筛选
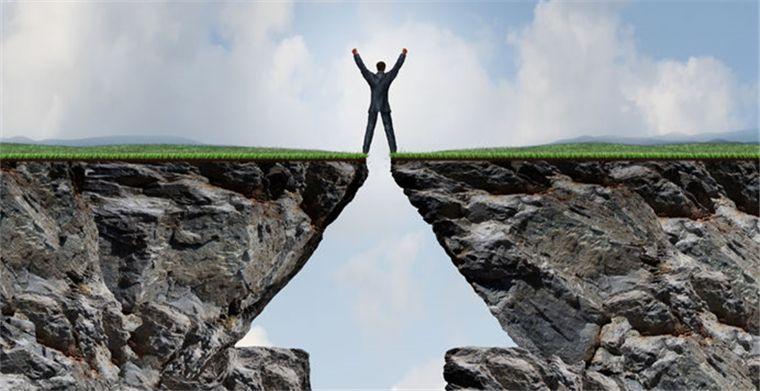
Data Warehousing for Insurance Reporting and Analytics
The significance of data warehousing for insurance cannot be overstated. It forms the bedrock of modern insurance operations, facilitating data-driven insights and streamlined processes to better serve policyholders. In recent years, data warehouses have emerged as foundational tools that allow insurance companies to efficiently manage, analyze, and leverage the immense volume of data at their disposal for seamless reporting and analytics. These repositories play a crucial role in transforming raw data from disparate sources into actionable insights, steering insurance companies toward informed decision-making. The data warehouse has the highest adoption of data solutions, used by 54% of organizations. (Flexera 2021) Data Warehousing for Insurance: Creating a Single Source of Truth Insurance companies generate and receive large amounts of data from various business functions and subsidiaries that are stored in disparate systems and in a variety of formats. Centralizing and organizing data from disparate sources, such as customer information, policies, claims, and market trends, provides a unified platform for comprehensive analysis. This analytical capacity enables insurance professionals to conduct intricate risk assessments, predictive modeling, and accurate trend analyses, facilitating the formulation of effective strategies. That’s where a data warehouse comes in! A data warehouse enables them to integrate this disparate data into a single source of truth, persist it in a report-oriented data structure, and create a centralized gateway to enable seamless reporting and analytics at the enterprise scale. The benefits of data warehousing for insurance companies are many, including: Centralized Data: A data warehouse allows the consolidation of data from diverse sources spread across different systems. This centralized repository ensures consistent, accurate, and up-to-date information is available for analysis. Efficient Reporting: Standardized data in a data warehouse simplifies the reporting process. The company can generate consistent and accurate reports for regulatory compliance, financial audits, and performance evaluation. Enhanced Analytics: A data warehouse facilitates advanced analytics, including predictive modeling, risk assessment, and customer behavior analysis. This enables the company to identify opportunities, optimize processes, and mitigate risks effectively. Cross-subsidiary Insights: With a data warehouse, the insurance company can gain insights that cut across subsidiaries. This can highlight cross-selling opportunities, identify areas of operational synergy, and improve customer experiences. Deeper Customer Awareness: Using a data warehouse, an insurance company can learn more about its customers. They can pinpoint customer preferences, behaviors, and requirements, thereby enabling precise marketing and customer service strategies. Improved Decision-Making: Access to a comprehensive dataset enables better decision-making. Executives can analyze trends, performance, and risk factors across the entire organization, leading to more informed strategic choices. Build your data warehouse in minutes Discover how! In addition, data warehousing helps improve other data management aspects, including: Data Security: Centralizing data in a data warehouse enables the implementation of robust security measures, ensuring that sensitive information is appropriately protected. Data Integration: A data warehouse supports data integration across various subsidiaries, systems, and data formats, fostering interoperability and reducing data silos. Data Quality and Consistency: A well-maintained data warehouse enforces data quality standards, ensuring that data is accurate, complete, and consistent. Data Warehousing for Insurance: Who Can Benefit? Data Team Leaders and Senior Personnel As heads of the data team or senior members of an insurance organization, these individuals play a critical role in shaping data strategies. Utilizing a data warehouse empowers them to streamline reporting and analytics processes. By centralizing data, incorporating data quality management, and providing efficient querying capabilities, they can make more informed decisions and drive the company’s overall data-driven strategy. This leads to improved operational efficiency and a competitive edge in the insurance industry. Data Analysts and Engineers Data analysts and engineers within the organization benefit significantly from a data warehouse. They often find themselves spending a substantial amount of time on mundane, repetitive tasks like data extraction, transformation, and loading (ETL). With a data warehouse in place, these tasks can be automated, allowing them to focus on higher-value activities such as data analysis, modeling, and innovation. This not only boosts their job satisfaction but also empowers them to contribute more effectively to building innovative insurance products and solutions that can drive business growth. Business Users Business users in the insurance industry face challenges related to data dependency, often experiencing delays in obtaining critical information. They rely on timely insights to make informed decisions and solve problems swiftly. A data warehouse addresses this by providing self-service reporting and analytics capabilities. Business users can generate reports instantly, reducing their dependence on IT or data teams. This agility accelerates their ability to respond to changing market conditions, customer needs, and emerging opportunities, ultimately enhancing the organization’s agility and competitiveness. Fraud Detection Prevention Using Data Warehouse Utilizing a data warehouse, insurance companies can improve their fraud detection process. A consolidated data repository enables them to employ anomaly detection and process integrity checks. Through continuous analysis of policyholder data and transaction records, the system establishes behavioral baselines, promptly flagging deviations for potential fraud. This centralized approach enables correlations across diverse data sources, unveiling hidden patterns indicative of fraudulent activities. A data warehouse’s capability to consolidate information empowers insurers to minimize financial losses caused by fraud. Monitoring various operational aspects allows insurers to gain a comprehensive overview, facilitating rapid identification of irregularities and potential fraud indicators. Real-time transaction monitoring aids in halting fraudulent payouts, while predictive models, built on historical patterns, enable proactive risk mitigation. Free Whitepaper - The Blueprint for End-to-End Data Warehouse Automation Download Data Warehousing for Insurance: A Smart, Long-term Financial Decision An enterprise-grade data warehouse with end-to-end automation offers a great return on investment (ROI) to insurance companies by improving operational efficiency, introducing cost-saving opportunities, and enabling faster business intelligence. The ROI depends on the business goals and size of each organization, but in most cases, companies recover their cost of investment within the first three years. Data warehousing for insurance require a considerable allocation of organizational resources, which sparks significant interest in both their initial justification and ongoing evaluation. It’s essential to acknowledge that despite this commitment, data warehouses frequently demonstrate themselves to be exceptionally valuable and rewarding investments. Data Warehousing for Insurance: Try LIKE.TG DW Builder! LIKE.TG DW Builder is our flexible and scalable data warehouse automation tool that allows you to design, develop, and deploy high-volume data warehouses for your insurance business in days. Built upon LIKE.TG’s industrial-strength ETL engine and refined by years of use by Fortune 1000 companies, our solution allows you to lead with speed through its robust automation capabilities. LIKE.TG DW Builder accelerates design, development, and implementation phases by automating low-level, repetitive tasks, allowing the business to refocus resources on optimizing data processes and generating winning results. Go from Source to Insights at Unprecedented Speeds Combining the power of advanced data modeling features and parallel processing ETL/ELT engine with enhanced automation capabilities, LIKE.TG DW Builder streamlines data warehousing for insurance companies, allowing them to speed up time-to-information and reduce dependency on IT, ensuring that analysts and underwriters have the right data at the right time to facilitate better decision-making. An All-Round Platform for Data Warehouse Development LIKE.TG DW Builders offers a comprehensive set of data warehousing features tailored to insurance companies’ data management requirements. It simplifies the process of bringing together data from on-premises and cloud sources, ensuring a unified and high-quality data foundation for improved reporting and analytics. Insurance companies can benefit from LIKE.TG’s capabilities to manage historical data effortlessly and connect to a wide array of sources and destinations. The push-down optimization (ELT) feature enhances query performance, enabling them to focus on value-added tasks like data analysis and modeling. LIKE.TG also caters to the needs of insurance companies by providing instant access to data through self-service reporting and analytics dashboards. This reduces dependency and empowers them to make agile, data-driven decisions. With the ability to scale via cloud deployment, LIKE.TG ensures seamless growth and scalability as insurance organizations expand. Additionally, LIKE.TG’s data lineage capabilities offer transparency and confidence in data management processes, while secure data movement from on-premises to the cloud ensures data security and compliance. In summary, LIKE.TG equips insurance professionals with the tools they need to harness the full potential of their data for informed decision-making and competitive advantage. LIKE.TG Advantage LIKE.TG’s enterprise-grade data warehousing solution eliminates the need for extensive coding and complex infrastructure, reducing upfront and ongoing costs associated with traditional data warehousing development projects. You don’t need a team of certified data architects and modelers to manage your data warehouse. Moreover, our solution is built on a no-code architecture, enabling faster project completion. Designing and maintaining a sustainable data warehouse for timely reporting and insights requires fewer (and lower) man hours with LIKE.TG. Projects that would typically take several months can be completed within a few weeks or even days through our tool’s intuitive, drag-and-drop environment and advanced data modeling and ETL/ELT capabilities. With over 40 pre-built transformations, the LIKE.TG ETL solution offers increased uptime and greater reliability as compared to custom-coded solutions, which rely on certified data architects and engineers for maintenance. Additionally, LIKE.TG’s unified solution saves resources by eliminating the need for separate licenses, maintenance, and support for multiple tools and vendors, resulting in cost savings and improved resource allocation. As a result, data warehousing with LIKE.TG offers a substantially lower total cost of ownership (TCO). "Building a data warehouse is no longer exclusively for IT coders and coders." Take the lead now! By partnering with LIKE.TG, you can build a data warehouse that would serve your current and future data management needs. To get started, reach us at [emailprotected]or request afree trialtoday.
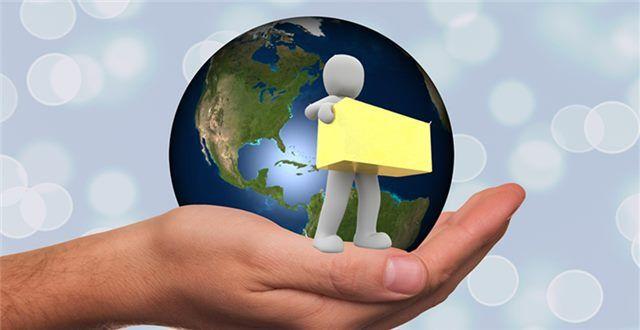
The EDI Implementation Handbook: Best Practices
Electronic Data Interchange (EDI) facilitates standardized data exchange and drives efficiency, accuracy, and speed. The COVID-19 pandemic and the subsequent surge in online business activities have caused an uptick in the use of EDI to maintain seamless continued operation for businesses. Consequently, the global EDI software market is projected to grow at a Compound Annual Growth Rate (CAGR) of 12.5%. However, merely adopting EDI is not enough; successful EDI implementation is key. To maximize the benefits of EDI, businesses must adhere to a series of best practices during their EDI implementation process.” Free E-book - The Essential Guide To Streamlining EDI Exchange Simplify EDI Exchange Now! EDI Implementation: 7 Best Practices 1. Analyze and Understand the Current Process Before implementing EDI, take time to analyze existing business processes. Understanding how the business currently handles transactions, such as purchase orders, invoices, and shipping notices, will identify areas that can be optimized and streamlined with EDI. This will allow users to communicate their needs to potential EDI solution providers and ensure the solution they select is tailored to their specific needs. 2. Choose the Right EDI Solution for A Business Choosing an EDI solution should be a strategic choice tailored to unique business requirements. These are some of the factors to consider: Analyze the volume and types of transactions processed. If a business is handling a high volume of transactions or dealing with complex, industry-specific documents, they’ll need an EDI solution that is capable of dealing with a variety of transactions. Consider the number of trading partners. If a company has a large and diverse set of partners, each with their own specific EDI standards and protocols, they will need a solution that offers flexibility in terms of EDI formats and communication protocols. Assess budget. Implementing EDI is an investment, and companies need to ensure they’re getting a solution that offers a good return on investment. Consider not only the upfront cost but also the long-term maintenance and support costs. Overall, it’s important to take into account the flexibility of the solution, the level of customer support provided by the vendor, and the solution’s scalability as the business grows. Requesting a demo or a trial period can also provide first-hand experience of the solution before making a commitment. 3. Assemble a Competent and Dedicated Project Team To implement EDI effectively, companies need a dedicated project team that has the necessary technical and operational expertise to ensure a smooth transition. This includes IT professionals who understand the technical aspects of the EDI system and are equipped to manage its implementation and handle troubleshooting issues. Operations staff can guide the configuration of the EDI system to align with the organization’s workflow. Finally, financial personnel can ensure their EDI investment is sound, oversee budgetary restrictions, and maximize potential cost savings. With a diverse team, companies can take a holistic approach to EDI implementation, which takes into account all aspects of business. 4. Prioritize Training and Support After the EDI implementation is complete, it is important to ensure that everyone who will be interacting with the new system has a thorough understanding of how it works and the changes it will bring to their workflow. This training should include not only the technical aspects of how to use the software but also a broader perspective of the operational shifts that will come with EDI integration. Moreover, training should be tailored to the specific needs of each role. For example, IT staff will need detailed technical training, while operations staff may need more process-oriented instruction. Consider creating easy-to-follow user manuals or guides as quick reference materials. It’s also essential to have technical support readily available to handle any issues that arise once the system goes live. The availability of support not only reduces downtime but also increases user confidence in the new system. 5. Collaborate with Experienced EDI Solution Providers An experienced EDI solution provider can be a valuable resource during the EDI implementation journey. Their expertise and insights from previous implementations can guide companies toward best practices and help them avoid common pitfalls. These providers can offer tailored solutions that cater to specific business needs, enhancing the compatibility and efficiency of the EDI system. In addition, they can provide ongoing technical support, assisting with upgrades, troubleshooting, and ensuring system optimization. This ensures the smooth operation of an EDI system long after the initial implementation, allowing users to focus more on core business activities. 6. Perform Thorough Testing Before Going Live Testing the EDI system before it is fully deployed can preemptively address a host of potential issues. It’s important to test if the data is being accurately and securely transmitted and if the system is integrating seamlessly with the existing IT infrastructure. Additionally, running simulations of typical transactions will ensure that the system is correctly translating, transmitting, and acknowledging EDI documents. Finally, conducting tests with trading partners goes a long way. This ‘pilot testing’ phase will ensure that both systems can communicate effectively with each other and any discrepancies in EDI standards or communication protocols are identified and resolved. 7. Monitor and Review the EDI System Performance Regularly Once the EDI system is live, it’s crucial to make sure it’s delivering the efficiency gains and expected cost savings. Develop key performance indicators (KPIs), such as reduced order processing times, lower error rates, or improved invoice accuracy, which can be regularly tracked. Collect feedback from system users and trading partners and use this feedback to further refine and optimize the EDI system. Remember, EDI implementation is not a one-off project but a continuous process of improvement and adaptation to evolving business needs. Conclusion Implementing EDI offers tangible benefits beyond data exchange for businesses. It streamlines operations, enhances decision-making with real-time data, fosters stronger partner relationships, and ensures robust security. With careful planning, the right team, and a commitment to continuous improvement, businesses can seamlessly integrate EDI into their operations and reap its immense benefits. LIKE.TG Software is here to support businesses on their digital transformation journey. From choosing the perfect EDI solution to providing ongoing post-implementation support, LIKE.TG is ready to help every business streamline its processes and unlock its data’s potential. Contact us today, and let's drive your business forward together. Contact!
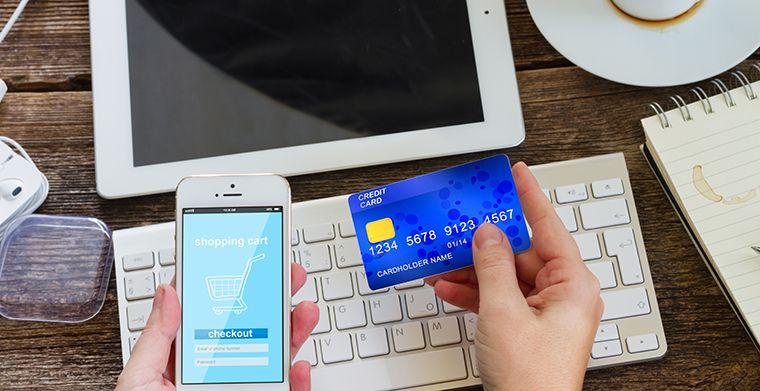
Data Merging Essentials: Process, Benefits and Use-Cases
Did you know that marketing professionals alone use an average of 15 different data sources to collect customer information? While this may seem surprising, the predictions show that this number will increase to 18 this year, and that’s not even looking at other departments like customer service, sales, accounting, and finance. The diverse applications used by different functions in an organization to gather information also make it difficult to review each source for accurate insights. These various tools tend to collect similar information, resulting in duplicates. Data merging is the solution to counter duplication issues, empowering organizations to access complete, accurate, and consistent data. What is Data Merging? Data merging is the process of combining two or more data sets into a single, unified database. It involves adding new details to existing data, appending cases, and removing any duplicate or incorrect information to ensure that the data at hand is comprehensive, complete, and accurate. However, different organizational departments collect similar information using different tools and techniques. Consider a company analyzing customer data: The marketing team uses surveys to gain insights regarding customer preferences, pain points, and opinions. The sales team uses customer relationship management (CRM) systems to gauge information such as past purchases, customer satisfaction, and preferences. The customer support team uses helpdesk software to create tickets and keep a detailed record of customer interactions, ensuring that customer concerns are promptly addressed. Since these teams collect customer information with their specific objectives in mind, the data collected is often similar and needs to be integrated to avoid silos. Data stored separately includes several problems like: Scattered information makes it difficult for analysts to parse various data sets to interpret the data correctly and make the right decisions. Data may be inconsistent, inaccurate, or incomplete. Duplicate data can lead to wasted resources. Combining disparate data into a centralized dataset will allow the company to generate a comprehensive customer profile to run tailored campaigns and create content that resonates with the target audience. In response, data merging unifies the data sets and creates a single source of truth, offering benefits like: Resource Efficiency: By providing access to information in a consolidated framework, data merging expedites information retrieval, eliminates manual, repetitive processes, and enhances search capabilities. This centralization ensures that resources are allocated to strategic, value-adding tasks. Convenience: By combining multiple data sets into one, users no longer have to piece together information from several sources. The convenience of having relevant data in one place makes it easier to analyze the data and extract relevant insights. Improved Decision-Making: Data merging ensures that the information available is complete, accurate, and consistent, presenting a holistic and comprehensive view of what is happening within the organization—facilitating informed, data-driven decision-making. When is Data Merging Needed? Data merging is a technique that allows organizations to analyze data stored in diverse locations, spreadsheets, or databases. This approach is crucial in multiple scenarios. Let’s explore the key ones below: Digital Transformation Organizations embracing digitization must realize the importance of combining data sets. By leveraging digital technologies, data stored in disparate files such as Excel, CSV, and SQL can be consolidated into a unified and structured format and stored in a centralized data processing and hosting system. Business Intelligence Access to the right information at the right time is essential for data-driven decision-making. In today’s competitive landscape, businesses must ensure optimal resource utilization. According to Starmind, 50% of employees reported that spending long hours searching for data points hinders productivity and overall performance. Therefore, data residing in different applications (CRM, web analytics, social media insights) should be combined to gain actionable insights. Mergers and Acquisitions (MA) When a company takes over or merges with another company, it must consolidate resources to operate as a single unit or organization. Data is a vital asset that must be combined and stored in a single repository for a complete picture of the merged entity’s operations. MA scenarios introduce new aspects such as customer profiles, demographics, supplier relationships, employee data, and more that encompass almost all facets of an organization. Therefore, data merging is crucial to ensure frictionless integration and enhance operational efficiency. Stages of Data Merging: A Step-by-Step Process 1. Pre-Merging Profiling Before merging the data, it is critical to know the current state of an organization’s data sources and the type of data they are working with. This comprises attribute analysis, which helps an organization understand how the merged data will scale, which characteristics the data will be joined on, and what additional information may have to be appended. This step also analyzes the data values of each attribute concerning uniqueness, distribution, and completeness. By profiling the data, organizations can identify the potential outcomes of the merged data and prevent any errors by highlighting invalid values. Transformation Next, it is vital to transform the data (cleanse, standardize, and validate) into a usable format. This is done by replacing missing/null values, rectifying incorrect ones, converting data sets into a common format, parsing long data fields into small components, and defining conditions for data integration. By harmonizing the data formats, an enterprise ensures compliance with legal rules and regulations, data accuracy, and consistency across various touchpoints. Filtering Data is often filtered when a subset of the data rather than the complete data set needs to be merged. In this scenario, the data can be segmented horizontally (data from a specific time frame is required or only a subset of rows meet the criteria defined for merging) or vertically (data consists of attributes containing unimportant information). By filtering the data, the information is refined, and only relevant and accurate information is incorporated, enhancing the overall quality of the merged data set. Deduplication It is essential to ensure that the data sets have unique records. Duplicate information is a significant concern with data merging since often similar information is collected and stored separately by departments. Organizations should, therefore, conduct thorough data cleansing and deduplication to identify and remove duplicates. This helps to streamline the data merging process, ensuring that only distinct records are stored. 2. Merging Once the pre-processing steps are performed, the data is ready to be merged. Aggregation and integration can be employed to combine data. Depending on the intended use, here are a few ways to execute this process: Append Rows When data is present in different databases and needs to be combined into one, this option is used. To implement this, it is essential that the data sets being merged have an identical structure. For example, if an organization has monthly sales data stored in separate files, it can append the rows to create a consolidated data set covering multiple months to uncover trends or patterns. Append Columns When a company wants to add new elements to its existing data set, i.e., enrich it, appending columns is a suitable approach. Consider a company that has customer data (demographics and contact information) in one database and purchase history in another. By appending the columns on a unique identifier (customer ID), it can have a comprehensive view of the customer profile and purchase patterns, enabling it to run targeted campaigns. Conditional Merge A company might have incomplete or missing records that need filling by looking up values from another database. In this scenario, conditional merge is a helpful approach. Therefore, information from the source database is selectively combined with the target database based on specific rules of alignment to ensure synchronization and accurate information. For instance, a food chain’s restaurants are listed in one database, and the customer ratings are listed in another. To determine the average rating for each restaurant, the two data sets are merged by matching the restaurant names against the correct customer review and rating. Note: In Conditional Merge, the lookup database (Source) should have unique values, while the Target database should have duplicates. 3. Post-merging Once the merging process is complete, organizations should conduct a final audit of the data, like the profiling conducted at the start of the process, to highlight any errors, inaccuracies, or incomplete records so that immediate action can be taken to correct them. Challenges of Data Merging While data merging is critical to high-quality data, enterprises should be mindful of the potential problems that could arise during the process. Some factors to consider include: Data Complexity: While merging the data, structural and lexical differences can introduce inaccuracies into the dataset. Structural heterogeneity refers to a case when data sets under consideration do not have the same columns present, while lexical heterogeneity is when the data fields have a similar structure, but the information contained within them is in a different format. To address this, it is important to invest in tools that define mappings between different data set structures and enable the transformation of data elements to a standard format. Scalability: When datasets are combined, they increase in size and complexity, resulting in tasks such as data matching, alignment, and aggregation becoming more resource-intensive. As data volume increases, storage capacity becomes an emerging concern. Traditional, on-premises systems lack the capability to scale, slowing down the processing time and heightening the risk of inaccuracies. To overcome this, organizations should migrate to cloud-based solutions to handle large volumes of data smoothly. Duplication: Combining different data sets can lead to duplicates, especially when each source might independently capture the same information. Duplication can lead to overlapping information in data sets, resulting in inaccurate analysis and, by extension, incorrect decision-making. To combat this, organizations should employ matching algorithms, perform rigorous data scrubbing, and enforce uniqueness constraints to identify and remove duplicates promptly. Key Strategies for Ensuring Effortless Data Merging Evaluate data sources: Before combining data, organizations should analyze the nature of each data set. This includes understanding the types of variables, data formats, and overall structure. This aids in anticipating potential challenges during the merging process. Use visuals to understand data relationships: Visualizations like scatter plots, bar charts, correlation matrices, etc., provide an overview of the data and help select the right variables for merging. These visuals make it easier to identify patterns, outliers, and relationships within the data, ensuring the inclusion of relevant information. Clean and transform data: It is essential to clean the data by removing duplicates and handling missing values. This ensures the merged dataset is accurate and reliable, minimizing errors and inconsistencies. Choose merging methods carefully: The method of merging depends on the data’s structure and the intended goals. Different merging techniques, such as inner joins, left joins, and outer joins, have specific use cases. It is crucial to select the appropriate method to ensure meaningful data integration. Select the right merging tool: Organizations should conduct proper research and analysis to choose the right tool for their data needs. The tool should be equipped with data profiling, cleansing, and validation features and align with the data’s complexity and the user’s proficiency to simplify the merging process. Validate merged data: After merging, ongoing validation is vital. As new records are introduced in the data set, for example, customer transactions, it becomes imperative to regularly examine the merged data to identify any unexpected discrepancies and ensure that the final data set has up-to-date information. Streamline Data Merging with LIKE.TG LIKE.TG is an enterprise-grade, advanced data management solution that assists users throughout the data lifecycle, from extraction to analysis. By leveraging AI to effortlessly extract unstructured data and utilizing robust data preparation capabilities, the platform accelerates time-to-insights. Moreover, the platform enables users to intelligently read the data sets and perform join/union/lookup operations while monitoring the data health in real-time. Users can define rules and criteria for merging data sets, whether they are joining tables, combining datasets, or performing other data integration tasks. Its intelligent algorithms help users identify matching records efficiently. LIKE.TG empowers users to explore, visualize, interact, and modify data in an interactive and user-friendly manner. With LIKE.TG, users can review the changes made to track the history, providing greater transparency and control over the merging process. Data Merging in LIKE.TG Ready for Seamless Data Merging? Get LIKE.TG’s 14-Day free trial today! Combine Your Data for a Single, Integrated View 14-Day Free Trial
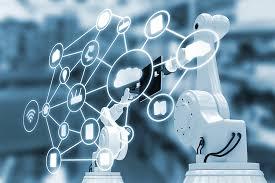
Unveiling 10.3: LIKE.TG Introduces New, Innovative AI Features and Enhanced Capabilities
Uncover hidden insights and possibilities with Generative AI capabilities and the new, cutting-edge data preparation add-on We’re excited to announce the release of LIKE.TG 10.3—the latest version of our enterprise-grade data management platform. This update marks a significant milestone in our journey toward a unified data platform as we’ve introduced new, powerful AI capabilities and features to simplify and accelerate end-to-end data management processes. Elevating the Bar: What Makes This Release Exceptional At the forefront of the 10.3 update is the cutting-edge AI capabilities, enabling data extraction at unprecedented speeds. With just a few clicks, you can effortlessly handle unstructured documents. This empowers large enterprises to swiftly extract valuable information, leading to quicker insights and informed strategies. Moreover, LIKE.TG Dataprep, our new add-on will accelerate and simplify data preparation, empowering you to leverage the full potential of your data. Let’s explore the exciting features of our new platform and see how LIKE.TG can help redefine your data management processes. Enhancements to Existing Solutions Here’s a summary of the new capabilities and upgrades in the 10.3 release: AI-Powered Data Extraction Powered by generative AI technology, the new data extraction feature allows you to effortlessly create extraction templates for multiple files at once. You simply need to specify the document type and layout, and ReportMiner’s intelligent algorithms will effortlessly extract and process data from multiple documents, significantly saving time and resources. This new AI feature accelerates and simplifies document processing. Here’s how it works: Select your source files and use the “Create AI Recommended Layouts” command,streamlining the process effortlessly. Specify the data layout and the fields you want to extract. This option allows you to define the structure of your document. ReportMiner automatically creates templates based on the extracted field values. These templates accurately capture the necessary information from your documents. Review and fine-tune the templates created by our AI feature. You can easily identify the missing fields and refine the templates to align with your specific requirements. Once your templates are finalized, you can effortlessly process multiple files by creating a dataflow. The workflow scheduler within our platform streamlines and fully automates document processing, improving the overall efficiency of your workflow. LIKE.TG Dataprep LIKE.TG Dataprep is an add-on that enhances the process of data cleansing, transformation, and preparation in LIKE.TG. With its user-friendly interface and focus on providing a clear preview of data, LIKE.TG Dataprep complements the powerful capabilities of LIKE.TG and simplifies data preparation tasks. LIKE.TG Dataprep supports data ingestion, cleaning, transformation, and integration, ensuring time savings and streamlined workflows. The key features include: Data Source Browser: The Data Source Browser is a centralized location that houses file sources, catalog sources, cloud sources, and project sources. It provides a seamless way to import these sources into the Dataprep artifact. Grid View: The Grid View presents a dynamic and interactive grid that updates in real time, displaying the transformed data after each operation. It offers an instant preview and feedback on data quality, helping you ensure the accuracy and integrity of your data. Profile Browser: The Profile Browser, displayed as a side window, provides a comprehensive view of the data through graphs, charts, and field-level profile tables. It helps you assess data health, detect issues, and gain valuable insights. Data Integration AI Auto-Mapper: This feature simplifies field mapping by leveraging semantic relationships. By harnessing the power of AI to analyze field context and meaning, it intelligently establishes connections, enhancing accuracy and efficiency in mapping tasks. Windows Authentication: Centerprise now utilizes Windows authentication, enabling you to log into the system using Windows credentials. The Server Browser interface also supports user registration with this authentication method. Modern Auth in SendMail and Cluster Setting: The SendMail object now supports Modern Authentication, following Microsoft’s recommended authentication standards. This update allows you to seamlessly add authentication credentials, ensuring compatibility and simplifying the process. Repo Upgrade Utility: You can upgrade to LIKE.TG Centerprise version 10.3 effortlessly using an executable script that automates repository updates. This utility boosts efficiency and ensures a smoother transition for our existing customers. Resource Catalog: A new centralized repository to store and shares artifacts. It gives you greater control over permissions, allowing selective sharing with desired individuals. API Management Import CAPI Connectors: The API browser in LIKE.TG now offers a simple way to import pre-built and pre-tested CAPI connectors from LIKE.TG’s GitHub repository. These connectors come with a comprehensive list of endpoints and are thoroughly tested and configured for seamless integration. Developer Portal (Beta): LIKE.TG 10.3 introduces a beta release of the developer portal, a customized platform that showcases deployed API products. This central hub provides documentation and support for developers to understand, explore, and use APIs effectively. Multipart/form-data Support: The API Client now supports Multipart/form-data, making it easy to upload and download files through API calls. This update allows you to integrate file transfer capabilities into your services and workflows seamlessly. Certificate Store: This feature enables easy generation and management of certificates for SSL authentication, data encryption, and secure communication. You can generate, import, and export certificates to seamlessly integrate client certificates into your security infrastructure. Show Swagger UI: Swagger UI integration with LIKE.TG API Management allows you to conveniently view API deployments in a user-friendly interface and test API calls on the go, ensuring seamless interaction with well-formatted and comprehensive API documentation. Testflow Generation Enhancements: You can now perform test flow generation directly from the server browser. This update streamlines the testing process for single or multiple APIs. Data Warehousing AI Select: This feature aids you in identifying potential Fact and Dimension tables from selected entities. By leveraging AI capabilities, it automatically determines the appropriate classification, streamlining the data modeling process for entities with uncertain categorization. Infer Relationships with AI: By harnessing AI algorithms, this advanced functionality empowers you to automatically deduce complex relationships between entities, including self-referencing and inter-entity associations. Connectors SharePoint Production Grade: LIKE.TG Centerprise seamlessly connects to Microsoft SharePoint’s Document library, enabling easy file access. You can effortlessly extract and utilize SharePoint files in ETL pipelines, including dataflows and workflows. Google Big Query Production Grade: Google BigQuery is a fast, serverless data warehouse with a built-in query engine. In LIKE.TG Centerprise, you can connect it as a source to read flat and hierarchical files or a destination to write flat files. Azure SQL Production Grade: You can access Azure SQL Databases easily and perform various operations such as DB Lookup or Run SQL Script tasks in a workflow. Azure Data Lake Gen 2 Storage: Azure Data Lake Gen 2 is a cloud-based solution for storing and analyzing big data. It’s available in LIKE.TG Centerprise’s Cloud Connector, enabling seamless connectivity to Microsoft’s cloud storage service. Step Into the Future: Take Charge with LIKE.TG 10.3! LIKE.TG Data Stack 10.3 is here! A unified, all-in-one solution that simplifies complex data workflows and maximizes efficiency. Explore the cutting-edge features and upgraded capabilities that empower you to integrate, transform, and analyze your data seamlessly. Download our 14-day trial version of this enterprise-grade solution today. For any inquiries or assistance, please don’t hesitate to contact us at [emailprotected]. Try LIKE.TG Data Stack First-hand for All Your Data Management Needs Explore our Platform
