyalty and retention rates. Banks can also offer rewards programs where customers receive discounts, cashback, or exclusive deals based on their spending patterns. Tailored Financial Advice: Banks can provide individualized financial guidance, such as personalized investment strategies based on a customer’s income, risk apetite, and goals. This not only improves customer satisfaction but also increases the likelihood of achieving financial goals. Increased Revenue Opportunities: Through data analysis, banks can identify additional services or products that align with customers’ needs, leading to cross-selling and upselling opportunities. This targeted approach benefits both customers and the bank by expanding product offerings and revenue streams. Financial Literacy and Education: Personalized banking includes providing dedicated educational materials to improve customers’ understanding of complex financial concepts. Educating customers contributes to their financial well-being and strengthens long-term relationships. Source: info.blend.com How are Banks Using Personalized Banking? Targeted Product Recommendations Banks analyze customer spending patterns and offer personalized recommendations for credit cards, loans, or investment products that align with the customer’s financial goals. For instance, imagine a customer who frequently shops at grocery stores and spends a significant portion of their income on groceries. The bank would analyze this customer’s transaction history and identify their spending patterns. Based on this information, they might recommend a credit card that offers cashback or rewards specifically for grocery purchases. This personalized recommendation not only helps the customer maximize their savings, but also strengthens their relationship with the bank. Customized Marketing Campaigns Banks personalize their marketing efforts by sending targeted offers based on customers’ transaction history and preferences. For example, a customer who frequently travels may receive offers for travel insurance or foreign currency services. Consider a customer who frequently uses their credit card to book flights and hotels. A bank utilizing personalized banking would analyze this customer’s transaction history and identify their travel-related expenses. Armed with this knowledge, the bank could tailor their marketing campaigns to offer the customer exclusive travel benefits, such as discounted travel insurance or preferential foreign currency exchange rates. By providing personalized offers that align with the customer’s interests, the bank enhances the customer’s experience and increases the likelihood of them engaging with the bank’s services. Personalized Customer Service Banks use customer data to provide personalized customer service experiences. For instance, a customer calling the bank’s helpline will be routed to a representative who specializes in their specific financial needs. Imagine a customer who recently started a small business and needs guidance on managing their business finances. With personalized banking, when this customer calls the bank’s helpline, their call is directed to a representative who specializes in assisting small business owners. This representative would have a deep understanding of the challenges and opportunities faced by small businesses, allowing them to provide tailored advice and support to the customer. Overcoming Challenges in Data-Driven Personalized Banking While data-driven banking offers numerous benefits, it also poses challenges. Banks need to address these roadblocks effectively to ensure their strategies are successfully impelemented. Legacy Infrastructure: Older data processing systems often struggle with: Utilizing Unstructured Data: Inability to process and derive insights from the rapidly growing unstructured and alternative data sets. Open Data Sharing: Lack of capabilities for open data sharing, hindering collaboration and integration with external sources. Financial institutes should invest in modernizing their infrastructure to handle unstructured data efficiently. Upgrading data integration systems and adopting advanced analytics tools can aid in this process. Additionally, fostering partnerships and embracing open APIs can facilitate seamless integration with external sources. Strict Data Regulations: Increasing concerns about data breaches have led to: Consumer Caution: Heightened consumer wariness in sharing personal data due to high-profile instances of data theft and breaches. Regulatory Compliance Burden: Stricter regulations necessitate more comprehensive measures to safeguard customer information, adding complexity and cost to operations. Implementing robust data protection measures such as advanced encryption technologies and multi-factor authentication is crucial. Banks should also regularly audit data access and invest in monitoring systems to ensure compliance. Transparent communication about data privacy policies is essential to build trust and reassure consumers about the security of their information. Access to Third-Party Data: Issues in accessing and leveraging external data arise due to: Disjointed Tools: Challenges in performing real-time analytics and democratizing financial decisions due to fragmented tools and systems. Banks can diversify their data sources by reducing dependency on single vendors or technologies. Integration of unified analytics platforms and adopting standardized tools across business units can streamline data access and enable real-time analytics for better decision-making. Data Silos: Internal challenges revolve around: Complex Workflows: Highly intricate processes hinder collaboration and smooth data flow. Disparate Technologies: Using multiple systems across various business units creates data silos, preventing cohesive insights and decision-making. Banks should focus on streamlining workflows by implementing centralized data management systems. Integration of technologies across departments and fostering a culture of data sharing and collaboration can break down silos, enabling better data flow and cohesive insights for decision-making. The Role of ETL in Personalized Banking ETL is a process of extracting data from different sources, transforming it into a standard format, and then loading it into a target system. In personalized banking, ETL plays a crucial role in enabling data-driven decision-making and delivering personalized experiences to customers. Extract The extraction phase involves gathering customer-centric data from numerous sources. This process includes pulling essential information from internal systems, such as transaction logs, customer databases, and account histories. External sources also play a vital role in enriching this data pool. These sources might include social media platforms, where individuals may engage with financial institutions or express preferences that can inform personalized services. Moreover, data from credit bureaus and other financial institutions can offer insights into credit histories, loan obligations, and other relevant financial behaviors, contributing significantly to the holistic view of a customer’s financial profile. This extracted data forms the foundation upon which various analytics and machine learning models operate. Transform During the cleansing process, data is carefully examined, and any inconsistencies, errors, or duplicates are identified and corrected. For example, if a customer’s name is misspelled in one source, the ETL process can be designed to ensure consistent customer information across all relevant datasets. This attention to detail is essential in personalized banking, as even small errors can lead to incorrect recommendations or decisions. is another critical step in the ETL process. It involves combining data from multiple sources. Integration can be time-consuming, especially when dealing with large volumes of data. However, ETL tools, such as LIKE.TG, have advanced significantly, making integration more efficient and seamless. During the transformation phase, companies can apply enrichment as an additional step. It involves enhancing the data with additional information to provide a more comprehensive view. For example, demographic data, such as age, gender, or location, can be added to customer records to enable better segmentation and targeting. Load Finally, the transformed data is loaded into a centralized data warehouse or data mart, where it can be further analyzed and utilized for personalized banking initiatives. This unified data enables banks to gain a 360-degree view of their customers, leading to meaningful insights and personalized recommendations. How Does LIKE.TG Help Financial Institutions with Personalized Banking? LIKE.TG empowers financial institutions to craft personalized banking experiences by offering a comprehensive suite of features, ensuring seamless integration, data security, compliance, and operational efficiency. Here’s how Asters cater to your needs: Intuitive Drag-and-Drop Interface: LIKE.TG streamlines operations with a no-code interface, allowing seamless data management to facilitate personalized banking solutions. Extensive Connectivity to Diverse Data Sources: Seamlessly integrate data from various sources to ensure a comprehensive view of your customer data. LIKE.TG facilitates connectivity, bridging gaps between disparate data sources for a unified perspective. Library of Pre-Built Transformations: Accelerate data processing with our pre-built transformations, effortlessly manipulating and enriching data for actionable insights. Flexible Data Pipeline Builder for ELT and ETL: Construct tailored data pipelines with an industry0grade ELT and ETL engine. LIKE.TG ensures efficient data processing from extraction to loading, meeting specific institutional needs. Simplified Data Mapping: Streamline complex data mapping processes for precision and accuracy, enabling efficient mapping of data elements. Efficient Workflow Automation: Increase operational efficiency with automated workflows, orchestrating processes seamlessly for a more personalized service. Real-Time Change Data Capture (CDC): Stay updated with LIKE.TG’s Change Data Capture feature, integrating real-time changes for swift responses and personalized services. Secure Managed File Transfer (MFT): Safeguard critical data with our Managed File Transfer capabilities, ensuring the secure flow of sensitive information within your data ecosystem. Robust Security Measures: Prioritize data security with encryption protocols, role-based access control, and publishing workflows deployed on-premise, ensuring utmost security for personalized banking data. Compliance Alignment (e.g., GDPR): LIKE.TG helps align with global data regulations such as GDPR, ensuring banking services are delivered ethically and responsibly. Ready to revolutionize your banking experience? Experience the power of personalized services with LIKE.TG. Sign up for a demo or a 14-day- free trial now to discover the future of finance!
