数据分析
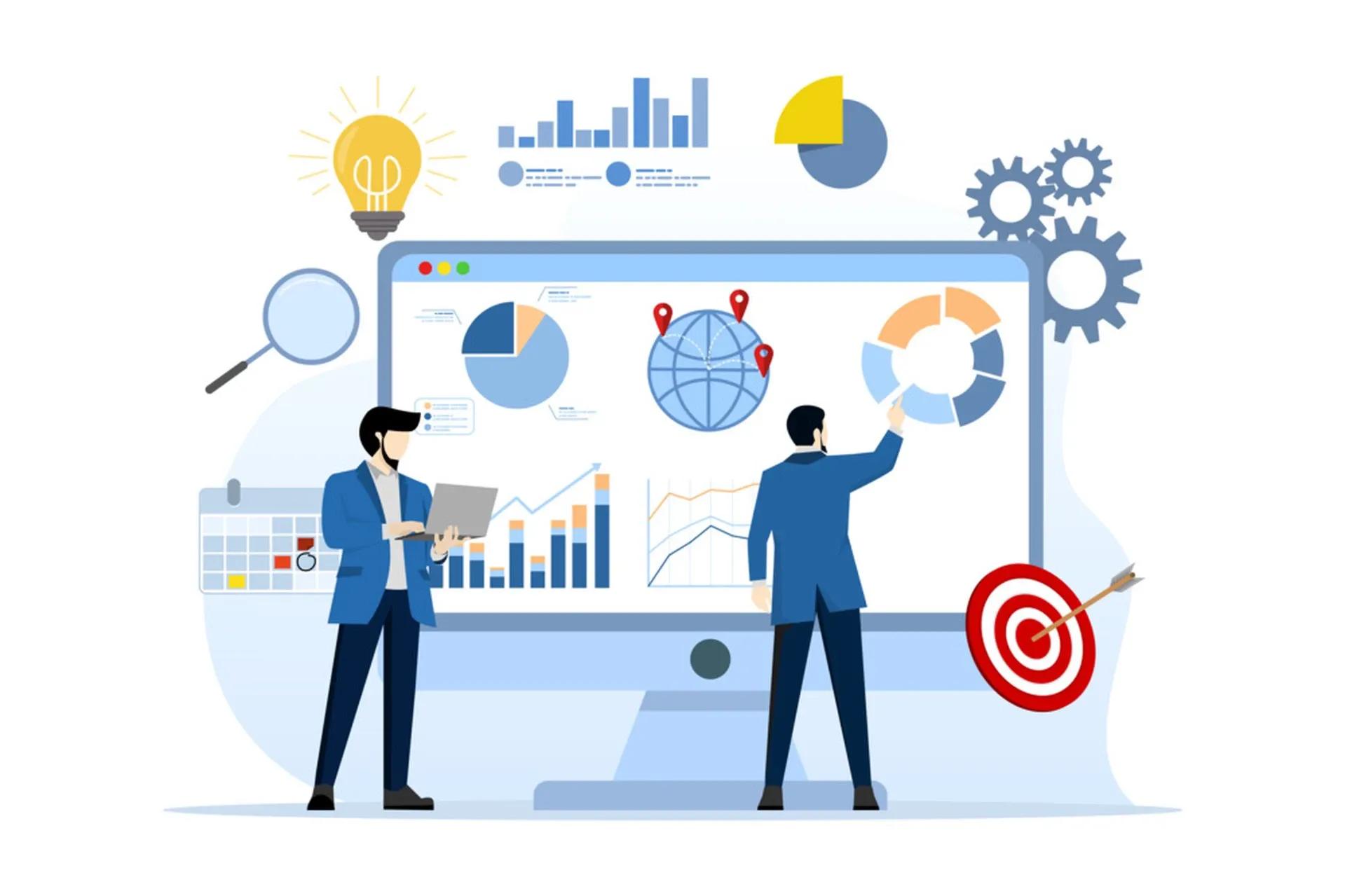
The Best Talend Alternatives & Competitors to Consider in 2024
Mergers and acquisitions don’t only involve the shareholders—in fact, all stakeholders, including the customers, are affected by these transformative events. Customers must decide whether to stay or to jump ship. The same is the case with Talend’s recent acquisition by another business, posing the question: “What does it mean for Talend’s customers?” Some might have started their search for Talend alternatives the moment the news broke, while others may be patiently waiting for updates and assurances from the acquiring company. Either way, it falls on the customers to determine their next line of action. In this article, we will explore some of the best Talend alternatives so you can make an informed decision when deciding between data integration tools. Manage All Your Data From End-to-End With a Single, Unified Platform Looking for the best Talend alternative? Try LIKE.TG. Get data extraction, transformation, integration, warehousing, and API and EDI management with a single platform. Learn More What is Talend and What Does It Offer? Talend is a data integration solution that focuses on data quality to deliver reliable data for business intelligence (BI) and analytics. Data Integration: Like other vendors, Talend offers data integration via multiple methods, including ETL, ELT, and CDC. Additionally, it supports integration with big data technologies like Hadoop and Spark. Data Transformation: Users can perform data transformation using Talend’s platform. However, advanced transformations require high-level proficiency in Java, prompting businesses with limited in-house Java expertise or a preference for no-code solutions to look for Talend alternatives. Data Quality: It includes features for data quality management, ensuring that the integrated data is accurate and consistent. Data Governance: Talend’s platform offers features that can help users maintain data integrity and compliance with governance standards. Application and API Integration: Finally, Talend also offers application and API integration capabilities, enabling users to combine data from different applications and APIs. Why Consider Talend Alternatives for Data Integration Forward-thinking organizations know what they need and live by a set of principles that go beyond mere size or recognition. These modern businesses are interested in solutions that directly cater to their use cases, solve their problems, are easy to use and implement, are economical (with transparent pricing), and are serious when it comes to customer support. Moreover, with advancements in technology and the growing number of unique business requirements, traditional solutions are no longer capable of solving the most pressing challenges. This also means that consumers have a lot more options now compared to five years ago. The availability of multiple vendors and the ease of switching has created space for businesses to look for and adopt alternative solutions. The same applies to the data integration landscape—there are multiple Talend alternatives to choose from. Here are some reasons to consider Talend alternatives when it comes to data integration: Acquisition or Merger With Another Business Mergers and acquisitions introduce a level of uncertainty about the future direction of the product and the overall roadmap. On the strategic front, leadership changes post-acquisition can impact the vision and strategy of the company. Then there is the issue of delays and disruptions in product development, support, and overall service as the two companies integrate. Given that Talend was acquired recently, it’s expected that some of its existing customers might be looking for alternatives to Talend’s data integration solution, especially if they are unsure about its stability and continuity and sense a shift away from their needs. Complexity of the UI While Talend can be a capable solution for advanced technical users, its UI deters business users from taking charge of their data integration and BI initiatives. This is because Talend is primarily a low-code tool unlike some other fully no-code platforms, such as LIKE.TG. Additionally, according to popular review sites, like G2, Talend’s UI feels outdated, making it even more complex for business users to navigate and make the most of their investment. Specifically, the error messages are not self-explanatory and make debugging a challenge. Overall, the learning curve can be steep, especially for users without a technical background. Quality of Customer Support Talend’s customer support is not amongst the best in class. In fact, it has an