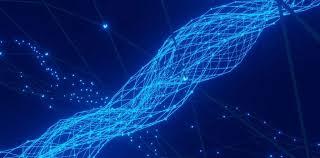
What is ETL (Extract, Transform, Load)? A Complete Guide
What Is ETL?ETL stands for extract, transform, and load. ETL is a process to integrate data into a data warehouse. It provides a reliable single source of truth (SSOT) necessary for business intelligence (BI) and various other needs, such as storage, data analytics, and machine learning (ML).With reliable data, you can make strategic decisions more confidently, whether it’s optimizing supply chains, tailoring marketing efforts, or enhancing customer experiences.The Evolution of ETLTraditional ETL was primarily designed forbatch processingand involved manual processes: data extraction, transformation, and loading being time-consuming and resource-intensive tasks. However, the explosion of real-time data generated by IoT devices, social media platforms, and other sources necessitated a shift towards handling continuousstreams of data.The rise of big data technologies and the emergence of Hadoop, Spark, and NoSQL databases have alsohad a profound impact onETL practices, which have evolved to handle large volumes of data distributed across clusters.The advent of modern ETL tools—now powered by automation and AI—means greater efficiency and scalability fordata integrationprocesses. Sophisticated features for orchestrating, scheduling, monitoring, and managing workflows have become indispensable for organizations as they significantly reduce the need for manual intervention.In addition to technological advancements, ETL processes havealso evolved to address the growing importance of data quality and data governance. Organizations now prioritize ensuring data accuracy and compliance throughout the ETL pipeline.Why Is ETL important?Organizations store and use large amounts of structured and unstructured data to successfully run day-to-day operations. This data comes from multiple sources, and in different formats. For example, customer and marketing data from different channels and CRMs, partner and supply chain data from vendor systems, financial reporting and HR data from internal systems, and so on. The problem is further exacerbated by the fact that these data sets are often isolated, which makes accurate data analysis and effective decision-making a distant reality.ETL enables you to extract data from all these sources, transform it so that every data set conforms to the requirements of the destination system, and load it into a repository where it is easily accessible for analysis. The importance of ETL lies not only in the sheer volume of data that it handles but also in the precision and efficiency with which it manages that data.ETL BenefitsUnified View: Integrating data from disparate sources breaks down data silos and provides you with a unified view of your operations and customers. This holistic picture is critical for informed decision-making.Enhanced Analytics: The transformation stage converts raw, unstructured data into structured, analyzable formats. The data readiness achieved empowers data professionals and business users to perform advanced analytics, generating actionable insights and driving strategic initiatives that fuel business growth and innovation.Historical Analysis: You can store historical data, which is invaluable for trend analysis, identifying patterns, and making long-term strategic decisions. It allows you to learn from past experiences and adapt proactively. TigOperational Efficiency: ETL automation reduces manual effort and lowers operational costs. This newfound efficiency ensures that valuable human resources are allocated to more value-added tasks.Data Quality: ETL facilitates data quality management, crucial for maintaining a high level of data integrity, which, in turn, is foundational for successful analytics and other data-driven initiatives.Build Fully Automated ETL Pipelines With a 100% No-Code UILearn MoreETL Process: How Does ETL Work?Extract, transform, and load (ETL) works by extracting data from various sources, transforming it to suit the requirements of the destination system, and loading it into a data warehouse. ETL is a three-step process:Data ExtractionThe process starts by extracting raw data from relevant data sources, including databases, files, etc. The extracted data is stored in a landing zone, also called a staging area. A staging area is an intermediate storage where data is only stored temporarily.There are three common ways to extract data in ETL:Incremental ExtractionOnly new or changed data since the last extraction is pulled in this method. This approach is common when dealing with large data sets as it reduces the amount of data transferred. For instance, you might extract only the new customer records added since the last time you extracted data.Full ExtractionThis extracts all data from the source system in one go. For example, a full extraction would mean pulling all customer records if you’re extracting data from your customer database.Update NotificationIt focuses on monitoring changes in data and notifying relevant parties or systems about those changes before data extraction. You can use this method when you need to keep stakeholders informed about updates or events related to a data set.Data TransformationData transformation is the second stage in the process of ETL. Data stored in the staging area is transformed to meet business requirements since the extracted data lacks standardization. The degree to which the data is transformed depends on factors such as data sources, data types, etc.Any improvements to data quality are also finalized here. Data teams typically rely on the following data transformations to maintain data integrity during ETL:Data CleansingIt includes identifying and correcting errors or inconsistencies in data sets to ensure data accuracy and reliability. For example, in a customer database, data cleaning could involve removing records with missing email addresses, correcting typographical errors in customer names, etc.Data DeduplicationDeduplication identifies and removes duplicate or redundant records within a data set. The process involves comparing data records based on specific criteria, such as unique identifiers or key attributes, and removing duplicate entries while retaining one representative record. It helps in reducing data storage requirements and improving data accuracy.Joins and Tree JoinsJoins are operations in database management and data processing that combine data from two or more tables based on related columns. It allows you to retrieve and analyze data from multiple sources in a unified manner.Tree joins are used in hierarchical data structures, such as organizational charts, to connect parent and child nodes. For instance, in a hierarchical employee database, a tree join would link employees to their respective supervisors, creating a hierarchy that reflects the organizational structure.Normalization and De-normalizationNormalization involves organizing a database schema to minimize data redundancy and improve data integrity. You can achieve this by breaking down tables into smaller, related tables and defining relationships between them.On the other hand, de-normalization involves intentionally introducing redundancy into a database schema to optimize query performance. This might entail merging tables, duplicating data, or using other techniques that make data retrieval faster at the expense of some data redundancy.MergeMerge transformation is commonly used in ETL to consolidate information from various sources. It is a data transformation operation that combines data from two or more data sets or sources into a single data set by aligning records based on common attributes or keys.Data LoadingLoading data into the target system is the last step in the ETL process. The transformed data is moved from the staging area into a permanent storage system, such as a data warehouse.The loaded data is well-structured, which data professionals and business users can use for their BI and analytics needs. Depending on your organization’s requirements, you can load data in a variety of ways. These include:Full LoadAs the name suggests, the entire data from the source systems is loaded into the data warehouse without considering incremental changes or updates. Full loads are often used when initially populating a data warehouse or starting a new data integration process. In such cases, you need to bring all the historical data from the source into the target system to establish a baseline.It’s important to note that while a full load is suitable for initial data setup, it’s not practical for continuous, real-time, or frequent data updates. In such cases, incremental loading or other strategies should be employed to optimize resource utilization.Batch LoadBatch loading in ETL refers to the practice of processing and loading data in discrete, predefined sets or batches. Each batch is processed and loaded sequentially. Batches are typically scheduled to run at specific intervals, such as nightly, weekly, or monthly.Bulk LoadA bulk load refers to a data loading method that involves transferring a large volume of data in a single batch operation. It is not specific to whether all data is loaded or only a subset. Instead, bulk loading can be employed in various scenarios, including both full and incremental load. Think of it as a loading method to optimize the speed and efficiency of data transfer.Incremental LoadIncremental load only loads the new or changed data since the last ETL run. It’s used in situations where it is necessary to minimize the data transfer and processing overhead when dealing with frequently changing data sets.StreamingIn this case, data is loaded in near real-time or real-time as it becomes available. It is often used for streaming data sources and is ideal for applications requiring up-to-the-minute data for analytics or decision-making. Streaming user activity data into a real-time analytics dashboard is a common example.Start Your ETL Journey With LIKE.TG – 14-day Free TrialDownload TrialETL vs. ELTExtract, transform, and load (ETL) and extract, load, and transform (ELT) are two of the most common approaches used to move and prepare data for analysis and reporting. So, how do they differ? The basic difference is in the sequence of the process. In ELT, data transformation occurs only after loading raw data directly into the target storage instead of a staging area. However, in ETL, you must transform your data before you can load it.The table below summarizes ETL vs. ELT:ETL (extract, transform, load)ELT (extract, load, transform)SequenceExtracts data from the source first, then transforms it before finally loading it into the target system.Extracts data from the source and loads it directly into the target system before transforming it.Data TransformationData transformation occurs outside the destination system.Data transformation occurs within the destination system.PerformanceLikely to have performance issues when dealing with large data sets.Can benefit from parallelization during loading due to modern distributed processing frameworks.StorageRequires an intermediate storage location for staging and transforming data, called staging area.May use direct storage in the destination data store.ComplexityTypically involves complex transformation logic in ETL tools and a dedicated server.Simplifies data movement and focuses on data transformation inside the destination.ScalabilityRequires additional resources for processing large data volumes.Can scale horizontally and leverage cloud-based resources.ExamplesTraditional scenarios like data warehousing.Modern data analytics platforms and cloud-based data lakes.What Is an ETL Pipeline?ETL pipeline is the means through which an organization carries out the data extraction, transformation, and loading processes. It’s a combination of interconnected processes that execute the ETL workflow, facilitating data movement from source systems to the target system.These pipelines ensure that the data aligns with predefined business rules and quality standards. You can automate your pipelines and accelerate the process using data integration tools to further your data-driven initiatives.Data Pipeline vs. ETL PipelineAt the most basic level, a data pipeline is a set of automated workflows that enable data movement from one system to another. Compared to ETL pipelines, data pipelines may or may not involve any data transformations. In this context, an ETL pipeline is a type of data pipeline that moves data by extracting it from one or more source systems, transforming it, and loading it into a target system.Read more about the differences between data pipeline vs. ETL pipeline.What is Reverse ETL?Reverse ETL is a relatively new concept in the field of data engineering and analytics. It involves moving data from analytical storage systems, such as data warehouses and data lakes, back into operational systems, applications, or databases that are used for day-to-day business operations. So, the data flows in the opposite direction.While traditional ETL processes focus on extracting data from source systems, transforming it, and loading it into data warehouses and data lakes for analysis, reverse ETL is geared towards operational use cases, where the goal is to drive actions, personalize customer experiences, or automate business processes.This shift in data movement is designed to empower non-technical users, such as marketing teams or customer support, with access to enriched, up-to-date data to fuel real-time decision-making and actions.Looking for the best ETL Tool? Here's what you need to knowWith so many ETL Pipeline Tools to choose from, selecting the right solution can be overwhelming. Here's a list of the best ETL Pipeline Tools based on key criteria to help you make an informed decision.Learn MoreETL Challenges to Be Aware OfData Quality and Consistency: ETL heavily depends on the quality of input data. Inconsistent, incomplete, or inaccurate data can lead to challenges during transformation and may result in flawed insights. Ensuring data quality and consistency across diverse sources can be a persistent challenge.Scalability Issues: As data volumes grow, you may face scalability challenges. Ensuring that the infrastructure can handle increasing amounts of data while maintaining performance levels is a common concern, especially for rapidly growing businesses.Complexity of Transformations: Complex business requirements often necessitate intricate data transformations. Designing and implementing these transformations can be challenging, especially when dealing with diverse data formats, structures, business rules, or using SQL to ETL data.Data Security and Compliance: Handling sensitive information while moving data raises concerns about data security and compliance. Ensuring that data is handled and transferred securely poses a continuous challenge.Real-time Data Integration: The demand for real-time analytics has grown, but achieving real-time data integration via ETL can be challenging. Ensuring that data is up-to-date and available for analysis in real-time requires sophisticated ETL solutions and can be resource intensive.How Do ETL Tools Help?Extract, transform, and load (ETL) tools help businesses organize and make sense of their data. They streamline data collection from various sources, transforming it into a more digestible and actionable format.Here’s how you can benefit from ETL tools:ETL AutomationETL tools streamline ETL workflows by automatically extracting data from various sources, transforming it to your desired format, and loading it into a central data repository. This process operates autonomously and reduces the need for manual processes, such as coding for ETL (SQL for data extraction and transformation). You can efficiently handle vast data volumes without the expenditure of excessive time and human resources, leading to increased operational efficiency and cost savings for your organization.Single Source of Truth (SSOT)In the contemporary business landscape, data often resides in multiple systems and formats, leading to inconsistencies and discrepancies. ETL tools bridge these divides, harmonizing data into a unified format and location. This SSOT serves as a reliable foundation for decision-making, ensuring that all stakeholders access consistent and accurate information.Real-Time Data AccessIn the age of instant gratification and rapid decision-making, businesses require access to up-to-the-minute data insights to remain competitive. Modern ETL tools offer the capacity to integrate real-time data streams, enabling you to respond promptly to changing circumstances and trends. This real-time data access equips your business with a competitive edge, as you can make agile decisions based on the most current information available.Better ComplianceBusinesses today operate in a heavily regulated environment, necessitating compliance with regulations such as HIPAA and GDPR. Modern ETL tools offer features such as data lineage tracking and audit trails, which are critical for demonstrating adherence to data privacy, security, and other compliance mandates. This capability mitigates legal and reputational risks, safeguarding your organization’s standing in the market.Better ProductivityThese tools liberate human resources to focus on higher-value tasks by automating labor-intensive data integration and transformation processes. Employees can direct their efforts toward data analysis, interpretation, and strategy formulation rather than spending excessive hours on manual data wrangling or using SQL to ETL data. This shift in focus amplifies productivity, fosters innovation, and drives business growth.See LIKE.TG's No-Code ETL in ActionView DemoETL Best Practices to KnowOptimize company-wide data management processes by incorporating the following ETL best practices into your data warehouse strategy:Understand Your Data SourcesBegin by identifying all the data sources that you need to extract data from. These sources can include databases, files, APIs, web services, and more. You should also understand the individual source’s structure, location, access methods, and any relevant metadata.Prioritize Data QualityData profiling provides insights into the data’s characteristics and enables you to identify issues that might impact its reliability and usability. By identifying anomalies early in the process, you can address these issues before they propagate into downstream systems, ensuring data accuracy and reliability.Use Error LoggingEstablish a uniform logging format with details like timestamps, error codes, messages, impacted data, and the specific ETL step involved. Additionally, categorize errors with severity levels, for example, INFO for informational messages, WARNING for non-fatal issues, and ERROR for critical problems, to enable prioritization and efficient troubleshooting. This systematic error-logging practice empowers data professionals to swiftly identify and resolve issues that may arise during the process.Use Incremental Loading for EfficiencyUse change data capture (CDC) for incremental loading if you want to update only the new or changed data. It reduces processing time and resource consumption. For example, a financial services company can significantly optimize the performance of its ETL pipelines by using the incremental loading technique to process the daily transactions’ data.Use ETL Tools to Automate the ProcessUse automated ETL tools to build your ETL pipeline and streamline company-wide data integration. Automated workflows follow predefined rules and minimize the risk of errors that are otherwise highly likely with manual processing. Leveraging tools that offer automation features can do wonders for your business as they offer a visual interface for designing workflows and scheduling ETL jobs.ETL Use CasesHere are some ETL use cases that are applicable to most organizations:Data WarehousingETL is one of the most widely used methods for collecting data from various sources, making it clean and consistent, and loading it into a central data warehouse. It enables you to generate reports and make informed decisions. For instance, retail companies can combine sales data from stores and online sales platforms to gain insights into customer buying patterns and optimize their inventory accordingly.Legacy System ModernizationIn the context of legacy system migration and modernization, ETL can help your business transition from outdated systems to modern platforms. It can extract data from legacy databases, convert it to a format compatible with contemporary systems, and seamlessly integrate it.This use case is crucial for sectors such as healthcare, where patient records must be migrated to modern electronic health record systems while preserving data accuracy and accessibility.Real-Time Data IntegrationReal-time data integration is another key application, especially beneficial if your business needs to respond instantly to changing data streams. You can optimize ETL to continuously extract, transform, and load data as it’s generated. For online retailers, this could mean leveraging real-time customer behavior data to personalize product recommendations and pricing strategies in the ever-changing e-commerce landscape.Cloud MigrationETL is indispensable when it comes to data migration and transitioning to cloud environments. It extracts data from on-premises systems, adapt it for compatibility with cloud platforms, and load it seamlessly into the cloud. Startups and enterprises alike benefit from it in their quest for rapid scaling, taking full advantage of cloud resources without compromising data consistency or availability.Improving Data QualityBusinesses leverage ETL to enhance their data quality management efforts. You can utilize several techniques, such as data profiling, validation rules, and data cleansing, to detect and rectify anomalies in data sets. By ensuring data integrity at the extraction, transformation, and loading stages, you make decisions based on reliable and error-free data. This not only minimizes costly errors and operational risks but also cultivates trust in the data, enabling informed and precise decision-making across various business functions.Start Your ETL Journey With LIKE.TG – 14-day Free TrialDownload TrialLIKE.TG—the Automated ETL Solution for All BusinessesLIKE.TG is an end-to-end data management solution powered by artificial intelligence (AI) and automation. From data extraction to transformation to loading, every step is a matter of drag-and-drop with LIKE.TG’s intuitive, visual UI.LIKE.TG empowers you to:Connect to a range of data sources and destinations with built-in connectorsExtract data from multiple sources, whether structured or unstructuredTransform data according to business requirements with pre-built transformationsLoad healthy data into your data warehouse using embedded data quality featuresBuild fully automated ETL pipelines without writing a single line of codeWant to learn more about our 100% no-code ETL platform? Sign up for a demo or contact us.
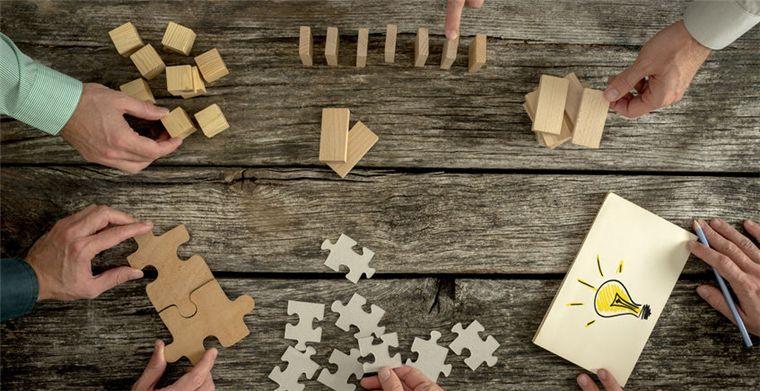
EDI Cost Savings: Transforming Data Management in Healthcare
Due to the growing volume of data and the necessity for real-time data exchange, effective management of data has grown increasingly important for businesses. As healthcare organizations are adapting to this change, Electronic Data Interchange (EDI) is emerging as a transformational solution. EDI offers a standardized and automated approach to data exchange. This method helps organizations streamline operations, reduce errors, and save significant costs. By substituting manual and paper-based procedures, which are often riddled with errors and delays, businesses can employ a digital system. This system promotes quick and precise transactions, thereby driving efficiency and cost-effectiveness in data management. The Ultimate EDI Solution For The Healthcare Industry Explore Now! How Does EDI Save Costs? In healthcare, managing vast amounts of data is an everyday task. Patient records, billing information, insurance details, and more all require efficient data management processes. Implementing EDI in healthcare systems can address significant and sometimes hidden costs, delivering substantial savings. Enhanced Efficiency in Healthcare Processes EDI shines brightly in the healthcare sector due to its ability to enhance efficiency. Traditional data entry methods, often riddled with inaccuracies and delays, can lead to a host of avoidable expenses. By automating these processes, EDI paves the way for efficient and swift data exchange. For instance, healthcare providers can speed up the billing cycle, resulting in quicker payments and improved cash flow. Less manual data processing also means lower labor costs and more time for strategic initiatives, boosting productivity. Reduced Error Rates in Patient Data Management Data errors in healthcare can lead to serious consequences, including incorrect patient information, billing mistakes, and delayed treatments. These errors incur direct rectification costs along with causing damage to the healthcare provider’s reputation. EDI reduces manual data handling, significantly curbing the potential for human error. Given the direct and indirect costs of error rectification, the savings from this error reduction can be substantial. Streamlined Supply Chain Management Healthcare providers need a wide inventory of medical supplies and pharmaceuticals. EDI offers real-time data exchange, which allows for precise inventory management. By reducing overstocking and stockouts, healthcare providers can save significant costs. Decrease in Paper Usage Expenses related to printing, storing, and securely disposing of paper records add up quickly. In addition, these systems indirectly impact the environment and can potentially harm a provider’s reputation among eco-conscious patients. EDI reduces the dependence on paper, thus improving efficiency and environmental sustainability. Besides cost reduction, this change also strengthens a provider’s commitment to eco-friendly practices, enhancing their reputation among various stakeholders. Automated Insurance Claim Processing The insurance claim processing in healthcare can be a labyrinth of procedures involving various stakeholders, each with unique data requirements. Missteps along the way, from incorrect patient information to improper billing codes, may lead to rejections, adding more processing time and effort. EDI implementation in healthcare provides a structured and consistent approach to claim processing. It standardizes data exchange between healthcare providers and payers, making the process more efficient and less prone to errors. In turn, this accelerates the reimbursement process and improves the cash flow for healthcare providers. By leveraging EDI, providers can effectively navigate the complexity of insurance claims, avoid costly delays, and mitigate the risk of payment denials. Improved Regulatory Compliance Healthcare providers are mandated to comply with several regulations, such as the Health Insurance Portability and Accountability Act (HIPAA). Failure to adhere to these rules could result in significant fines and potential reputational damage. Implementing an EDI system can help healthcare providers avoid these pitfalls by inherently supporting compliance with regulatory standards. EDI provides secure data transmission, safeguarding sensitive patient information and maintaining privacy. Enhancing compliance mitigates the risk of costly penalties while simultaneously protecting the healthcare provider’s reputation. Improved Patient Satisfaction and Retention By ensuring accurate and timely billing, quick resolution of insurance claims, and efficient transfer of medical records, EDI enhances the patient experience. Satisfied patients are more likely to continue using the healthcare provider’s services, which means lower patient acquisition costs in the long term. By automating and streamlining processes, enhancing compliance, and improving patient experiences, EDI can significantly reduce costs in the healthcare sector. These savings go beyond operational costs and impact the bottom line, making EDI a valuable investment for healthcare providers. Free E-book - Optimizing Healthcare Processes With EDI Streamline Healthcare Ops Now! Case Study: Cost Savings Realized Through EDI Implementation Chorus Community Health Plans (CCHP), a non-profit Health Maintenance Organization (HMO) affiliated with Children’s Wisconsin, provides healthcare services to over 140,000 adults and children across 28 counties in the state. Like many Health Maintenance Organizations (HMOs), CCHP had a major challenge: they had to ensure a high rate of data submission and approval for patient encounters. This was critical because the Wisconsin Department of Health Services used this data to calculate capitation rates, which directly affected CCHP’s revenue. Before implementing an EDI solution, the organization’s process of reviewing and correcting EDI files was labor-intensive. Each transaction document could take up to three hours to rectify, consuming significant time and resources. This demanding manual process was also prone to human errors. The Solution: LIKE.TG EDIConnect Recognizing these inefficiencies, CCHP decided to revolutionize its process by leveraging the power of an EDI tool. The choice of which tool to select was clear; LIKE.TG EDI Connect stood out with its superior functionality, effortless learning curve, and intuitive development environment that made the process a breeze. The Results: Improved Approval Rates and Cost Savings Implementing LIKE.TG’s EDI Connect led to impressive results. CCHP’s data submission and approval rates skyrocketed to 99%. The improved data accuracy led to a higher number of accepted claims that solidified its Medicaid-certified vendor status and brought in more revenue from the state. By automating their EDI process, CCHP saved about five weeks’ worth of manual coding and reviewing efforts to resolve errors, which roughly translated into $26,000 per year. Additionally, the organization saved $65,000 per year that it would have spent hiring an additional full-time employee to manage these EDI files. Moreover, due to the improved EDI data accuracy, CCHP could retain over $100,000 in reimbursements, directly contributing to their bottom line. In a nutshell, LIKE.TG’s EDI Connect played a crucial role in helping CCHP streamline its operations, reduce costs, and improve data accuracy. Now, CCHP enjoys a seamless, automated EDI process, which allows them to focus more on their primary mission of providing top-quality healthcare services to their members. Unlocking Potential with EDI The transformative power of Electronic Data Interchange (EDI) is evidently significant in optimizing business operations, as reflected by CCHP’s successful adoption of LIKE.TG EDIConnect. This case study serves as a testament to the substantial benefits of automating EDI processes.By embracing modern data exchange methods, healthcare organizations can unlock significant cost savings and operational enhancements. Interested in exploring how EDI solutions can improve healthcare operations? Contact us today! Contact!
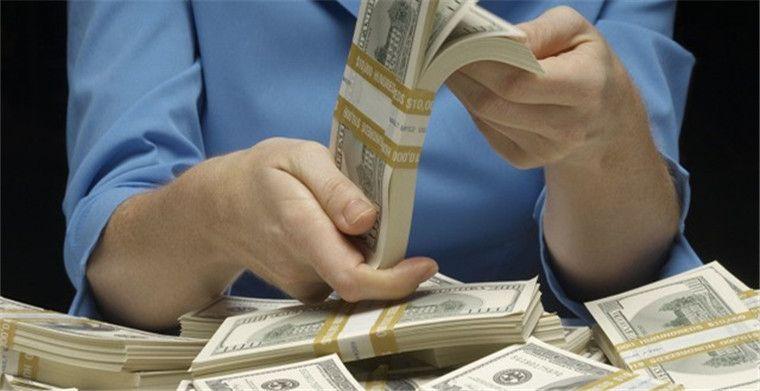
Streamlining Prior Authorization with EDI 278: Minimizing Costs, Delays, and Provider Burnout
Manual prior authorization (PA) is a critical step for medical coverage approval but is notoriously time-intensive, with physicians spending an average of 41 hours weekly to process these requests. This not only leads to provider burn out but delays caused by manual prior authorization an also impact patient care. To address this, legislation in over 30 states in 2023 advocates for a shift to electronic PA processes. PA requests surged by 61% after lifting COVID-19 restrictions, yet a staggering 72% are still manually processed. The Electronic Data Interchange (EDI) 278 transaction set is designed to overhaul these outdated practices, offering a solution that cuts down on claim rejections, streamlines workflows, prevents delays in patient care, and reduces provider burnout, leading to overall improved healthcare delivery The Ultimate EDI Solution For The Healthcare Industry Explore Now! The Challenges: Delays in Patient Care In the traditional system, a patient’s urgent need for medication is met with a slow, manual prior authorization (PA) process. Healthcare providers send PA requests via fax, which then undergoes a lengthy review by the insurance provider, involving multiple departments and often requiring additional information. On average, manual PA requests can take anywhere from one day to one month to complete, due to varying requirements across payers. Such delays can have a significant impact on patient health outcomes. According to a survey of healthcare providers, 94% believe that PA restrictions cause delays in accessing necessary care. 89% of respondents believe PA delays have a negative impact on patient clinical outcomes. Likewise, 82% of doctors said prior authorization sometimes leads to patients abandoning a recommended course of treatment. Provider Burnout Prior authorization often involves complex administrative tasks, such as manually filling out forms, gathering supporting documentation, and submitting requests through multiple channels. Physicians, on average, handle 45 PA requests per week, creating significant administrative burdens and contributing to provider burnout. Claim Denials Approximately 80% of denied claims result from a lack of authorization or improper requests, creating significant financial burdens for providers. Streamlining prior authorization can help providers reduce claim denials. Increasing Cost amongst PA Surge Prior authorization has garnered attention for its inefficiencies, delays, and administrative burdens, but the cost of processing these requests manually has also increased over the years. Manual data entry, paper-based workflows, errors or duplications of various degrees contribute to increased during in prior authorization.The recent surge in PA requests has exacerbated the issue, leading to even higher administrative spending. EDI 278’s Role in Enhancing Prior Authorization Workflows EDI 278 replaces manual processes with electronic transactions, streamlining and enhancing prior authorization (PA) workflows in the healthcare industry. Streamlining Response Times EDI 278 replaces manual processes, such as faxing PA requests and waiting up to a month for approval, with electronic submissions. This allows healthcare providers to submit requests directly to the insurer’s system, where they are automatically directed to the correct department for processing. According to the CAQH Index 2022, EDI 278 requests take 11 minutes less to process compared to manual PA requests, potentially saving providers up to 35.36 hours per physician each month. Improving Patient Care EDI 278 ensures the request is automatically and efficiently routed to the correct department within the insurer’s system. With EDI 278, insurers too can automate their workflows and process requests more quickly, enabling them to respond to PA requests within hours instead of days or months. The impact of this swift process is profound. Patients can get the treatment they need approved on the same day, thereby improving their chances of a better health outcome. Reducing Administrative Burden EDI 278 automates prior authorization requests processes by automating electronic data entry and document submission. This automation significantly reduces administrative burdens on providers. As a result, healthcare providers experience a significant reduction in manual work, freeing up valuable time and resources to focus on patient care. Additionally, it helps reduce provider burnout. Increasing Accuracy EDI 278 ensures the accuracy and completeness of information in PA requests, reducing the chance of claim denials. Providers and payers can send and receive authorization requests promptly, leading to early resolution of issues. Saving Time and Costs PA workflows enabled by EDI 278 can help providers save time and resources and avoid unnecessary costs. EDI 278 eliminates the need for manual data entry, reducing paperwork and the potential for errors. According to an HFMA study, EDI 278 is 5.25 times faster than manual PA requests and twice as fast as web portal processing. Likewise, according to the CAQH Index 2022, organizations can save up to $645 million annually by transitioning from manual workflows. Transforming Prior Authorization with LIKE.TG EDIConnect With the ever-increasing demand for efficient prior authorization in US healthcare, EDI 278 has emerged as a game-changer, replacing cumbersome manual processes. By streamlining workflows, automating administrative tasks, and enhancing data accuracy, EDI 278 reduces delays and lowers operational expenses, ultimately improving patient care and financial outcomes. In this landscape, LIKE.TG EDIConnect takes the stage as a comprehensive solution, empowering healthcare organizations to leverage EDI 278 for streamlined prior authorization. Seamlessly integrating EDI 278 transactions, LIKE.TG EDIConnect delivers cost savings, faster response times, and reduced administrative burden. How LIKE.TG simplifies EDI 278 workflows to streamline prior authorization LIKE.TG EDIConnect offers: Data Translation and Mapping: Simplify data exchange by automating translation and mapping between different EDI formats, ensuring accurate communication with trading partners and reducing errors. Validation and Compliance: Ensure data accuracy and compliance by performing real-time validation checks against industry standards and trading partner specifications, minimizing errors, improving data integrity, and meeting regulatory requirements. Secure Communication: Safeguard sensitive patient information through secure communication protocols and ensuring compliance with data security regulations. Seamless Integration with Provider Systems: Seamlessly integrate with existing provider systems, such as EHR and prior authorization software, eliminating manual data entry, reducing administrative burdens, and enhancing operational efficiency. Real-time Updates and Communication: Enable real-time updates, prompt decision-making, and efficient coordination between providers and payers. Receive timely authorization responses, track authorization status, engage in real-time communication, and receive alerts and notifications for any changes in the authorization process. Streamline your prior authorization process with EDI 278 and LIKE.TG EDIConnect. Join our demo session and see how you can benefit from faster, accurate, and secure data exchange between providers and payers. Improve patient care and financial outcomes with lower costs, quicker responses, and fewer denials.