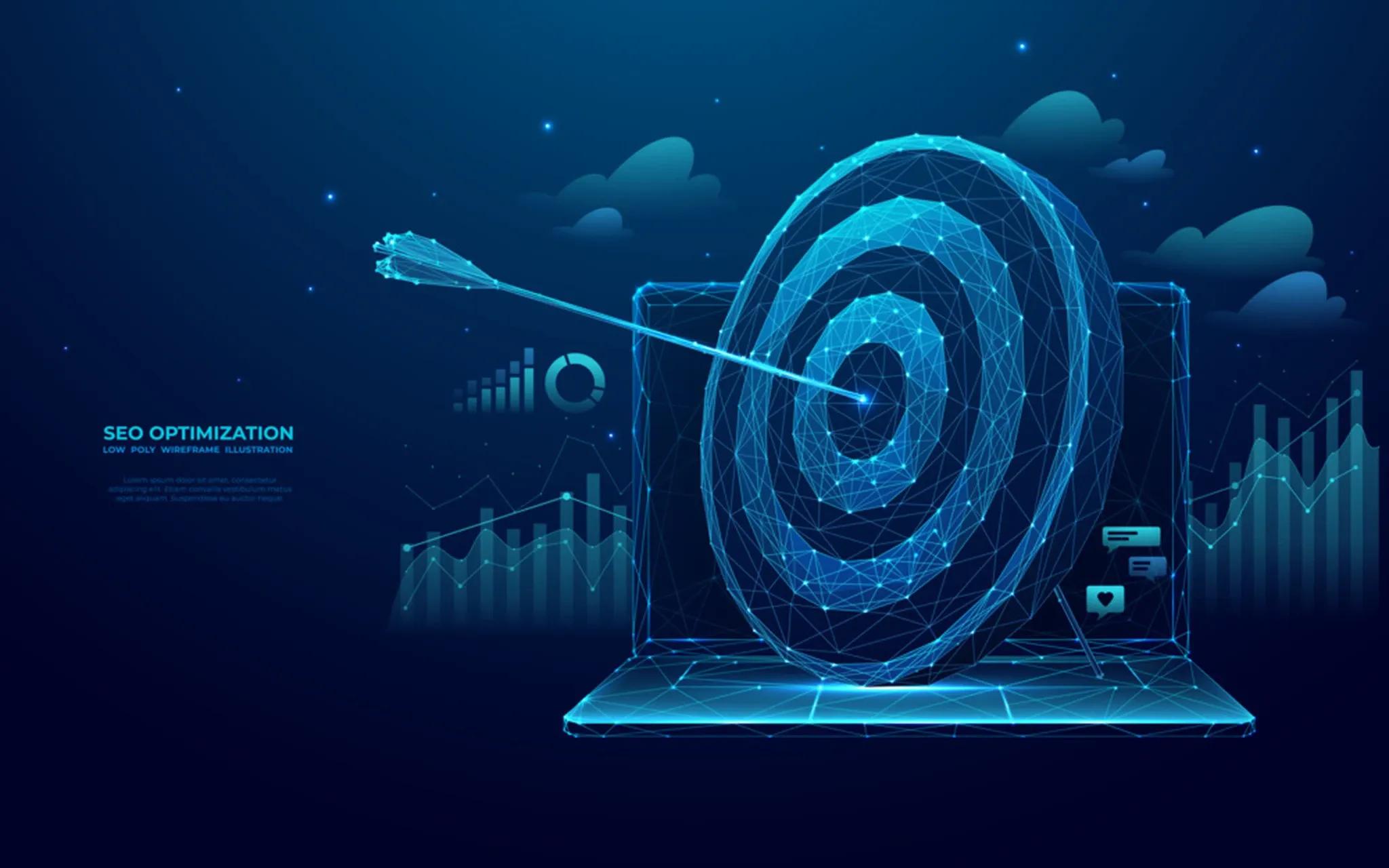
Data Profiling: Types, Techniques and Best Practices
Clean and accurate data is the foundation of organizations’ decision-making processes and is the reason why they’re heavily investing in data quality solutions. The global Data Quality Tools market was valued at $3.23 billion in 2023, and projections show that it’ll cross the $8 billion mark by 2030. Obtaining high-quality data is among the primary objectives of data preparation across different industries and sectors. This is where data profiling comes into play. It provides organizations with a general overview of all their data, which they can then use to detect errors and inconsistencies. These insights enable them to promptly rectify issues, make informed decisions, and enhance operational efficiency. Let’s dive into the specifics of data profiling and how it helps in data preparation. What is Data Profiling? Data profiling ensures that the data is in good health and fit for its intended use. It is essentially the first step in the process of managing and using data. Data profiling can uncover a range of data quality issues, such as missing data, duplication, and inaccuracies. It also highlights patterns, rules, and trends within the data. This information is crucial as it helps organizations improve data quality, streamline data transformation, and make informed decisions. Types of Data Profiling Data profiling can be classified into three primary types: Structure Discovery: This process focuses on identifying the organization and metadata of data, such as tables, columns, and data types. This certifies that the data is consistent and formatted properly. For instance, in a healthcare database, structure discovery reveals the presence of tables like “Patients” and “Appointments” with columns such as “PatientID,” “AppointmentDate,” and data types like “integer” and “date.” Content Discovery: This involves a deep dive into the actual content of the data. It examines individual data records to identify errors. For example, in a customer database, content discovery reveals that the “Phone Number” column contains numerous missing values, highlighting incomplete contact information for certain customers. Relationship Discovery: This process identifies the relationships and dependencies between different data elements. For instance, in a retail database, relationship discovery would analyze the associations between different fields and tables, such as the relationship between the ‘Customers’ table and the ‘Orders’ table, understanding how different data elements are interconnected and how they influence each other. Data Profiling Techniques Profiling data involves a variety of techniques that help analyze, assess, and understand data. Four primary techniques are: Column Profiling: This technique analyzes each column in a database. It looks at the type of data in the column, how long the data is, and if there are any empty values. A crucial part of this process is frequency analysis, which counts how often each value appears, helping to spot patterns and unusual values. Cross-Column Profiling: Here, the focus is on the relationships between different columns within the same table. It includes key and dependency analysis. Key analysis finds columns where each row has a unique value, while dependency analysis looks at how values in one column depend on values in another column. This can help find connections, overlaps, and inconsistencies between columns. Cross-Table Profiling: This method looks at relationships between different tables in a database. It includes foreign key analysis, which finds columns in one table that match up with unique key columns in another table. This helps show how data in one table is related to data in another table and can provide important information about the structure and accuracy of the database. Data Validation and Cleansing: This approach involves verifying the accuracy and quality of data against specific criteria or standards. It includes format checks, range checks, and consistency checks to ensure data is clean, correct, and logically consistent. Understanding the Difference: Data Profiling vs. Data Mining Data profiling and data mining are two distinct processes with different objectives and methodologies. Data profiling is the initial step in data preparation, focusing on understanding the data’s basic characteristics, quality, and structure. It helps identify data issues like missing values or anomalies. This helps ensure that data is clean and reliable for further use. In contrast, data mining involves exploring the data to discover hidden patterns, trends, and valuable insights using advanced techniques like machine learning. These techniques can help with different tasks including: Pattern recognition Classification and prediction Clustering Anomaly detection Association rule mining Feature selection and dimensionality reduction Text and image mining Model evaluation and optimization Data Profiling Benefits Data profiling offers a multitude of specific benefits that can significantly enhance an organization’s data management strategy. Here are some of the distinct advantages of data profiling: Informed Decision-Making: Data profiling provides a clear understanding of the available data, its quality, and its structure. This knowledge aids in making informed, data-driven decisions, thereby improving strategic planning and operational efficiency. Increased Operational Efficiency: It helps in identifying and eliminating redundant or irrelevant data. This leads to improved efficiency of data processing and analysis, resulting in faster insights, improved productivity, and a better bottom line. Risk Mitigation: Data profiling can help businesses identify potential risks and issues in their data, such as compliance violations or security threats. By addressing these issues proactively, businesses can mitigate risks and avoid costly penalties or damage to their reputation. Cost Savings: By improving data quality and efficiency, data profiling can lead to significant cost savings. Businesses can avoid the costs associated with poor-quality data, such as inaccurate decisions, wasted resources, and lost opportunities. Compliance Assurance: Data profiling can help businesses ensure compliance with industry regulations and standards. By addressing compliance issues, businesses can avoid legal complications and maintain their credibility in the market. Data Profiling Challenges Understanding the challenges and limitations of data profiling is key to ensuring data profiling methods’ effectiveness. Here are some of the data quality challenges and how to overcome them: Scalability When datasets grow and become increasingly complex over time, conventional data profiling techniques may prove insufficient for handling the growing data volume. Left unchecked, this can make data profiling tasks more resource-intensive and time-consuming — ultimately delaying data preparation and disrupting timely analysis. Organizations can address scalability issues by implementing parallel processing. Parallel processing techniques distribute profiling tasks across multiple nodes or processors. This measure improves efficiency and significantly reduces processing time for larger datasets. Resource Requirements Data profiling can require substantial computational resources such as memory, storage capacity, and processing power. Insufficient resources create performance bottlenecks and slow down profiling processes, impacting productivity and efficiency. Organizations can mitigate bottlenecks and boost productivity by optimizing resource allocation in the following ways: Investing in scalable infrastructure, such as cloud-based solutions, to ensure flexibility. Implementing dynamic resource allocation based on changing workload requirements. Complex Data Structures Modern data environments typically feature diverse data formats and structures. They also contain large amounts of semi-structured and unstructured data. Conventional data profiling techniques may not be as useful for analyzing such complex data structures, causing them to generate inaccurate or incomplete profiling results. Fortunately, enterprises can resolve this by incorporating advanced profiling techniques such as natural language processing and machine learning algorithms. These modern techniques can identify relationships and patterns within unstructured and semi-structured data and aid in obtaining more accurate profiling results. Data Privacy and Security Accessing and analyzing sensitive information — such as confidential business data and personally identifiable information — are standard components of data profiling. Maintaining data privacy and security throughout the profiling process is essential, as it helps defend data against data breaches, unauthorized access, and regulatory non-compliance. Organizations can resolve data privacy concerns by integrating data anonymization and masking techniques into their profiling methods. Doing so facilitates meaningful analysis while comprehensively protecting sensitive information. 