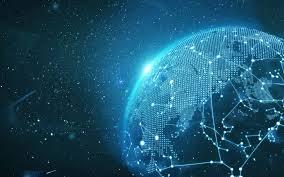
What is Data Integration? Definition, Benefits, & Best Practices
What is Data Integration?Data integration is all the different ways of combining and centralizing organizational data in a cloud data warehouse or a data lake for various purposes. This article serves as a complete guide to data integration, covering its definition, types and techniques, benefits, challenges, use cases, and best practices.Data Integration DefinitionData integration is a strategic process that combines data from multiple sources to provide organizations with a unified view for enhanced insights, informed decision-making, and a cohesive understanding of their business operations.The data integration processData integration is a core component of the broader data management process, serving as the backbone for almost all data-driven initiatives. It ensures businesses can harness the full potential of their data assets effectively and efficiently. It empowers them to remain competitive and innovative in an increasingly data-centric landscape by streamlining data analytics, business intelligence (BI), and, eventually, decision-making.The ultimate goal of integrating data is to support organizations in their data-driven initiatives by providing access to the most up-to-date data. In other words, data integration means breaking down data silos and providing enterprises with a single source of truth (SSOT). The concept of SSOT implies that data must be accurate, consistent, and readily available for use across the organization, a critical requirement for making effective business decisions.Data integration is not merely a technical endeavor. Instead, it transcends the domain of IT and serves as the foundation that empowers business users to take charge of their own data projects.Data Integration vs Data IngestionBothdata ingestionand data integration are essential processes in data management. However, they serve different purposes. While data ingestion focuses on bringing data into a storage or processing environment, data integration goes beyond and unifies, transforms, and prepares data for analysis and decision-making.Here are the main differences between the two processes:Data IngestionData IntegrationDefinitionImports data into a storage or processing system.The process of combining data from diverse sources into a unified and cohesive view.ObjectiveTo bring data into a storage or processing environment as quickly as possible.To create an accurate and comprehensive representation of data for analysis, BI, and decision-making.FocusThe initial stage of data acquisition.Encompasses the broader process of data standardization.Data MovementData movement from source to destination, with minimal transformation.Data movement in integration involves data cleaning, transformation, formatting, and standardization.Data Quality ConsiderationEmphasis is on data availability rather than extensive data quality checks.Enforces data quality standards through transformations and cleansing as part of the integration process.Use CasesUse cases include data lakesanddata warehouses for storage and initial processing.Use cases include creating data warehouses, data marts, and consolidated data views for analytics and reporting.ExampleCollecting log files from multiple servers and storing them in a data lake.Extracting, transforming, and loading customer data from various CRM systems into the central customer database for analytics.Data Integration vs Application IntegrationApplication integration is another concept that’s frequently used in this space. It’s important to differentiate between application integration and data integration, especially since the two often complement each other in achieving seamless operations.While application integration focuses on enabling software applications to work together by sharing data, the latter focuses on consolidating and harmonizing data from disparate sources for analysis and decision-making. Once again, we have a table below to summarize the differences between the two:Application IntegrationData IntegrationDefinitionConnecting and coordinating software applications and systems for data sharing and process automation.Combining data from various sources into a unified and accurate view for analysis and decision-making.ScopeEnable applications to work together seamlessly.Data consolidation and harmonization from multiple sources, focusing on data movement and transformation.Business ObjectiveEnhancing business process efficiency, automating workflows, and improving user experiences through seamless application interactions.Providing a holistic view of data across the organization, supporting data-driven decision-making, reporting, and analytics.Data FlowManaging data and process flow between applications, ensuring real-time communication and collaboration.Involves data extraction, transformation, and loading processes, among others.Use CasesIntegrating CRM with marketing tools, connecting e-commerce websites with inventory management systems, etc.Creating centralized data warehouses, consolidating customer data, merging data for financial reporting, etc.Tools and TechnologiesMiddleware, APIs, message queues, ESBs, integration platforms, and API gateways.Data integration and ETL tools, data warehouses, data lakes, and database management systems.How Does Data Integration Work?The data integration process can be a challenge, especially if you deal with multiple data sources. Each source may have its own format, structure, and quality standards, making it essential to establish a robust data integration strategy.Additionally, you’ll need to plan your project to ensure data accuracy and timeliness throughout the process. Overcoming these challenges often involves using specialized data integration toolsthat streamline the process and provide a unified, reliable dataset for informed decision-making and analysis.As far as the process is concerned, it can be done in real time, in batches, via streaming, etc. Generally, though, the data integration process involves the following key steps:Identifying Data SourcesThe first step is to consider where your data is coming from and what you want to achieve with it. This means you’ll need to identify the data sources you need to integrate data from and the type of data they contain. For example, depending on your organization and its requirements, these could include databases, spreadsheets, cloud services, APIs, etc.Data ExtractionOnce you have your sources in mind, you’ll need to devise an efficient information extraction plan to pull data from each source. Modern organizations use advanced data extraction tools to access and retrieve relevant information. These tools are powered by artificial intelligence (AI) and machine learning (ML) algorithms and automate the entire extraction process, including document data extraction.Data TransformationTransforming the extracted data is the next step in data integration. You may have data in various formats, structures, or even languages when your data sources are disparate. You’ll need to transform and standardize it so that it’s consistent and meets the requirements of the target system or database.Organizations use specializeddata transformation toolssince the process can become tedious if done manually. Data transformation typically includes applying tree joins and filters, merging data sets, normalizing/de-normalizing data, etc.Data Quality ImprovementWhen consolidating data, you’ll find it often comes with errors, duplicates, or missing values. A robust data quality managementframework will ensure that only healthy data populates your destination systems. It involves checking data for incompleteness, inaccuracies, and other issues and resolving them using automateddata quality tools.Data MappingData mapping involves defining how data from different sources correspond to each other. More specifically, it is the process of matching fields from one source to fields in another. Therefore, it’s a step of significant importance in data integration. Data mapping tools automate this step as they provide intuitive, drag-and-drop UI, ensuring that even non-technical users can easily map data and build data pipelines.Data LoadingOnce you correctly map your data, the next step is all about loading it into a central repository, such as a database or a data warehouse. Loading only healthy data into this central storage system guarantees accurate analysis, which in turn improves business decision-making. Apart from data being accurate, it’s also important that data be available as soon as possible. Today, organizations frequently employ cloud-based data warehouses or data lakes to benefit from the cloud’s uncapped performance, flexibility, and scalability.Data SynchronizationAfter your initial integration, set up a mechanism for continuous data synchronization. This could be periodic updates or, in cases where real-time data is crucial, it might involve immediate synchronization as new data becomes available. Note that data synchronization needs oversight. So, you need to monitor the process to identify any hiccups or discrepancies in the integrated data and ensure it’s working as intended.Data Governance and SecurityEnsure data security, privacy, and compliance with regulations by implementing data governance policies. You may need to set up access controls, encryption, and auditing measures to safeguard your data, especially if your business operates in a highly regulated industry, for example, finance or healthcare.Metadata ManagementMaintain a metadata repository to document information about your integrated data. This should include details about its source, transformation processes, and business rules. Doing so will help you understand and manage your integrated data environment more effectively.AnalysisOnce your data is integrated, it’s ready for consumption. Depending on your requirements, you may need to use a combination of various tools like BI software, reporting tools, or analytics platforms to access and present the integrated data. Whether it’s understanding customer behavior, optimizing operations, or making strategic choices, the insights you gain are the fruits of your data integration efforts.However, the process does not stop here, the insights gained might prompt adjustments in your data integration strategy. It’s a bit of a feedback loop – the more you learn from the data, the better you can refine your integration processes for future insights.Explore Cloud Data Integration In Depth With This GuideLearn MoreTypes of Data IntegrationTypes of data integration generally refer to the different data integration techniques useful in different scenarios. They are also referred to as data integration strategies or methods.On the other hand, data integration technologiesrefer to the platforms, tools, or software solutions that facilitate data integration.Data Integration Techniques and StrategiesThese are the different ways of integrating data. Depending on your business requirements, you may have to use a combination of two or more data integration approaches. These include:Extract, Transform, Load (ETL)ETL has long been the standard way of integrating data. This data integration strategy involves extracting data from multiple sources, transforming the data sets into a consistent format, and loading them into the target system. Consider using automated ETL tools to accelerate data integration and unlock faster time-to-insight.Extract, Load, Transform (ELT)Similar to ETL, except for the sequence of the rest of the process, data extraction is the first step inELT, which is a fairly recent data integration technique. Instead of transforming the data before loading it into, say, a data warehouse, the data is directly loaded into the target system as soon as it’s extracted. The transformation takes place inside the data warehouse, utilizing the processing power of the storage system.Change Data Capture (CDC)Change data capture is a way to integrate data by identifying and capturing only the changes made to a database. It enables real-time or near-real-time updates to be efficiently and selectively replicated across systems, ensuring that downstream applications stay synchronized with the latest changes in the source data.Enterprise Data IntegrationWhen it comes to integrating data across an organization, it doesn’t get any broader than this. Enterprise data integration is a holistic strategy that provides a unified view of data to improve data-driven decision-making and enhance operational efficiency at the enterprise level.It is typically supported by a range of technologies, such as ETL tools, APIs, etc. The choice of technology depends on the enterprise’s specific data integration needs, existing IT infrastructure, and business objectives.Data FederationData federation, also known as federated data access or federated data integration, is an approach that allows users and applications to access and query data from multiple disparate sources as if they were a single, unified data source system. It provides a way to integrate and access data from various systems without physically centralizing or copying it into a single repository. Instead, data remains in its original location, which users can access and query using a unified interface.However, data federation can introduce some performance challenges. For example, it often relies on real-time data retrieval from multiple sources, which can impact query response times.Data VirtualizationData virtualization allows organizations to access and manipulate data from disparate sources without physically moving it. It provides a unified and virtual view of data across databases, applications, and systems. Think of it as a layer that abstracts these underlying data sources, enabling users to query and analyze data in real-time.Data virtualization is a valuable data integration technique for organizations seeking to improve data agility without the complexities of traditional ETL processes.Middleware IntegrationIn simple terms, middleware integration is a data integration strategy that focuses on enabling communication and data transfer between systems, often involving data transformation, mapping, and routing. Think of it as a mediator that sits in the middle and connects different software applications, allowing them to perform together as a cohesive unit.For example, you can connect your old on-premises database with a modern cloud data warehouse using middleware integration and securely move data to the cloud.Data PropagationData propagation is when information or updates are distributed automatically from one source to another, ensuring that all relevant parties have access to the most current data.For example, let’s say you have a database of product prices, and you make changes to these prices in one central location. Now, suppose you want to automatically update these new prices across all the places where this data is needed, such as your website, mobile app, and internal sales tools. In this case, data propagation can be a viable solution.Data Integration TechnologiesConsumers have many choices today when it comes to data integration technologies. From basic ETL tools to full-fledged data integration platforms, a solution exists for every business.Explore The 10 Best Data Integration ToolsLearn MoreThe following are the most widely used data integration technologies:ETL Tools: ETL tools extract, transform, and load data into the target system. These are mostly standalone tools that specifically focus on the ETL aspect of data integration.Data Integration Platforms: Data integration platforms are high-end solutions that provide a suite of products to simplify and streamline data integration from end to end.Cloud Data Integration Solutions: These are specialized solutions designed to simplify data integration in cloud-based environments.Change Data Capture Tools: These tools capture and replicate changes in the source data to keep target systems up to date in near real-time.Data Migration Tools: Data migration tools allow you to integrate data by moving data sets from one place to another seamlessly.Data Warehousing Solutions: Not exactly a technology to integrate data, but a technology used for data integration. Data warehouse tools provide the infrastructure and tools necessary to design and build data warehouses used as target systems for data integration.Benefits of Data IntegrationBesides providing a unified view of the entire organization’s data, data integration benefits them in multiple ways.Enhanced Decision-MakingData integration eliminates the need for time-consuming data reconciliation and ensures that everyone within the organization works with consistent, up-to-date information. With information silos out of the way and an SSOT at their disposal, the C-level executives can swiftly analyze trends and identify opportunities. Consequently, they make more informed decisions, that too at a much faster rate.Cost SavingsCost savings are an undeniable benefit of data integration. The initial investment in data integration technologies is outweighed by the long-term savings and increased profitability it leads to. Data integration streamlines processes, reducing duplication of efforts and errors caused by disparate data sources. This way, your organization will be better positioned to allocate and use its resources efficiently, resulting in lower operational expenses.For example, a retail company not only gains real-time visibility into its inventory by integrating its sales data into a single database but also reduces inventory carrying costs.Better Data QualityThe fact that data goes through rigorous cleansing steps, such as profiling and validation, applying data quality rules, fixing missing values, etc., means you can make critical business decisions with higher levels of confidence.Improved Operational EfficiencyWith disparate data sources merged into a single coherent system, tasks that once required hours of manual labor can now be automated. This not only saves time but also reduces the risk of errors that otherwise bottleneck the data pipeline. As a result, your team can focus on more strategic endeavors while data integration streamlines routine processes.Enhanced Data SecurityIt is much easier to secure data that’s consolidated in one place compared to safeguarding several storage locations. Therefore, security is another aspect greatly benefits organizations. Modern data integration software enable you to secure company-wide data in various ways, such as applying access controls, using advanced encryption and authentication methods, etc.Data Integration ChallengesBefore proceeding, let’s take a moment to realize that combining several data sources in itself is a significant challenge. Here are the challenges you can expect to encounter:Overcome All Your Data Integration Challenges With LIKE.TG's 100% No-Code SolutionLearn MoreRising Data VolumeThe data sources keep changing—more pop up every now and then— and the volume keeps rising. Just as data integration is a continuous process, ensuring that your systems can handle increased loads and new data sources is also an ongoing challenge. The sheer volume of data you may need to integrate can strain your organization’s infrastructure and resources if it lacks a scalable solution.CompatibilityDealing with data coming in from various sources and in different formats is the most common issue that teams encounter. Integrating such heterogeneous data requires careful transformation and mapping to ensure that it can work together cohesively. It also involves reconciling disparate data structures and technologies to enable seamless interoperability.Data QualityMaintaining data quality can also be a challenge. You might face issues like missing values, duplicates, or data that basically doesn’t adhere to predefined standards. Cleaning and transforming data to resolve these issues can be time-consuming, especially if done manually. These issues create bottlenecks in the ETL pipeline, potentially impacting downstream applications and reporting.Vendor Lock-InVendor lock-in is when an organization becomes heavily dependent on a single service provider’s technology, products, or services to the extent that switching to an alternative solution becomes challenging and costly. The underlying issue with this challenge is that it’s often too late before organizations realize that they have this problem.MaintenanceMaintaining the data integration pipeline is a significant challenge as it includes the ongoing upkeep and optimization of integrated systems to ensure they function efficiently and deliver accurate and up-to-date information. It’s one of those challenges that don’t get as much limelight as some of the others. Over time, sources may change, new info may become available, and business requirements may evolve. Such circumstances necessitate adjustments to the integration process, hence the importance of maintenance.Data Integration Best PracticesThere’s more to data integration than combining data sources and loading it into a centralized repository—successful data integration requires careful planning and adherence to best practices.Define Clear ObjectivesData integration often involves complex processes, diverse data sources, and significant resource investments. So, before embarking on a data integration project, it’s essential to define clear objectives from the outset. Doing so provides a roadmap and purpose for the entire effort. It also helps in setting expectations and ensuring that the project delivers tangible business value.Select the Right Integration ApproachThere are various methods to choose from, including ETL, API-based integration, and real-time data streaming. Select the approach that best aligns with your organizational objectives and data sources. A financial institution, for example, needs to aggregate data from various branches and systems to detect fraud in real time. In this case, real-time streaming will ensure prompt detection, protecting the institution from financial losses and reputational damage.Take Data Quality SeriouslyYour efforts will only yield the desired results if the integrated data is healthy. It’s a simple case of “garbage in, garbage out.”Implement data quality checks, cleansing, and validation processes to maintain consistency and accuracy.Make it ScalableConsider the scalability and performance requirements of your organization. As data volumes grow, your system architecture should be able to handle increased loads without degradation in performance. Opt for a scalable integration architecture that can handle data growth without performance bottlenecks. This may involve using distributed systems, cloud-based solutions, or data warehousing technologies designed for scalability.Pay Attention to Security and ComplianceImplement robust security measures, encryption, and access controls to ensure data privacy and compliance with relevant regulations, such as GDPR and HIPAA. Ensure that your organization complies with industry and regulatory standards when integrating data.Data Integration Use CasesBusiness Intelligence (BI): Use data integration to bring together information from different sources. This gives you a unified view, making reporting and analytics more efficient. You can then make better, data-driven decisions and gain insights into your business performance.Data Warehousing: Data warehousing means you integrate data from your various operational systems into a centralized data warehouse. This allows for efficient querying and reporting, giving you a comprehensive view of your historical and current data.Customer Relationship Management (CRM): Integrate customer data from different touchpoints, like sales, marketing, and support systems. This helps you improve customer service, personalize interactions, and target your marketing efforts more effectively.E-commerce Integration: Connect and synchronize data between your e-commerce platforms, inventory management systems, and other backend systems. This ensures accurate product information, inventory levels, and streamlined order processing.Supply Chain Management: Integrate data across your supply chain, from procurement and manufacturing to distribution and logistics. This improves visibility into your entire supply chain process, reducing inefficiencies and optimizing inventory levels.Healthcare Integration: Integrate patient data from electronic health records (EHR), laboratory systems, and other healthcare applications. Healthcare data integration enables you to have a comprehensive view of patient information, leading to improved patient care and treatment outcomes.Human Resources (HR) Integration: Integrate HR data from various systems, including payroll, recruitment, and employee management. This ensures accurate and up-to-date employee information, streamlining HR processes and compliance reporting.Mergers and Acquisitions (MA): When your organization undergoes mergers or acquisitions, use data integration to merge information from disparate systems for a smooth transition. This includes combining customer databases, financial systems, and other operational data.Internet of Things (IoT) Integration: Connect and integrate data from your IoT devices to central systems for analysis. This is particularly useful in industries like manufacturing, agriculture, and smart cities, where data from sensors and devices is crucial for decision-making.Take the first step toward seamless data integrationDownload Free TrialStreamline Enterprise Data Integration With LIKE.TGLIKE.TG is an end-to-end data integration solution powered by automation and AI. With LIKE.TG, you can:Handle unstructured data formats seamlesslyClean and prepare data for processingBuild fully automated data pipelinesBuild a custom data warehouseManage the entire API management lifecycleExchange EDI documents with trading partnersLIKE.TG empowers you to do all this and much more without writing a single line of code using its intuitive, drag-and-drop UI. Its vast library of native connectors and built-in transformations further simplify the process for business users.Want to learn more about how LIKE.TG can streamline and accelerate your data integration project? Visitour websiteorcontact usto get in touch with one of our data solutions experts and discuss your use case.
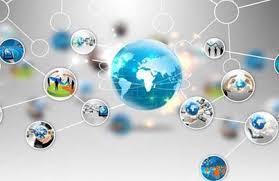
12 Best Data Mapping Tools & Solutions in 2024
Managing data effectively is a multi-layered activity—you must carefully locate it, consolidate it, and clean it to make it usable. One of the first steps in the data management cycle is data mapping. Data mapping is the process of defining how data elements in one system or format correspond to those in another. Data mapping tools have emerged as a powerful solution to help organizations make sense of their data, facilitating data integration, improving data quality, and enhancing decision-making processes. In this blog, we will explore the top data mapping tools in the market and how you should select the right one for your business. What is Data Mapping? Data mapping connects data elements from one data source to another. It defines how data fields in one system correspond to fields in another, allowing smooth data exchange between the two. Data mapping starts with identifying data sources and target systems, like databases or APIs, and analyzing their structures to establish mapping rules for transforming and transferring data. It involves examining data fields in the source system, such as customer ID, and finding commonalities among these fields, for example, identifying that ‘Customer ID’ in the source system corresponds to ‘Client Number’ in the target system. This way, data mapping defines how data from these source fields will be mapped or transformed to match the format and structure of the target system, ensuring seamless data integration between various applications, databases, platforms, etc. Importance of Data Mapping in Data Integration Data mapping facilitates data integration and interoperability. It allows businesses to break down data silos by combining data from multiple sources, such as customer relationship management (CRM) systems, enterprise resource planning (ERP) systems, and third-party data providers, to create a unified view of their operations. This unified view provides organizations with a single source of truth (SSOT), offering a holistic understanding of their business processes to identify areas for improvement and drive operational efficiency. Another benefit of data mapping in data integration is improved data quality management. When data is mapped correctly, it ensures that the integrated data is accurate, complete, and consistent. This helps avoid data duplication, inconsistencies, and discrepancies that can lead to costly errors and operational inefficiencies. With high-quality data, organizations can make more reliable decisions, identify trends, and better understand their business operations. Your complete guide to code-free data mapping Download eBook What are Data Mapping Tools? Data mapping tools are software applications or platforms that facilitate the process of transforming data from one format, structure, or source to another. These tools are used to establish a connection between different data sources, such as databases, files, or APIs, and then define how data should be extracted, transformed, and loaded (ETL) to meet specific requirements. Data mapping tools are commonly used in various industries and scenarios, such as data warehousing, business intelligence, data migration, and application integration, to ensure that data flows easily between different systems and is in the right format for analysis, reporting, or storage. Importance of Having the Right Data Mapping Tool for Your Enterprise While data mapping can be done manually, it becomes a time-consuming and error-prone task as the complexity and volume of data increase. This is where data mapping tools come into the picture. Having the right data mapping tool is crucial for efficient data integration. It simplifies and automates the process, reduces manual effort, and ensures accurate mapping between data sources. Modern data mapping tools provide a visual interface, making it easier for business users to understand and manage the data mapping process. Key Features of An Effective Data Mapping Tool Effective data mapping tools possess certain key features that make them invaluable to businesses. These features ensure that the data mapping process is efficient, accurate, and reliable: Intuitive User Interface: A user-friendly interface ensures data mapping is a straightforward and streamlined process, even for users with limited technical expertise. Business users can easily define mapping rules, transform data, and validate mappings. Data Compatibility: Top data mapping tools support various data formats, including XML, CSV, JSON, and more, allowing you to integrate multiple systems and applications without compatibility issues. Advanced Mapping Capabilities: Data mapping tools offer advanced mapping functionalities, such as conditional mapping, transformation rules, and automated data validation. These capabilities enable businesses to handle complex data mapping scenarios and ensure data accuracy and consistency. Data Governance: Data mapping tools provide features for data governance, including version control and data quality monitoring. These features help businesses maintain data integrity, track data changes, and ensure compliance with data governance policies and regulations. Integration with Existing Systems: Integrating existing systems, databases, and applications is crucial for seamless data mapping implementation. Effective data mapping tools offer integration capabilities that allow businesses to connect and map data between different systems, ensuring smooth data flow and synchronization. Instant Data Mapping Preview: Modern data mapping tools enable you to see how your data will change in real-time in the design stage, ensuring it’s accurate and error-free before proceeding. Top 12 Data Mapping Tools Whether you are a small startup or a large enterprise, finding the right data mapping tool is essential for efficient data management. Here are the top 12 data mapping tools: 1. LIKE.TG LIKE.TG is an enterprise-grade end-to-end data management platform offering organizations various no-code solutions, including AI-powered data extraction, data preparation, integration, EDI, data warehousing, and API management. The tool has an intuitive drag-and-drop user interface that lets you easily map data elements, handle hierarchical data conversion, and define complex transformation rules with just a few clicks. With a built-in data quality module, LIKE.TG ensures that data is accurate and reliable with advanced data profiling, correction, and validation capabilities. Moreover, LIKE.TG’s industrial strength ETL engine allows seamless integration with various data sources and destinations, including databases, cloud platforms, and APIs, making it easier for organizations to extract, integrate, and manipulate data efficiently. Its automation and orchestration features reduce the time needed to manage ETL processes by as much as 80%. Key Features: No-code Visual Interface Rich Data Transformations Industrial strength ETL engine Workflow Automation and Job Scheduling Advanced Data Quality Features Connectors for Multiple On-Premises and Cloud-Based Sources API Integration Create data mappings and integrations in a code-free environment Try It Now! 2. Talend Talend is a cloud-based data integration tool with data mapping capabilities. It provides connectivity to various sources, including multiple file formats and databases. The tool is low-code, which means users will need to write scripts.. Talend also provides features, such as batch processing, for data mapping across bigger data sets. Key Features: Low-code Data Profiling Pre-built Connectors Big Data Compatibility. Data cleansing functionalities before loading data into a warehouse. 3. Informatica Informatica offers a low-code, data management and mapping tool. It allows users to define reusable data flow logic for mapping datasets. The low code functionality of the tool means that users might have to write code for complex transformations. Key Features: Low-code AI-powered Tool. Re-usable Scripts and Dynamic Mapping. Built-in Connectors Workflow Automation 4. Dell Boomi Dell Boomi is a cloud-based data mapping and integration solution. Users can use its point-and-click interface and pre-built connectors to map a source system to a destination. Key features: Low-code tool Workflow automation Intelligent data mapping suggestions Pre-built connectors Custom logic through data map extensions. Field-locking to enforce certain standards. 5. Mulesoft Anypoint MuleSoft Anypoint is a cloud-based integration platform (iPaaS) that provides organizations with a platform for building and managing their APIs and integrations. The tool has data transformation capabilities allowing users to map data between different file formats and protocols. Key Features: Low code tool Real-time Error Handling Advanced Security Multiple transformations Automapping between same-structure schemas. 6. Pentaho Pentaho is a data integration and business analytics platform that enablesenterprises to collect, prepare, blend, and analyze data from various sources. It provides a set of tools for data mapping,ETL, data warehousing, mining, and reporting.Pentaho allowsusers to create and manage complex datamappingsvisually. Key Features: Connectors for databases, flat files, and cloud sources. Re-usable scripts for data mapping Real-time error detection 7. IBM InfoSphere IBM InfoSphere is a data management solution with data mapping capabilities. The tool uses the Mapping Assist functionality for object mapping and workflow creation. Key Features: Self-learning model for improving mapping accuracy with each new task. Intelligent JSON-format transformation suggestions. Compatible with Big data sources. 8. Jitterbit Jitterbit is alow-code data mapping platform as a service that allows businesses to connect their applications and data, automate business processes, and create new digital experiences. It also lets users to create ETL pipelines and perform data migration. Jitterbit is also deployable on the cloud. Here are some of the key data mapping features of Jitterbit: Automapping. Condition-based mapping. Loop node mapping for hierarchical structures. Connectivity with various CRM and accounting systems. Point and click interface for easy mapping and transformations. 9. CloverDX CloverDX is anopen-sourceenterprise data management platform that allows businesses to integrate, transform, and manage their data. It provides a set of tools which includes data mapping, data migration, data warehousing, and data quality management. The key features of CloverDX’s data mapping include: Drag-and-drop interface. Flexibility of creating both code-based and no-code mappings. Expression-based mapping. Free alternative to other options on this list. Parallel processing. 10. Altova MapForce Altova MapForce is a graphical data mapping tool for any-to-any conversion and integration. It supports a wide range of data formats, including XML, JSON, databases, EDI, Excel, XBRL, and Web services. Altova MapForce can be used to build data integration and conversion applications, as well as to automate recurring data transformations. Key data mapping features of Altova MapForce include: Interactive data mapping debugger. A library of built-in data mapping functions. Extensive data source support. Chained data mappings. Data mapping output preview. Automation and job-scheduling. 11. Skyvia Skyvia is a cloud-based data integration platform that allows users to connect to and integrate data frommultiple sources, including cloud applications, relational databases, and CSV files. Skyvia providesvarious data mapping features so users can transform their data into the format required by their target destination. Skyvia’s data mapping features include: No-code visual interface. Advanced mappings, including column and relation mapping. Mapping in synchronization. A mapping editor. 12. Fivetran Fivetran is a fully managed data pipeline platform allowing companies to connect and sync their data from various sources to popular cloud data warehouses and analytics destinations. It automates the data pipeline process, allowing companies to get their data into the cloud and use it to power their businesses. Key data mapping features of Fivetran consist of the following: Pre-built and custom transformations. Cloud deployment. Automated and custom data mapping. Automatic schema migrations. Choosing the Right Data Mapping Tool for Your Needs With several data mapping solutions available, choosing the right one for your business can be challenging. However, you can make an informed decision by considering some key factors. Assessing Your Business’s Data Mapping Needs Start by assessing your business’s specific data mapping requirements. Consider the types of data sources you need to integrate, the complexity of your data transformations, and the level of technical expertise within your team. For example, if your business deals with various data sources, such as databases, APIs, and flat files, you will need a data mapping tool that supports multiple data formats. Furthermore, consider the scalability of the tool. As your business grows, your data mapping needs may evolve. Choose a tool that can accommodate future requirements and handle increasing data volumes. Comparing Costs and Features of Different Tools Compare the costs and features of different data mapping software. Evaluate pricing models, including licensing fees and ongoing maintenance costs. Also consider the long-term costs associated with the tool and any additional expenses for training and support. Additionally, carefully review the features and functionalities offered by each tool and determine which ones align best with your business requirements. Some common features to consider include data validation, data enrichment, and data transformation capabilities. Look for tools that offer intuitive user interfaces and provide comprehensive documentation. Implementing Data Mapping Tools in Your Business Once you have chosen the right data mapping tool for your needs, it’s time to implement it effectively in your business processes. Implementing data mapping tools requires careful planning and execution. Here are some steps to help you successfully integrate a data mapping tool into your organization: Identify Integration Points: Determine the applications and systems that need to be integrated with the data mapping tool. Define Mapping Requirements: Clearly define your data mapping requirements, including data formats, transformation rules, and validation criteria. Configure the Tool: Set up the data mapping tool according to your specific requirements, including mapping connections and transformation rules. Test and Validate: Conduct thorough testing and validation to ensure that the data mapping tool functions correctly and meets your integration goals. Train Users: Provide comprehensive training to your team members to ensure they can use the data mapping tool proficiently. Businesses may encounter certain challenges. Understanding these challenges and taking proactive measures can significantly contribute to accurate data mapping. Complex Data Structures: Dealing with complex data structures can pose challenges during mapping. It is crucial to analyze data structures and plan the mapping accordingly carefully. You can simplify mapping and ensure accurate data transformation by breaking down complex data structures into manageable components. Data Validation: Ensuring data accuracy and integrity is vital. Implementing appropriate data validation mechanisms can help identify and address any inconsistencies or errors in the data. By performing data validation checks at various stages of the mapping process, you can minimize the risk of data corruption and ensure the reliability of your mapped data. Change Management: Implementing a data mapping tool may require changes in existing processes and workflows. Effective change management practices can help smooth the transition and gain user acceptance. By involving key stakeholders early in the implementation process, providing clear communication and training, and addressing any concerns or resistance, you can facilitate a smooth transition and ensure successful adoption of the data mapping tool. Conclusion Data mapping tools are crucial in simplifying data integration and transformation. Organizations can comprehensively view data, enhance its quality, and make informed decisions by accurately linking data elements between systems. LIKE.TG, with its robust features, intuitive interface, and extensive connectivity options, emerges as a top choice for organizations from among the data integration tools listed above. Experience how LIKE.TG’s data mapping tool can make your data integration tasks easier and quicker. Download our 14-day free trial, or sign up for a free demo today! Discover the easier way to perform complex data mappings quickly Get Started
{
"@context": "https://schema.org/",
"@type": "Product",
"name": "LIKE.TG",
"image": "https://www.LIKE.TG.com/wp-content/uploads/2023/07/centerprise-logo.png",
"description": "Kickstart your data integration projects with LIKE.TG Centerprise – an ETL platform to cleanse, transform, and consolidate disparate data.",
"brand": {
"@type": "Brand",
"name": "LIKE.TG"
},
"aggregateRating": {
"@type": "AggregateRating",
"ratingValue": "4.3",
"bestRating": "5",
"worstRating": "",
"ratingCount": "26"
}
}
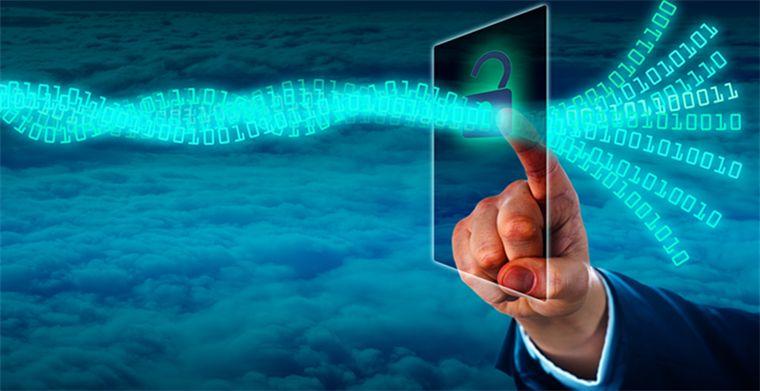
What Is Data Standardization? A Complete Guide
Modern companies heavily rely on data to drive their decision-making processes. However, poor data consistency and quality can lead to inaccurate conclusions. Gartner’s 2018 report highlights that organizations incur an average cost of $15 million annually due to poor data quality. That’s a substantial financial impact that can be avoided through data standardization. What Is Data Standardization? Data Standardization is the process of transforming data from various sources into a consistent format. It includes defining and implementing common data standards and protocols for capturing, storing, and sharing data. The data standardization process sets guidelines for data elements, such as naming conventions, units of measurement, and categorical values, to facilitate the integration and interpretation of data. Types of Data Set Inconsistencies Data set errors can occur for various reasons, impacting the quality and reliability of data. The table below shows some data set inconsistencies that can be resolved by standardizing data. Date Formats Different interpretations of dates (e.g., “01/02/2023” and “1st Feb 23”) Numeric Formats Variation in decimal separators (e.g., “1,000.50” vs. “1000.50”) Units and Measurements Metric vs. Imperial: Incorrect usage of units (e.g., meters vs. feet) Inconsistent Spelling Various spellings for the same category (e.g., “USA” vs. “United States”). Abbreviation Variations Inconsistent abbreviations (e.g., “St.” vs. “Street” vs. “Str.”) Case Sensitivity Misclassification due to case sensitivity (e.g., “apple” vs. “Apple”) Data Types Inappropriate data types for attributes (e.g., treating numbers as strings) Data Format Phone numbers stored inconsistently with and without separators, such as “+1 316-465-3322” and “3164653322”. The Importance of Data Standardization Data standardization enables consistent data exchange across various systems. This means that organizations can ensure that everyone speaks the same data language by standardizing data across various systems, departments, and external partners, giving a holistic view of the company’s operations, customers, and markets. This approach promotes interoperability between different systems and platforms. When data is standardized, it becomes easier to integrate and synchronize information across various software applications and databases. Identifying and correcting errors is also easier, reducing the risk of making decisions based on incorrect or incomplete information. Therefore, data standardization helps improve data quality, enabling accurate analysis, reliable reporting, and informed decision-making. The Data Standardization Process The data standardization process involves several steps that transform data into a harmonized format, enabling accurate analysis and interpretation. 1. Identifying Data Sources The first step in the data standardization process is identifying all the data sources, which may include internal databases, external data providers, and APIs. This allows organizations to gain insights into the data landscape and determine the scope of standardization efforts. They can assess the reliability and accuracy of the data, determine the frequency of new information added to the dataset, and identify the data elements that require standardization, among other factors. 2. Defining Data Standards Defining rules and guidelines for each data element and ensuring the data is consistent, valid, and reliable is also important. These standards may include data formats, allowable values, validation rules, and transformation requirements. Defining clear standards allows organizations to ensure that data is interpreted consistently across different systems and processes. 3. Cleaning Data Data cleaning means identifying and rectifying data errors, inconsistencies, and inaccuracies. This process includes removing duplicate entries, correcting misspellings, and resolving missing or incomplete data. Data cleaning is not a one-time task but an iterative process that requires ongoing monitoring and maintenance to ensure the continued accuracy and quality of the data. 4. Performing Data Transformation The next step is converting the data into a consistent format and structure to ensure that all data can be easily compared and analyzed. This includes tasks such as changing dates into a standardized format or converting units of measurement to a common standard. During the data transformation process, organizations may also need to address issues such as data normalization, where data is scaled or adjusted to eliminate redundancies and improve data integrity. 5. Validating Data The crucial next step is validating data by running tests and checks on the data, such as verifying data integrity, checking for outliers or anomalies, and validating against predefined rules or constraints. Users must promptly rectify any inconsistencies or errors identified during the validation process. It may include revisiting the previous steps in the data standardization process, such as data cleaning or transformation, to ensure the accuracy and reliability of the data. The Traditional Method of Standardizing Using Excel Manual standardization using Excel is one of the most common techniques to standardize data. This traditional method requires extensive computation and application of formulas to validate the data manually. The formula for standardization is: Where: x is a data point. μ is the mean of the dataset. σ is the standard deviation of the dataset. Note: The “Z-test” and “Standardize” functions perform data standardization in Excel. This manual approach to data standardization requires human intervention, attention to detail, and expertise to transform and validate the data. Analysts must carefully review the data to ensure consistency and accuracy, which is why this approach can be time-consuming. While this method is useful for small-scale projects that require quicker analyses for smaller data sets, the manual nature of the process makes it less efficient when dealing with high volumes of data. Self-Service Automated Tools: The Better Alternative Another modern approach to data standardization is using self-service data prep tools that leverage machine learning algorithms and artificial intelligence to clean, transform, and validate data. Automatic data standardization software allows organizations to automate the application of data standards. These tools can identify data elements, apply predefined rules and transformations, and cleanse and transform data automatically. Leveraging these tools helps organizations save time and effort in the data standardization process, ensuring consistent and reliable data. This comparison table highlights the benefits of automated data standardization tools over Excel: Automated Tools Excel Data Volume Efficient for large and complex data sets Suitable for small to moderate data sets Manual Effort Automates data transformation and cleaning Requires manual data manipulation Standardization Offers advanced standardization algorithms Limited built-in standardization functions Scalability Scales well to process large data volumes Not scalable for processing extensive data Time-efficiency Rapid data processing, saving time Takes a considerable amount of time for repetitive tasks Complex Transformations Handles complex transformations with ease Not suitable for complex transformations Error Handling Built-in error detection and reporting Limited error detection and handling Version Control Offers version control for data processes Lacks proper version control mechanisms Consistency Provides consistent results every time Difficult to ensure consistent results repeatedly Using LIKE.TG for Automated Data Standardization LIKE.TG’s unified data management platform has automated data standardization capabilities involving rigorous and agile data cleansing, transformation, and validation features. The point-and-click interface makes it easy to quickly rectify incomplete or inaccurate data, ensuring data accuracy and consistency. LIKE.TG also offers a dynamic grid view that allows users to explore, visualize, interact, and analyze data in real-time, providing instant feedback on data quality. Here is a step-by-step guide on how users can utilize LIKE.TG for their data standardization use cases: 1. Read the .csv file in the LIKE.TG Data Prep Artifact. 2. View overall data health on the right side of the window. 3. Select the column to view its profile on the right side. The “Country” column has been selected. From the profile browser on the right, we can see that this column has inconsistent capitalizations: “Germany”, “Germany”, and “GERMANY”. 4. For this use case, click on the “Change Case” function to change the case of the values in the “Country” column to make the values consistent. 5. Apply Change Case transformation with case type = “Title” as standard. 6. After applying the transformation, all the inconsistent values have been standardized in the “Country” column. Unlock the true potential of data for a more efficient data ecosystem and accurate insights. Contact LIKE.TG Today or sign up for a free 14-day.