5 Best Practices of Data Profiling When performing data profiling, organizations follow some best practices to ensure accurate results and efficient analysis: Define Clear Objectives: Clearly define the goals, objectives, and expectations to ensure it’s aligned with business needs and requirements. Choose Relevant Data Sources: Select relevant data sources based on their importance, relevance, and potential impact on decision-making processes. Establish Data Quality Metrics: Define appropriate metrics and validation rules to assess the quality and accuracy of data based on business requirements and industry standards. Document Data Profiling Results: Document and communicate the findings, recommendations, and actions taken during data profiling to facilitate understanding, accountability, and compliance. Regularly Monitor Data Quality: Implement regular data quality monitoring processes to ensure data consistency, accuracy, and compliance over time. Data Profiling Made Easier with LIKE.TG LIKE.TG's drag-and-drop, zero-code functionality simplifies the data profiling process, helping you quickly assess and understand your data. Start your free trial today! Start a Free Trial Applications of Data Profiling Data profiling finds applications in various areas and domains, including: Data Integration and Data Warehousing: Data profiling facilitates the integration of multiple datasets into a centralized data warehouse, ensuring data accuracy, consistency, and compatibility between sources. Data Migration and System Development: Before migrating data from one system to another or developing new software systems, data profiling helps identify potential data issues, detect data schema and structure, assess data distribution and patterns, and understand data dependencies and relationships. Data Analytics and Business Intelligence: By understanding the quality, structure, and relationships within data, data profiling empowers organizations to generate more accurate insights, make data-driven decisions, and enhance overall business intelligence. Data Profiling’s Role in Data Governance and Compliance Data profiling is vital in supporting organizational data governance and compliance initiatives. Data governance comprises all policies, processes, and controls that ensure data assets’ availability, integrity, and security. In contrast, compliance involves adherence to regulatory requirements and the industry standards governing data handling and usage. Here are five ways data profiling contributes to data governance and compliance: Data Quality Assessment: Data profiling serves as the first step in determining data assets’ quality. Analyzing the structure, content, and relationships within the data reveals any inconsistencies, inaccuracies, and anomalies that can potentially damage data integrity and impact compliance. Risk Identification and Mitigation Data profiling enables organizations to identify potential risk factors that can compromise data quality, privacy, and security. This can help them proactively deal with issues that can threaten compliance — such as regulatory violations, data breaches, or inaccurate reporting. Data Classification and Tagging Data profiling allows businesses to classify and tag data based on its regulatory requirements, sensitivity, and criticality. Understanding data attributes’ nature and context simplifies the application of relevant data classification policies and access controls.This helps organizations comply with privacy regulations such as the California Consumer Privacy Act (CCPA) and the General Data Protection Regulation (GDPR). Monitoring and Auditing Data profiling supports an organization’s ongoing monitoring and auditing protocols to maintain compliance with data governance policies and regulations. Creating baseline profiles of their data assets allows enterprises to observe data quality, integrity, and usage patterns consistently. It also helps them spot deviations that may warrant further investigation or corrective measures. Documentation and Reporting Data profiling results offer meaningful insights into the metadata of data assets by examining their structure and content. These insights are important for documentation and reporting initiatives. Organizations can utilize profiling reports to demonstrate their compliance with regulatory mandates, audit requirements, and internal governance policies. Conclusion As organizations continue to harness the power of data for competitive advantage, data profiling remains critical in ensuring data quality. By systematically examining and evaluating data, organizations can ensure data accuracy, reliability, and compliance, leading to more informed decision-making and better business outcomes. To ensure that high-quality data is being used for analysis, it’s crucial to invest in advanced data profiling tools. LIKE.TG stands out as a comprehensive solution that offers advanced data profiling, cleansing, and validation capabilities. It provides real-time health checks that continuously monitor your data quality as you work, providing immediate feedback on its overall health. LIKE.TG’s capabilities extend to both global and field-level data analysis, enabling early identification of irregularities, missing values, or anomalies. This proactive approach to data quality allows for timely measures to be taken to rectify any issues. LIKE.TG’s drag-and-drop visual interface empowers business users to examine and evaluate the data, facilitating necessary adjustments as needed. Therefore, LIKE.TG simplifies the data profiling process and enhances data accuracy, reliability, and overall quality, enabling improved operational efficiency and better business outcomes. Want to learn more about data profiling and how LIKE.TG streamlines the entire data prep process? Download your free whitepaper now!
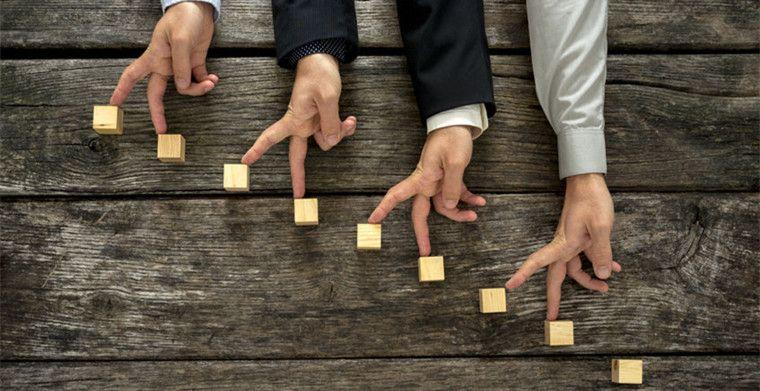
API-Led Connectivity: A Complete Guide
Today, APIs are everywhere —so much so that the global API market is set to reach USD 6263.00 million by 2028. Among the usage of APIs, API-led connectivity is a new approach, revolutionizing how systems, applications, and data interact. This disruptive technology has emerged as the backbone of seamless connectivity by playing a significant role in retrieving information from diverse sources. What is API-led Connectivity? API-led connectivity is an approach to connecting and integrating different applications, systems, and data sources using APIs as the primary building blocks. This approach organizes the development of integrations and connections between various systems based on reusable and manageable APIs. It involves creating and exposing APIs that encapsulate specific functionalities or data sets, allowing different systems to interact and share information in a secure and controlled manner. Why is API-led Connectivity Important? API-led connectivity revolutionizes digital interactions. They empower businesses to maximize the potential of their existing applications and systems. By seamlessly integrating various components through APIs, organizations can overcome traditional data silos. This fosters a more interconnected data ecosystem. Through this approach, businesses unlock numerous benefits. Firstly, they can enhance operational efficiency by breaking complex systems down into manageable parts. This not only streamlines processes but also facilitates easier integration, enabling a more agile and innovative environment. Furthermore, by promoting the flow of information across previously isolated departments or systems, valuable insights are revealed, enabling informed decision-making. Security is paramount in this interconnected landscape. APIs serve as gateways that ensure controlled access to information, safeguarding sensitive data. Ultimately, API-led connectivity doesn’t just improve internal operations. It transforms customer experiences. By leveraging APIs to optimize services, businesses create seamless digital ecosystems that deliver enhanced experiences to customers. API-Led Connectivity Architecture API-led connectivity follows a well-defined architectural approach that consists of three key components: System APIs System APIs serve as a standardized interface, exposing the functionalities of back-end systems or applications to facilitate seamless interaction with various front-end applications. They act as a bridge, enabling easy access and communication between different services ensuring that resources are readily available for utilization across diverse applications. Here are a few examples of System APIs: Operating System APIs: These APIs allow applications to interact with the operating system. For instance, Windows API or POSIX (Portable Operating System Interface) for Unix-like systems. Database APIs: Such as JDBC (Java Database Connectivity) or ODBC (Open Database Connectivity) that enable applications to communicate and interact with databases like MySQL, PostgreSQL, or Oracle. Cloud Service APIs: For example, Amazon Web Services (AWS) API, Google Cloud API, or Microsoft Azure API allow developers to access and manage cloud services like storage, computing resources, and machine learning tools. Messaging APIs: Services like Twilio offer APIs for sending and receiving SMS, MMS, and other communication services programmatically. Process APIs Process APIs simplify complex integrations by orchestrating and composing multiple system APIs. These APIs act as a higher-level abstraction layer. They simplify complex integrations by providing a unified interface to fulfill specific business processes or use cases. Process APIs ensure that the different systems and applications work together harmoniously by orchestrating the sequence and flow of data between them. The result? Streamlined business operations and improved efficiency. Here are a few examples: Order Processing: Consider an e-commerce platform that involves multiple steps—like inventory check, payment processing, shipping, and notification. A process API can orchestrate communication between inventory management APIs, payment gateways, shipping APIs, and notification services to ensure a smooth order processing flow. Customer Onboarding: Banks or financial institutions may have a complex