Top 5 Data Preparation Tools In 2024
Data analysis demands precision, and at its core lies the vital role of data preparation tools. These tools serve as the cornerstone for ensuring your data is accurate, consistent, and reliable. Before embarking on your data analysis journey, it’s crucial to choose the right tool for the job. This guide will introduce you to the top five data preparation tools currently shaping the market. What Are Data Preparation Tools? Data preparation tools are software or platforms that automate and streamline the entire data preparation process. These user-friendly tools collect, clean, transform, and organize raw and incomplete data into a suitable and consistent format for further data processing, modeling, and analysis tasks. Data Preparation tools help users clean and transform large volumes of data faster and more efficiently than manual processes. Key Features of a Good Data Preparation Tool Here are some essential features of good data preparation software: Connectors for Diverse Data Sources A quality data preparation tool connects to in-demand relational databases such as Azure, Oracle, Redshift, and SQL Server. It should also have connectors for various CRM systems, CSV/JSON files, and multi-structured sources such as log files, PDFs, images, texts, etc. Built-in connectivity for these sources allows for easier data extraction and integration, as users will be able to retrieve complex data with only a few clicks. Data Security Data security and privacy checks protect sensitive data from unauthorized access, theft, or manipulation. Despite intensive regulations, data breaches continue to result in significant financial losses for organizations every year. According to IBM research, in 2022, organizations lost an average of $4.35 million as a result of data breaches. This was up 2.6% from the previous year. Data security is necessary to keep this number down. Most data preparation tools allow for access control. With access controls defined, only authorized users can access sensitive data. Additionally, access can be customized based on the user’s role or level of access needed. By limiting access to sensitive data pipelines or architectures, preparation tools can enhance accuracy by reducing the risk of errors and ensure compliance with data protection regulations. End-to-End Process Automation One of the main reasons organizations turn to data preparation solutions is to automate all the manual data preparation tasks and processes. Businesses significantly improve efficiency and productivity by automating data integration, cleaning, standardization, transformation, and storage tasks. Preparing reliable data can normally take weeks or months; however, automation can reduce this cycle to just a few hours or days. Easy-to-Use, Code-Free Environment By eliminating the need for writing complex code, data preparation tools reduce the risk of errors. These tools allow users to manipulate and transform data without the potential pitfalls of manual coding. This improves data quality and saves valuable time and resources that would otherwise be devoted to error detection and correction. Interoperability Once you’ve accessed, cleansed, and organized your data, the next crucial step is to utilize it within your analytics infrastructure effectively. While all data transformation solutions can generate flat files in CSV or similar formats, the most efficient data prep implementations will also easily integrate with your other productivity business intelligence (BI) tools. Manual export and import steps in a system can add complexity to your data pipeline. When evaluating data preparation tools, look for solutions that easily connect data visualization and BI reporting applications to guide your decision-making processes, e.g., PowerBI, Tableau, etc. Flexibility and Adaptability Flexibility is the tool’s ability to work with various data sources, formats, and platforms without compromising performance or quality. An agile tool that can easily adopt various data architecture types and integrate with different providers will increase the efficiency of data workflows and ensure that data-driven insights can be derived from all relevant sources. Adaptability is another important requirement. As businesses grow and evolve, so do their data requirements. This means that a data preparation automation tool should be capable of scaling and adapting to the organization’s changing needs. It should be able to adjust to new technologies, handle increasing data volumes, and accommodate new business goals. Top 5 Data Preparation Tools for 2024 1. LIKE.TG LIKE.TG is a unified data management platform with advanced data preparation, extraction, integration, warehousing, electronic data exchange, and API management capabilities. The platform’s easy-to-use visual interface allows you to design and develop end-to-end data pipelines without coding. LIKE.TG’s dynamic platform includes rigorous data cleaning, transformation, and preparation features. The solution lets you connect to various data sources, including databases, files, and APIs, to access raw data easily. With its preview-focused interface, you can perform various data-cleaning activities, such as removing duplicates, handling missing values, and correcting inconsistencies. LIKE.TG supports advanced transformations such as filtering, sorting, joining, and aggregating to restructure and improve the data quality. The integrity and quality of the prepared data can be verified using custom validation rules, data profiling, and verification checks to ensure reliability and consistency. Once satisfied, easily export the organized data to various formats or integrate it with downstream systems for analysis, visualization, or consumption with just a few clicks. Key Features: Point-and-Click Navigation/ No-Code Interface Interactive Data Grid with Agile Correction Capabilities Real-Time Data Health Checks Effortless Integration of cleaned data with external systems Workflow Automation Data Quality Assurance with Comprehensive Checks and Rules Rich Data Transformations Connectors for a wide range of on-premises and cloud-based sources AI-powered Data Extraction 2. Altair Monarch Altair Monarch is a self-service tool that supports desktop and server-based data preparation capabilities. The tool can clean and prepare data from a wide range of data sources and formals, including spreadsheets, PDFs, and big data repositories. Altair Monarch has a no-code interface to clean, transform, and prepare data. It supports data source access, profiling and classification, metadata management, and data joining. Key Features: No-code, visual interface Workflow automation Pre-built data transformation features Reusable custom models 3. Alteryx Alteryx data preparation tool offers a visual interface with hundreds of no/low-code features to perform various data preparation tasks. The tool allows users to easily connect to various sources, including data warehouses, cloud applications, and spreadsheets. Alteryx can conduct a predictive, statistical, and spatial analysis of the retrieved data. The tool also lets users visually explore data through data exploration and profiling. Alteryx is available both as a cloud-based solution and on-premise. Key Features: AI-infused data quality enhancement recommendations Data Exploration Profiling Data connectors for on-premises and cloud User-friendly interface 4. Talend Talend’s data prep module is a self-service data preparation application that uses machine learning algorithms for standardization, cleansing, and reconciliation activities. The tool’s browser-based interface and machine learning-enabled data prep features let users clean and prepare data. Talend connects to various data sources such as databases, CRM systems, FTP servers, and files, enabling data consolidation. Key Features: Workflow Automation No-Code self-service interface Role-based access for data security and governance Real-time data quality monitoring 5. Datameer Datameer is a SaaS platform designed for data preparation within the Snowflake environment. The tool gives an option to prepare data using SQL code or through the drag-and-drop Excel-like interface to ingest and prepare data. Datameer uses a graphical formula builder for data transformations, profiling, etc. The tools allow for integrations with BI tools for further analysis. Key Features: No-code or SQL-code Snowflake centered Excel-like Interface Runtime validation Support for all data formats (structured, semi-structured, and unstructured) Data Profiling and Transformations Workflow Automation How to Choose the Right Data Preparation Tool for Your Needs Choosing the right data preparation tool is an important task. There are some key factors you must keep in mind to find a solution that fits your data requirements. Consider the complexity of your data and the level of technical expertise available within your organization. Some tools are more suitable for technical users, while others focus on simplicity and ease of use for non-technical users. Additionally, evaluate the performance and scalability of the tool, as well as its compatibility with your existing infrastructure. Evaluate the volume and variety of your data and the frequency of data updates. Consider whether you require real-time data integration, advanced data profiling capabilities, or specific data transformation functions. Emerging Trends in Data Preparation The rise of big data and the increasing complexity of data sources have led to the development of intelligent data preparation tools. These tools leverage AI and machine learning algorithms to automate data cleansing and transformation tasks, making the data preparation process more efficient and accurate. Additionally, data preparation tools are becoming more integrated with other data analytics technologies, such as data visualization and predictive analytics, enabling organizations to derive more value from their data. Advancements in technology, such as cloud computing and distributed processing, are also revolutionizing the data preparation process. Integrating data preparation tools with data lakes and warehouses enables organizations to leverage the power of distributed processing, making data preparation faster and more efficient than ever before. Streamline Your Data Preparation with Self Service Tools Data preparation is a critical step in the data analysis process. With the right data preparation tool, you can ensure data quality, consistency, and accuracy, leading to more reliable insights and informed decision-making. By considering the key features and evaluating your specific needs, you can choose a data preparation tool that suits your requirements. As technology advances, the future of data preparation looks promising, with intelligent tools and seamless integration shaping how we prepare and analyze data. LIKE.TG is a powerful and AI-powered platform that enables self-service data preparation for users with varying technical expertise. You can automate repetitive tasks, such as data cleansing, transformation, and enrichment, reducing manual effort and saving time. With advanced data preparation capabilities, LIKE.TG is invaluable in any data-driven operation. It bridges the gap between data and analysis, accelerating business time-to-insights. Experience how LIKE.TG can make your data preparation tasks easier and quicker. Sign up for our 14-day free trial or a free demo today!
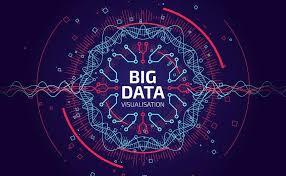
A Comprehensive Guide to Data Cleaning
The more data we generate, the more cleaning we must do. But what makes cleaning data so essential? Gartner reveals that poor data quality costs businesses $12.9 million annually for businesses. Data cleansing is critical for any organization that relies on accurate data. The monetary cost is just one problem—poor-quality data also costs organizations and data scientists considerable time to fix. A recent survey revealed that data scientists spend as much as 60% of their time cleaning and organizing data. What is Data Cleansing? Source: Allied Infoline Data cleansing, also known as data cleaning or data scrubbing, is the process of detecting and correcting (or removing) any errors or inconsistencies in data. As part of the overall data quality management framework, its primary goal is to improve data health, ensuring that information is accurate, consistent, and usable. But why can’t we use raw data instead of spending so much time on data cleansing? Misspelled Entries: Typos and spelling mistakes can lead to categorization errors. Inconsistent Formats: Dates, numbers, or categories might be represented differently within the same dataset. Outliers and Errors: Unusual or erroneous entries can lead to inaccurate analysis. Duplicate Records: Redundant data can lead to inaccurate statistics and conclusions. Null or Missing Values: Incomplete data may lead to gaps in analysis and can lead to inaccurate and/or limited insights. Inaccurate Data: Incorrect or outdated information can lead to inaccurate decisions. Unstandardized Units: Different units of measurement can create data inconsistency issues, particularly when comparing or aggregating data. Incompatible Data: Conflicting data from different sources can cause discrepancies in data integration and analysis. Data Cleansing Techniques Cleaning the data addresses these challenges by using various techniques. Removing Duplicates: How to Do It: Utilize algorithms to identify and remove duplicate rows based on selected vital attributes. Examples Considerations: In a sales database, duplicates can arise from multiple entries for the same transaction. The data cleansing process involves merging or removing such duplicates to ensure accurate sales reporting. Handling Missing Data: How to Do It: Options include imputation, deletion, or using algorithms that can handle missing values. Imputation might use mean, median, or model-based strategies like k-NN. Examples Considerations: In a healthcare dataset, missing patient data, such as blood pressure readings, may be imputed using statistical methods to maintain data completeness and integrity. Correcting Incorrect Data: How to Do It: Utilize data validation rules, consistency checks, and manual review if needed. Data preparation tools can help in pattern matching and corrections. Examples Considerations: For instance, in a retail dataset, ensuring that all occurrences of “Los Angeles” are consistently spelled the same way, rather than appearing as “L.A.” or “LA,” ensures accurate location-based analysis and reporting. Handling Outliers: How to Do It: Identify outliers through statistical methods like the Z-score or IQR, then decide whether to cap, transform, or remove them. Examples Considerations: In financial data, an unusually high transaction amount may indicate fraud. Deciding how to handle such outliers is crucial for fraud detection and risk management. Normalizing Data: How to Do It: Apply techniques like Min-Max scaling, Z-score normalization, or log transformations. Examples Considerations: In a dataset with variables like temperature and humidity, normalization ensures that these variables are on a consistent scale, facilitating accurate weather prediction models. Validating Data Consistency: How to Do It: Create validation rules to check relationships and consistency across attributes. Examples Considerations: In an inventory database, validating that the total stock value matches the sum of individual item values is essential for inventory accuracy. Transforming Data: How to Do It: Use data transformations like encoding for categorical data or creating interaction terms based on analytical needs. Examples Considerations: In a recommendation system, one-hot encoding is applied to product categories to convert them into a format suitable for machine learning algorithms, improving the accuracy of product recommendations. Benefits of Data Cleansing Data cleansing is an essential component of the data processing pipeline. Rather than being a standalone task, it works in conjunction with other preprocessing techniques to prepare data for analysis. Normalization, transformation, and error correction are all integral to the data cleansing process. Benefits of data cleaning include: Enhanced Accuracy: Clean data leads to precise insights. Data cleansing establishes a solid foundation for accurate analysis and decision-making by removing errors and standardizing formats. Efficiency: Properly cleansed data streamlines the analysis process, minimizing the time spent on error detection and correction during the analytical stages. Revenue Growth: Clean data refines marketing strategies by offering insights into customer behavior, allowing for targeted campaigns. This accuracy in profiling helps tap into new opportunities and optimize offerings, boosting efficiency and driving revenue growth. How to Choose the Right Data Cleansing Tool Understanding how to clean your data is only useful if you can apply these techniques effectively. Choosing the right tools can make this process seamless. It’s important to choose products that are aligned with your specific business needs. Let’s delve into how you can select the right data cleansing tool for your business: Compatibility and Integration It is important to select a data cleansing tool which is compatible with your existing systems and data formats. If, for example, your data is stored in SQL databases, the selected tool should be able to connect to and manipulate this data directly. Consider support for a wide array of data sources like SQL, NoSQL, and Excel, and the tool’s ease of connecting with other analytical platforms. Ease of Use and Learning Curve Finding a tool that is accessible to both beginners and experienced professionals is vital. Look for tools with intuitive interfaces that are suitable for users with varied experience levels. The availability of tutorials, documentation, and community support can ease the learning process, making the transition smooth for everyone involved. Scalability and Performance A tool’s ability to handle large datasets and scale with growing data needs is crucial, especially for organizations with large volumes of data. Solutions that can manage large-scale operations efficiently might be the perfect fit. Assessing the speed and responsiveness of various cleansing operations helps ensure the tool performs well under pressure. Flexibility and Customization It’s crucial to choose a data cleansing tool that can be customized to handle your unique or specialized data cleaning needs. You want a tool that allows you to create or modify custom rules if you deal with intricate data, such as specific financial formats or custom transaction categories. This adaptability ensures that you can tailor the data cleansing process to the unique aspects of your data, regardless of its complexity or the specific demands of your industry. Data Quality Assurance Continuous monitoring and data quality validation are essential, and robust features for visualization and reporting should assist in this task. Automation capabilities, data quality metric visualizations, and ongoing support should all factor into your decision. Security Ensuring that the tool adheres to relevant data protection standards is essential. When working with sensitive data, tools that provide encryption and follow industry-standard security protocols are crucial. A careful evaluation of the tool’s security features and compliance with industry standards can help you make an informed choice. Cost and Support Analyzing the pricing model, including subscription fees and any hidden costs, will help align the tool with your budget. Open-source options may be suitable for smaller budgets, while enterprise-grade solutions offer extensive support at a premium. Evaluating the quality of customer support, weighing free vs. paid options, and considering the overall value for investment are essential steps in finding the right tool for your organization. Learn more about the best data cleaning tools. Now that you have an understanding of what data cleansing entails and its significance in modern data-driven businesses, the next step is choosing the right tool. LIKE.TG has been rapidly gaining attention in various industries for its ease of use and robust data cleansing functionalities. Let’s explore what makes this tool a preferred choice for many organizations. LIKE.TG: Your Gateway to Seamless Data Preparation LIKE.TG is an intuitive and user-friendly data management platform that enables users to seamlessly extract, cleanse, and prepare the data. Its key features include: AI-Powered Data Extraction: Extract unstructured data in a few clicks. Interactive Data Profiling: Gain insights into your data visually. Automated Data Quality Checks: Implement standard quality rules. Data Transformation: Design transformations effortlessly. Integration with Various Sources: Connect with different data formats. Built-in Scheduling and Automation: Enhance efficiency with automated workflows. LIKE.TG ReportMiner stands out for its scalability, quality assurance, and intuitive interface. It’s suitable for various industries, including retail, healthcare, and finance, and offers a cost-effective solution without compromising performance or security. LIKE.TG represents a strategic investment that can revolutionize how your organization handles data, enabling you to focus more on deriving insights and less on managing the intricacies of data cleaning. Unlock the power of clean data today! Your Data Preparation Journey Begins with a Click Gain in-depth knowledge and practical insights into data quality management with our comprehensive eBook. Download Now
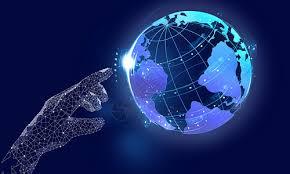
The 7 Best Data Migration Tools In 2024
Moving data from point A to point B can be time-consuming and complex. Let’s take a trip down memory lane. You’ve just purchased a new phone and can’t wait to use it. But wait! Without the data transfer software, you may end up spending hours manually moving contacts, photos, and messages from your old phone to the new one, turning your excitement into frustration. You can think of enterprise data migration as transferring data to a new smartphone, but on a much larger scale. Enterprises transfer vast amounts of important business data during migrations—data that’s scattered across disparate systems—and they cannot afford downtime or data loss. That’s why they opt for data migration tools that automate the process and ensure that complete, high-quality data reaches the target destination. In this blog, we’ll explore the top 7 data migration tools in the market and cover the essential factors for selecting the right solution for your business. Let’s dive in! TL; DR? Here’s the list of the best data migration tools to consider in 2024: LIKE.TG AWS Database Migration Service Fivetran Integrate.io Matillion Stitch Hevo Data What is Data Migration? Data migration is the process of transferring data from one location, format, or application to another. It is a complex process that requires careful planning and execution to minimize risks and ensure a successful transition. The primary goal of data migration is to ensure that data remains accessible, accurate, and secure throughout the process. The process commences with a critical phase of assessment and planning. During this stage, the data to be migrated is identified and thoroughly analyzed. Once the assessment is complete, data is extracted from the source systems using tools, scripts, or APIs. Post-extraction, data is transformed, particularly if there are disparities in data format or structure between the source and target systems. The data is then loaded into the target system. When it comes to the actual migration, it’s necessary to schedule a time for the process, which can involve planned downtime or reduced system activity to minimize data inconsistencies and disruptions. Finally, the old source system is safely decommissioned after confirming the successful migration. Streamline Fortify Your Data Migration Processes with LIKE.TG Seamlessly and securely move your valuable data with LIKE.TG. Simplify your migration process and unlock the power of automated data movement today! Download Your Free 14-day Trial! Importance of Data Migration Businesses usually take up data migration when they have to move data between storage systems, upgrade to a new software platform, or transition to a cloud-based infrastructure. Research from the Flexera 2022 State of the Cloud Report indicates that 57% of organizations are actively migrating more workloads to the cloud, while 42% are shifting from on-premise systems to Software as a Service (SaaS) applications. Here are some more reasons why businesses migrate data: Upgrading Technology: As technology evolves, enterprises often need to update their software, hardware, or infrastructure. Data migration allows them to seamlessly transition to newer systems while preserving critical data and minimizing disruption. Data Consolidation: Over time, data can become scattered across various systems and locations. Data migration enables businesses to consolidate data, making it easier to manage, access, and analyze. Compliance and Security: Organizations must comply with data protection regulations and ensure data security. Data migration allows them to meet compliance requirements and safeguard sensitive information during transfer. Business Expansion and Mergers: When businesses expand or merge with others, they must integrate data from multiple sources or locations. Data migration facilitates this process, enabling a smooth transition of data and systems. Types of Data Migration Tools Having explored their significance, it is important to have an in-depth understanding of the various types of tools that are available. The three primary categories of data migration tools are: 1. On-Premise Data Migration Tools On-premise data migration tools are designed for deployment within an organization’s own physical infrastructure or data centers and facilitate the transfer of data between on-premises servers or systems. These tools are typically installed and operated on the organization’s hardware and so provide a high level of control over data security and compliance. On-premise data migration tools are well-suited for scenarios where a business needs to move data between in-house servers, databases, or applications. They are an ideal solution for dealing with sensitive data or in highly regulated industries where maintaining complete control over data security and compliance is crucial. Organizations usually employ these tools for tasks such as server upgrades, data center consolidations, or database migrations within their infrastructure. Prominent examples of these tools include Microsoft SQL Server Integration Services (SSIS), Oracle Data Integrator, and open-source solutions like Apache Nifi. 2. Cloud Data Migration Tools Cloud data migration tools are specialized solutions designed to seamlessly transfer data to and from cloud-based services and platforms. These tools are typically offered as cloud-based services themselves and are tailored for data migration to cloud providers like AWS, Azure, or Google Cloud. These tools are particularly valuable for businesses embracing cloud infrastructure and services. Cloud data migration tools usually offer data synchronization, transformation, and validation features, which makes them ideal for tasks like migrating on-premises applications to the cloud, replicating data for disaster recovery, or consolidating data from multiple cloud sources. Examples of these tools include AWS DataSync, Azure Data Factory, Google Cloud Data Transfer Service, and third-party solutions like Informatica Cloud and Talend Cloud. 3. Self-Scripted Data Migration Tools Self-scripted data migration tools are custom-built solutions tailored to meet an organization’s unique data migration needs. These tools are highly customizable and often developed in-house or by external experts with programming expertise. They are usually created with programming languages like Python, Java, or PowerShell. Businesses opt for self-scripted data migration tools when they have specific data migration requirements that off-the-shelf solutions cannot easily address. They are suited for scenarios where fine-grained control over data extraction, transformation, and loading processes is essential. Some of the use cases where self-scripted data migration tools work the best include,migrating legacy systems, integrating disparate data sources, or handling specialized data formats. Examples of these tools include custom Python scripts for ETL processes, Java-based data migration frameworks, or PowerShell scripts for automating data transfers between systems. Top 7 Data Migration Tools For 2024 Let’s look at the top 7 data migration tools available in the market and their features: LIKE.TG AWS Database Migration Service (DMS) Fivetran Integrate.io Matillion Stitch Hevo Data 1. LIKE.TG LIKE.TG is an enterprise-grade, end-to-end data management solution that streamlines data integration, enabling businesses to move data from various sources to destination systems like databases, data warehouses, and cloud-based applications. It is a versatile solution that can be deployed on-premises, in the cloud, or both, providing a smooth data migration experience. Its visual data mapping interface simplifies the process of defining transformations, ensuring that data is accurately transferred from the source to the target system. Additionally, LIKE.TG’s platform supports complex data transformations, so you can easily cleanse, validate, and standardize data during the migration process. These data quality and transformation features ensures that the migrated data is in the right format and meets the business rules of the target system. Key Features: Built-in connectors: LIKE.TG offers native connectivity to various popular databases, data warehouses, and cloud providers, including Snowflake, Amazon RedShift, Netezza, SQL Server, Amazon S3, Microsoft Blob Storage, and more. User-Friendly Interface: LIKE.TG’s drag-and-drop, visual, and intuitive interface, along with point-and-click navigation, simplifies data management tasks, eliminating the need for coding. Hierarchical Data Handling: LIKE.TG offers rich hierarchical data mapping capabilities, making it ideal for migrating hierarchical structures like XML, EDI, and web services. Easy Configuration: The platform offers standardized configurations for cloud storage connectors, allowing business users to connect to cloud applications effortlessly. Data Quality: LIKE.TG ensures data quality through profiling, cleansing, and data quality rules, guaranteeing the transfer of only high-quality and reliable data. Automation: LIKE.TG streamlines data migration with automated data pipelines and job scheduling, reducing repetitive manual tasks and enhancing efficiency. 2. AWS Database Migration Service (DMS) AWS Database Migration Service (DMS) is a fully managed service that makes it easy to migrate databases to AWS. It supports a wide range of source and target databases, including Oracle, SQL Server, PostgreSQL, MySQL, MongoDB, and Amazon Redshift. You can use DMS to migrate data incrementally or in full load mode. It also supports continuous data replication to keep data in sync between two databases. Key Features: Schema conversion: DMS can automatically convert database schemas to a format compatible with the target database. Data transformation: The solution can transform the data as it is migrated. This feature can be useful for cleaning up the data or making it conform to a specific format. Monitoring and reporting: DMS provides detailed monitoring and reporting capabilities so users can track the progress of their migration and timely identify errors. 3. Fivetran A cloud-based ELT platform that automates the process of moving data from SaaS applications to data warehouses. It has pre-built connectors for popular services such as Salesforce, Amazon Redshift, Google Analytics, MongoDB, among others. These connectors streamline the extraction of data from the source systems and facilitate its migration into the target data warehouses. Key Features: Automated data pipelines: Fivetran enables users to automatically create and manage data pipelines to move the data into their data warehouse. Incremental data syncs: The solution syncs the data incrementally, which means that only new or changed data is transferred to your data warehouse. Schema drift handling: Fivetran automatically detects and handles schema drift when the structure of your data sources changes over time. Security and compliance: The tool offers security and compliance features, including data encryption, role-based access control, and audit logs. 4. Integrate.io Integrate.io is a cloud-based data integration tool that provides businesses with a suite of tools to connect, transform, and load data from a variety of sources into a single data warehouse. The platform supports ETL, ELT, and r processes, along with data replication and change data capture (CDC), facilitating data migration and enabling access to real-time insights. Key Features: Visual workflow builder: Integrate.io uses a visual workflow builder to make it easy to create and manage data pipelines. Users can drag and drop connectors to create a workflow that moves their data from one source to another without writing code. Pre-built connectors: The platform allows users to connect to various data sources, including SaaS applications, databases, cloud storage platforms, and APIs. Data transformation: Integrate.io users to perform data transformations on your data, such as filtering, joining, aggregating, and converting data types. Scheduling and automation: Integrate.io allows users to schedule their data pipelines to run on a regular basis to automate the data migration and integration process. 5. Mattilion Matillion provides a cloud-native solution for data movement and transformation. It seamlessly integrates with popular cloud data platforms and supports reverse ETL, bidirectional data flows, and system synchronization. It allows users to move and transfer the data irrespective of its location. Key Features: Pre-built Connectors: Matillion provides access to a wide range of connectors and supports connections to other databases through JDBC drivers. Batch Loading: The drag-and-drop interface allows users to automate data pipelines and manage schema changes by setting the batch-run frequency to ingest data. Change Data Capture (CDC): Matillion enables the real-time replication of database changes using log-based data capture to track the changes as they occur. Automation: The platform allows users to automate and schedule jobs based on custom parameters, eliminating manual intervention. 6. Stitch Stitch is a cloud-first, open-source solution designed to move data from source to warehouse. It is recognized for its ETL capabilities, enabling users to extract, transform, and load data into target applications. The platform offers data replication, orchestration, and error-handling capabilities, providing visibility into the process. Key Features: Data connectors: Stitch offers connectivity to a wide range of sources, including SaaS applications, databases, cloud storage platforms, and APIs. Data replication: Stitch can replicate data from your source systems to your data warehouse in real-time or on a scheduled basis, ensuring that the data warehouse is always up to date. Data quality: The platform offers capabilities for data quality management, such as validation and cleansing. This helps to ensure that your data is accurate and complete. Security and compliance: Stitch provides security and compliance features to protect the data. This includes data encryption, masking, role-based access control, and audit trails. 7. Hevo Data Hevo Data is a zero-maintenance, no-code, cloud-based data pipeline platform. It is built for modern ETL, ELT, and Reverse ETL needs, allowing users to migrate data from multiple sources to data warehouses like Redshift, BigQuery, and Snowflake in real time. It enables data teams to consolidate data from various sources, execute transformations for analytics, and offer operational intelligence to business tools. Key Features: Data Connectors: Hevo offers a range of plug-and-play integrations for various applications and databases, including CDC support and custom connectivity to any application using Rest API. Codeless Data Pipeline: Users can set up their databases and data warehouses for analytics without relying on IT teams using Hevo’s codeless data pipeline. Data Security and Compliance: Hevo Data prioritizes data privacy and security, complying with standards such as HIPAA, GDPR, CCPA, and SOC2 to ensure user data remains confidential and regulatory requirements are met. How the Right Data Migration Tool Benefits Enterprises One way to go about data migration is to do it manually. However, as we all know, it is time-consuming and prone to errors. Data migration is no easy feat, which is why it is important to use a data migration tool. Here is how a data migration tool can make the process easier: Efficiency and Speed: A suitable data migration tool automates and streamlines the migration process and reduces the time and effort required to move data. Data Accuracy: Data migration tools include data validation and transformation features, ensuring that data is migrated accurately. Robust data quality features reduce the risk of errors, data corruption, or data loss during the migration process. Competitive Advantage: Leveraging an efficient data migration tool allows enterprises to quickly adapt to changing market needs. These tools enable seamless transition to new systems, platforms, or cloud environments and allow businesses to outpace competitors, respond swiftly to market shifts, and enhance customer offerings. How To Select the Right Data Migration Tool for Your Organization? There are several factors that an organization must consider while selecting the right data migration tool. Some of them include: Scalability Make sure that the tool chosen can scale to handle large data volumes effectively. It should be able to grow alongside your organization’s needs. Reliability Consider the reliability of the data migration tool to maintain data integrity and minimize downtime during migration projects. It should offer robust error handling and recovery features to ensure a smooth migration process. Compatibility and Adaptability Opt for a tool that seamlessly integrates with your existing systems and adapts to different data formats and sources. This flexibility is crucial when dealing with diverse data sources and technology stacks. User-friendly Interface Look for a tool with an intuitive design and clear usability that can significantly enhance the efficiency of your migration process, reduce the risk of errors, and ensure smoother data transfers. Prioritize tools that are easy to navigate and require minimal training for your team. Regulatory Compliance Confirm that the chosen tool adheres to relevant industry standards and data protection regulations such as GDPR, HIPAA, etc. Customization and Extensibility Ensure that the tool empowers customization and extensibility to accommodate unique data migration scenarios. Conclusion Data migration is critical for organizations looking to modernize their systems, improve data management, and stay competitive. Selecting the right data migration tool is crucial, as it should align with an organization’s specific needs and objectives. LIKE.TG Centerprise offers an intuitive user interface, automation capabilities, and connectivity to diverse data sources. The platform enables efficient data transfer, empowering you to unlock the true value of your data assets. Don’t let data migration activities hold you back. Download LIKE.TG’s 14-day free trial today to experience hassle-free data transfer.