onboarding process involving identity verification, credit checks, account creation, and notifications. A process API can orchestrate interactions between various APIs handling these tasks, ensuring compliance and a seamless experience for the customer. Travel Booking: Travel agencies often integrate with airlines, hotels, car rental services, and payment gateways. A process API can coordinate communication between these different APIs to manage flight bookings, hotel reservations, car rentals, and payment processing for a comprehensive travel booking system. Experience APIs Experience APIs focus on delivering seamless and personalized experiences to end-users, abstracting the underlying complexities of the integrated systems. They aggregate diverse data sources by acting as a unified interface, transforming this information into formats tailored for specific channels and devices. This ensures that end-users encounter consistent, optimized experiences across various touchpoints. Some examples nclude: E-commerce Platforms: Companies like Amazon or eBay leverage Experience APIs to unify product information, user preferences, and purchase history from diverse sources. These APIs ensure a seamless shopping experience across devices, recommending personalized products and streamlining the checkout process. Hospitality and Travel: Booking platforms such as Airbnb or Expedia use Experience APIs to aggregate data from multiple providers, including accommodations, transportation, and activities. This integration allows users to seamlessly plan and book entire travel experiences through a single interface. Streaming Services: Platforms like Netflix or Spotify utilize Experience APIs to collect user data, including viewing or listening history, preferences, and device information. They then leverage this data to provide personalized content recommendations, creating a cohesive and engaging user experience. How API-Led Connectivity Works The API-led connectivity approach follows a structured flow that ensures seamless integration and efficient data flow. It involves: Discovering and Creating APIs:Identify the systems and data sources that need to be integrated and define the APIs that will expose their functionalities. This involves understanding the existing landscape of applications and systems within an organization and determining the key functionalities that need to be exposed through APIs. Once the systems and data sources are identified, APIs are created to expose their functionalities, providing a standardized interface for accessing and interacting with their resources. Building and Implementing APIs:Develop and deploy the APIs, ensuring they adhere to industry standards and best practices for security, scalability, and reliability. This involves implementing the necessary logic and functionality to expose the desired functionalities of the back-end systems or applications. It is crucial to ensure that the APIs adhere to industry standards and best practices for security, scalability, and reliability. By following these standards and best practices, organizations can ensure that their APIs are secure, performant, and capable of handling the expected load. Orchestrating APIs:Use process APIs to orchestrate the sequence and flow of data between different systems and applications, ensuring they work together harmoniously. Process APIs enable the seamless flow of data between different systems and applications by defining the sequence and logic of how the APIs should interact with each other. By orchestrating the APIs, organizations can ensure that the data flows smoothly and that the systems and applications work together harmoniously to fulfill specific business processes or use cases. Managing and Monitoring APIs:Implement a robust API management platform to manage, secure, and monitor the APIs throughout their lifecycle. Organizations that implement a robust API management platform can better manage, secure, and monitor the APIs throughout their lifecycle. These solutions include versioning and lifecycle management, security and access control, rate limiting and throttling, analytics and monitoring, and developer portal management. By effectively managing and monitoring the APIs, organizations can ensure their availability, performance, and security, enabling them to deliver a seamless and reliable user experience. Benefits of Implementing API-Led Connectivity API-led connectivity offers several advantages, making it important in modern software development and integration practices: Maximizing Existing Investments: It allows organizations to connect and integrate existing applications and systems without replacing them entirely. This maximizes the value of these assets, saving time, resources, and minimizing disruptions to ongoing processes. Data-Driven Decision-Making: Utilizing APIs to integrate diverse data sources enables businesses to gain a comprehensive overview of operations and customer behaviors. This integrated approach not only facilitates informed decision-making for growth and competitive advantages but also ensures a seamless and consistent customer experience across various platforms Security and Control: APIs provide a structured way to manage data access, allowing for fine-grained control over who accesses what data and under what conditions, thus enhancing security measures. Modularity and Reusability: API-led connectivity encourages a modular approach where specific functionalities of a system or application are exposed through APIs. This modularity allows for easier reuse of these APIs across various projects and applications, fostering efficiency and consistency. Vendor Neutrality: It reduces dependency on specific technologies or vendors. APIs provide a standardized way to communicate between systems, making it easier to swap out components or technologies without major disruptions. Overcoming Challenges in API-Led Connectivity As API-led connectivity becomes increasingly prevalent, organizations face various challenges that need to be addressed for successful implementation and operation. Security Concerns One of the primary challenges is ensuring the security of the APIs. As APIs become involved in integrations, they handle sensitive data and provide access to critical systems. Therefore, implementing robust security measures is of paramount importance. Implementing strong authentication mechanisms enables organizations to verify the identity of users and systems accessing the APIs. This can involve using techniques such as API keys, OAuth, or even biometric authentication. For example, banking apps often employ biometric authentication (fingerprint or facial recognition) for accessing financial APIs. This technology verifies the user’s identity before allowing access to account details or transactions. Another crucial aspect of API security is encryption. Organizations can prevent unauthorized access and protect sensitive information by ensuring they transmit sensitive data through encrypted APIs. This can be achieved by implementing secure communication protocols such as HTTPS and using encryption algorithms like AES and DES to encrypt the data at rest. Integration Issues Integrating different systems with varying protocols, data formats, and standards can pose significant challenges. Organizations often have a mix of legacy systems, modern applications, and third-party services that need to be seamlessly integrated to enable efficient data flow. To address integration issues, organizations need to carefully plan and design their integration architecture. This involves analyzing the systems and applications to be integrated, understanding their data requirements, and identifying any potential conflicts or compatibility issues. Organizations can adopt an API-led approach to integration, where APIs act as the building blocks for connecting different systems. By designing APIs that abstract the underlying complexity of systems and provide a standardized interface, organizations can simplify the integration process and ensure seamless data flow. Furthermore, organizations can leverage integration platforms and tools that provide pre-built connectors and adapters for popular systems and applications. These tools can help streamline the integration process by providing out-of-the-box support for different protocols, data formats, and standards. How LIKE.TG Enables API-led Connectivity The future of API-led connectivity holds tremendous potential. As businesses continue to embrace digital transformation, API-led connectivity will play a crucial role in enabling seamless integration and data flow. With emerging trends such as event-driven architecture, API marketplaces, and API monetization, businesses are finding innovative ways to leverage APIs for their growth and success. As integration capabilities evolve, API-led connectivity will remain a crucial enabler for businesses seeking to stay ahead in a rapidly changing world. Enter LIKE.TG. LIKE.TG is a no-code platform for developing, testing, deploying, governing, and overseeing APIs, enabling organizations to expedite API creation and management. Crafted with an emphasis on user-friendliness, efficiency, and automation, LIKE.TG API Management reduces API development and management time by up to 80%. Integrating LIKE.TG into your API strategy can enhance your ability to adapt to evolving business requirements, refine dataflows and workflows through API-led connectivity, and ensure a seamless user experience for your customers. Want to experience the benefits of no-code API management? Sign up for LIKE.TG API Management’s14-day free trialor schedule ademowith our experts today!
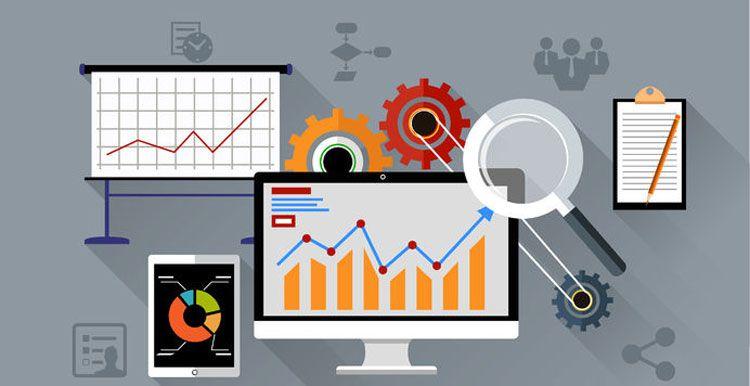
CDC for ETL Process Optimization in Finance Industry
Every day, customers produce an immense volume of data through hundreds of thousands, if not millions, of individual transactions. From managing customer transactions and financial records to dealing with regulatory requirements and risk management, data plays a crucial role in every aspect of banking operations. This data is categorized as big data, a term denoting “large, diverse sets of information that grow at ever-increasing rates.” To put this into perspective, a staggering 2.5 quintillion bytes of data is generated daily. Banks rely on Extract, Transform, Load (ETL) processes to make sense of data and extract valuable insights. These processes are critical for banks to manage and utilize their vast amounts of data effectively. However, as data volumes continue to grow and the need for real-time insights increases, banks are pushed to embrace more agile data management strategies. Change data capture (CDC) emerges as a pivotal solution that enables real-time data synchronization and analysis. Understanding ETL Processes in Banking ETL refers to the three fundamental steps in data integration. First, data is extracted from various sources, including databases, applications, and external systems. This extraction process involves identifying relevant data points and retrieving them in a structured manner. For example, customer transaction data may be extracted from a database using SQL queries, while regulatory data may be obtained from external systems through APIs. Next, the extracted data is transformed into a standardized format and cleansed of any inconsistencies or errors. This transformation phase involves applying various data manipulation techniques, such as data validation, cleansing, and enrichment. For instance, if the extracted data contains missing values or outliers, these issues are addressed during the transformation process to ensure data accuracy. Finally, the transformed data is loaded into a target system or data warehouse for reporting and analysis. The loading phase involves storing the data in a structure that facilitates easy retrieval and analysis. For example, by loading the transformed data into a data warehouse, banks can perform in-depth analysis, generate reports, and gain valuable insights. Key Challenges in Current ETL Processes Growing volume and velocity of data: With the advent of digital banking, mobile payments, and other technological advancements, banks are generating data at an unprecedented rate. This exponential data growth has created significant demands on traditional ETL processes, which struggle to keep up with the speed and scale required for real-time insights. Complexity of data sources and formats: Banks have to deal with structured and unstructured data from various sources, such as transactional databases, log files, social media feeds, and more. Each data source may have its own data format and schema, requiring careful mapping and transformation during the ETL process. Need for near real-time data integration: Traditional ETL processes typically operate on a batch basis, meaning data is extracted, transformed, and loaded in predefined intervals (e.g., daily or weekly). However, in an era where timely insights can make a significant difference, banks are looking for ways to reduce the latency in their ETL processes. Introduction to Change Data Capture (CDC) To address the challenges faced by banks in optimizing their ETL processes, CDC has emerged as a valuable tool. What is Change Data Capture? CDC captures the changes (inserts, updates, deletes) made to data at the source system level as events. These events are then propagated to the target system, where they are applied, to keep the data in sync between the two systems. Imagine a scenario where a customer updates their contact information in the bank’s online portal. Without CDC, the traditional ETL process would extract the entire customer dataset, transform it, and load it into the target system. However, with CDC, only the specific change made by the customer is captured and propagated to the target system. This targeted approach not only saves time and resources but also ensures that the data remains consistent across systems. Furthermore, CDC provides a granular view of the changes made to the data. Each change is captured as an event, which includes information such as the type of operation (insert, update, delete), the affected rows, and the timestamp of the change. This level of detail allows banks to have a comprehensive audit trail of data modifications. How CDC Works in Data Integration? CDC operates by leveraging the transaction logs or change logs present in source systems. By continuously monitoring these logs, CDC technology can identify and capture the changes as they occur. Moreover, CDC allows banks to choose between different synchronization methods, such as one-way replication or bi-directional synchronization, depending on their specific requirements. This flexibility empowers banks to tailor their data integration approach to suit their unique business needs. Enhancing ETL with CDC By incorporating CDC into their ETL processes, banks can enhance their data integration capabilities. Traditional ETL processes can be supplemented with CDC technology to capture and replicate real-time data changes. This enables banks to have a more accurate and up-to-date view of their data, leading to more meaningful insights and better decision-making. When CDC is integrated with ETL, the ETL process can be triggered by the captured data changes, ensuring that the target system is always synchronized with the source systems. This eliminates the need for periodic batch updates and reduces the latency in data integration. Furthermore, CDC can capture not only changed data but also the metadata associated with said changes. This additional information can be valuable for auditing, compliance, and data lineage purposes. Steps to Optimize ETL Processes Using CDC Optimizing ETL processes using CDC requires a systematic approach that considers the unique requirements and challenges of each individual bank. The following steps provide a general framework for banks to implement CDC in their ETL processes: Identifying Opportunities for Optimization The first step in optimizing ETL processes is to assess the current state and identify areas for improvement. Banks should conduct a thorough analysis of their existing ETL workflows, data sources, and integration requirements to pinpoint bottlenecks and inefficiencies. This assessment helps banks identify the specific areas where CDC can bring the most value. For example, banks may find that certain data sources produce a high volume of changes, making them ideal candidates for real-time replication using CDC. By focusing on these high-impact areas, banks can prioritize their optimization efforts and achieve maximum benefits. During this stage, banks should also consider the scalability and performance requirements of their ETL processes. CDC technology can address these challenges by enabling incremental updates instead of full data loads, reducing the overall processing time and resource consumption. Implementing CDC in ETL Processes Once optimization opportunities are identified, banks can proceed with implementing CDC in their ETL processes. This involves deploying CDC technology that is compatible with the bank’s data source and target systems. When selecting a CDC solution, banks should consider factors such as data source support, scalability, ease of integration, and real-time data replication capabilities. It is essential to choose CDC technology that aligns with the bank’s specific requirements and can seamlessly integrate into the existing ETL infrastructure. Additionally, banks need to establish data mapping and transformation rules to ensure that the captured changes are correctly applied to the target system. This step involves defining the mapping between the source and target data structures, handling data type conversions, and resolving any conflicts or inconsistencies. Properly configuring and setting up CDC technology is crucial for seamless data integration and synchronization. Banks should also test the CDC implementation to ensure that it meets the desired performance, reliability, and data quality requirements. Furthermore, banks should consider implementing data validation and reconciliation mechanisms to ensure the integrity and consistency of the replicated data. This involves comparing the source and target data sets to identify and resolve any discrepancies. Automated Tools for ETL and CDC in Banking Operations Adopting automated tools for ETL and CDC processes is instrumental in managing and processing vast volumes of data with precision and agility. These tools offer a sophisticated framework for extracting data from heterogeneous sources, transforming it to meet specific banking requirements, and seamlessly loading it into the target systems. Moreover, CDC capabilities ensure real-time modification tracking within critical datasets, enabling banks to promptly identify changes in transactional records, customer profiles, or compliance standards. By leveraging automated tools for ETL and CDC, banks optimize operational efficiency, streamline data workflows, and maintain a competitive edge in an increasingly data-driven financial landscape. Centerprise can make building CDC-enabled ETL pipelines easy and fast! Powered by a no-code interface, Centerprise makes creating and automating ETL pipelines super simple, with increased time and cost-savings. Give it a try! Book FREE trial Using LIKE.TG to Easily Set Up An ETL Pipeline With CDC LIKE.TG’s user centric approach, code free environment, and intuitive UI allows it to empower business users in their data-driven endeavors. Here’s a breakdown LIKE.TG’s key features for CDC-enabled ETL: Diverse Connectivity: Supports a broad range of connectors for popular databases, data warehouses, and file formats, facilitating seamless integration into the ETL process. Unified CDC Management: Consolidates CDC oversight for relational databases within a single platform, eliminating the need for separate management, and ensuring comprehensive control across all relevant databases. Built-in Transformations: Offers built-in transformation capabilities, empowering users to cleanse and manipulate data effortlessly, enhancing data quality and accuracy within CDC-enabled pipelines. Data Profiling and Quality Assurance: Provides robust data profiling and quality features, facilitating checks to ensure accuracy and reliability, which is especially crucial for real-time or near-real-time data processing scenarios. Automation and Job Scheduling: Accelerates data transfer through automation and scheduling features, optimizing the efficiency of CDC-enabled ETL pipelines. This includes scheduling near real-time job runs. By incorporating scheduling options like ‘Continuous,’ LIKE.TG ensures timely updates and synchronization across data sources. Comprehensive Security Protocols: LIKE.TG prioritizes data security through robust measures such as bearer token authentication, granular user access control, customizable role-based management, and seamless integration with Windows Active Directory. In conclusion, leveraging CDC is a pivotal strategy for banks to streamline and optimize their ETL processes. By capturing and processing only altered data, CDC minimizes redundancy, enhances efficiency, and ensures real-time synchronization across systems. Transform your bank’s data management with LIKE.TG’s powerful CDC-enabled ETL workflows. Secure, streamline, and synchronize data effortlessly. Sign up for a demo or a 14-day- free trial now!