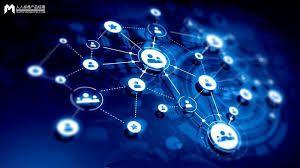
Self-Service Data Preparation: The Pathway to Business Growth
The pursuit of accelerated business growth hinges on data-driven strategies. McKinsey suggests that companies who use consumer behavior insights strategically perform 85% better in sales growth compared to their peers. But, the traditional manual data preparation process, which often involves extensive data cleaning, formatting, and integration, can consume valuable time and resources, impeding the pace of progress. Self-service data preparation emerges as a solution, offering organizations the means to streamline data handling and drive success. What is Self-Service Data Preparation? Before delving into self-service data preparation, let’s learn about data preparation. It’s the process of collecting, cleaning, transforming, and organizing raw data into a format that can be easily analyzed and used for decision-making. It’s a crucial stage in the data lifecycle that bridges the gap between raw, unstructured data and actionable insights. Data preparation is traditionally handled by data scientists and IT professionals, but businesses have recognized that this approach is not the most efficient. Enter self-service data preparation. This approach is about putting the power of data straight into the hands of those who need it most: business users, analysts, managers, and others who might not have technical expertise in data handling. The idea is to enable these users to perform data preparation tasks themselves without constant reliance on specialized IT teams. This approach recognizes that the insights derived from data are essential across all areas of an organization; it aims to make data preparation accessible to all. Benefits of Self-Service Data Preparation Increased Speed and Efficiency Self-service data preparation plays a vital role in accelerating data processing, allowing immediate access to vital information. Unlike traditional methods that could take days or even weeks due to lengthy approval processes and reliance on IT departments, self-service tools enable employees to pull, clean, and analyze data on the fly. This swift turnaround means businesses can make real-time decisions that accurately align with market dynamics. Such agility not only keeps a company in step with trends and market shifts, but also allows companies to capitalize on new opportunities, directly contributing to growth. Democratization of Data By making data accessible to all staff, not just technical experts, a more extensive portion of the organization can engage with this critical resource. It fosters cross-department collaboration, leading to more cohesive and innovative strategies. This inclusive approach means a more informed and aligned workforce, driving collective efforts towards shared goals. In this way, data democratization enhances the organizational culture of a company and fosters growth by tapping into diverse insights and ideas. Cost Savings Economic efficiency often drives growth, and here, too, self-service data preparation shines. By reducing the need for specialized staff and enabling more efficient resource utilization, businesses can see substantial cost savings. These savings aren’t merely about improving the bottom line; they represent funds that can be reinvested in core growth areas. Whether it’s expanding marketing efforts, funding research and development, or entering new markets, the financial flexibility provided by self-service data preparation can be vital fuel for expansion. Enhanced Data Quality and Compliance Automated cleaning and validation processes take the guesswork out of data preparation, ensuring that insights are based on solid foundations. Additionally, built-in compliance features handle industry standards and legal requirements, safeguarding against potential legal hurdles. These aspects contribute to growth by ensuring that decisions are sound and that the company’s actions are always within the bounds of the law. Self-Service Data Preparation vs. Scripting Self-service data preparation tools and scripting in languages like Python or R represent two distinct approaches to data preparation. Self-service tools offer a user-friendly, visual interface that simplifies data transformation tasks, making them accessible to a broad range of users, including those with limited coding experience. Scripting, on the other hand, provides the flexibility to write customized code for data preparation, requiring technical expertise. Let’s illustrate this difference with an example. If a user wants to use Python to remove duplicate records from a dataset called ‘data.csv’, the following code can be used: Python script for removing duplicates Whereas, in self-service data preparation tools, this process is simplified for non-technical users. Instead of writing code, a user can apply the “Distinct” transformation, and choose the column(s) to identify duplicates. The tool handles the rest, simplifying the process without writing any code. Additionally, self-service tools often offer visual cues and previews, helping users understand the impact of their transformations in real time. This ease of use empowers a broader range of individuals within an organization to perform data preparation tasks efficiently. Efficient Data Prep at Your Fingertips Effortlessly clean and transform data with LIKE.TG's no-code, point-and-click navigation, making data preparation accessible to all. Download Free Trial Key Factors to Consider When Evaluating a Self-service Data Preparation Tool There are several factors that an organization should consider before selecting a self-service data preparation tool. Some of them include: User-Friendly Interface: A user-friendly interface is a crucial aspect of a self-service data preparation tool. The tool should be chosen with business users in mind, offering an intuitive and easy-to-navigate environment. Look for a tool that allows users to perform tasks like data cleansing, transformation, and visualization without requiring extensive technical expertise. Features such as drag-and-drop functionality make it accessible to a wider audience within your organization. Data Integration: Data often resides in various sources and formats. A robust self-service data preparation tool should have the capability to seamlessly connect and integrate data from these diverse sources. It should support a wide range of data connectors and APIs, allowing users to pull in data from databases, spreadsheets, cloud services, and more. This integration capability ensures that your tool can handle the data sources critical to your business. Data Cleaning and Transformation: Data is rarely perfect, and it often requires cleaning and transformation to make it suitable for analysis. When evaluating a tool, assess its data cleaning and transformation capabilities. Look for features that enable users to cleanse data by identifying and addressing inconsistencies, missing values, and outliers. Additionally, the tool should provide transformation functions to reshape, aggregate, and enrich data to meet specific analysis requirements. Data Exploration and Visualization: Effective data preparation goes beyond cleaning and transformation — it also involves exploring and visualizing data to gain insights. Seek a tool that offers robust data exploration capabilities, allowing users to interact with data and perform exploratory data analysis. Look for features that enable the creation of visualizations, including charts, graphs, and dashboards. Visualization aids in understanding data patterns and trends, making it a valuable component of self-service data preparation. Real-Time Data Health Visuals: A good self-service data preparation tool should provide real-time data health visuals. These visuals offer continuous monitoring of data quality and health, enabling users to detect and address issues promptly. Alerts and notifications are valuable for promptly informing users about potential data quality issues, facilitating data-driven decisions. Real-Time Data Processing: Timely decision-making is crucial. Consider a tool that supports real-time data processing or near-immediate data preparation. This capability allows organizations to work with the most up-to-date data, ensuring that decisions are based on current information. Real-time data processing can be particularly valuable in industries where market conditions change rapidly. These key components collectively contribute to the effectiveness and efficiency of a self-service data preparation tool, enabling organizations to harness the full potential of their data for accelerated business growth. Final Word At its core, self-service data preparation embodies a fundamental shift—democratizing data access. It empowers every member of an organization, regardless of technical expertise, to participate in the data preparation process. Doing so expedites data handling and decision-making, and nurtures collaboration among diverse departments, resulting in a more informed and aligned workforce. This inclusive approach fundamentally transforms the organizational culture and, in turn, becomes a catalyst for sustainable growth. To reap its benefits, enterprises must invest in the right self-service data preparation tool. In this regard, LIKE.TG stands out as a reliable solution. With its user-friendly platform, LIKE.TG breaks down technical barriers, ensuring that data access and preparation are accessible to everyone. Some of its key features include: Point-and-Click Navigation Connectivity to a Wide Range of Sources Pre-Built Data Transformations Interactive Data Grid with Agile Correction Capabilities Real-Time Data Health Checks Data Quality Rules Workflow Automation Ready to embrace the transformative potential of self-service data preparation and accelerate your business growth? Discover how LIKE.TG’s advanced data preparation solutions can streamline your data handling processes, promote collaboration, and ensure data-driven success. Sign up for the 14-day trial and take the first step towards efficient and accessible data preparation. Get Clean, High-Quality Data Without Writing Code Try 14-Day Free Trial
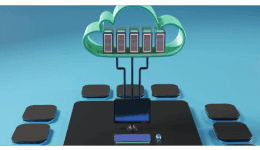
What is an ETL Pipeline? A Comprehensive Guide
What is an ETL Pipeline? An ETL pipeline is a type of data pipeline that includes the ETL process to move data. At its core, it is a set of processes and tools that enables businesses to extract raw data from multiple source systems, transform it to fit their needs, and load it into a destination system for various data-driven initiatives. The target system is most commonly either a database, a data warehouse, or a data lake. ETL pipelines are crucial for maintaining data quality during data integration and, ultimately, enabling organizations to make informed decisions based on a unified and well-organized dataset. ETL Pipeline Example ETL pipelines are all about transforming the data to meet the requirements of the target system. For example, your website could have data spread across various databases, including customer information, order details, and product information. To ensure that the data is consistent with the destination, you will need to transform the data—typically using ETL tools. Transformation usually involves cleaning the data, enriching it for additional context, removing duplicates, etc. Once your data is in the required format, it moves along the ETL pipeline and is loaded into the destination tables. This centralized website data with a consistent format allows you to conduct accurate data analysis and make better, more informed decisions. Looking for the best ETL Tool? Here's what you need to know With so many ETL Pipeline Tools to choose from, selecting the right solution can be overwhelming. Here's a list of the best ETL Pipeline Tools based on key criteria to help you make an informed decision. Learn More ETL vs ELT Pipeline ETL andELT (extract, load, transform)pipelines are similar in the context that both involve data extraction, transformation, and loading. However, the primary difference between an ELT and ETL pipeline is the sequence of the transformation and loading steps. The choice betweenETL vs ELTdepends on factors such as data volume, structure, and the capabilities of the target storage and processing systems. ETL pipeline extracts data from different sources and then stores it in a staging area where you can apply complex transformations. Only once the data is transformed can it be moved from the staging area to a target database or data warehouse. Use an ETL pipeline when you need to cleanse, enrich, or aggregate data before it reaches its final storage, ensuring that the data in the destination is already refined and ready for analysis. ETL pipelines are often preferred when dealing with structured data and when the target system requires a specific format. On the other hand, an ELT pipeline is geared towards loading data into the destination system as quickly as possible. The data is then transformed using the destination system’s processing capabilities when required. An ELT pipeline is well-suited for big data scenarios where the target system, such as acloud data warehouse, is capable of handling large-scale transformations efficiently. ELT pipelines are more flexible in dealing with raw or semi-structured data and leverage the computational power of modern data warehouses for on-the-fly transformations during analysis. ETL Pipeline vs Data Pipeline ETL pipelines are a subset ofdata pipelines. While both pipelines move data from source to target systems, what separates an ETL pipeline from a data pipeline is that a data pipeline doesn’t always involve data transformation. In fact, you can say that an ETL pipeline is a type of data pipeline that involvesdata extraction, transformation, and loading as the core processes. The table below summarizes theETL pipeline vs data pipeline: ETL Pipeline Data Pipeline Focus Emphasizes data extraction, transformation, and loading processes. Focuses on data movement. It may or may not involve data transformation. Purpose Extract data from various sources, transform it, and load it into a destination for analysis. The end goal is to transport data efficiently. Processes Extraction, transformation (data cleansing, normalization, etc.), and loading into a target system. Data movement, organization, and structuring. Scope Specifically deals with processes related to ETL. Has a much broader scope, covers a range of data handling tasks. Data Transformation Involves significant data transformation to make it suitable for analysis. May involve minimal transformation, mainly for organization. Build Fully Automated ETL Pipelines in Minutes Building and maintaining ETL pipelines does not have to be complex or time-consuming. LIKE.TG offers a 100% no-code solution to build and automate ETL pipelines. Learn More Benefits of ETL Pipelines ETL pipelines make data more accessible, especially when built and maintained via ETL pipeline tools. The following benefits together contribute to improved decision-making: Integrated Data Your data resides in various formats across databases, spreadsheets, and cloud storage. One significant advantage of ETL pipelines is that they bridge the gap between different data repositories. These data pipelines extract relevant data, transform it into a consistent structure, and load it into a destination where it can be seamlessly integrated with existing datasets. In essence, ETL pipelines break down data silos and lead to a unified view of company-wide data, whether in traditional relational databases or scattered across cloud-based platforms. Enhanced Data Quality ETL pipelines can be used to identify and rectify discrepancies in your data. The “T” in ETL pipelines stands for transformation, and this step facilitates data quality management. Through predefined rules and algorithms, the transformation phase addresses issues such as missing values, duplicate entries, and format inconsistencies. Transformation not only cleanses the data but also enriches and standardizes it, ensuring uniformity that is essential for robust analytics and decision-making. High Compatibility As your business evolves, so do your data needs. ETL pipelines are designed with a modular and scalable architecture that enables you to incorporate new data sources. This adaptability ensures that the ETL framework remains compatible with emerging technologies and diverse data formats. Furthermore, the transformative nature of the ETL pipelines allows for the modification of data formats and structures to meet evolving analytical needs. For example, ETL pipelines continue to incorporate newer data storage systems, such as data lakes, for data consolidation. Regulatory Compliance The transparency and traceability inherent in ETL pipelines contribute significantly to regulatory compliance. These pipelines often incorporate features that allow you to track and audit the entire data lifecycle and provide a comprehensive record of data lineage. Data lineage is invaluable in regulatory audits, where organizations are required to demonstrate the integrity and security of their data processes. Automation Automated ETL pipelines serve as a robust strategy for minimizing the risk of human errors, especially since manual data handling is inherently susceptible to inconsistencies due to oversights and inaccuracies. The automation of repetitive and time-consuming tasks, such as data extraction and transformation, also enables you to streamline workflows and allocate resources more strategically. It accelerates the pace at which you process data while reducing operational costs associated with manual labor. Take The First Step To Building ETL Pipelines. 100% No-Code! LIKE.TG empowers users to build and maintain high-performance ETL Pipelines without writing a single line of code. Download a 14-day free trial and start building your ETL Pipelines. Download Trial ETL Pipeline Use Cases Data Warehousing:Loading data from multiple operational systems into a central data warehouse is a classic ETL pipeline use case. It enables you to prepare and consume data for analytics and reporting. Data Migration:ETL pipelines facilitate data migration from one source system to another where it is temporarily stored for further processing, or a final destination for consumption. Data Integration: Businesses frequently use ETL pipelines to integrate company-wide data and build a single source of truth (SSOT). Business Intelligence: It includes leveraging ETL pipelines for business intelligence (BI) by connecting them directly to BI tools, such as Power BI, and generating insights. Legacy System Modernization: In this scenario, ETL pipelines play a critical role in streamlining the transition. They allow organizations to incorporate modern systems into their technology and data stack without losing valuable historical data. How to Build an ETL Pipeline There are two ways to build an ETL pipeline—you can either write code using languages like Python or use a dedicated ETL tool, such as LIKE.TG. The choice between writing custom code and using an ETL pipeline tool depends on various factors, such as the complexity of your ETL pipelines, the scale of your data, the required flexibility, and the skill set of your team. Building an ETL Pipeline Using Python UsingPythonto build ETL pipelines provides flexibility and customization. It allows you to tailor the process to your specific needs. So, this method is suitable when you have unique requirements or when you regularly deal with complex data transformations. Here is a high-level view of the process of building an ETL pipeline using Python: Define Requirements: Identify the data sources you need to extract data from, the required transformation logic to make it consistent, and the destination where the processed data will be stored. Install Necessary Libraries:Ensure you have the required Python libraries installed, such asPandasfor data manipulation and transformation, and any database connectors if needed. Extract Data:Write code to extract data from data sources such as databases, APIs, file formats, etc. Transform Data:Apply necessary transformations to the extracted data. Use Pandas or other libraries to clean, filter, and manipulate the data as required. Load Data: Write code to load the transformed data into the destination system. Schedule and Automate:Implement scheduling to automate your ETL pipelines. You can use tools like Apache Airflow or create your own scheduling mechanism usingPythoncronjobs. Logging and Monitoring: Implement logging to track, debug and monitor your pipeline’s health. Error Handling:Make sure to include error handling mechanisms to deal with issues during extraction, transformation, or loading. These mechanisms protect your pipelines and allow them to recover from failures. Testing:Test your ETL pipeline thoroughly with different scenarios and edge cases to ensure its reliability. Documentation:Document your ETL pipeline, including details about data sources, transformation logic, and destination. Documentation makes it easier for others to understand and maintain the pipeline. The downside to this manual method is that it requires more development effort and time. Writing custom Python code for ETL pipelines means that you have to handle all aspects of the process, including data extraction, transformation, and loading, as well as error handling and monitoring. Unlike dedicated ETL pipeline tools with graphical interfaces, custom Python code might not be as user-friendly for non-technical users involved in designing or monitoring ETL workflows. Simplify Data Integration with No-Code ETL Pipelines LIKE.TG empowers you to combine all your data, whether on-premises or in the cloud. Sign up for a free demo and see what it takes to build and maintain ETL Pipelines in a no-code environment. View Demo Building an ETL Pipeline via No-Code ETL Pipeline Tools Dedicated ETL pipeline tools come with graphical interfaces and pre-built connectors and transformations, making it easier to design and manage ETL workflows without coding. Once you’ve decided on a tool, the next step is to build the ETL pipeline. Building an ETL Pipeline using LIKE.TG While the specific steps can vary depending on the actual tool, the high-level process remains the same: Connect to Data Sources:Drag and drop the connectors onto the dataflow and connect to the data sources from which you want to extract data. These could be databases, APIs, flat files, or any other supported source. Extract Data:Use the ETL tool’s graphical interface to design the extraction process. Configure connectors and settings to pull data from the defined sources. Transform Data:Implement data transformations built into the ETL pipeline tool. These might involve applying filters, aggregations, or other transformations to clean and prepare the data for the destination. Connect to the Destination: Specify and establish connectivity to the destination where the transformed data will be loaded. ETL tools feature capabilities like AI auto data mapper to make the process effortless. Configure Loading Process:Use the ETL tool to configure the loading process. Define how the transformed data will be written to the destination, including any formatting or schema considerations. You can also configure whether the data will be loaded in batches, as well as the size of the batches. Schedule and Automate: Set up scheduling to automate the execution of your pipeline. Define when and how often the pipeline should run. The ETL pipeline can be configured to run at specific intervals or events, such as when an e-mail is received or a file is dumped into a folder. Error Handling and Logging:Configure error handling mechanisms within the ETL tool. Define how the tool should handle errors during extraction, transformation, or loading. You can also implement logging to track the execution and identify issues. Test the Pipeline:Thoroughly test the ETL pipeline with sample data to ensure that it functions as expected. Verify that data is extracted, transformed, and loaded accurately. Deployment:Deploy the ETL pipeline to your production environment. Monitor its performance and make any necessary adjustments. Using an automated tool can be beneficial when you need to quickly set up ETL pipelines, especially in scenarios where there’s a need to involve a non-technical user or a business professional in designing, using, maintaining, or monitoring the pipeline. Additionally, custom code requires ongoing maintenance—you’ll need to update and test the code every time there are changes in data sources, transformation logic, or destination formats. Take The First Step To Building ETL Pipelines. 100% No-Code! LIKE.TG empowers users to build and maintain high-performance ETL Pipelines without writing a single line of code. Download a 14-day free trial and start building your ETL Pipelines. Download Trial ETL Pipeline Best Practices Promote Modularity: Design modular and reusable components in your ETL pipeline. Breaking down the process into reusable components, each responsible for a specific task, makes the overall system more flexible and easier to manage. The modular approach simplifies updates to individual components without affecting the entire ETL pipeline, fostering consistency across the workflow. Implement Incremental Loading and CDC: If you don’t deal with real-time data streaming, consider implementing incremental loading and change data capture (CDC) to process only the changed data. It enables you to reduce processing time and unnecessary resource utilization. This technique is particularly effective for large datasets where processing the entire dataset in each run is not practical. Optimize Performance: You can employ several strategies to optimize the performance of your ETL pipelines. For example, consider partitioning and parallelizing data processing tasks to distribute the workload across multiple resources and enhance overall speed. Utilize indexing and proper data structures to expedite data retrieval and transformation processes. Additionally, you can leverage caching mechanisms to store and reuse intermediate results and reduce redundant computations. Implement Error Handling and Logging: Enable swift identification and resolution of issues during data processing via error handling. Comprehensive logging provides insights into the pipeline’s behavior, facilitating troubleshooting and auditing processes. This tandem approach enhances the reliability and maintainability of the ETL pipelines. Metadata Management: Organize and document metadata about data sources, transformations, and destinations to track changes and dependencies within your ETL pipeline. Metadata management enhances traceability and simplifies the process of understanding the lineage of data. This, in turn, makes it easier to troubleshoot issues, ensure data quality, and implement changes. LIKE.TG—The No-Code Way to Build Automated ETL Pipelines LIKE.TG is a 100% no-code solution for building fully automated ETL pipelines. Whether your data is on-premises or in the cloud, LIKE.TG empowers you to combine and move it to the destination of your choice. LIKE.TG offers native connectivity to a range of data sources and destinations with its pre-built connectors, including Amazon Redshift, Google BigQuery, Snowflake, Microsoft Azure, etc. With LIKE.TG, you can: Build fully automated ETL pipelines Accelerate data mapping with AI Auto Mapper Connect to multiple data sources and destinations Improve the quality of your data for a reliable single source of truth Handle large volumes of data effortlessly with a parallel processing ETL engine And much more—all without writing a single line of code. Ready to build end-to-end ETL pipelines with a 100% no-code data pipeline builder? Download a 14-day free trial or sign up for a demo. Alternatively, you can get in touch with our data solution experts directly at +1 888-77-LIKE.TG.
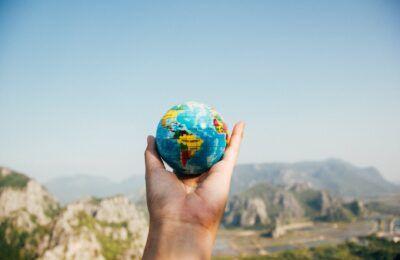
Mastering Data Transformation: A Comprehensive Guide
Did you know that only 3% of companies have data that meets basic quality standards? Data quality is essential for any business leveraging data for insights, decision-making, and innovation. However, data quality is not something that happens automatically. It requires a process of data transformation. Data Transformation Process The goal of data transformation is straightforward – extract data from the source, change it to a usable format, and load it to a destination. It is an essential part ofETL pipelines. It is an essential part ofdata preparation. Data transformation allows you to clean, reshape, and compute new data dimensions and metrics based on your needs. Traditionally, it takes hundreds to thousands of lines of code to buildETL or ELTpipelines. However, you can quickly build these pipelines on a dataflow visual canvas with a no-code ETL tool. Here are the critical steps in the data transformation process: Identify Sources: Start by pinpointing the data sources that require transformation. These sources range from databases and Excel files to delimited files, legacy sources, or cloud connectors. Some advanced data extraction tools even allow you to extract data from unstructured files like PDFs. Data Mapping: Plan the actual transformation by deciding where the data originates and where it will be stored. This phase involves converting source data into a format suitable for the destination, often requiring operations like adding, deleting, copying, and replicating fields and records. Data Validation: In this crucial step, data is thoroughly examined for errors, inconsistencies, duplicates, missing values, outliers, and more. Validation can be executed through rules, formulas, functions, or algorithms, depending on the data’s complexity and quality. Choose Transformation: Select the appropriate transformation methods based on your planned data mapping. Here are a few ways you can convert the data: Filtering: Selecting a subset of data based on specific criteria. Enriching: Adding new information or value to existing data. Splitting: Breaking down complex data into simpler parts. Joining: Combining data from different sources based on a common attribute. Cleansing Raw Data: Removing errors and eliminating redundant or repeated data elements. Data Loading: The final step involves loading the transformed data into the target destination, such as a data warehouse, data lake, data mart, dashboard, or report. Depending on data frequency and volume, loading can be done using batch, incremental, or streaming methods. Beyond these steps, you might need to perform customized operations to tailor the data to your desired format. Remember, data transformation is an ongoing process requiring constant monitoring and improvement. It’s not a one-size-fits-all solution and depends on data type, source, purpose, business objectives, and requirements. Remember that not all data requires transformation; sometimes, the source data is suitable for immediate use. Why Do Businesses Need Data Transformation? Data transformation is not just a checkbox on the to-do list; it’s a crucial and strategic process that can significantly impact your business’s performance and success. Let’s delve into the reasons why data transformation is indispensable: Improve Data Quality Data quality is the linchpin for businesses leveraging data for analysis, reporting, or decision-making. Transforming data allows you to identify and resolve errors, inconsistencies, duplicates, and outliers. For instance, envision a scenario where a financial institution employs data transformation to clean and standardize customer information, ensuring accurate and reliable data for risk assessments. Enhance Data Usability Usable data is critical for deriving insights, fostering innovation, and facilitating collaboration. Through data transformation, you can structure data in tables, charts, graphs, or maps for easy interpretation. Imagine a marketing team transforming raw customer engagement data into visually appealing dashboards, making it accessible and understandable for strategic planning. Enable Data Integration Combining data from various sources is vital for a holistic view and comprehensive analysis. Data transformation ensures that data adheres to common standards, facilitating seamless integration. Picture an e-commerce platform integrating customer data from website interactions, mobile app usage, and social media engagement through a unified data transformation process. Support Data Governance With its policies and procedures, data governance is critical for compliance, security, and quality assurance. Data transformation aligns data with predefined rules, regulations, and requirements. Consider a scenario where a financial institution transforms customer transaction data to comply with privacy regulations, ensuring secure and governed data practices. Now, let’s explore some real-world examples of how businesses can benefit from data transformation: Retail Company: Imagine a retail company extracting data from point-of-sale systems, inventory databases, and customer feedback platforms. Data transformation allows you to calculate key performance indicators and gain insights into sales, revenue, profit, and customer satisfaction. Loading this transformed data into a warehouse enables comprehensive analysis and reporting. Healthcare Organization: Data transformation helps extract information from electronic health records, medical devices, and insurance claims. Validating and anonymizing sensitive data, such as names and addresses, lets you load this transformed data into a data lake for research and innovation while ensuring patient privacy. Manufacturing Company: Data transformation allows you to extract data from sensors, machines, and quality control systems. Validating, detecting anomalies, and optimizing data ensure timely and relevant information. Loading this transformed data into a data mart facilitates monitoring and optimization of manufacturing processes. Data Transformation in the ETL Process In the ETL (Extract, Transform, Load)process, data transformation is central between the extraction and loading phases. Once data is extracted from diverse sources—like databases, applications, or external systems—often arrives in varied formats, structures, or states. This diversity demands a meticulous transformation before loading it into a target destination. MostETL toolsalso provide predefined functions that you can use to change your data quickly and efficiently. Here are some of the steps that are involved in this process: Identify the structure of the source files andextract datafrom them. Next, map the data from the source file to the transformation tool. Apply the transformation (i.e., filter, sort, cleanse, or aggregate data). Finally, send the transformed file to the destination. Why Transformation is Essential in ETL Data Homogenization: ETL processes often pull data from disparate sources, each with its peculiarities. Transformation standardizes this data into a unified format, ensuring consistency and compatibility for downstream analytics. Example: Consider a retail company extracting sales data from multiple stores with distinct databases. Transformation harmonizes the diverse data structures, allowing for seamless analysis and reporting. Aggregation and Calculation: Transformation facilitates data aggregations, calculations, and derivations. This step is crucial for deriving meaningful insights and metrics, such as calculating total revenue, average customer spending, or year-over-year growth. Example: A financial institution extracting transaction data may transform it to calculate daily transaction volumes or monthly revenue trends, aiding strategic decision-making. Cleansing and Enrichment: ETL transformation addresses data quality issues by cleansing and enriching the information. This involves handling errors, eliminating duplicates, and adding missing values, ensuring the integrity of the data. Example: In healthcare, ETL transformation might involve cleansing patient records, removing inconsistencies, and enriching them with additional data for comprehensive analysis. Data Transformation in the ELT Process Unlike ETL, ELT flips the sequence by performing a transformation after loading data into the destination. This approach leverages the processing power of modern data warehouses, allowing transformations to occur closer to the data storage point. Why Transformation is Essential in ELT Raw Data Storage: ELT processes often load raw, unaltered data into the destination first. Transformation then occurs within the data warehouse itself. This raw data storage provides flexibility for future analysis and on-the-fly transformations based on evolving business requirements. Scalability and Parallel Processing: ELT takes advantage of the scalable architecture of modern data warehouses, enabling parallel processing of transformations. This scalability ensures efficient handling of large datasets and accelerates the transformation process. Near-Real-Time Analytics: ELT supports near-real-time analytics by transforming data within the destination. This allows organizations to derive insights promptly, respond swiftly to market changes, and make informed decisions. In ETL and ELT, data transformation is the linchpin that converts raw, heterogeneous data into a refined and usable form. It ensures data quality and consistency and empowers organizations to derive actionable insights from their vast and diverse datasets. Data Transformation Challenges Data Complexity Data can be intricate, varying in volume, variety, velocity, integrity, or value. This complexity demands additional resources, tools, techniques, and skills to handle and process the data effectively. Data Inconsistency Inconsistencies in format, structure, schema, or semantics are challenging during data transformation. Aligning and integrating such inconsistent data requires extra effort, time, and logical considerations. Data Security Sensitivity in terms of privacy, confidentiality, or integrity makes data security a critical concern. Safeguarding the data throughout the transformation process necessitates stringent measures, controls, and audits. Data Scalability Scalability, driven by growth, change, or demand, adds complexity to data transformation. Accommodating and adjusting to scalable data requires the transformation process’s flexibility, adaptability, and agility. According to Gartner, 60% of data experts believe data quality across data sources and landscapes is the biggest data management challenge. Solutions and Best Practices Data Profiling Engage in data profiling to analyze and understand the data before transformation. This step provides essential insights into data characteristics, such as size, type, distribution, and quality. With this knowledge, you can more effectively plan and design the data transformation process. Example: Conduct data profiling to identify potential outliers before transforming a vast dataset, ensuring a more accurate and streamlined transformation. Data Mapping Utilize data mapping to define and document relationships and rules between source and target data. It adds clarity and guidance to the transformation process by specifying what to convert, modify, enrich, or standardize. It ensures an organized and monitored transformation journey. Example: Document data mapping between customer information sources to maintain consistency across various platforms during transformation. Data Encryption Implement encryption to transform data into an unreadable form using secret keys or algorithms. This protective measure ensures the security and preservation of sensitive data, preventing unauthorized access, modification, or disclosure throughout the transformation process. Example: Encrypting personally identifiable information (PII) during transformation to comply with data protection regulations and secure customer data. Data Automation Leverage data automation to perform the transformation process automatically or with minimal human intervention. Automation adds flexibility, adaptability, and agility, facilitating faster, easier, and cost-effective data transformation. Example: Automate routine data transformations in a dynamic e-commerce environment to swiftly adapt to changing product catalogs or promotional data. Turn Disparate DataInto Powerful Insights Transform raw data into accurate insights in minutes. Easily establish connectivity with a wide range of sources. Get Your Free Trial! How No-Code Enables Data Transformation within a Few Minutes. The significance of data transformation in today’s organizational landscape cannot be overstated. It transforms raw, heterogeneous data into a refined and usable form, allowing businesses to enhance data quality, usability, integration, and governance. The transformative power of data lies in its ability to unlock valuable insights, support informed decision-making, and foster innovation. Yet, navigating the complexities of data transformation poses challenges, from managing data complexity and inconsistency to ensuring security and scalability. In overcoming these challenges, organizations truly harness their data’s potential. A no-code data transformation tool like LIKE.TG empowers you to perform data transformation within a few minutes, eliminating the need for extensive coding or technical expertise. With LIKE.TG, the process becomes seamless and accessible, allowing even non-technical users to build ETL or ELT pipelines effortlessly. It offers an intuitive visual canvas, making data transformation swift and agile. As a result, you can democratize data transformation and reshape data as needed, ensuring insights are accessible across your organization. Ready to experience the game-changing efficiency of no-code data transformation? Start your data transformation journey with LIKE.TG – get a 14-day trial now!
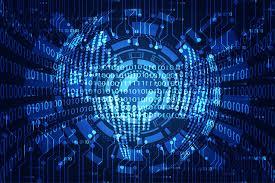
Top 7 Data Validation Tools to Explore in 2024
In 2015, PayPal had to pay $7.7 million to the US government when their lack of proper screening mechanisms led to 500 PayPal transactions worth $44,000, violating sanctions against Iran, Cuba, and Sudan. In 2018, Samsung Securities incurred a cost of $105 billion when an employee issued 2 billion shares to 2,018 company employees instead of dividends totaling 2 billion won (South Korean currency). Bad data costs companies billions of dollars every year. That’s why you need a data validation tool to ensure your data is accurate, consistent, and reliable. What are Data Validation Tools? Data validation tools automatically check and verify data for accuracy, completeness, and conformity to predefined standards. An organization can set up certain rules or conditions like including data from up to five years for analysis. Any value that doesn’t meet the specified criteria will be excluded. These tools meticulously examine and scrutinize the data by employing various functions to flag and rectify discrepancies. Let’s review the top 7 data validation tools to help you choose the solution that best suits your business needs. Top 7 Data Validation Tools LIKE.TG Informatica Talend Datameer Alteryx Data Ladder Ataccama One 1. LIKE.TG LIKE.TG is an enterprise-grade, unified data management solution with advanced data validation features. By offering agile data cleansing and correction capabilities, the tool empowers you to access trusted, accurate, and consistent data for reliable insights. The platform also allows you to implement rigorous data validation checks and customize rules based on your specific requirements. Furthermore, by providing real-time data health checks, the platform provides instant feedback on the data quality, enabling you to keep track of changes. For efficient data validation, LIKE.TG has a rich set of transformations that allow you to: Find and replace null/missing values. Convert the data formats and values into a common format. Remove duplicates from a column or the entire dataset. Apply custom validation rules to use relevant data for analysis Entries flagged as erroneous in the data validation process Other Key Features: Drag-and-drop Interface: The visual and interactive interface empowers you to simply drag the objects, manipulate the data, and write it to the destination of your choice without writing a single line of code. Connectivity to Wide Range of Sources: LIKE.TG allows you to seamlessly connect to on-premise systems as well as cloud-based sources. Moreover, now you can build API-based connectors for any integration or import connectors from LIKE.TG’s library of custom-built connectors. Built-in Transformations: LIKE.TG provides a comprehensive library of pre-built transformations such as join, reconcile, aggregate, normalize, and more allowing you to perform complex data operations with just a few clicks. Workflow Automation: The tool includes job scheduling and automation capabilities based on time-based and event-based triggers, eliminating manual intervention. Parallel Processing: Its industrial-strength ETL engine splits large data sets into smaller subsets that are processed in parallel to ensure high-speed integration, regardless of the data size and format. AI Integration: LIKE.TG is leveraging AI by incorporating it into its various operations such as data extraction, mapping, and modeling, simplifying the data management process. See It in Action: Sign Up for a Demo Curious about how LIKE.TG streamlines data validation? Sign up for a demo and explore all the features you can leverage to get analysis-ready data without writing a single line of code. View Demo 2. Informatica Informatica is a data management platform that allows users to perform critical data quality tasks, such as deduplication, standardization, enrichment, and validation. Users can identify, rectify, and track data quality issues both in the cloud and on-premises. Key Features: Connectivity to Diverse Sources: The platform has built-in connectors, allowing users to connect with various source systems such as databases, file systems, or SaaS-based applications. Data Preparation: Informatica allows users to profile, standardize, and validate the data by using pre-built rules and accelerators. Data Monitoring: The solution provides users with visibility into the data set to detect and identify any discrepancies. Parallel Processing: Informatica enables users to run multiple jobs simultaneously by splitting up tasks to improve performance time. 3. Talend Talend is a data quality and integration solution providing users with access to accurate data. Its data quality solution profiles, cleanses, and standardizes data across systems. Driven by machine-learning algorithms, it offers recommendations to correct and rectify data quality issues. Moreover, the built-in Trust Score provides an evaluation of the overall health of the data to identify discrepancies and irregularities within the dataset. Key Features: Self-Service Data Integration: Talend’s self-service data integration platform allows users to build and deploy data integration jobs without writing a single line of code. Data Transformation: Talend offers a wide range of data transformation capabilities, including filtering, sorting, aggregating, and joining data. Data Preparation: Talend allows users to prepare the data, apply quality checks, such as uniqueness and format validation, and monitor the data’s health via Talend Trust Score. Data Security and Compliance: Talend allows users to protect sensitive information by providing role-based access and ensures compliance with regulations like GDPR and HIPAA. 4. Datameer Datameer is a data preparation and transformation solution that converts raw data into a usable format for analysis. The platform is engineered for Snowflake, a cloud-based solution, managing all aspects of the data lifecycle, from exploration to preparation to sharing trusted datasets. By offering a spreadsheet-style interface, the platform allows users to navigate and interact with complex data in an intuitive manner. Key Features: Data Preparation: Datameer’s self-service data preparation interface is spreadsheet-like, making it easy for users to explore, transform, and visualize data. Native integration with Snowflake: Datameer enables data engineers and analysts to transform data directly in Snowflake via a simple SQL code or code-free interface. Data Encoding: Datameer’s encoding feature automatically converts categorical data into a binary format for use in machine learning models. Data Catalog: Datameer’s catalog feature provides a centralized view of all data assets within an organization, with intelligent search capabilities. 5. Alteryx Alteryx is a data preparation and analytics platform that enables access to timely insights. It works as a workflow designer, offering connectivity to various sources such as flat files, database connections, APIs, etc., – both on-premises and cloud, allowing users to transform and access data in a single platform. The platform leverages AI to provide recommendations regarding data quality improvements. This aids in validating, transforming, and filtering the data according to the requirements. Key Features: Data Profiling: Alteryx Designer offers data profiling capabilities that allow users to understand the characteristics of data and identify potential problems. Data Quality: Alteryx enables users to uncover and validate data quality issues with its AI-powered recommendation systems. Data Governance: The platform allows users to track and manage data lineage, audit logs, and ensure role-based access control. Performance Monitoring: Alteryx Designer offers performance monitoring features that can be used to track the performance of data preparation and analytics workflows. 6. Data Ladder Data Ladder is a data quality solution with built-in data profiling, cleansing, and deduplication capabilities. The software verifies the data before storing it in a database, offering real-time data quality validation. The platform has an intuitive visual interface, allowing effortless integration with custom-built or third-party applications. Key Features: User-friendly Interface: Data Ladder offers a visual and interactive interface, enabling technical business users to process data in a code-free environment. Data Preparation: The platform allows users to discover, cleanse, validate, and match according to the business’ specific data quality requirements. Data Import: Data Ladder allows users to integrate data from multiple disparate sources, including file formats, relational databases, cloud storage, and APIs. Data Matching: The platform enables users to employ proprietary and industry-grade match algorithms, allowing them to define custom criteria and match confidence levels for exact, fuzzy, numeric, or phonetic matching. 7. Ataccama One Ataccama One is a data management solution that offers data quality and validation features to improve the accuracy and reliability of the data. It ensures continuous data quality management by leveraging AI to automatically detect anomalies and irregularities and make changes as needed. Moreover, users can set custom rules to validate their data by using sentence-like conditions or the rich expressions the solution provides in an interactive interface. Key Features: Data Quality: Ataccama One helps users improve the accuracy, completeness, and consistency of their data by offering data profiling, cleansing, enrichment, and validation capabilities. Data Catalog: Ataccama One enables users to discover, understand, and manage their data assets, including features for data search, lineage, and documentation. Data Lineage: Ataccama One allows users to track the flow of data through their systems to identify data quality issues and improve the accuracy of their data. AI Integration: Ataccama One uses AI and machine learning to automate data management tasks and to improve the accuracy of data quality checks. Features to Look Out for in Data Validation Tools Custom Rules The ability to create specific rules, expressions, and conditions based on business requirements is crucial. A data validation tool should be able to tailor, customize, and modify the criteria based on evolving needs to ensure adaptability. Data Profiling Data profiling enables an organization to analyze the current sources of its data and understand the structure, quality, and relationships between attributes. This overview of data highlights problems within the dataset, such as inaccuracies, inconsistencies, and irregularities. Therefore, it is essential for a data validation tool to provide an assessment of the data quality in real time. Data Transformation Data is often in raw form and needs to be transformed into a usable format. A data validation tool should have features that include cleaning the dataset to account for missing values, converting inconsistent data into a standard format, joining/merging different datasets to provide a complete view, and, finally, enriching the data by adding new variables from external sources. Data Monitoring Another vital feature of a data validation tool is the ability to monitor and track data over time and alert in case of errors, discrepancies, or anomalies. By informing users of any changes in data events, alerting can assist in responding, rectifying, and resolving mistakes promptly to preserve the overall quality of the data. Data Testing Data testing or data validation refers to verifying whether the data meets the predefined conditions and constraints set so that the data aligns with the business objectives. By applying various data quality checks, the data is filtered and refined for consumption. This feature ensures that the data is healthy, trustworthy, compliant, and ready to be used for analysis. Must-have features While selecting a data validation tool, it is essential to look out for these key features: Real-time health checks: The ability to track and monitor the data in real-time enables users to identify and resolve data quality issues as they arise. This offers a complete view of the data’s health, highlighting opportunities for cleansing, transforming, standardizing, and validating the data. Interactive Data Grid: The ability to preview, analyze, and interact with data offers the flexibility to modify it as needed. Users should be able to make the necessary changes within the grid to ensure data accuracy and consistency. Automation: A data validation tool should have a built-in scheduler so that whenever a file is dropped in the mailbox, the process automatically runs the data against the predefined rules, ensuring accuracy and automating the process. Criteria for Selecting the Right Data Validation Tool There are several factors that influence the decision regarding the data quality tools selection process. It is important to ensure that the features and capabilities of a data validation tool are aligned with the organization’s requirements. The following factors stand out: Data Requirements: A data validation tool should be capable of dealing with diverse data structures, formats, and sources. An organization may be dealing with structured, semi-structured, and unstructured data. Hence the data validation tool should be flexible enough to accommodate the unique demands of the various data types while maintaining quality. Scalability: As data volume continues to grow, an organization should choose a solution that can handle vast amounts of data efficiently. The tool should, therefore, exhibit scalability and apply data quality checks on large datasets without compromising the speed and accuracy of data processing. User-friendly Interface: Setting up custom data validation rules and checks is complex for users with a non-technical background, emphasizing the need for a simple and easy-to-use tool. By offering an intuitive approach to validate the data, such as a drag-and-drop interface and point-and-click navigation, the tool results in short learning curves and few errors, streamlining the validation process. Ease of Integration: Finally, data validation tools should integrate easily with existing systems and workflows. Compatibility and connectivity with various data types and sources within an organization, such as databases, cloud systems, and APIs, are crucial to integrating the data effortlessly. Benefits of Using Data Validation Tools Without proper data validation mechanisms, enterprises might run into issues such as skewed analysis, flawed insights, and delayed or inaccurate decision-making. A data validation tool enhances an organization’s data management efforts for several reasons: Improved Data Accuracy Data validation solutions prevent the spread of erroneous data throughout an organization’s systems by detecting mistakes early in the data entry or import process. This allows for well-informed decisions and accurate analysis. Resource Efficiency Manual data validation takes time and is prone to human errors. Data validation software automates the process, quickly discovering anomalies and irregularities without requiring extensive human interaction. This automation saves time, allowing teams to focus on more strategic responsibilities. Regulatory Adherence Maintaining correct data is not only desirable but necessary for businesses governed by strict norms and standards. Data validation solutions help organizations achieve compliance obligations by guaranteeing the correctness and integrity of their data. Compliance, in turn, helps avoid penalties and instills confidence in stakeholders who rely on accurate data for audits and reporting. Final Words Validation is essential for ensuring the integrity and trustworthiness of data throughout its lifecycle. As new data sources emerge, it is crucial to apply business-specific data validation rules and conditions to ensure that it is in the desired format. Carefully analyzing the factors discussed above will help you choose the right data validation that meets your data requirements. Investing in data validation tools, such as LIKE.TG, can help your business avoid costly errors, ensure operational efficiency, and gain a competitive edge. Want to convert raw data into a usable format? Download LIKE.TG’s 14-day free trial today!