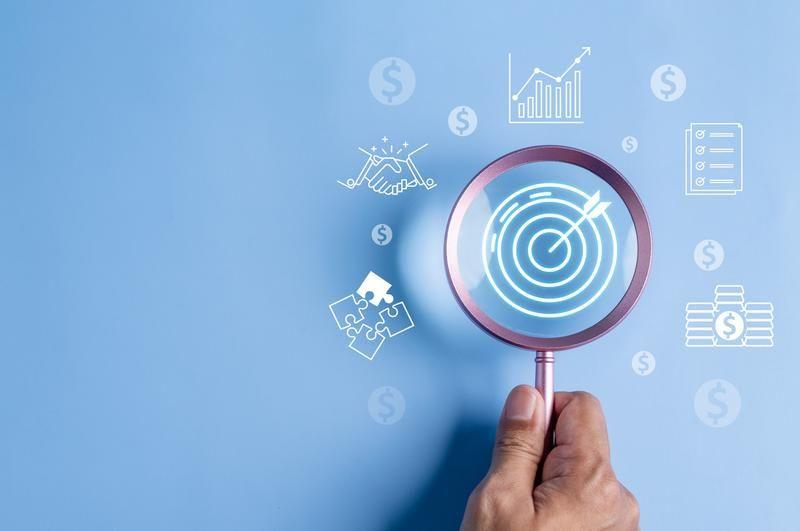
Data Vault 2.0: What You Need to Know
With rising data volumes, dynamic modeling requirements, and the need for improved operational efficiency, enterprises must equip themselves with smart solutions for efficient data management and analysis. This is where Data Vault 2.0 comes into play. It supersedes Data Vault 1.0, the original data modeling methodology specifically designed for data warehousing. With its foundation rooted in scalable hub-and-spoke architecture, Data Vault 1.0 provided a framework for traceable, auditable, and flexible data management in complex business environments. Building upon the strengths of its predecessor, Data Vault 2.0 elevates data warehouse automation by introducing enhanced scalability, agility, and adaptability. It’s designed to efficiently handle and process vast volumes of diverse data, providing a unified and organized view of information. With its ability to adapt to changing data types and offer real-time data processing capabilities, it empowers businesses to make timely, data-driven decisions. This is further substantiated by BARC’s study, which reveals that 45% of leaders have adopted Data Vault 2.0, primarily for its ability to expedite data delivery. What is Data Vault 2.0? Data Vault 2.0 is a modern data modeling methodology designed to provide a solid foundation for managing an organization’s data assets. It serves as a comprehensive framework that supports data integration, storage, and retrieval in a way that is highly adaptable, scalable, and conducive to business agility. This approach is particularly valuable in the era of big data, where organizations need to quickly adapt to changing business needs and incorporate diverse data sources. What’s New in Data Vault 2.0? In 2013, Dan Linstedt and Michael Olschimke introduced Data Vault 2.0 as a response to the evolving data management landscape, taking Data Vault 1.0 to new heights. While maintaining the hub-and-spoke structure of its predecessor, The upgrade introduces new, innovative concepts to enhance its efficiency and adaptability. Three key components of this system are the Business Vault, the Raw Vault, and the Information Mart and Data Mart layers. Raw Vault: In contrast to the Business Vault, the Raw Vault serves as the primary storage for original source data. It preserves the integrity of the data, ensuring that the original, unaltered data is always available for reference or further processing. Business Vault: This component of Data Vault 2.0 is a dedicated repository for business-specific rules and transformations. It optimizes data to meet precise business requirements, ensuring that the data is tailored to the specific needs and context of the business. Information Mart and Data Mart Layers: These layers in Data Vault 2.0 offer advanced analytical and reporting capabilities. They create a seamless connection between raw data and actionable insights, allowing businesses to easily transform their raw data into meaningful information that can drive decision-making. Understanding the Difference: Data Vault 1.0 vs Data Vault 2.0 The primary difference between the two lies in their implementation. Data Vault 2.0 adopts a groundbreaking approach by employing Hash Keys as surrogate keys for hubs, links, and satellites, effectively replacing the conventional sequence numbers. This substantially enhances data performance, scalability, and traceability. Additionally, Data Vault 2.0 introduces the concept of Business Keys, which are unique identifiers or natural keys that represent the core business entities within an organization. Data Vault 2.0 establishes comprehensive standards and guidelines for naming, modeling, loading, and documenting data. This ensures a foundation of quality, clarity, and manageability, making Data Vault 2.0 a comprehensive solution for modern data warehousing. Aspect Data Vault 1.0 Data Vault 2.0 Hash Keys Hash Keys weren’t a central concept, limiting data integrity and traceability. Prioritizes Hash Keys, ensuring data integrity and improving traceability for enhanced data security. Loading Procedures Loading procedures in Data Vault 1.0 could be complex, often involving sequence numbers, impacting efficiency. Simplifies loading procedures, enhancing efficiency and eliminating the need for complex sequence numbers. Dependencies Had significant dependencies, potentially slowing down data loading due to sequential processing. Reduces dependencies, enabling faster data processing through parallelization. Scalability Faced challenges with large datasets due to design limitations. Efficiently handles big data, making it suitable for complex datasets. Agility Less adaptable to changes in data sources and business requirements. Agile and responsive to changes, ideal for dynamic environments. Tech Agnosticism Limited flexibility with technology tools and platforms. More versatile, accommodating various technologies and platforms. The Data Vault 2.0 Advantage The need for Data Vault 2.0 stemmed from the changing dynamics of the data landscape. As organizations began to handle larger volumes of diverse data sources and adopted more agile development practices, it became evident that Data Vault 1.0, while initially effective, had certain limitations. The technology: Struggled to adapt to changing data types. Couldn’t handle vast volumes of data. Lacked real-time data processing capabilities. Didn’t align well with current technology or data governance requirements. 2.0 was developed to address the issues with the original Data Vault methodology and to better keep up with modern data management and analytics requirements. Here are some key reasons why Data Vault 2.0 was needed: Evolving Data Ecosystems: Data Vault 1.0 was developed in the early 2000s when data ecosystems were simpler and less complex compared to today. With the advent of big data, cloud computing, and advanced analytics, data architectures have become more intricate. Data Vault 2.0 was needed to better fit into these modern, complex data environments. Integration with Modern Technologies: Data Vault 1.0 was primarily designed for relational databases. Data Vault 2.0 extends its capabilities to work with big data platforms and NoSQL databases, allowing organizations to integrate a wider range of data sources. Managing Rising Data Volumes: Data Vault 2.0 offers better scalability and flexibility for handling large and diverse data volumes from various sources seamlessly. It leverages technologies like distributed computing and parallel processing to ensure efficiency. Industry Standards: Over time, industry standards and best practices for data management have evolved. Data Vault 2.0 incorporates these updated standards and aligns more closely with data governance and compliance requirements. Business-Centric Focus: Data Vault 2.0 focuses on making sure that data modeling and warehousing are closely aligned with what a company wants to achieve by introducing the concept of Business Data Vault. This helps IT and business teams work together better and ensures that data solutions really meet what the company needs. Benefits of Data Vault 2.0 Data Vault 2.0 offers three key advantages: scalability, adaptability, and auditability. Let’s explore these benefits in detail: Scalability Scalability is vital because data volumes are continually expanding, and organizations need systems that can handle this ever-growing demand. For example, consider a retail company that experiences a surge in online sales during the holiday season. Without a scalable data management system, the company may struggle to process and analyze the increased volume of transactional data efficiently. Data Vault 2.0 addresses scalability through various means: Separation of Concerns: It divides data into three layers (Raw Data, Business Data Vault, and Information Delivery Layer), allowing each layer to be scaled independently. This ensures resources can be allocated as needed, optimizing performance during high-demand periods like the holiday season. Parallelization: It encourages parallelization of data loading and processing tasks, distributing workloads across multiple servers or resources. This enhances efficiency, ensuring