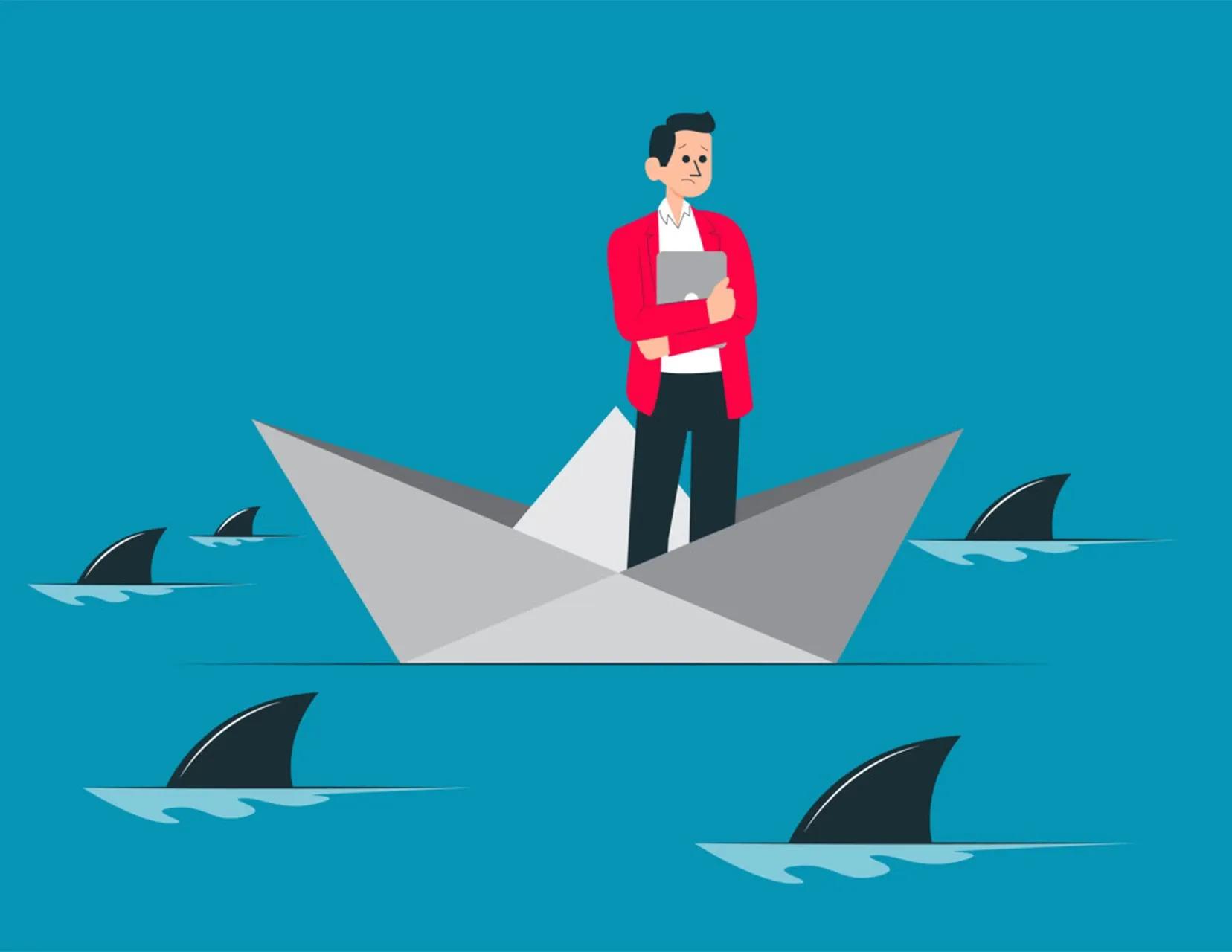
What is a Cloud Data Warehouse? A Complete Guide
What is a Cloud Data Warehouse? Simply put, a cloud data warehouse is a data warehouse that exists in the cloud environment, capable of combining exabytes of data from multiple sources. Cloud data warehouses are designed to handle complex queries and are optimized for business intelligence (BI) and analytics. The benefits of a cloud data warehouse extend to breaking data silos, consolidating the data available in different applications, and identifying opportunities that would otherwise go unnoticed with a traditional on-premises data warehouse. Cloud Data Warehouse Definition A cloud data warehouse is a centralized database in a public cloud for storing, processing, integrating, and managing large volumes of structured and semi-structured data. The “cloud” part means that instead of managing physical servers and infrastructure, everything happens in online — offsite servers take care of the heavy lifting, and you can access your data and analytics tools over the internet without the need for downloading or setting up any software or applications. A cloud data warehouse is critical to make quick, data-driven decisions. It offers improved computational ability and simplified data management, allowing you to extract valuable insights from updated, accurate, and enriched data when needed. Key Features of a Cloud Data Warehouse There are certain key features inherent to a cloud data warehouse that position it as a valuable solution for businesses looking to benefit from the cloud. It offers the right balance of security, scalability, and accessibility, along with numerous other features. These include: Performance: Quick and efficient querying of large datasets. Integration: Seamless integration with various analytics tools. Security: Strong measures like encryption and access controls. Cost Management: Pay-as-you-go model for cost-effectiveness. Scalability: Easily adjusts to data volume and processing needs. Accessibility: Data access from anywhere with an internet connection. Automatic Updates: Regular automatic updates for the latest features and security patches. Practical Tips To Tackle Data Quality During Cloud Migration The cloud offers a host of benefits that on-prem systems don’t. However, cloud migrations are not a straightforward journey. Here are some tips to ensure data quality when taking your data warehouse to the cloud. Download Whitepaper Cloud Data Warehouse vs On Premise Data Warehouse The traditional data warehouse architecture can no longer cope with the growing analytics needs of businesses today. The fact that the cloud data warehouse market is expected to reach $3.5 billion by 2025 only means that traditional, on-premises data warehouses have increasingly been unable to provide organizations with the speed, scalability, and agility they seek. The table below summarizes the difference between cloud data warehouse vs on-premises data warehouse: On-Premises Data Warehouse Cloud Data Warehouse Deployment Deployed on physical servers on-site Deployed on virtualized servers on the internet Scalability Offers limited scalability, requires upfront hardware investment Easily scalable with on-demand resources adjustment Maintenance Requires in-house IT management for updates and troubleshooting Managed services, less maintenance burden Cost Structure Involves capital expenditure (CapEx) with upfront costs for hardware and infrastructure Operational expenditure (OpEx), pay-as-you-go pricing model offers flexibility and efficiency Flexibility Fixed capacity, harder to adapt to changing needs Flexible, can scale resources based on demand Integration Limited integration with cloud services Seamless integration with various cloud services Accessibility Limited accessibility, tied to physical location Accessible from anywhere with an internet connection Deployment Speed Longer lead times for hardware procurement, setup, and configuration Quick deployment with on-demand resources, reduced time-to-value Updates and Upgrades Manual updates and upgrades, potentially causing downtime Automated updates, minimal downtime with managed services Disaster Recovery Relies on on-premises backup and recovery solutions Built-in disaster recovery options in the cloud Cloud Data Warehouse Architecture Cloud data warehouse architecture refers to the structural design and organization of components within a data warehouse that is hosted and managed in the cloud. It includes key elements and their interactions, ensuring efficient data processing, storage, integration, and retrieval. The following components make up the cloud data warehouse architecture: Data Sources: The data sources refer to the diverse origins from which data is collected and ingested into the data warehouse for analysis. These sources can vary widely in terms of data types, formats, and delivery mechanisms, ranging from transactional databases to streaming data and external APIs. One of the biggest strengths of cloud data warehouses is their ability to handle diverse types of data, including structured, semi-structured, and unstructured data. Data Ingestion Layer: The data journey in a cloud DWH begins with the data ingestion layer, which is responsible for seamlessly collecting and importing data. This layer often employs ETL processes to ensure that the data is transformed and formatted for optimal storage and analysis. Some cloud data warehouses support real-time data ingestion, allowing you to ingest and process data as it becomes available. Storage Layer: The storage layer organizes and stores data in a structured format optimized for analytical processing. This format may involve columnar storage, which is well-suited for analytics due to its ability to compress and store similar data types together. The storage layer integrates with the compute layer for data retrieval based on the requirements of analytical queries. Many cloud data warehouses utilize distributed file systems for storage, distributing data across multiple nodes and providing scalability and parallelism. Compute Layer: The compute layer is responsible for processing queries and performing analytical operations on the stored data. It manages the allocation of resources, such as CPU and memory, to different queries and workloads. Resource allocation is dynamic and can be adjusted based on the priority and requirements of the ongoing tasks. Query Optimization and Execution: The compute layer incorporates query optimization techniques to improve efficiency. The cloud data warehouse’s engine optimizes SQL queries by choosing optimal execution plans, indexing strategies, and through other optimizations to minimize query response times. Many cloud data warehouses use cost-based optimization to parse queries. This approach evaluates different execution plans and selects the one with the lowest estimated cost. Integration with BI Tools: Cloud data warehouses provide connectivity protocols and interfaces that allow seamless integration with BI tools. Common protocols include Java Database Connectivity (JDBC), Open Database Connectivity (ODBC), and RESTful APIs. These data warehouses also support Online Analytical Processing (OLAP) capabilities, allowing BI tools to create data cubes for multidimensional analysis. This is particularly valuable for complex analytical scenarios. Design, develop, and deploy your data warehouse in the cloud Building a data warehouse no longer requires coding. With LIKE.TG Data Warehouse Builder you can design a data warehouse and deploy it to the cloud without writing a single line of code. Learn More Benefits of Cloud Data Warehouse Cloud data warehouses are easier to set up compared to their traditional counterparts, which generally entails a complex setup. A modern CDWH stores, integrates, and processes large volumes of data from several sources, whether on-premises or on the internet. Here are more benefits of a cloud data warehouse: Enhanced Accessibility Data warehouses hosted on he cloud allow access to relevant data from anywhere in the world. What’s more, they come with access control features to ensure that the data required for BI is only visible to the relevant personnel. Interestingly, even though multiple employees may be accessing the data warehouse simultaneously, data integrity remains intact. The added layer of governance enhances the overall data quality management efforts of an organization. Limitless Scalability The virtual architecture enables organizations to modify their resource allocation according to changing demands. With a cloud data warehouse, companies with fluctuating needs have the option to pay only for the features and capabilities they need – something impossible with on-premises alternatives. For instance, tourism companies may need more computational power for enhanced analytics during the high season while it may only consume a fraction of this processing power during the low season. Uncapped Performance A cloud data warehouse allows all departments in an organization to access relevant data simultaneously without sacrificing performance. This is possible because they typically have multiple servers that share the load, ensuring that large amounts of data are processed simultaneously without any delays. Abundant Data Storage One of the most convincing reasons to opt for a cloud data warehouse is the excess amount of storage it offers. As mentioned earlier, cloud data warehousing solution providers often have a pay-as-you-go pricing model, which allows organizations to scale up or down without wasting storage space. The same also applies to other capabilities and features that allow businesses to experiment with data warehousing projects without incurring high costs. Seamless Integration According to a recent study, companies use data from over 400 sources for analytics and business intelligence. So, the data is not only in several different formats, but also structured in different ways, which makes integration difficult. Cloud data warehouses can help maneuver through the challenges of integration as they are designed to integrate data from multiple sources, including cloud applications, databases, and file formats. This structure also allows extraction and consolidation of semi-structured and unstructured data. Disaster Recovery Disaster recovery with legacy databases is often questionable. Companies using legacy tools must spend large amounts of money for additional hardware required to create data backups in case of a disaster or a system failure. A cloud data warehouse mitigates most of these problems by regularly creating backups, protecting important data in case of a disaster. Additionally, organizations adopting virtual solutions for their analytics avoid the unnecessary costs of purchasing equipment or storage areas to store their hardware. Design a Cloud Data Warehouse From Scratch With LIKE.TG Data Warehouse Builder, you can design purpose-built, cloud data warehouses from scratch within days. Sign up for a demo and see how it's done. View Demo Cloud Data Warehousing Challenges While cloud data warehouses offer significant benefits, especially when it comes to scalability and flexibility, it has its own set of challenges and complexities. Data Integration Data integration challenges in the cloud are due to the diversity in data sources, the dynamic nature of the infrastructure, and the need to manage and govern data effectively. Additionally, organizations often have a mix of on-premises and cloud-based systems and integrating data between these systems can involve several additional considerations, including security, latency, and connectivity. Security The need to align encryption practices with specific organizational requirements can be complex due to the diverse data environments. For example, if your organization has a hybrid infrastructure, including on-premises and cloud-based systems, integrating encryption practices between them seamlessly can be challenging. Additionally, operating in multi-cloud environments requires access control standards that are compatible across different cloud platforms. Ensuring consistent access controls when data is distributed across multiple cloud providers requires standardization efforts. Compliance Cloud service providers operate on a shared responsibility model, where they manage certain aspects of security, but customers are responsible for others. Understanding and fulfilling this shared responsibility can be complex. The dynamic and diverse nature of regulatory landscapes, which often span industries and jurisdictions, can become a hurdle in ensuring compliance with regulatory bodies. Cost Management While cloud data warehouses offer unparalleled flexibility and on-demand resources, the pay-as-you-go model can lead to unexpected costs if not carefully monitored. The challenge lies in optimizing resource utilization to match variable workloads and data processing demands. It can be difficult to predict costs accurately, particularly when dealing with fluctuating data volumes and complex analytical queries. Additionally, the diverse range of services and features offered by cloud data warehouses can result in unintentional over-provisioning or underutilization, impacting cost efficiency. Vendor Lock-In Organizations leveraging the features and services of a specific cloud data warehouse solution provider risk becoming tightly integrated with that provider’s proprietary technologies and APIs. While these technologies enhance efficiency and functionality, they also create dependencies that can be challenging to unravel. Transitioning to a different cloud provider or adopting a multi-cloud strategy becomes complex, as the migration process may involve rewriting queries, adapting data models, and addressing compatibility issues. Dimensional Modeling or Data Vault Modeling? We've got both! Whether you're into Dimensional Modeling for intuitive analytics or Data Vault Modeling for agile scalability, we have you covered. Get the best of both worlds with LIKE.TG Data Warehouse Builder. Download Trial Best Cloud Data Warehouse Solutions for Businesses Most cloud data warehousing solutions operate on the pay-as-you-go pricing model preferred by businesses, especially startups that are new to the world of data warehousing. This pricing option is also helpful for businesses that foresee new sources and platforms being added to their data architecture because a cloud data warehouse can evolve quickly to meet these needs. Additionally, the most common cloud data warehouse solutions offer similar value when it comes to delivering high-performance, scalability, flexibility, ease-of-use, and pricing. What varies is how these are implemented. Organizations should carefully evaluate the unique features and strengths of each cloud data warehouse solution based on their specific requirements and preferences. Cloud Data Warehouse: Microsoft Azure Synapse Analytics Microsoft Azure Synapse Analytics combines big data analytics with enterprise data warehousing to accelerate time to insight. Specifically, it uses SQL for data warehousing, Spark technologies to handle big data, and Pipelines for data integration via ETL and ELT. Azure Synapse Analytics also integrates seamlessly with BI tools like Power BI. It can be a viable data warehouse solution if your organization is involved in all, or most of, these data management endeavors. Additionally, if you already use multiple other Microsoft services, consider integrating Azure Synapse Analytics into your existing data stack since Microsoft’s services integrate smoothly together. Pros of Azure Synapse Analytics Seamless integration with other Azure services and advanced BI, analytics, and ML platforms Support for diverse data types, including unstructured data Cost-effective on-demand serverless querying Easily scales to handle large datasets On-demand resource provisioning offers added flexibility Robust security features for data protection Cons of Azure Synapse Analytics High dependency on the Azure ecosystem Fine-tuning for optimal results can be complex Frequent updates and changes mean users must continuously adapt Potential cost escalation with increased usage Learning curve for teams unfamiliar with the platform. Users often need training to adapt to the platform Use Azure Synapse Analytics for: Big data analytics Real-time analytics Serverless querying on data lakes Predictive analytics and forecasting Enterprise-grade cloud data warehousing Integrating advanced analytics and ML Cloud Data Warehouse: Amazon Redshift Amazon Redshift is a fully managed, petabyte-scale data warehouse service provided by Amazon Web Services (AWS). It is designed to handle large datasets and deliver high-performance analytics for organizations seeking a scalable and cost-effective solution. Amazon Redshift is particularly well-suited for analytical workloads and business intelligence applications. Pros of Amazon Redshift Easily scales from small to large datasets Offers fast query performance, especially for analytics workloads Seamless integration with other AWS services for comprehensive solutions Automated backups and maintenance reduce operational burden Robust security features to protect sensitive data Cons of Amazon Redshift Optimized for analytical queries; less suitable for transactional workloads Feature availability varies by region Users might need time to familiarize themselves with AWS platform and ecosystem While cost-effective, large-scale usage can incur significant costs Use Amazon Redshift for: BI and analytics Cloud data warehousing Ad-Hoc analysis Integration with AWS services Complex queries and aggregations Scalable data processing Cloud Data Warehouse: Google BigQuery Google BigQuery is a fully managed, serverless cloud data warehouse solution provided by Google Cloud Platform (GCP). It is designed to handle large-scale analytics workloads and enables you to analyze and query large datasets in real-time. Its integration with other Google cloud services makes it a comprehensive platform for various data analytics needs. Pros of Google BigQuery Serverless operation means the platform scales automatically Optimized for fast query performance, suitable for real-time analytics Efficiently handles large datasets, scaling automatically based on workload Seamless integration with other Google Cloud services Familiar SQL syntax for easy adoption by data analysts and developers Support for real-time data streaming Cons of Google BigQuery Not designed for transactional processing; optimized for analytics Integration with GCP may result in some degree of vendor lock-in While cost-effective for small to medium workloads, expenses can escalate for large-scale usage Users might need time to familiarize themselves with Google’s platform and ecosystem Use Google BigQuery for: Ad-hoc data analysis Real-time dashboards Log analytics IoT data analytics Predictive analytics Cloud data warehousing Cloud Data Warehouse: Snowflake Snowflake is a cloud-based data warehousing platform that provides a fully managed and scalable solution for storing and analyzing data. It operates as a Software-as-a-Service (SaaS) platform and is designed to be simple, flexible, and efficient for organizations seeking a modern cloud data warehouse. Pros of Snowflake Users can deploy Snowflake on multiple cloud platforms, offering flexibility and avoiding vendor lock-in Automatic scaling ensures optimal performance for varying workloads Facilitates easy and secure sharing of data between organizations or departments Efficient cloning of databases or tables without additional storage usage (zero-copy cloning) Access to historical data and recovery from changes Ability to scale storage and compute independently Cons of Snowflake Transferring data between different cloud providers can incur additional costs Users might need time to familiarize themselves with Snowflake’s platform Some complex workloads require fine-tuning for optimal performance While offering cost-effectiveness, large-scale usage can result in significant costs Use Snowflake for: Cross-cloud data replication Data-intensive application development Company-wide data sharing Cybersecurity analytics Cloud data warehousing Enhanced data access Dimensional Modeling or Data Vault Modeling? We've got both! Whether you're into Dimensional Modeling for intuitive analytics or Data Vault Modeling for agile scalability, we have you covered. Get the best of both worlds with LIKE.TG Data Warehouse Builder. Download Trial Opting for a Cloud Data Warehouse: Factors to Consider It’s crucial to consider several factors when selecting a cloud data warehouse solution for your organization. Here are some considerations you can take into account: Ease of Use When evaluating a cloud data warehouse, the simplicity and familiarity of the query language are paramount, particularly if your team is well-versed in SQL. A seamless transition is crucial for efficiency and productivity. Additionally, assess the solution’s integration capabilities with your current BI tools and data integration services. A cloud data warehouse that effortlessly fits into your existing technology and data stack ensures a cohesive and streamlined workflow and minimizes disruptions. Performance Assessing query performance, particularly for complex analytical queries, provides insights into the platform’s ability to handle your specific workloads effectively. Concurrent user and query handling capabilities are equally important, as a robust solution should be able to manage multiple simultaneous users and queries without compromising responsiveness. Scalability, both in terms of storage and compute resources, is an important consideration to ensure the solution can seamlessly grow with your evolving data demands. Pricing Evaluate the pricing structure to ensure it aligns with your usage patterns to avoid any unforeseen costs. Beyond per-query or per-GB pricing, assess the total cost of ownership (TCO) and remember to account for factors like storage costs and data transfer expenses. Taking the bigger picture into account will ensure that the chosen cloud data warehouse not only meets your immediate budgetary considerations but also proves economically sustainable in the long run. Vendor Lock-in Prioritizing multi-cloud support contributes to a resilient and adaptable data stack. Evaluate the cloud data warehouse solution’s capability to seamlessly deploy across multiple cloud providers, ensuring flexibility in choosing and transitioning between services. This not only mitigates the risks associated with dependence on a single vendor but also provides the ability to leverage the unique offerings of different cloud environments. Vendor Support A responsive and reliable vendor support system is crucial for timely issue resolution and ensuring that your team can leverage the full potential of the cloud data warehouse. Evaluate factors such as response times and the availability of support plans. Additionally, consider looking at the platform’s community engagement and the quality of available documentation, as these resources often prove invaluable in navigating challenges and optimizing usage. How LIKE.TG Can Facilitate Your Move to the Cloud Adopting a cloud data warehouse for your organization is a big decision. In addition to training and preparing your employees for the move, you’ll also have to ensure everyone involved in the migration process is well-versed in the intricacies of the selected platform, as well as the migration process itself. This is exactly where LIKE.TG comes in with its Data Warehouse Builder—a unified, metadata-driven data warehouse solution. With LIKE.TG, you can: Build a full-fledged data warehouse from scratch in a matter of days, not weeks Deploy high-volume, fully operational data warehouses both on-premises and cloud Automate the data vault modeling process to create hubs, links, and satellites Connect to BI and analytics tools seamlessly for reporting and in-depth analyses And much more—all without writing a single line of code. Ready to leverage the benefits of a cloud data warehouse? Get in touch with one of our experts today. Alternatively, you can download a 14-day free trial or view demo.
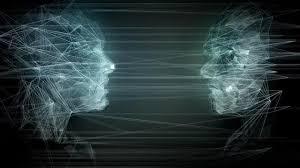
The Importance of Data Preparation for Machine Learning
Machine Learning (ML) focuses on developing algorithms and models that enable computers to learn from and make predictions or decisions based on data. It encompasses various techniques, such as supervised learning, unsupervised learning, reinforcement learning, and more. In ML, getting accurate results depends on having clean and well-organized data. That’s where data preparation comes in. It’s the process that ensures the data is in the best possible shape for making reliable predictions and gaining meaningful insights. Data scientists commit nearly 80% of their time to data preparation, but only 3% of company data fulfills basic data quality standards. This highlights the critical importance of investing in data quality and efficient data preparation processes; they form the foundation for successful machine learning projects. Data Preparation’s Importance in ML A machine learning model’s performance is directly affected by data quality. Let’s explore what happens if the data is not prepared thoroughly: Compromised Model Accuracy: Machine learning models rely on data patterns. Inaccurate data leads to models built on ‘dirty’ data, resulting in off-the-mark predictions. This can result in both compromised accuracy and increased costs. For instance, a healthcare model trained on unclean data may show an impressive 95% accuracy rating during testing, but when deployed in real healthcare settings, it could fail to diagnose critical conditions. Compounding Errors: In interconnected systems where outputs from one model feed into another, poor data quality can lead to compounding errors. This cascading effect can result in large-scale inaccuracies, especially in integrated digital ecosystems or complex supply chains. Biased Models and Ethical Concerns: When models learn from biased data, they mirror and exacerbate these biases, raising ethical concerns. In areas such as hiring or lending, this perpetuates unfair practices. For example, a hiring algorithm trained on historically biased data might consistently discriminate against qualified candidates from certain demographics. How To Effectively Prepare Data for Machine Learning Machine learning model efficiency hinges on data quality. Let’s explore key steps of data preparation for machine learning to ensure that the models yield reliable and actionable insights. Problem Identification and Understanding First, you must have a comprehensive understanding of your goals, desired outcomes, and any constraints or limitations. With a clear objective you can easily identify which data features are vital and extraneous for the model’s training. Additionally, the nature of the problem inherently dictates the standard for data quality. For instance, a machine learning model tasked with predicting stock prices needs a higher level of data precision than one designed to suggest movie recommendations. Data Collection Next is gathering relevant data that can feed into our machine learning model. This process might involve tapping into internal databases, external datasets, APIs, or even manual data logging. It’s crucial at this stage to ensure data diversity and comprehensiveness in order to safeguard against potential biases and ensure a representative sample. Data Exploration This phase involves summarizing key statistics, creating visual representations of the data, and identifying initial patterns or outliers to check for data quality issues such as duplicates, inconsistent data types, or data entry errors. Data Cleaning Data cleaning focuses on sifting through the data to identify and rectify imperfections in the dataset. It involves tasks like handling missing data, detecting and handling outliers, ensuring data consistency, eliminating duplicates, and correcting errors. This step is crucial as it lays the foundation for reliable insights and ensures that machine learning models work with accurate, high-quality data. Data Transformation Once the data is clean, it might still not be in an optimal format for machine learning. Data transformation involves converting the data into a form more suitable for modeling. This can entail processes like normalization (scaling all numerical variables to a standard range), encoding categorical variables, or even time-based aggregations. Essentially, it’s about reshaping data to better fit the modeling process. Feature Engineering With the data transformed, the next step is to delve deeper and extract or create features that enhance the model’s predictive capabilities. Feature engineering might involve creating interaction terms, deriving new metrics from existing data, or even incorporating external data sources. This creative process involves blending domain knowledge with data science to amplify the data’s potential. Data Splitting Lastly, once the data is prepared and enriched, it’s time to segment it for the training and validation processes. Typically, data is split into training, validation, and test sets. The training set is used to build the model, the validation set to fine-tune it, and the test set to evaluate its performance on unseen data. Proper data splitting ensures the model isn’t overfitting to the data it’s seen and can generalize well to new, unseen data. Data Preparation with LIKE.TG LIKE.TG has exceptional data preparation capabilities for organizations seeking to harness the power of clean, well-prepared data to drive insightful machine-learning outcomes. LIKE.TG not only provides real-time data health visuals for assessing data quality but also offers an intuitive point-and-click interface with integrated transformations. This user-friendly approach makes data preparation accessible to individuals without extensive technical expertise. Let’s look at how LIKE.TG streamlines the process of data preparation for machine learning models: Data Extraction LIKE.TG excels in data extraction with its AI-powered capabilities that allow you to connect seamlessly with unstructured sources. This feature ensures that even data from unconventional sources can be effortlessly integrated into your machine learning workflow. Data Profiling LIKE.TG’s preview-centric UI provides a detailed preview of your data, enabling you to explore and understand your data better before the actual preparation begins. Real-time data health checks ensure you can spot issues immediately and address them proactively. Data Cleansing LIKE.TG offers advanced data cleansing capabilities, including the removal of null values, find-and-replace operations, and comprehensive data quality checks. Additionally, its “Distinct” action ensures that your data is clean and free from redundancies, making it ideal for machine learning applications. Data Transformation LIKE.TG’s visual, interactive, no-code interface simplifies data transformation tasks. You can perform actions like normalization, encoding, and aggregations using point-and-click navigation, making it easy to reshape your data to suit the requirements of your machine-learning models. Ready to optimize your data for machine learning success? Download LIKE.TG’s 14-day free trial today and experience the power of effective data preparation firsthand! Enhance Your ML Models With Trustworthy Data Leverage the power of clean, reliable and well-prepared data to elevate ML model performance in LIKE.TG's no-code environment. Download 14-Day Free Trial
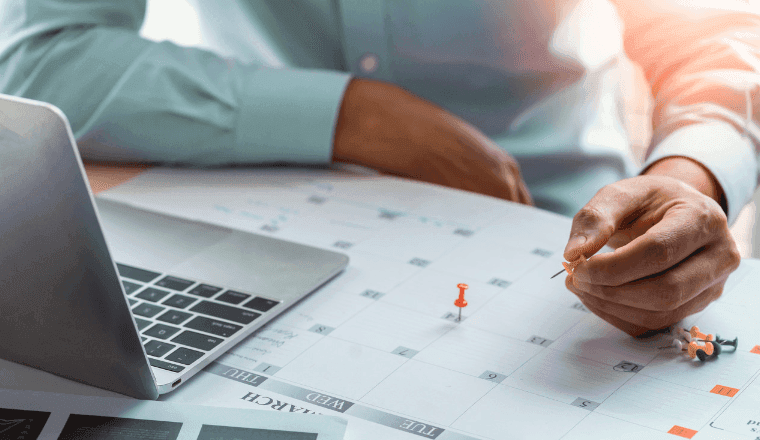
6 Crucial Questions to Help You Prepare Your Data For Analysis
Alexander Graham Bell’s motto, ‘Preparation is the key to success,’ resonates powerfully in data analysis. Raw data is an intricate puzzle—complex, unstructured, and scattered. Data preparation helps unravel the intricacies, transforming chaos into clarity. It involves refining, structuring, and formatting raw data, setting the stage for insightful analysis. By preparing data for analysis, organizations can eliminate errors, ensure completeness and accuracy, and reveal hidden patterns, driving accurate analysis and informed decision-making. The significance of this preparatory phase cannot be emphasized enough; it’s the crucial step that paves the way for actionable intelligence. Questions to Ask When Preparing Data for Analysis As organizations delve into data preparation, they should consider the following questions to ensure their data is primed for analysis. What is the main issue the analysis aims to solve? Organizations must define the underlying business problem the analysis intends to address. This ensures that data preparation is laser-focused, aligning every subsequent step with the ultimate goal. Whether it’s optimizing supply chains, predicting customer behavior, or enhancing marketing strategies, a well-defined goal serves as a compass, guiding the course of data preparation endeavors. Is the data accurate, complete, and suitable for the project? Data quality is the foundation of reliable analysis. Anomalies and inconsistencies can lead to incorrect conclusions. Rigorous data cleansing and validation procedures are required to ensure consistency. The dataset should also be evaluated for accuracy, completeness, and relevance. How are missing values and outliers being managed? Addressing missing values and outliers is crucial for analysis integrity. Analysts should decide whether to omit, impute, or transform missing values and devise strategies for managing outliers that could potentially impact results. Proficiently handling these anomalies ensures dependable and accurate insights, a cornerstone for meaningful analysis. Will any variables require transformation? Data seldom presents itself in the ideal format for analysis. Variables may need to be transformed, normalized, or standardized to ensure meaningful comparisons and interpretations. The specific analytical goals and the underlying assumptions of the chosen analysis method should guide decisions regarding which variables to transform and how to do so. Which documentation procedures ensure transparency? Transparent documentation acts as a breadcrumb trail, guiding collaborators through the analytical process. Each step, from data cleaning to transformation, should be documented systematically. This documentation fosters accountability and enables others to follow and validate the approach, ensuring a transparent and replicable analysis. How will the data preparation process be validated? Validation serves as the litmus test for the data preparation process. Analysts must establish validation strategies to ascertain that the data is accurately transformed and manipulated. This often involves cross-validation techniques, comparing outcomes against established benchmarks, or conducting sensitivity analyses to assess the impact of various data preparation decisions. The Importance of Preparing Data for Analysis Embarking on data analysis without proper preparation sets up organizations for unreliable, misleading results. Here’s why preparing data for analysis is crucial: Reliable Insights Depend on Clean Data Well-prepared data is crucial for business intelligence. The conclusions drawn will be inaccurate if the data is messy or inaccurate, and vice versa. Data preparation ensures the data is accurate, consistent, and trustworthy, forming a solid basis for insightful findings. It includes data cleansing, validation, and imputation, which collectively enhance the reliability of the conclusions drawn from the information. Noise Reduction and Outlier Handling Real-world data often contains irregularities like noise and outliers that can distort analysis results. Analysts can prevent outliers from skewing the overall conclusions by addressing them carefully. This process helps to focus the analysis on meaningful trends and patterns. Noise reduction techniques, such as smoothing and filtering, help distinguish genuine patterns from random fluctuations. Outlier handling means identifying extreme data points that can unduly influence statistical measures and making informed decisions about their treatment. Drawing Meaningful Comparisons Variations in units, scales, or formats can hinder fair comparisons when dealing with data from different sources or timeframes. Data preparation standardizes these aspects, making it possible to draw accurate conclusions by ensuring consistency across the dataset. Standardization ensures that data from diverse sources are transformed into a consistent format, facilitating meaningful comparisons. This step often includes converting units, harmonizing naming conventions, and aligning scales to create a unified dataset. Time Savings A significant portion of the analysis process is wrangling and cleaning data. Investing effort in data preparation upfront reduces troubleshooting during analysis, making the entire process more efficient. Effective data preparation also saves valuable time in later stages of analysis by minimizing the need for repeated data fixes. It empowers analysts to focus on exploring insights and crafting meaningful visualizations, thereby enhancing the overall efficiency of the analytical workflow. Guarding Against Bias Preparing data for analysis presents an opportunity to detect and correct biases in the data. By addressing these biases proactively, enterprises can minimize their impact on the analysis results, ensuring a more objective and accurate interpretation. Data scientists detect bias by examining the data for potential sources of bias, such as underrepresented groups or skewed samples. Data cleaning and transformation techniques can be applied to mitigate these biases, ensuring that the analysis provides a fair and unbiased representation of the underlying phenomena. Boosting Model Performance For predictive modeling to succeed, well-prepared data is essential. Techniques like normalization and feature engineering, part of data preprocessing, enhance the accuracy and robustness of models, leading to more reliable predictions. Data preprocessing transforms raw data into a suitable format for machine learning algorithms. Normalization scales data to a consistent range, and feature engineering involves creating new relevant features from existing ones. Both steps contribute to improved model performance and more accurate predictions. Point-and-click Data Prep With LIKE.TG Organizations are increasingly recognizing the importance of well-prepared data in decision-making- be it strategic or tactical, to gain a competitive advantage. It is crucial for analysts to carefully assess and evaluate the information available and take the necessary steps to prepare the data for analysis. Accurate, complete data paves the way for actionable insights, strategic outcomes, and informed choices, consequently enhancing the operational efficiency, profitability, and, ultimately, the bottom line of enterprises. Ready to elevate your data game? Dive into the future with a 14-day free trial of LIKE.TG and unlock the potential of seamless data preparation for your organization. Prepare your data in seconds with LIKE.TG’s intuitive interface and robust data profiling and cleansing features.