substantial datasets, like a surge in holiday sales, can be processed efficiently. Adaptibility Adapaptability is critical because business requirements, data sources, and data structures are subject to change. For instance, a healthcare organization may need to incorporate new types of patient data or adapt to evolving regulations. Data Vault 2.0 offers adaptability through several methods: Flexibility: Its inherent design is flexible and agile due to its modular structure of Hubs, Links, and Satellites. This allows for easy integration of new data sources, such as new patient data, without disrupting the existing structure. Future-Proofing: Its architecture is not tied to specific business rules or data sources, allowing the data architecture to evolve with changing business requirements or regulations, ensuring the healthcare organization remains compliant. Auditability Auditability is crucial for ensuring transparency, accountability, and compliance with data governance standards. Without it, organizations may struggle to track changes to data or maintain data quality. For example, in the financial sector, it is vital to track every change made to financial transaction data for regulatory compliance. Data Vault 2.0 enhances auditability through several ways: Traceability: It incorporates tracking mechanisms that capture metadata related to data sources, transformations, and data lineage. This detailed documentation ensures that every change made to financial transaction data can be traced back to its origin. Versioning: It allows for data versioning, enabling financial organizations to maintain historical records of data changes over time, which is invaluable for auditing and regulatory compliance purposes. Data Vault 2.0: A Glimpse Forward As we look towards the future, the question arises: Will Data Vault 2.0 continue to stay relevant in the face of technological advancements and the increasing volume and complexity of data? The answer is yes. Data Vault 2.0 is designed to handle the challenges of big data, integrating data from diverse sources, including social media, IoT devices, and traditional databases. Its ability to manage vast volumes of data, maintain data history, and ensure data consistency makes it ideal for large-scale businesses seeking to maintain long-term historical storage of data. To further highlight the relevance of Data Vault 2.0 in the future, let’s discuss some emerging trends in the data sphere and how this approach facilitates them: Machine Learning and Artificial Intelligence (AI): The integration of ML and AI in data management is revolutionizing the way businesses process and analyze data. Data Vault 2.0’s ability to handle high data volumes and support parallel processing ensures that data scientists and AI practitioners have access to clean, comprehensive data sets to train and refine their models. Its focus on data lineage and traceability aligns with the need for transparency and accountability in AI, especially in industries like healthcare and finance. Rise of Hybrid and Multi-Cloud Infrastructures: With businesses increasingly adopting hybrid and multi-cloud strategies, Data Vault 2.0’s compatibility with various cloud platforms and on-premises systems is a significant advantage. Its modular design allows seamless integration with diverse cloud platforms, facilitating efficient and secure data storage and processing. Self-Service Analytics: The trend toward self-service analytics is set to grow, empowering business users to explore and analyze data independently. Data Vault’s structured architecture provides a foundation for self-service analytics, allowing non-technical users to confidently navigate and query data. By fostering a data-driven culture and reducing reliance on IT for data provisioning, Data Vault accelerates decision-making and innovation within organizations. Data Vault 2.0: Utilizing Advanced Tools and Automation The implementation and maintenance of a Data Vault is an elaborate process, requiring a high level of expertise and significant time investment. However, employing advanced data warehouse tools tailored for Data Vault 2.0 can greatly simplify these processes. These tools offer the advantage of automation at every stage — from design and modeling to deployment and maintenance, significantly enhancing efficiency. Let’s delve into how these tools transform each stage of the Data Vault 2.0 process, making it more accessible and manageable for businesses. Design Stage At the design stage, advanced tools assist in creating the blueprint of the data vault. They enable the easy mapping of business concepts to data vault structures, leading to a well-structured and efficient design. The result is a time-efficient process that minimizes potential errors, ensuring a more accurate and effective design. Modeling Stage During the modeling stage, these tools automate the generation of data vault models based on the initial design. This includes the creation of hubs, links, and satellites, which are the core components of any data vault. The automation process significantly accelerates the modeling stage and guarantees consistency and accuracy in the models. Deployment Stage In the deployment stage, automated solutions streamline the process of populating the data vault with data from various sources. They automate the extraction, transformation, and loading (ETL) processes, ensuring a smooth and efficient data flow into the data vault. This accelerates the deployment process and mitigates the risk of data errors. Maintenance Stage During the maintenance stage, these solutions continue to provide value by automating routine tasks and checks. This ensures the data vault remains accurate, up-to-date, and efficient over time, reducing the manual effort required for maintenance and enhancing the overall longevity and reliability of the data vault Final Words Data Vault 2.0 emerges as a highly effective system for building agile and auditable data warehouses. Its unique data modeling methodology offers a holistic approach to designing, developing, and deploying data warehouses. As the demand for agile and auditable data warehouses grows, the role of specialized tools and automation in facilitating the effective implementation of Data Vault 2.0 becomes increasingly significant. This is where LIKE.TG comes into play. LIKE.TG is an enterprise-grade, end-to-end data management solution that enables enterprises to simplify the process of creating a Data Vault. With features that align perfectly with the principles of Data Vault 2.0, LIKE.TG provides businesses with a robust, scalable, and flexible data warehousing solution. It’s designed with user-friendliness and efficiency in mind, enabling businesses to automate the process of creating a Data Vault with just a few clicks. Elevate your data warehousing capabilities with LIKE.TG. Download our 14-day free trial today and see how easy it is to build a Data Vault.
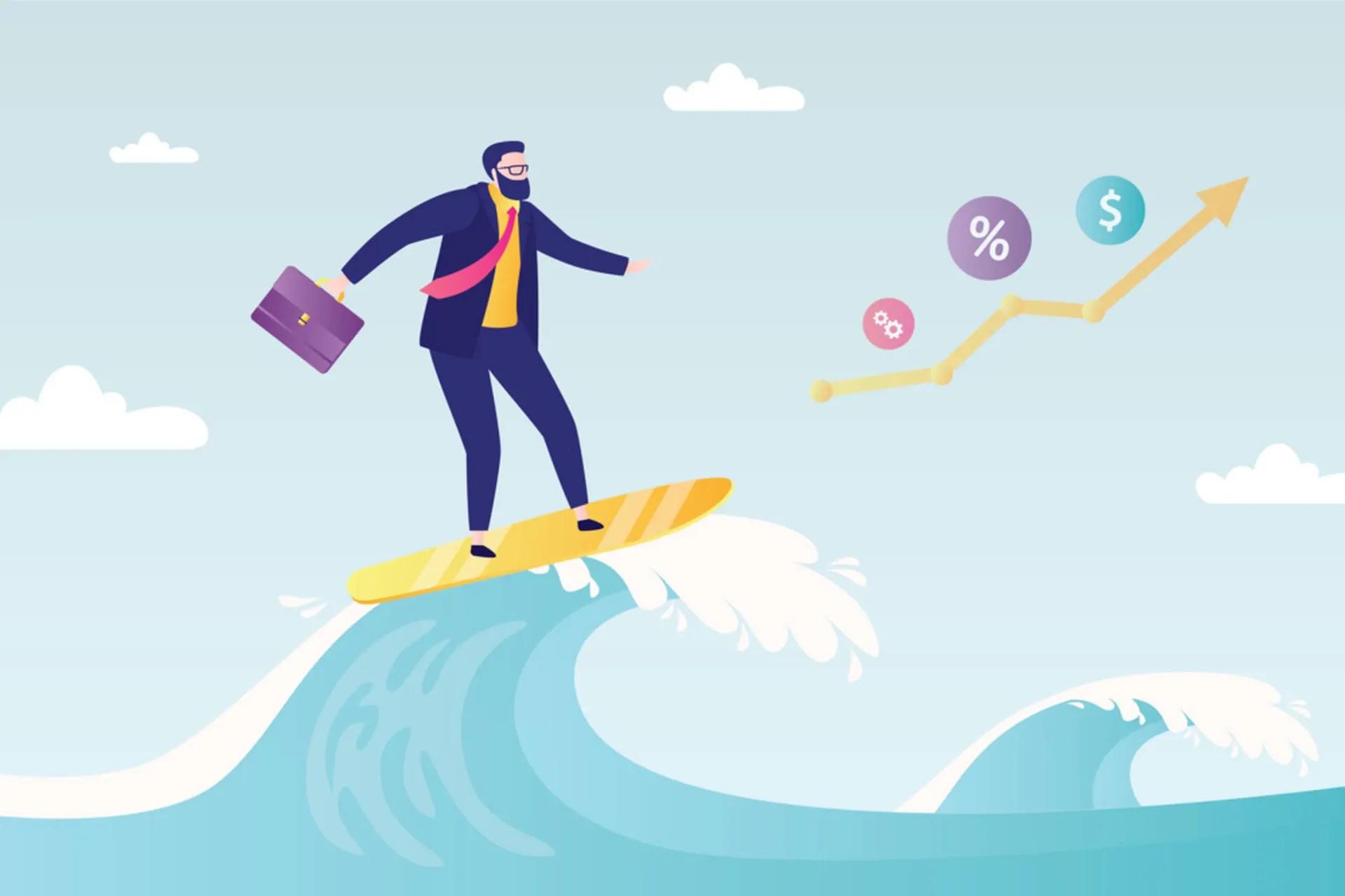
Fivetran vs. Matillion for ETL: A Detailed Comparison
As data becomes more complex and spreads across various sources and touchpoints, it is becoming increasingly difficult to combine it at the speed required by the business. Data teams usually use ETL tools to integrate data into a unified view. Thankfully, these ETL tools are also evolving along with the data needs. Today, there are a plethora of options available in the market, that choosing the right one can be a little tricky. Two established ETL tools in the market are Fivetran and Matillion. This blog will help users evaluate their pricing, features, integrations, support, and feasibility. Also, it will give you another alternative to both the solutions that you might find helpful. What is Fivetran? Fivetran is that was launched in 2012 out of Y Combinator. It took its inspiration from Fortran, a coding language developed in 1954 by John Backus. Fivetran operates as a single platform, offering data movement, transformation, and governance features. It’s fully managed on the cloud and allows users to set up and manage their data pipelines easily. The tool comes with a range of pre-built connectors to streamline data integration and operates on a usage-based pricing model. Customers pay monthly for each MAR (monthly active row) in use. What is Matillion? Matillion is also a cloud ETL tool. It was founded in 2011 in Manchester, UK. Matillion focuses on simplifying data integration and business intelligence. Matillion’s ETL offering focuses on robust transformations and connectivity. Not only do users have access to pre-built connectors, but they can also access community connectors and create their own. A distinguishing feature of Matillion is that it can run on major cloud platforms such as Amazon, Google Cloud Provider, and Microsoft Azure. It uses a credit model with three tiers: basic, advanced, and enterprise. Fivetran Vs. Matillion: Pricing Fivetran Fivetran charges users based on active rows, where each active row is counted once a month. There are 4 tiers: Free Starter Standard Enterprise It offers a free tier for low data volumes, which provides limited features for 500,000 MARs. Higher tiers allow unlimited users and API access, with users getting priority support, enterprise connectors, and advanced security in the Enterprise. Matillion Unlike Fivetran, Matillion uses progressive per-credit pricing with 3 tiers: Basic ($2/credit) Advanced ($2.5/credit) Enterprise ($2.7/credit) The credits consumed are dependent on vCore usage per hour. For every 1 hour of vCore use, 1 credit is used. The basic tier offers limited connectors, users, and security. Enterprise customers receive CDC pipelines, unlimited users, mission-critical support, auto-documentation, and more. No-code, End-to-end ETL/ELT With LIKE.TG Maximize your data's potential with LIKE.TG's no-code solution. Extract, prepare, transform, and load your data with ease with a flexible pricing structure - tailored to your specific needs. Download Free 14 Day Trial Fivetran Vs. Matillion: Services Fivetran Fivetran is purely an ELT product. For extraction, Fivetran has an extensive library of ready-made connectors. Users can find connectors based on connector type and features. In terms of replication, Fivetran supports SaaS, streaming, file, database, and SAP. It uses dbt Core for custom transformations alongside its native library. Major data warehouses, such as Redshift and Snowflake, support these models. To streamline efficiency, Fivetran automatically switches between full and incremental loads. It helps users automate the entire ETL process. It also provides auditing, schema drift handling, and incident handling. Matillion Matillion boasts a wide range of connectors to cloud sources and destinations. While the number of pre-built connectors is limited compared to Fivetran, the availability of community connectors and quick custom ones makes up for it. Users can build no/low-code pipelines based on experience and resources. The company supports various data integration initiatives: ETL/ELT Reverse ETL Data replication CDC Verdict: Both tools have robust ETL features. While Fivetran has more pre-built connectors, Matillion has community connectors, offering more flexibility in making custom options. Both tools provide a minimum code approach to building data pipelines. Fivetran Vs. Matillion: Support Fivetran Fivetran provides the usual SaaS support features: FAQs and support tickets. They also have a status page to provide a real-time service functionality check. Fivetran has a support rating of 7.9/10 on G2 and 4.4/5 on Gartner. Matillion Matillion has a dedicated support subdomain for submitting tickets. The subdomain also comes with example cases and a knowledge base. Users can also join a community for more information and tips. Matillion has a support rating of 8.8/10 on G2 and 4.3/10 on Gartner. LIKE.TG provides industry-leading support with a robust ETL and ELT platform. Try it for 14 days – totally free. Fivetran Vs. Matillion: Ease of Use Fivetran Fivetran has a user-friendly, drag-and-drop web interface that makes it easy to operate. It uses a no-code approach to ELT. The large number of connectors and cloud deployment make it easy to load and manage data. It also has role-based access, versioning, and other collaboration features. It has an 8.9/10 ease of use rating on G2. Matillion Matillion offers both no-code and low-code development. This makes it accessible to both developers and business users. Like other ETL tools, it also has a drag-and-drop GUI for creating mappings and pipelines. Mattilion also provides Git integration, version control, and role-based access for improved collaboration. Their strong documentation further helps teams use the product more effectively. It has a 9.3/10 ease of use rating on G2. Fivetran Vs. Matillion: Architecture Fivetran Hosted on AWS and Google Cloud, Fivetran is a fully managed SaaS focused on efficient Extract-Load processes. It fetches data from the user’s local environment, manages it on its cloud, and then pushes it to the customer’s cloud – keeping the 3 separate. Other core features of its design are: Incremental loading Idempotence Schema drift handling Matillion Matillion’s supports 3 architecture types: Single node: All components of Matillion run on a single VM. Single node RDS: All nodes are on a single virtual machine, but the database runs on a separate Redshift instance. Clustered: Multiple VMs are used for the nodes. Data is never pushed out of the cloud to an external server, keeping data sensitivity in mind. The focus is on carrying out transformations through the power of the cloud warehouse. Users can access the ETL client on Amazon EC2, Google Cloud, and Microsoft Azure. Fivetran Vs. Matillion: Deployment Fivetran While Fivetran mainly works fully managed on the cloud, it also offers self-deployment options. It can be deployed on-premises – users can host it on a VM or air-gapped. Hybrid deployment is also an option. This setup provides users with added flexibility. Small teams can choose the cloud option, while large corporations with strict security requirements can choose self-hosting. Matillion Users can deploy Matillion in 3 ways through the web interface: Using Environments (a target data warehouse) Using Multiple Projects (each project has one Environment) Using Multiple Instances (each Matillion VM is one Instance) There are also other deployment options through their REST API. Comparison Summary Fivetran Matillion Pricing Based on Monthly Active Rows – has 4 tiers Based on credits consumed – has 3 tiers Free Trial Yes – 14-day Yes Deployment On-cloud on-prem On-cloud on-prem, multiple instance Customer Support Support tickets, email, live status page, FAQs, documentation Subdomain for tickets, email, user community, knowledge base, Matillion Academy Customer Support Ratings 7.9/10 G2, 4.4/5 Gartner 8.8/10 G2, 4.3 Gartner Connectors 459, can ask for custom connectors 100+ pre-built, community versions and custom-building options are also available Custom API SQL coding required No SQL coding Interface No-code, drag-and-drop Low-code/no-code, drag-amd-drop Ease of Use Rating 8.9/10 G2 9.3/10 G2 Transformations Pre-built, custom transformations through dbt Core Pre-built, custom through SQL ide Connectivity All major cloud warehouses All major warehouses LIKE.TG – A Better Way to ETL LIKE.TG provides a simple yet powerful way to manage your data. It offers a no-code solution for creating enterprise-level ETL and ELT pipelines in a drag-and-drop interface. In short, it empowers both technical and non-technical teams to automate data management. LIKE.TG provides: A drag-and-drop interface for building ETL/ELT pipelines. Connectors for all major databases. OData connectivity. Automation. No-code, in-built transformations. Data quality rules. Change Data Capture. LIKE.TG is built-on industrial strength engine and can easily process large volumes of data. It has a Top Rated certification on Trustradius and multiple Fortune 500 clients. LIKE.TG is also an industry leader in User Support, boasting customer support excellence awards from leading publications like TrustRadius and G2. In terms of pricing, LIKE.TG has a flexible approach – users can choose and pay for the exact modules they need. So, no overpaying for features that are not required. Try out LIKE.TG for free with a 14-day trial, or get in touch with us for a free demo!