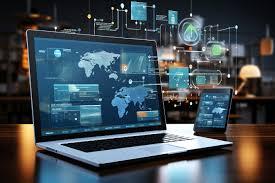
Data Governance in Financial Services: How to Ensure Data Integrity for Risk Management and Reporting
Introduction As financial institutions navigate intricate market dynamics and heighten regulatory requirements, the need for reliable and accurate data has never been more pronounced. This has spotlighted data governance—a discipline that shapes how data is managed, protected, and utilized within these institutions. Data governance is vital in maintaining the accuracy, consistency, and reliability of financial information. Data integrity is a foundational pillar within data governance, specifically in risk management and reporting. Maintaining the integrity of financial data is essential for facilitating effective risk identification, assessment, and mitigation strategies. Plus, accurate and trustworthy reporting is critical for regulatory compliance, investor confidence, and informed decision-making. As the financial services sector grapples with an ever-expanding volume of data and increasingly stringent compliance demands, the need for a holistic approach to data governance is undeniable. This blog delves into data governance in financial services, focusing specifically on how it ensures data integrity for risk management and reporting. Regulatory Landscape and Compliance Requirements in Financial Services Data governance and compliance are related but distinct concepts. Data governance focuses on managing and using data within an organization, while compliance focuses on adherence to laws, regulations, and data management and privacy standards. The financial services industry is among the most heavily regulated worldwide, and data governance is a critical component of regulatory compliance. Regulatory bodies like the Securities and Exchange Commission, Financial Industry Regulatory Authority (FINRA), and the European Banking Authority (EBA) impose stringent regulations to protect investors, maintain market stability, and ensure fair practices. In addition to data privacy regulations, financial services organizations must comply with specific industry standards and regulations. For example, Basel III requires banks to establish robust data governance frameworks for risk management, including data lineage, data validation, and data integrity controls. Also, the Consumer Protection Act and Dodd-Frank Wall Street Reform require accurate and timely reporting of financial transactions and positions. Data governance plays a vital role in supporting compliance efforts by providing a structured approach to data management. Organizations must navigate regulations related to data privacy, data protection, information security, and reporting standards. For instance, in the European Union, the GDPR imposes strict requirements on collecting, processing, and protecting personal data. By understanding the regulatory landscape and compliance requirements specific to the financial industry, organizations can design and implement data governance frameworks that address these regulations effectively. Effective data governance is essential in the financial services industry to ensure data integrity for risk management and reporting. This requires a clear understanding of data governance’s definition and core principles, the regulatory landscape, and compliance requirements specific to the financial industry. Financial institutions should prioritize developing robust data governance frameworks that align with regulatory requirements, enhance transparency, and support compliance. Understanding Data Governance in Financial Services Data Governance Data governance is an approach for specifying who within an organization controls data assets and how those data assets can be used. It is a critical component of financial services, ensuring that data is managed and protected to meet regulatory requirements and support effective risk management and reporting. Core Principles of Data Governance Data governance operates based on a set of core principles: Accountability: In financial services, data governance establishes accountability by assigning ownership for data tasks, ensuring accuracy and transparency. This structure prevents data quality issues, enhances decision-making, and enables compliant operations. Transparency: Data governance mandates transparent communication about data usage in the financial sector. This encompasses well-defined data policies, accessible standards, and procedures that resonate with stakeholders. Data Quality: Data governance prioritizes accurate, complete, and consistent data. Through defined quality metrics and controls, institutions uphold data integrity, facilitating informed financial decisions and regulatory compliance. Compliance: Compliance is critical for financial firms, and data governance aligns with regulatory requirements and industry standards specific to the financial services sector. It enables organizations to demonstrate compliance with regulations such as the General Data Protection Regulation (GDPR), Basel III, or the Sarbanes-Oxley Act (SOX). Collaboration: Robust governance drives cross-departmental collaboration, promoting communication and informed decision-making. It unites stakeholders, facilitating cohesive data management that enhances operational efficiency and compliance. Standardization: Standardization within data governance ensures uniform data practices, improving reporting accuracy and informed decisions. This minimizes errors, enhances compliance, and strengthens operational coherence across the financial landscape. Risks Associated with Poor Data Governance Poor data governance introduces significant risks to financial institutions, jeopardizing their ability to manage risks and report accurate information effectively. Here are some risks that highlight the importance of robust data governance: Data Inaccuracy and Inconsistency: Without proper data governance, errors, inconsistencies, and inaccuracies can arise in the data being used for risk management and reporting. These inconsistencies can undermine the accuracy of risk models, compromise decision-making, and lead to incorrect risk assessments. Data Breaches and Security Risks: Inadequate data governance practices increase the vulnerability of financial institutions to data breaches and security risks. Poor data security measures, lack of access controls, or inadequate encryption protocols can expose sensitive financial information to unauthorized access, leading to financial fraud, identity theft, and regulatory non-compliance. Compliance and Regulatory Risks: Financial institutions function in a regulated setting. Poor data governance can result in non-compliance with regulatory requirements, leading to penalties, reputational harm, and legal repercussions. Inaccurate or incomplete data can also hinder the ability to meet reporting obligations, further increasing compliance risks. Reiterated compliance breaches can even put corporations out of business. Key Components of Data Governance for Risk Management and Reporting Effective data governance serves as a critical framework for ensuring data integrity in risk management and reporting in the financial services industry. It encompasses several key components that collectively contribute to data reliability, accuracy, and consistency. Data Sourcing and Acquisition Data sourcing and acquisition are the first steps in data governance for risk management and reporting. It involves identifying, collecting, and integrating data from various internal and external sources. Financial institutions must establish robust mechanisms to ensure the reliability and relevance of data sources. This includes evaluating data providers, implementing data validation processes, and verifying the accuracy and completeness of acquired data. Accurate data sourcing and acquisition are critical for obtaining reliable data supporting decision-making and risk analysis. Examples: A global investment firm utilizes data governance practices to validate and onboard data from multiple market data vendors. By implementing rigorous data sourcing and acquisition procedures, the firm ensures the accuracy of pricing data, market indices, and other critical financial information used in risk management and reporting. Credit rating agencies may use data from credit bureaus to evaluate creditworthiness and assign credit ratings to borrowers. Data Quality Management Data quality management involves monitoring and controlling data quality to ensure its suitability for the intended purpose. It focuses on maintaining high data accuracy, completeness, consistency, and timeliness standards. Financial institutions require high-quality data to accurately identify, assess, and mitigate risks. By establishing data quality frameworks, financial institutions can detect and rectify data anomalies and discrepancies, ensuring the reliability and usability of their data assets. Example: A commercial bank leverages data governance principles to establish data quality rules and automated data validation processes. The bank’s data governance framework enforces data completeness checks and performs data quality assessments to identify and resolve any inconsistencies or errors in customer transactional data. This ensures accurate risk assessment and reliable reporting for regulatory compliance. Data Lineage Data lineage refers to the tracing of the lifecycle of data, including its origin, transformation, and usage over time. It clearly explains how data is derived, manipulated, and utilized within an organization. It enables financial institutions to establish data provenance, track data dependencies, and ensure the reliability and integrity of data for risk management and reporting purposes. Example: A banking regulator may track the lineage of a particular financial instrument throughout its lifecycle to assess the financial institution’s risk exposure. A credit risk manager may track the lineage of a portfolio of loans to identify potential issues, such as aging loans, non-performing loans, or loans with documentation errors. Metadata Management Metadata management involves capturing and managing descriptive information about data assets. It includes metadata creation, documentation, and maintenance, allowing stakeholders to understand the context, structure, and meaning of data elements. Effective metadata management supports data comprehension, data integration, and data sharing. It improves data quality by enabling risk managers to identify issues or discrepancies in data attributes. Example: A financial regulatory agency employs data governance principles to establish comprehensive metadata management practices. The agency ensures consistent interpretation and accurate reporting across financial institutions by documenting the metadata associated with regulatory reporting data, including data definitions, business rules, and reporting requirements. Data Access and Security Data access and security are the processes and procedures that govern how data is accessed, used, and protected. Financial institutions must implement robust access controls, encryption mechanisms, and data security protocols to safeguard sensitive financial information. Data governance plays a crucial role in defining data access policies, monitoring data usage, and maintaining compliance with data protection regulations. Example: A wealth management firm may use role-based access control to ensure clients’ financial information is only accessible to authorized financial advisors. A central bank may implement data encryption protocols to protect sensitive data from unauthorized access, reducing the risks of data breaches or cyberattacks. Data Governance Frameworks Data governance frameworks refer to the policies, procedures, and guidelines that govern data management within a financial institution. A comprehensive data governance framework is essential in ensuring that all components of data governance are working together efficiently to support risk management and reporting. Example: A multinational investment bank adopts a comprehensive data governance framework that encompasses data governance policies, data stewardship roles, and cross-functional data governance committees. The framework provides a structure to enforce data standards, resolve data-related issues, and ensure data integrity in risk management and reporting across the organization. By incorporating these key components of data governance for risk management and reporting, financial institutions can establish robust data governance practices that enhance data integrity, mitigate risks, and enable accurate and reliable reporting. The Importance of Data Integrity in Risk Management and Reporting In the world of finance, it is important to keep data accurate and trustworthy, especially when dealing with risks and reports. Imagine data as the building blocks for making smart decisions and following rules. Having reliable data is like having a strong base for understanding risks and creating honest reports. Importance of Reliable and Accurate Data for Risk Management Risk management in financial services relies heavily on high-quality and trustworthy data availability. Accurate and reliable data forms the foundation of robust risk management practices within financial institutions. Here’s why data integrity is crucial: Informed Decision-Making: Sound decision-making requires access to accurate, timely, and relevant data. Reliable data allows risk managers to identify, assess, and quantify potential risks, enabling them to make informed decisions. Risk Identification and Assessment: Reliable data is critical for identifying and assessing risks. It enables financial institutions to correctly identify potential threats, analyze their impact, and formulate effective strategies to mitigate them. Scenario Analysis and Stress Testing: Scenario analysis and stress testing are vital tools for risk management. Accurate data ensures that financial institutions can simulate various scenarios, test the resilience of their portfolios, and evaluate their exposure to different risk factors accurately. Potential Consequences for Financial Institutions Financial institutions that neglect data governance and overlook data integrity face several potential consequences. The consequences include: Financial Losses: Imagine a company relying on inaccurate risk assessments to invest in a project. The flawed data might lead them to make a bad investment, resulting in significant financial losses. For instance, a real estate developer might misjudge the market trends and invest in an area that doesn’t yield the expected returns, causing financial setbacks. Reputational Damage: Picture a bank reporting incorrect financial figures due to data errors. This misinformation can damage its reputation, causing clients and investors to doubt its credibility. Regulatory Penalties: Consider a credit card company failing to comply with data protection regulations, leading to a data breach exposing customers’ information. Regulatory bodies can impose heavy fines on the company for not safeguarding data properly. Increased Operational Costs: Think of an insurance company struggling with inaccurate data records. This might lead to delays in claim processing and customer dissatisfaction. The extra effort required to rectify errors and handle customer complaints increases operational costs. Ensuring Data Integrity in Financial Services High-quality data is essential for regulatory compliance, effective risk management, and delivering exceptional financial services to customers. In the financial services sector, ensuring data integrity is crucial for regulatory compliance. Financial firms must maintain high-quality data to accurately assess risks and report on transactions. Failure to do so can result in costly fines, reputational damage, and criminal liability. Challenges of Ensuring Data Integrity in Financial Services Ensuring data integrity in financial services comes with its own set of challenges. These challenges include: Data Volume and Complexity: Financial Firms manage vast volumes of complex data from multiple sources, including structured and unstructured data. This complexity makes it more challenging to ensure data consistency and accuracy. Data Integration and Transformation: Financial data often originates from disparate systems and must be integrated and transformed into a unified format for analysis and reporting. This process introduces risks such as data inconsistencies, errors in data mapping, and loss of data fidelity. Data Quality Issues: Poor data quality, including data duplication, incomplete records, and inaccuracies, can undermine the integrity of financial data. Ensuring data quality is a continuous challenge, particularly with the constant influx of new data and the need to maintain historical data accuracy. Data Governance Frameworks: Financial institutions must establish a data governance framework aligning with the organization’s goals and objectives. Financial firms need robust data governance practices to address these challenges and ensure data integrity. Best Practices for Data Governance Contributing to Data Integrity Robust data governance practices are crucial to address these challenges and ensure data integrity in financial services. Some best practices include: Data Validation: This involves using rules and algorithms to verify that data is accurate, complete, and consistent. Validation can happen at various stages of the data lifecycle, such as data entry, data processing, and reporting. Reconciliation: This is the process of comparing data from different sources or systems to identify and resolve discrepancies. Reconciliation helps ensure that data is accurate and complete, even when it comes from various sources. Data Quality Controls: These are the processes and tools used to ensure that data meets specific quality standards. Examples include data profiling, data cleansing, and data enrichment. Metadata Management: Implementing comprehensive metadata management practices to capture and document information about data sources, definitions, and business rules, enabling a better understanding of data context and lineage. By adopting these best practices and leveraging data governance frameworks, financial services organizations can ensure data integrity, meet regulatory compliance requirements, and enhance their risk management and reporting capabilities. Implementing Data Governance for Data Integrity This section will discuss the key steps and considerations involved in implementing data governance for data integrity. Additionally, we will discuss the significance of ongoing monitoring, auditing, and continuous improvement of data governance practices. 6 Steps to Implement Data Governance for Data Integrity Define Objectives and Scope: Identify the specific areas within risk management and reporting that require data governance focus, such as data sourcing, data quality management, or data lineage. Secure Executive Sponsorship and Organizational Buy-In: Obtain support from senior executives who can champion the data governance initiative. Executive sponsorship is crucial for allocating resources, driving organizational change, and fostering a data-driven culture across the organization. Establish Data Governance Frameworks: Develop data governance frameworks that outline the policies, processes, and procedures for managing data integrity. Define data governance principles, standards, and guidelines specific to risk management and reporting. Align the frameworks with industry regulations and best practices. Define Data Ownership: Define data ownership for different domains and data sets. Assign accountability to individuals or teams who will be responsible for the quality, accuracy, and integrity of specific data elements. Data owners should collaborate with data stewards to ensure adherence to data governance policies and practices. Assign Roles and Responsibilities: Identify key roles and responsibilities such as data stewards, data custodians, and data governance committees. Clearly define their roles, decision-making authority, and responsibilities to ensure effective data governance implementation. Develop Data Governance Policies and Standards: Establish data governance policies and standards that address data sourcing and acquisition, data quality management, data lineage, metadata management, data access, and security. These policies and measures should align with regulatory requirements and industry best practices. Ongoing Monitoring, Auditing, and Continuous Improvement Data governance is not a one-time implementation process; it requires continuous monitoring, auditing, and improvement. Regular monitoring helps identify any deviations from established data governance processes and enables prompt corrective actions. Continuous improvement involves regularly reviewing and enhancing data governance practices to align them with changing business requirements, regulatory changes, and technological advancements. Encourage feedback from data stakeholders, data users, and compliance teams to identify opportunities for refinement and enhancement. By prioritizing ongoing monitoring, auditing, and continuous improvement, financial institutions can ensure that data governance practices for data integrity remain effective, responsive to changing requirements, and aligned with the organization’s risk management and reporting needs. Technology Solutions for Data Governance In the era of rapidly advancing technology, leveraging the right tools and platforms is crucial for successful data governance initiatives in financial services. Technology plays a critical role in supporting data governance initiatives, especially in financial services, where vast amounts of data are managed, analyzed, and reported daily. Technology solutions can provide the necessary tools and platforms to ensure data integrity and improve risk management and reporting. Several data management tools and platforms are available to ensure data integrity within financial services organizations. These include: Master Data Management (MDM) Systems: MDM systems provide a centralized and consistent view of critical data entities, such as customers, products, and counterparties. These systems help establish data governance practices around standardization, validation, and stewardship. Data Quality Management Tools: Data quality management tools enable organizations to assess, monitor, and improve the quality of their data. These tools help identify data anomalies, perform data cleansing, and establish data quality controls to ensure data integrity. Data Governance Platforms: Dedicated data governance platforms offer comprehensive capabilities to manage and govern data assets. These platforms facilitate the enforcement of data governance policies, provide workflows for data stewardship, and enable collaboration across data governance teams. Incorporating technology solutions, such as data management tools, data governance platforms, and advanced analytics, strengthens data governance practices and ensures data integrity for risk management and reporting in financial services. When implemented effectively, technology solutions contribute to efficient data management, accurate decision-making, and compliance with regulatory requirements. Conclusion Data governance is a critical driver for data integrity in financial services. It establishes accountability, ensures compliance, and fosters a data-driven organizational culture. By implementing data governance best practices, financial institutions can strengthen their risk management capabilities, enhance reporting accuracy, and maintain regulatory compliance. In light of these insights, organizations in the financial services industry need to prioritize data governance. A call to action is necessary to recognize the value and urgency of implementing effective data governance practices. By doing so, organizations can ensure the integrity of their data, mitigate risks effectively, and drive better decision-making processes. Looking ahead, the future of data governance in financial services holds great promise. As technology advances, opportunities for leveraging data analytics, AI, and automation will expand. Data governance frameworks will evolve to adapt to emerging regulatory requirements and industry standards. Organizations must stay updated with the latest developments in data governance practices and embrace continuous improvement to ensure data integrity and remain competitive in the evolving financial landscape. The journey toward effective data governance starts now.
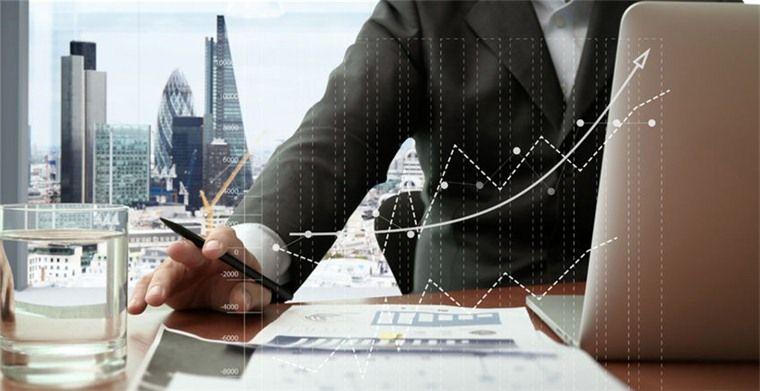
A Complete Guide to Data Enrichment
Businesses rely heavily on data to make informed decisions, understand customers and facilitate growth. However, the quality and depth of data can vary significantly, often leaving raw data insufficient to yield effective outcomes. Data enrichment can help you meet these expectations, enrich customer experiences, optimize cost-efficiency, and eliminate redundant data. What is Data Enrichment? Data enrichment is a process that enhances raw data with additional context, making it more useful for business insights and decisions. It allows you to add new data or add supplementary information such as demographic details, and behavioral insights to provide a more accurate, actionable, and complete dataset. Data enrichment further offers the ability to cross-reference, refine, and weave together data from multiple sources to make a unified whole. The result is a unified, all-encompassing dataset that reveals previously concealed insights and opportunities so you can make educated and strategic decisions. Elevate Your Data Quality, Zero-Coding Required High quality data is the backbone of data-driven companies. Clean and validate your unstructured data in a code-free interface with LIKE.TG. Automate your data prep now! View Demo Examples of Data Enrichment Data enrichment can take various forms. For example, customer data enrichment focuses on upgrading customer-related data with additional information to reach a wider audience and provide a more personalized experience.For example: Customer Insight Amplification: By appending social media profiles to existing customer contact information, businesses can gain a more comprehensive view of customer preferences and behaviors. Market Trend Integration: Incorporating current market trends into sales data can help companies anticipate shifts in consumer demand and adjust their strategies accordingly. Transaction History Enrichment: Enriching customer records with recent transaction history can provide valuable context for personalized marketing and improved customer service. Importance of Data Enrichment Trusted data is key for businesses to make informed decisions. Raw data often comes with inconsistencies and lacks depth because of data silos. Cleaning, standardizing, enriching, and verifying this raw data offer multiple benefits for organizations and their customers. Breaking Down Data Silos Data integration from multiple sources provides a unified data view, enabling the identification of hidden trends or patterns. These trends can be further used to predict customer behavior and optimize marketing campaigns. Giving Context to the Raw Data Giving a proper context to a raw data set is essential to extract meaningful insights. Customer data enrichment provides missing context by linking the data with relevant information and for instance, linking demographics and purchasing habits to social media activity or location-based behavior patterns. By adding context, organizations can gain a richer understanding of the data. Improved Customer Understanding Data enrichment provides context to the raw data and combines the existing customer data with external sources or third-party data. Enriching customer data helps organizations better understand their customer base, like their brand affinities and preferences. Improved Response Time Businesses that rely on immediate and emergency responses understand the importance of accurate and readily available data. Data enrichment gives response teams more information to plan and execute the response. Data Cleansing vs. Data Enrichment Data cleansing and data enrichment serve distinct but complementary roles in data management. Both concepts are indispensable to the data preparation process. Data cleansing is primarily concerned with the accuracy and consistency of data within a dataset. It consists of processes that help you correct errors, remove duplicates, and ensure that your data is updated. This is akin to tidying up a room to be clean and orderly. On the other hand, data enrichment is about adding value to the existing dataset by appending new, relevant information from external sources. If data cleansing is about cleaning the room, data enrichment is about furnishing it with new, valuable items that enhance its functionality and appeal. Data Enhancement vs. Data Enrichment While data enhancement and enrichment are often used interchangeably, they have subtle differences. Data enrichment typically refers to the process of improving, refining, or enhancing existing data by adding additional information or attributes to it. This can involve appending data from external sources to enrich the original dataset. For example, if you have a customer database with names and email addresses, data enrichment might involve adding demographic information such as age, gender, or location to provide a more comprehensive profile of each customer. On the other hand, data enhancement is a broader term encompassing various methods and techniques to improve the quality, usability, or value of data. While data enrichment is one way to enhance data, other methods exist. These may include data cleaning to remove errors or inconsistencies, data normalization to ensure consistency across different datasets, or data integration to combine multiple datasets into a single, unified view. Both processes aim to increase the value of your data but approach it from different angles. Data Enrichment Data Enhancement Purpose Enhancing existing data by adding additional information to make it more comprehensive and valuable. Improving overall data quality, usability, and value through various methods and techniques. Process – Identifying relevant external data sources. – Acquiring additional data from external sources. – Integrating new data with existing dataset. – Matching and merging new data with existing data. – Updating and validating enriched data. – Data cleaning: Removing errors, duplicates, etc. – Data normalization: Ensuring consistency across different datasets. – Data integration: Combining multiple datasets into a unified view. – Data enrichment: Adding additional information to existing data. Results – Comprehensive and enriched dataset. – More detailed insights and analysis. – Better understanding of customers or entities. – Improved data quality and accuracy. – Enhanced usability and accessibility of data. – Increased value and utility of data for decision-making and analysis. Benefits of Data Enrichment The data enrichment solution market will touch $3.5 billion by 2030, which is huge. The popularity of these solutions can be attributed to the many benefits that come with data enrichment. Here are some of the most prominent benefits of data enrichment: Informed Decision Making: Enriched data gives decision-makers a complete picture so they can make informed decisions, whether it is regarding new product creation or market expansion. Improved Customer Profiling: This is the age of hyper-personalization. Data enrichment can add demographic details like age, gender, income, and marital status to customer profiles so you can subsequently tailor your marketing efforts. For instance, a clothing retailer can use age and gender data to recommend relevant clothing styles to their customers. Competitive Advantage: Enriched data enables organizations to stay ahead of the competition by identifying market trends, understanding customer preferences, and adapting to changing market conditions. Cost Reduction: The best part about data enrichment is that it saves you tons of costs because then you can avoid costly errors. For example, if you have a database of customers, you can enrich the data with the right information and ensure that your marketing materials reach the right addresses. Data Enrichment Techniques So, how can you enhance the quality of your data and ensure it provides value? Techniques include: Data Consolidation: This technique can add new data fields or attributes to existing records. For instance, you can enrich a customer database with demographic information such as age, gender, and income. Data Validation and Correction: One of the most critical factors in data enrichment is ensuring the accuracy and integrity of data by validating and correcting inconsistencies, errors, or missing values, for example, validating email addresses, fixing formatting issues in addresses, or standardizing date formats. Geospatial Enrichment: You can add geographic data to records, such as latitude and longitude coordinates, city, state, or ZIP code. So, if you must do a location-based analysis, this can be useful in that scenario. Social Media Profiling: To gain a deeper understanding of your customers, you can enrich customer data with information from social media platforms, such as Twitter, LinkedIn, or Facebook profiles. Data Standardization: Standardizing data involves converting data into a consistent format. This can include standardizing units of measurement, date formats, or address formatting (e.g., converting “St.” to “Street”). Data Matching and Deduplication: Duplicate records are a common issue with raw data. Data matching identifies and merges duplicate records within a dataset to ensure data consistency and eliminate redundancy. 9 Steps for Data Enrichment Let’s walk through an example of data enrichment in the financial industry. If you are a bank manager who wants to enrich customer data to improve service personalization, you should follow these steps. Step 1: Data Collection and Preparation First, determine the dataset and data elements you want to enrich. Collect and compile them and make sure they are clean and free of obvious errors. You can leverage data integration tools to access and consolidate customer databases and transaction logs. Doing so will help you gain access to data connectors while supporting data type conversions and error logging to identify and rectify discrepancies. Step 2: Define Data Enrichment Goals Clearly define the objectives of your data enrichment project. What specific enhancements are you looking to achieve? Are you interested in demographic data, geospatial information, or other attributes? In the example, you aim to enrich customer financial behavior data with additional attributes such as investment patterns, risk tolerance, and preferred transaction channels to offer more personalized services. Step 3: Clean Data Once you have laid the groundwork, initiate your data enrichment journey by meticulously tending to data cleanliness and consistency. Data cleansing allows you to eliminate any duplicates, rectify inaccuracies, and standardize data formats. For instance, aligning customer phone numbers to a uniform format makes it usable across systems. Step 4: Segment and Profile Data Utilize the power of data segmentation and profiling to divide your dataset into meaningful segments guided by specific criteria. Data profiling will give you a complete picture of your data. It will assess the quality of your data by looking for inconsistencies, errors, and outliers and identify the data that doesn’t conform to expected patterns or business rules. For example, data profiling tools will help you detect inconsistencies in the customer data stored by your bank. It will also allow you to segment customers based on transactional behavior, product usage, and interaction channels. For instance, you might create segments such as ‘High-Value Customers,’ ‘Frequent Online Users,’ ‘Loan Seekers,’ or ‘Young Professionals.’ Step 5: Match and Integrate Data Data integration involves matching and merging records based on shared identifiers, reconciling data from multiple sources, and creating a cohesive dataset as a single source of truth. The integrated dataset will set the foundation for advanced analytics, reporting, and strategic planning. Data matching tools will help you merge related records across different systems using unique identifiers like customer IDs. This helps create a unified view of each customer’s interactions with the bank. Step 6: Data Consolidation Data consolidation enriches your dataset by adding external context. It’s a pivotal step because it deepens the dataset’s dimensions. You may append external data, such as credit scores from credit bureaus and geospatial data for regional analysis, to its customer profiles. This provides a more comprehensive view of the customer’s financial standing and geographical distribution. Step 7: Data Aggregation Aggregation summarizes data, vital for spotting trends that inform strategic decisions. You can summarize the data by region using aggregation tools, which will help you spot trends in customer behavior. This will inform your decisions on where to focus marketing efforts and where to expand services. Step 8: Data Transformation Transformation prepares data for analysis. It’s a critical step in the ETL process because it converts data into actionable intelligence. In this example, you can leverage data transformation tools to calculate new metrics, such as average transaction value, frequency of branch visits, and digital engagement scores. These metrics help classify customers into categories like ‘digital natives’ or ‘branch loyalists,’ aiding in targeted marketing and service design. Step 9: Validate Data and Implement Quality Control Safeguard data accuracy and reliability through regular validation and verification procedures. Implement stringent quality control checks to detect errors early, fortifying data integrity and trustworthiness. A proactive approach will ensure that your enriched dataset remains dependable for driving strategic actions and decisions. You can leverage automated data quality tools to monitor data integrity continuously. They will help you perform regular checks against transaction logs and external data sources to ensure the accuracy and reliability of the enriched data. Types of Datasets Enriching suitable datasets can become a valuable tool in the decision-making process. Different types of data sets contribute to a company’s goals. Customer Data Customer data enrichment goes beyond just updating names and emails. It includes demographics, purchase history, and website behavior data, which can create a much richer customer base. Enriched customer data also empowers you to predict customer behavior, enabling proactive service and targeted product recommendations that ultimately drive customer satisfaction and loyalty. Sales Data Sales data is valuable but enriching it with market trend data and customer demographics can transform it into a strategic tool. You can identify upselling and cross-selling opportunities by understanding market shifts and customer preferences alongside sales figures. It also helps you accurately forecast future sales trends and optimize your pricing strategies to maximize profitability. Marketing Campaign Data Campaign data tells you how many emails were opened, or links clicked. Enriching it with customer demographics and website behavior data allows you to evaluate the effectiveness of your campaigns across different audience segments and channels. This empowers you to identify high-performing channels and tailor future campaigns for maximum impact through audience segmentation. Financial Data Enriching financial data with market data and economic indicators adds valuable context. By understanding how interest rates, currency exchange rates, and broader economic factors influence your financial performance, you can improve the accuracy of your budgeting and forecasting. Enriched financial data also helps identify cost-saving opportunities and empowers you to make informed risk assessments. Operational Data Enriching operational data with weather, traffic, and supplier performance information allows you to optimize delivery routes, schedule preventive maintenance for equipment, and identify operational bottlenecks that hinder efficiency. This information translates to smoother operations, reduced downtime, and, ultimately, a more cost-effective business model. Data Enrichment Tools and Technologies Now that you are aware of how data enrichment works what its benefits are, let’s explore some of the tools and technologies through which you can implement data enrichment: Data Enrichment Software These solutions are often used for specific, niche enrichment tasks. They provide targeted improvements to your data and allow for fine-tuning enrichment processes. Key Features Specialized Functions: These software packages may focus on tasks like email validation, geocoding, or social media data extraction. Customization: They offer customizable options to tailor the enrichment process to your unique requirements. APIs for Data Enrichment Application Programming Interfaces (APIs) allow you to integrate data enhancement directly into your applications and workflows by providing real-time or batch data enrichment. Key Features Real-time Enrichment: APIs can enrich data in real-time as it’s collected or utilized in your applications. Customization: You can tailor APIs to your specific needs and leverage flexibility in accessing and using enrichment services. Scalability: APIs can handle large volumes of data, making them suitable for high-demand applications. Data Integration Tools Another option is data integration software. These tools have advanced data cleansing, quality, and profiling features that clean and standardize your data. They also have data integration capabilities, so you can easily combine data from multiple sources to get a complete view. Key Features Advanced data quality management, profiling, and cleansing features Built-in connectors to extract data from multiple sources and integrate it to form a unified view Advanced built-in transformations and functions so you can easily manipulate data however you want. Automated Data Enrichment Automated data enrichment streamlines data enrichment by automatically updating and consolidating existing datasets with additional, relevant information. This process is typically powered by predefined rules and algorithms that work to identify, verify, and incorporate new data points into an existing database without manual input. New data is validated for accuracy and relevance before merging with existing records. This process adds depth to the data and helps maintain a clean and organized database primed for analysis. Key Features Real-Time Processing: Automatically updates and enriches data as it is collected. Scalability: Handles increasing volumes of data without compromising performance. Customization: Offers the ability to configure rules and parameters to meet specific data enrichment needs. Data Transformation: Converts data into the required format or structure for analysis or reporting purposes. Automated Workflows: Sets up triggers and actions to automate the entire data enrichment process. Best Practices for Data Enrichment Optimizing data enrichment processes requires a strategic approach. To harness the full potential of your data, consider these best practices that emphasize precision, consistency, and adaptability: Start with High-Quality Data Ensure the foundation of data enrichment is clean and accurate. Use data munging to enhance dataset quality. Deduplicate entries, rectify inaccuracies, and standardize data formats. Use Multiple Sources of Data Expand data collection by tapping into diverse sources. Integrate information from customer databases, web analytics, IoT sensors, and ERP solutions. Construct a multidimensional view for deeper insights and understanding. Keep Your Data Up to Date Regularly update your dataset to maintain relevance and accuracy. Stay current with market trends and customer preferences. Automate Your Data Enrichment Processes Leverage data automation to reduce manual workload and human error. Ensure uniform data processing to maintain data integrity. Implement efficient workflows with purpose-built tools for data enrichment. Monitor Your Data Enrichment Results Keep track of the impact of data enrichment on operations. Monitor KPIs to gauge effectiveness. Regularly evaluate and adapt your data enrichment strategies for continuous improvement. Data Enrichment Use Cases B2C and B2B Data Enrichment B2C (Business-to-Consumer) and B2B (Business-to-Business) data enrichment is pivotal in deepening relationships and driving sales. For B2C companies, enriching customer data with personal preferences, purchase history, and social media activity can tailor experiences to individual needs. B2B firms benefit similarly by enriching prospect data with industry trends, company size, and decision-maker information to personalize outreach and proposals. Example: A B2C fashion retailer uses data enrichment to recommend clothing items based on a customer’s past purchases and browsing behavior, while a B2B software provider leverages enriched data to customize software solutions for potential clients based on their business requirements. Customer Data Enrichment Customer data enrichment is about creating a comprehensive profile of your customers. Businesses can gain insights into customer behavior, preferences, and needs by integrating data from various touchpoints. This enriched view enables companies to deliver more personalized services and anticipate customer needs. Example: A telecom operator enriches customer profiles with usage patterns and service preferences to offer tailored data plans and value-added services, increasing customer satisfaction and loyalty. Financial Data Enrichment Financial data enrichment is crucial for accuracy in reporting and compliance with regulatory standards. Financial institutions can improve fraud detection, risk assessment, and customer service by enriching transaction data with contextual information. Example: A bank enriches transaction data with merchant information and location data to detect unusual activity and prevent fraud, ensuring secure customer accounts. Marketing Data Enrichment Marketing data enrichment allows businesses to refine their targeting strategies. Marketers can create more effective campaigns that resonate with their audience by adding demographic, psychographic, and behavioral data to customer profiles. Example: A marketing agency enriches its database with social media interests and engagement data to create targeted ad campaigns with higher conversion rates. E-commerce Data Enrichment E-commerce data enrichment enhances the online shopping experience by personalizing product recommendations, optimizing search functionality, and improving customer service. By understanding customers’ shopping habits and preferences, e-commerce platforms can increase sales and customer retention. Example: An e-commerce platform enriches user profiles with browsing history and purchase data to provide personalized product recommendations, increasing sales and customer engagement. A Guide to Data Quality Management Data Quality Management helps organizations use their data to the fullest. Download our free guide and learn how you maximize the potential of your organizational data. Download Your Free Guide Streamline Data Enrichment with LIKE.TG Realizing the full potential of data enrichment requires understanding its principles, implementing efficient processes, and leveraging advanced technologies. This is where solutions like LIKE.TG come into play. LIKE.TG is an end-to-end data management platform with advanced data extraction, integration, preparation, and automation capabilities, empowering businesses to streamline their data enrichment workflows. It’s a code-free solution with an intuitive, drag-and-drop user interface designed with accessibility and usability in mind. Some essential features of LIKE.TG include: AI-powered data extraction to ingest data from unstructured sources. Streamline the entire data preparation process, from integration to transformation, saving valuable time and resources. A wide array of transformations to shape and refine your data to meet analysis requirements. Built-in data quality and cleansing features with customizable checks and rules to maintain data accuracy and consistency. A visual, interactive grid that allows you to explore data visually and enables hands-on, immediate data cleansing and adjustments. to explore and interact with data in an intuitive and user-friendly manner. Built-in job scheduler and automation capabilities to eliminate manual work. Want to get the best out of your data? Try LIKE.TG DataPrep. Start your journey with a 14-day free trial and see how the code-free tool can help you get insights from your data.
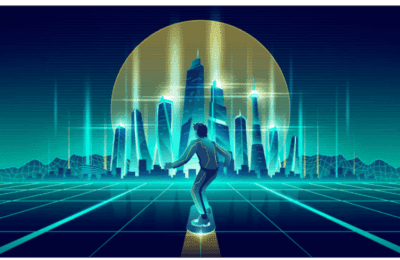
API First vs Code First: Selecting the Right Approach to Developing Applications
The ever-growing demand for digital solutions across industries has led to the prominence of two approaches to product development: API First and Code First. Let’s understand the basics of these approaches, their core differences and the key factors that can help businesses make informed decisions. The Traditional Code-First Approach The traditional code-first approach focuses on writing the code logic first and then designing the API based on the implemented functionality. This approach allows developers to quickly build a functional product and refine their API based on the code. The code-first approach allows developers to dive right into coding and focus on implementing the core features and functionalities of the application. This is particularly useful in situations where there is a tight deadline or when the requirements are not fully defined at the beginning of the project. However, the code-first approach can lead to a less structured and more difficult-to-maintain codebase. Ensuring consistency and coherence across different parts of the application can be challenging without a well-defined API. Additionally, making changes to the API after the code has been written may require significant refactoring. Pros and Cons of the Code First Approach Advantages: Rapid prototyping and quick iterations, leading to faster development cycles. Ideal for situations with unclear or changing requirements. Promotes flexibility and problem-solving rather than adhering to a strict design. Limitations: Lack of standardized interfaces. Potential for tightly coupled APIs, hindering integration and scalability. Inconsistent designs across components. Difficulty in maintaining a cohesive architecture and documentation. Testing and debugging challenges. What is the API-First Approach? For many years, the term “API-first” lacked a standardized definition in the industry. The word meant different things to API developers and professionals alike. According to Postman’s 2021 State of the API report, 42% of developers believed “API-first” was about planning and designing APIs and underlying schema before creating other dependent API components and applications. Meanwhile, 31% thought the term referred to building APIs before applications or integrations. While these two perspectives seem similar, there’s a slight difference. The first views API design as a big part of the overall system development lifecycle, while the second sees APIs as the foundation for building other systems. As of 2023, this approach has been defined as designing and developing the API before writing the actual code. By starting with the API design, developers can establish clear guidelines and specifications for how different parts of the system will interact with each other. This approach promotes a modular and scalable architecture, as the API acts as a central point of control and coordination. Pros and Cons of API First Approach Advantages: Clear and well-defined interface design for effective team collaboration and independent work. Promotes code reusability and facilitates integration with external systems. Improved security measures by incorporating security considerations from the beginning. Enhanced scalability and extensibility, allowing for easy accommodation of future enhancements. Automatic generation of API documentation from the design, reducing documentation efforts and ensuring up-to-date information. Here’s a clip from a recent LIKE.TG webinar, “Unlock the Power of APIs the Code-Free Way,” in which Mehdi Medjaoui, founder of the famous Apidays Conference, discussed the numerous benefits of adopting the API-first approach in detail: Limitations: Requires upfront planning and design effort, potentially slowing down development. Assumes that all design decisions can be made early, which may not always be feasible. Potential delay in implementation due to the need for a finalized API design. API Design First: A Subset of API First Approach API-design first is a subset of the broader API-first approach that focuses specifically on the design aspect of building APIs. With the API-design first approach, designers create a detailed API specification before coding occurs. This specification serves as a blueprint for developers to ensure the API is created according to the desired functionality and requirements. There are a few key principles that form the foundation of the API Design First approach: Designing for the Consumer: With Design First, the focus is on the needs and expectations of the developers consuming the API. Developers can create a user-friendly and efficient API by considering their requirements from the outset. Developers consider factors such as ease of use, simplicity, and consistency when designing for the consumer. API Contracts: An API contract defines the rules and specifications that govern the interaction between the API and its consumers. Designing the API contract first allows for better collaboration between API providers and consumers, ensuring that both parties are on the same page regarding functionality and expectations. Documentation as a Priority: Good documentation is critical to the success of any API. By prioritizing documentation from the design phase, developers can ensure that the API’s consumers can access clear, accurate, and up-to-date documentation, reducing the learning curve and facilitating integration. Comparing API-First and Code-First Development Methodologies The table below highlights the core differences between the two approaches: Aspect API First Code First Design Philosophy Design-driven approach, with the API carefully planned and designed before implementation. Implementation-driven approach, where the design evolves alongside the code. Development Process Iterative development of the API as the foundation, enabling parallel work by multiple teams. Sequential development where code is written first and API is refined afterward. May require synchronization efforts. Flexibility and Control High level of control with upfront planning, clear boundaries, and standardized interfaces. Prioritizes speed and agility, allowing rapid adaptation but may result in inconsistencies and lack of standardized interfaces. Integration Easier integration with external systems and third-party applications due to well-defined APIs. Integration may be more challenging if APIs are tightly coupled with the codebase. Scalability and Extensibility Scalability and extensibility are considered from the beginning, leading to a more robust and maintainable codebase. May face challenges in maintaining and extending the codebase as the design evolves with the code. Development Efficiency Promotes parallel development, reducing dependencies and bottlenecks, resulting in faster feature delivery. May lead to longer development cycles and potential bottlenecks when multiple teams are involved. Criteria For Selecting the Best Approach When deciding between both approaches, there are several key considerations to keep in mind: Project requirements: Businesses should consider the specific needs and goals of the project. Is the project focused on immediate functionality or long-term scalability? Team expertise: Organizations need to evaluate the skills and experience of their development team. Are they familiar with API design principles or have more experience with traditional code-first development? Time constraints: Assess the project timeline and available resources. Does the project have enough time for upfront API design, or is there a need for quick implementation? Making the Right Choice: API First or Code First? Choosing between API First and Code First in software development depends on the project’s requirements and constraints. API First is suitable for well-defined projects, scalability, and integration with external systems. It provides structure, security, and ease of integration when the team has API expertise. It’s also good for scalability planning. Code First is ideal for projects with evolving or ambiguous requirements, emphasizing flexibility and agility. It’s useful for rapid prototyping, quick iterations, and adapting to changing requirements. It’s best when the team is strong in coding and prefers working directly with the codebase, allowing for faster feedback and leveraging existing tools. Ultimately, the choice depends on project specifics, team expertise, and desired outcomes. Careful evaluation is essential for a successful development process. LIKE.TG offers a user-friendly, no-code API design solution that enables you to create and utilize APIs easily, simplifying the API implementation and maintenance process. Incorporating the LIKE.TG API design and implementation tool into your API strategy can strengthen your capacity to respond to changing business requirements, streamline your data workflows, and guarantee a smooth user experience. Contact us to learn more about how LIKE.TG can help you.
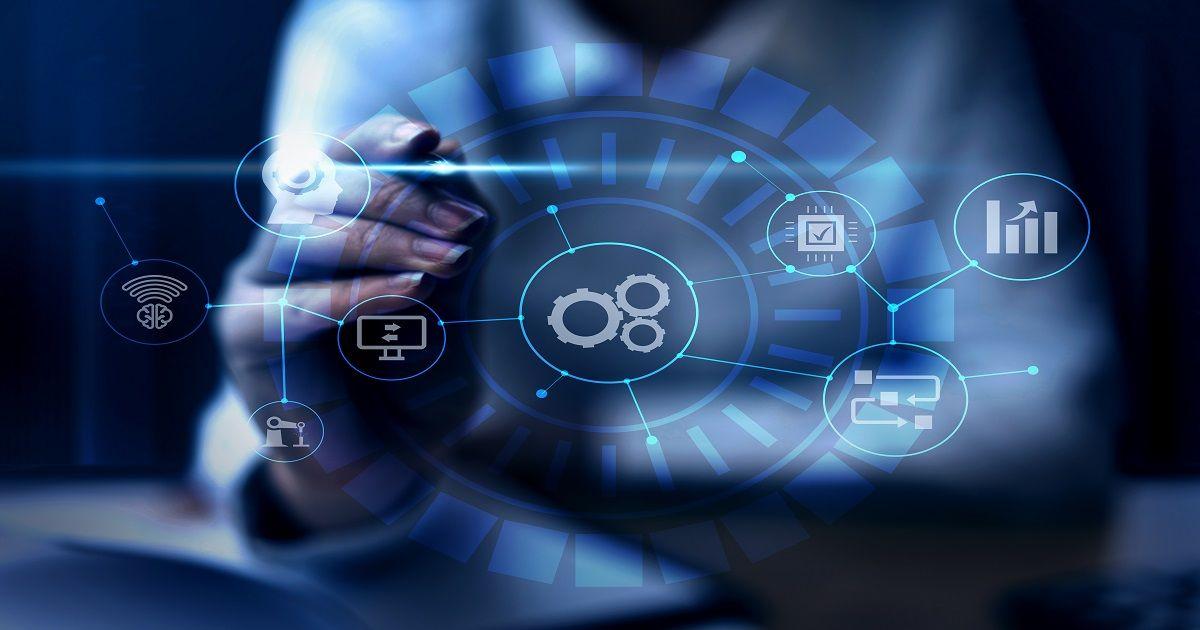
GraphQL vs. REST: Which API Design Architecture is Right for Your Business?
Application Programming Interfaces (APIs) play a crucial role in enabling communication between different software systems, including web applications. APIs define the methods and protocols that allow various software applications to interact and exchange data with each other. Choosing the appropriate API design architecture is highly important for developers as it influences project outcomes. This blog presents a head-to-head showdown: GraphQL vs. REST, i.e., two leading API design architectures. Understanding REST APIs REST (Representational State Transfer) is a design style that has gained widespread popularity due to its simplicity and scalability. According to Postman’s 2023 survey, 86% of developers use REST architecture style. RESTful APIs follow a client-server architecture, where the server exposes a set of resources that clients can access using standard HTTP methods such as GET, POST, PUT, and DELETE. These APIs leverage the existing HTTP protocol for communication, making them lightweight and highly scalable. Key Components of a RESTful API Resources: In a RESTful API, resources represent the entities that clients can interact with. These resources can be anything from a user profile to a product listing. Each resource has a unique identifier (URI) and can be accessed using HTTP methods. HTTP Methods: RESTful APIs use standard HTTP methods to perform operations on resources. The most commonly used methods are: GET: Used to retrieve a representation of a resource. POST: Used to create a new resource. PUT: Used to update an existing resource. DELETE: Used to delete a resource. PATCH: Used to make partial updates to an existing resource. Uniform Interface: RESTful APIs follow a consistent interface, which means that the interactions between clients and servers are standardized. This uniformity allows clients to understand and interact with different APIs consistently. Stateless: RESTful APIs are stateless, meaning that each request from a client to a server is independent and does not rely on previous requests. This independence makes them highly scalable and allows servers to handle many concurrent requests. This also means that the server does not store the client sessions, so any request from the client should independently contain all necessary information to process the request. Pros and Cons of RESTful APIs RESTful APIs offer several advantages: REST APIs are simple to understand and implement, making them developer-friendly. With a clear set of principles, developers can quickly grasp the concepts and build APIs. REST APIs enable clients to be built using any programming language or framework since they are platform independent. This flexibility makes it easier to integrate different systems and technologies. REST APIs leverage the caching capabilities of HTTP, resulting in reduced server load. By caching responses, RESTful APIs can minimize the amount of data transferred over the network, leading to faster response times. However, RESTful APIs also have their limitations: Managing REST APIs can become complex as the number of resources and operations increases due to the proliferation of endpoints and the inherent design characteristics of REST. REST APIs lack a standardized method of handling data validation. While they contain guidelines for structuring responses, there is no standardized approach for handling validation errors. RESTful APIs are unsuitable for real-time applications requiring bi-directional communication as these APIs are primarily designed for request-response style interactions. Understanding GraphQL APIs GraphQL is a relatively new API design approach that Facebook developed. It offers a flexible and efficient way of querying and manipulating datasets. Postman’s 2023 survey shows GraphQL APIs are the third-most widely used API architecture, with a 29% adoption rate. GraphQL APIs allow clients to specify the exact data they need by sending a single nested request to the server. The server then responds with a JSON (JavaScript Object Notation) payload that includes only the requested data. When using GraphQL, clients can define the structure of the response by creating a query that matches the shape of the data. Pros and Cons of GraphQL APIs GraphQL APIs offer several benefits: GraphQL enables clients to retrieve multiple resources in a single request. Clients can specify the relationships between resources in their query, and the server will return all the requested data in a single response. This capability reduces the number of round trips to the server, improving performance and reducing network overhead. They eliminate the problem of over-fetching or under-fetching data commonly encountered in RESTful APIs. GraphQL solves this issue by allowing clients to retrieve only the required data, reducing network latency and improving performance. GraphQL provides powerful tools for exploring and understanding the API schema through introspection. Developers can easily query the API to retrieve information about available types, fields, and relationships. This feature makes it easier for developers to understand the data model and build efficient queries. GraphQL APIs also have some limitations: GraphQL requires additional configuration and complexity on the server side. Implementing a GraphQL API may involve creating schema, resolvers, and server-side components. GraphQL may not be the best choice for simple, read-only APIs. A simpler RESTful API may be more suitable if an API primarily serves static data that doesn’t require complex querying or manipulation. They can be vulnerable to data exposure if not implemented correctly. Since clients can specify the structure of the response, API developers must properly validate and sanitize user input to prevent unauthorized access to sensitive data. A Comparison of GraphQL Vs REST API Requests To demonstrate how to make REST and GraphQL requests, let’s consider a scenario where you need to retrieve information about a third-grade student. Below, you’ll find sample ‘request code’ for both approaches: RESTful API Request To retrieve a student’s information using a RESTful request, you can use the HTTP GET method: GET /api/students/class3/{studentId} In this RESTful request, replace {studentId} with the ID or unique identifier of the student. GraphQL API Request On the other hand, with GraphQL, you can create a specific query to request only the fields they need. Here’s an example: query { studentById(studentId: “123”) { id name class # Add other fields you want to retrieve } } In this GraphQL query, specify the ‘studentId’ for which information needs to be retrieved. GraphQL’s flexibility allows users to request exactly the fields you require. GraphQL vs REST: Similarities and Differences The table below provides a concise overview of the key differences and similarities between GraphQL and REST APIs. Aspect GraphQL REST Definition A query language for APIs that allows clients to request only the data they need. A set of architectural principles for designing networked applications. Data Fetching Clients can specify the shape and structure of their desired data in a single request. Clients retrieve fixed data structures defined by the server. Multiple requests are needed for related data. Over Fetching Minimal over-fetching of data. Clients precisely get the data they request, reducing network overhead. Over-fetching can occur when clients receive more data than they need, leading to wasted bandwidth and increased latency. Versioning GraphQL doesn’t require versioning because clients can request the fields they need. REST APIs may require versioning to ensure backward compatibility when endpoints change. Response Size Responses contain only the data requested by the client, reducing response size. Responses often include a fixed set of data, which can result in larger responses that include unnecessary data. Flexibility Highly flexible for clients, as they can adapt queries to their specific needs without API changes. Less flexible for clients, as they rely on predefined endpoints provided by the server. Caching Caching can be more challenging due to the dynamic nature of GraphQL queries. Caching is often easier with REST because resources have predictable URLs. Complexity GraphQL can be more complex to set up and maintain, especially for large APIs. REST can be simpler to implement, especially for straightforward APIs. Discoverability GraphQL APIs provide strong introspection capabilities, making discovering the schema and available queries/mutations easier. REST APIs may require extensive documentation to understand available endpoints and data structures. Security Security depends on implementation; it requires careful input validation and query depth control to prevent security risks REST security is typically based on authentication and authorization at the endpoint level. Key Factors in Choosing an API Architecture Design When evaluating API design options, there are several key factors to consider. These include: Scalability: As businesses expand and attract more users, the API should be able to handle the increased load without compromising performance or stability. You must consider designing a system that can efficiently scale horizontally by adding more servers or vertically optimizing the code and infrastructure. Performance: API performance directly impacts user experience and the success of an application. A well-designed API should be able to process requests quickly and deliver responses promptly. You can improve the API performance by optimizing code, caching mechanisms, and leveraging efficient data retrieval techniques. Security: Implementing robust authentication and authorization mechanisms ensures that only authorized users can access sensitive data. You must employ encryption and data validation techniques to protect against data breaches and ensure data integrity. Developer Experience: A well-documented API with clear and concise documentation, code examples, and tutorials can significantly reduce the learning curve for developers. Comprehensive support, such as developer forums and dedicated support channels, can enhance the developer experience. Conclusion: Making the Right Choice Choosing the right API design for your business involves assessing your specific needs and evaluating the compatibility of each option with your existing systems and processes. GraphQL and REST APIs have advantages and disadvantages, and choosing the one that best suits your business requirements and long-term goals is important. LIKE.TG is a self-service API design tool that empowers you to create and utilize APIs efficiently while streamlining the implementation and maintenance process. Integrating LIKE.TG API design and implementation tool into your API strategy can enhance your ability to adapt to evolving business needs, optimize your data workflows, and ensure a seamless user experience. Contact us to learn more about how LIKE.TG can help you. Ready to Transform Your API Strategy? Empower your business using LIKE.TG's no-code interface with advanced data integration capabilities to develop, consume, and publish your APIs and integrations in a single platform. View Demo
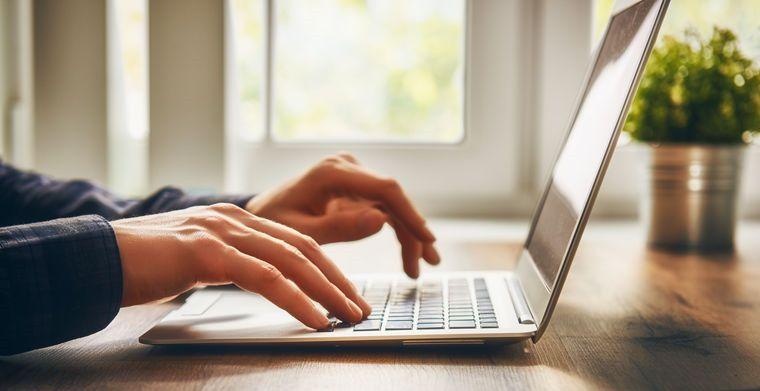
Data Exploration: A Comprehensive Guide
A clear understanding of data health enhances data quality and trustworthiness. This is where data exploration comes into play. Data exploration provides extensive insights into the characteristics of your data. You can uncover data anomalies and learn how to address them by delving deep. Whether identifying outliers, understanding correlations, or refining feature selection, data exploration empowers you to make informed decisions. What Is Data Exploration? Data exploration is the initial step in data preparation and analysis using data visualization tools and statistical techniques to uncover patterns and relationships within a dataset. It helps identify outliers, detect relationships between variables, and understand the nature of data. Data Exploration vs Data Visualization Data exploration often involves data visualization to help you understand the dataset’s structure, the presence of outliers, and the distribution of data values. On the other hand, data visualization tools, such as bar charts and scatter plots, are valuable in visual data exploration, providing a visual representation of the data that can aid in identifying patterns and relationships. Data Exploration vs Data Mining Data exploration manually sifts through data to understand its characteristics and structure. For example, a data analyst might use a scatter plot to identify outliers or understand the distribution of data points. On the other hand, data mining is an automated process that aims to extract useful information and patterns from large datasets. It uses sophisticated algorithms to discover patterns that are not immediately apparent. Data Mining is often used for predictive analysis, such as forecasting future trends or behaviors based on historical data. Data Exploration vs Data Discovery Data exploration and data discovery are related but distinct concepts. Data discovery is cataloging data assets, making it easier for users to search and understand what data is available. On the other hand, data exploration explores and visualizes data to uncover insights and identify areas or patterns to dig deeper into. Why Is Data Exploration Important for Businesses? Data exploration uncovers patterns and relationships between variables. These insights prove invaluable for businesses aiming to understand customer behavior, optimize operations, and outpace competitors. Here are some key benefits of data exploration: Identify Patterns and Trends Data exploration is integral to Exploratory Data Analysis (EDA). It statistically analyzes and visualizes data, revealing trends that, once confirmed, can help you develop more effective business strategies. Improve Operational Efficiency According to McKinsey, data-driven workflows can help organizations save 42% of time on internal processes, thus improving operational efficiency. Data exploration serves as a valuable starting point for uncovering potential areas of improvement. Drive Growth Data exploration lays the groundwork for more sophisticated analytics that drive business growth. It can help you identify new opportunities and segments or markets to target. Accenture finds that businesses that use data analytics to identify new opportunities and markets are 23% more likely to grow their revenue. Through data exploration, you can gain insights for formulating strategies that drive growth and establish a solid foundation for future business intelligence. How to Explore Data in 10 Easy Steps Step 1: Identify the Data Domain Learning the domain and familiarizing yourself with its structure and content will allow you to get the most value out of your data. Understanding the context will provide a better understanding of the data’s meaning, relevance, and purpose. For instance, Electronic Health Records (EHR) data is complex and requires deep knowledge of medical terminology, clinical workflows, and health information technology. Without this knowledge, users couldn’t utilize this data as effectively. You must also understand why you’re using that dataset. Knowing your objectives will help you set the right data exploration goals. Are you trying to identify patterns or outliers? Or do you want to detect errors or understand the distribution of your data? By understanding your goals, you can more effectively profile your data. Step 2: Gather Relevant Data You must consolidate, combine, or merge data from different sources based on your requirements. These sources can be database tables or other formats that store relevant data. However, not all sources are compatible with each other. You often need to find a common field or key to link them. For example, if you are working with EHR data, you can use the patient ID as a shared key to join data from tables such as patient demographics, medical history, medication orders, lab results, etc. This way, you can gain a more comprehensive understanding of the information. Step 3: Get Familiar with Your Data Before you start analyzing your data, you must get familiar with it. You should check the quality and suitability of your data for your analysis goals. For example, if you have a dataset of customer transactions, you could explore the following aspects of your data: The distribution: How are the transaction amounts spread across different ranges? What is the average, variance, and standard deviation of the transaction amounts? The frequency of transactions per customer: How often do customers make transactions? What is the average, median, and mode of the number of transactions per customer? Are there any customers who make very few or very many transactions? Any unusual patterns in the data: Are there any trends, cycles, or seasonality in the data?? Are there any missing or incorrect values in the data? Step 4: Evaluate the Suitability of Your Data You must ensure your data is suitable for your analysis objectives. Therefore, evaluating its relevance, timeliness, and representativeness is essential. Relevance means how closely your data relates to the questions you want to answer. Timeliness means how recent your data is and whether it reflects the current situation. Representativeness means how well your data covers the population or phenomenon you are interested in. By evaluating these aspects, you can decide whether your data is sufficient or whether you need to collect more data. If you want to analyze the long-term trends in customer behavior for an online store. If you only have a dataset of customer transactions from the past year, your data might need to be more relevant, timely, and representative. You might need to collect more historical data from previous years to capture the changes and patterns in customer behavior over time. Step 5: Identify Data Types, Formats, and Structures The next step is to identify the data types, formats, and structures of your data. Your data could be Date, numerical, Boolean, categorical, string, etc. You should review each column of your data and identify its data type. This will help you understand how your data is organized, what each variable means, and what values it can have. It’s also essential to review the structure of your data at a detailed level. This will help you choose the right statistical methods for your analysis. You can learn more about your data by reviewing the data dictionary, codebook, or metadata that comes with your data. These resources can help you interpret your data and make better decisions about how to analyze it. Step 6: Find Null or Missing Values Missing or null values are a common problem in data. They occur in data for various reasons, such as errors in data collection or entry or privacy concerns. Missing values affect the quality and reliability of your analysis. Data profiling will help you reveal the frequency of missing values in each field. Visualizations like heat maps or bar charts can help you study the extent of missing values in a dataset and their distribution across different variables. Doing so will help you find patterns and trends in the data and decide how to handle the missing values. Step 7: Discover Duplicates Duplicate data refers to rows of data that have identical or similar content. Such redundant data can affect the quality and reliability of your analysis, as well as your system’s storage space and performance. Therefore, data experts often remove duplicate records and keep only one instance of each unique record. However, before deleting duplicate records, you must consider which instance to keep and which to discard. Sometimes, duplicate records may have subtle differences relevant to your analysis. If you have a dataset of customer orders, but some records have errors or missing information. In this case, you can use duplicate records to fill in the gaps or correct the mistakes. In other cases, you may need to merge duplicate records into a single record. For instance, when the customer has two accounts with different information, you may need to combine the information from both accounts into one record to avoid confusion and inconsistency. Step 8: Identify Inconsistencies Datasets often have inconsistencies that can result in inaccurate analysis. These inconsistencies stem from a need for more explicit formatting or standards during data entry and collection. Validating your data for errors can help identify and flag such discrepancies. Here are some of the most common discrepancies in data sets. Typos in all data fields Inconsistencies in units of measurement Variations in naming conventions Fields with invalid phone numbers Inconsistent use of abbreviations Fields with unwanted characters Invalid addresses Fields with non-printable characters Variations in formatting or data types Fields with leading, trailing, and duplicate spaces Step 9: Highlight Outliers Outliers are data points that are very different from the rest of the data. They result from various factors, such as measurement errors, data entry errors, or natural variations in data. Outliers can distort the overall results of statistical analysis, so it is essential to identify and potentially remove them. A straightforward way to find outliers in a dataset is to plot the data on a graph, such as a scatter plot, and look for points far away from the main cluster. However, this method can be subjective and inaccurate. A more reliable way is to use statistical measures, such as the z-score, which tells how many standard deviations a data point is from the mean. A common rule is that a data point with a z-score more significant than 3 or less than -3 is an outlier. That said, identifying outliers is seldom straightforward. Depending on the context and the purpose of the analysis, some outliers may be more relevant than others. The domain knowledge and the statistical methods used to define outliers can also affect the outcome. Therefore, it is essential to understand the nature and the source of the outliers before deciding how to handle them. For instance, if you’re analyzing the income distribution in a town, you would collect a sample of 100 people and calculate their annual income. The research shows that most people earn between $15,000 and $120,000 annually, but two earn $1 million and $10 million annually. These people are outliers because they are very different from the rest of the sample. However, they may not be errors or anomalies. They may represent a small but significant group of wealthy people in the country. If you remove them from the analysis, you may underestimate the income inequality in the country. On the other hand, if you keep them in the analysis, you may overestimate the average income in the country. Therefore, you need to consider your analysis’s context and goal before deciding how to deal with these outliers. Step 10: Summarize and Display Your Data After you have gathered your data, you need to summarize and display it using descriptive statistics and visualizations. These tools can help you better understand the relationships among variables in your data. Imagine you have a dataset of customer transactions from an online store. Use descriptive statistics to calculate the average transaction amount, the range of transaction amounts, and the variability of transaction amounts. You may also want to use visualizations to show how the transaction amounts are distributed, how they vary by customer age, and how other factors, such as product category or season, influence them. Summarizing and displaying your data can gain valuable insights into customers’ behavior and preferences. Data Exploration in AI and ML Data exploration is pivotal in artificial intelligence (AI) and machine learning (ML) since it helps make predictive models more accurate. A machine learning algorithm is as good as the data you feed it. Exploring your data helps you understand how certain variables relate and interact with each other while analyzing their impact on the outcomes of predictive models. For instance, understanding how these features are related in a dataset with variables like age, income, and education level will help you make ML models more accurate. Data scientists often leverage data exploration to discern patterns, correlations, and outliers within large datasets. This process allows you to identify inaccuracies or irrelevant information and visualize it to illustrate complex relationships graphically. Data exploration can also help you perform feature selection, which is the process of identifying the most relevant variables that contribute to the predictive power of a model. Moreover, it can help you evaluate model performance by revealing underlying data structures that could affect predictions. Through iterative exploration, you can refine their models, enhance accuracy, and ensure robustness against overfitting. Data Exploration Tools The traditional manual approach to data exploration is labor-intensive and susceptible to human error. Data scientists have gravitated towards automated data exploration tools in response to these challenges. These sophisticated tools leverage algorithms and ML techniques to scrutinize data more precisely and efficiently. They can manage extensive datasets and unearth insights that might elude manual analysis. Prominent data exploration tools like LIKE.TG have revolutionized the exploration process. LIKE.TG is an all-encompassing, no-code data management platform designed to streamline the entire data journey. From intricate extraction processes across diverse data landscapes to meticulous data preparation and integration, LIKE.TG provides the tools to transform raw data into actionable insights. While LIKE.TG focuses on end-to-end data management, it also complements visualization tools like Power BI and Tableau for visualization and reporting. It ensures that data is clean and well-structured, crucial for creating compelling visualizations. You can use LIKE.TG to prepare data and then utilize Power BI or Tableau for detailed visual analysis. This combination allows for a comprehensive approach to data exploration, leading to more informed business decisions and strategies. These tools expedite the data exploration process and enhance its accuracy. Automating repetitive tasks will allow you to focus on strategic analysis and decision-making. As the field of data science evolves, these tools will continue to play a crucial role in unlocking the full potential of data exploration. Automating Data Exploration with LIKE.TG Data exploration helps you unlock various insights within a dataset. However, the right tools make the process significantly more efficient and effective. A data exploration tool equipped with a real-time, preview-centric design is the modern-day compass for navigating complex datasets. It streamlines the process by providing instant access to data previews, making it easier to comprehend the dataset’s structure, but it also helps monitor data integrity. LIKE.TG DataPrep offers: Interactive Data Grid: Agile correction capabilities that allow for hands-on, immediate data cleansing and adjustments. Data Quality Assurance: Implement comprehensive checks and rules to maintain data accuracy and consistency. Rich Data Transformations: A wide array of transformations to shape and refine your data to meet analysis requirements. No-Code Interface: An intuitive point-and-click environment that democratizes data preparation, making it accessible to users of all skill levels. Connectors for Diverse Data Sources: Seamless integration with various on-premises and cloud-based sources, ensuring smooth data extraction and consolidation. Workflow Automation: Streamline the entire data preparation process, from integration to transformation, saving valuable time and resources. LIKE.TG bridges the gap between raw data and actionable insights, facilitating quicker decision-making, predictive modeling, and ultimately better business outcomes. Experience firsthand how LIKE.TG’s AI-powered data extraction and sophisticated data preparation can revolutionize your data pipelines. Join us for a live demo and start your journey toward seamless, automated, and insightful data management today!