overall support rating of 6.6/10—this can be fact-checked on TrustRadius. While there’s community support for its open-source solution, Talend Open Studio, the documentation lacks depth, which makes it even more difficult for business users. Cost of the Solution Investing in Talend might not be budget-friendly for small businesses or startups as the costs quickly add up. It offers multiple plans; however, prospects will need to get in touch with their sales team for information on pricing. Additionally, most features require the Enterprise version, which further adds to the existing costs. Limitations of the Platform Being able to write code is a prerequisite to make the most of Talend’s capabilities. For example, users will need to be proficient in Java if they want to leverage Talend’s advanced data transformation features or create custom components. On the performance side, users report that Talend is generally less efficient with compute and memory usage. The overall speed of the platform is slow when compared to other vendors—this is on top of the fact that it’s already resource-intensive. As far as data integration and pipelines are concerned, Talend offers limited options. For example, its ETL component lacks support for the ability to join tables from different schemas. The Best Talend Alternatives in 2024 Finding the best Talend alternative is a question of weighing various factors such as functionality, ease of use, scalability, and compatibility with specific business needs. It involves a careful evaluation of different solutions to identify the one that aligns most effectively with the organization’s data integration requirements and long-term goals. Here is a list of the 5 best Talend alternatives to consider: LIKE.TG LIKE.TG is an automated, end-to-end data management platform powered by artificial intelligence (AI) capabilities. It features a 100% no-code, drag-and-drop UI that delivers a consistent user experience across all its products, keeping the learning curve short and smooth. It empowers all its users, whether with a technical or business background, to build data pipelines in minutes. The platform offers a range of products in its suite that helps businesses manage their data without writing a single line of code. Unlike Talend, LIKE.TG is a truly unified solution with all its products featuring the same UI and user experience. Its platform includes: ReportMiner for unstructured data extraction in bulk. Centerprise for data integration and building and orchestrating data pipelines. Data Warehouse Builder for creating a custom data warehouse and related data warehousing features. EDIConnect for EDI management. API Management for designing, testing, publishing, and monitoring APIs. LIKE.TG offers several features and capabilities that make it one of the best Talend alternatives. With LIKE.TG’s platform, you can: Establish native connectivity to a range of data sources and destinations, both on-premises and cloud-based, to build a single source of truth (SSOT). Transform and shape your data the way your business needs it using pre-built transformations and functions. Build ETL and ELT pipelines without writing a single line of code. Ensure only healthy data makes it to your data warehouses via built-in data quality management. Automate and orchestrate your data integration workflows seamlessly. Take the first step to No-Code Data Management with LIKE.TG Whether you want to extract unstructured data in bulk, integrate data from disparate source, or build a data warehouse from scratch, LIKE.TG's 100% no-code platform empowers you to do it all. Download Trial Informatica Informatica is another contender in the list of Talend alternatives. It is an enterprise-grade data management solution widely used for a variety of data integration use cases. Additionally, it caters to different data related processes, such as master data management, data quality and governance, etc. Pros: Support for multiple data sources and destinations. Ability to perform advanced transformations on data sets. Orchestration of data movement across systems. Cons: The breadth of services can be overwhelming. It’s a very complex platform, especially for business users. Being resource-intensive, it has high compute and memory requirements. Given the complex nature of the platforms, many businesses seek out Informatica alternatives. Oracle Oracle Data Integrator (ODI) can be another alternative to Talend when it comes to data integration. Being a data movement platform, it primarily leverages ELT. If the technology stack already includes multiple Oracle products and services and the goal is to keep the integration processes seamless, ODI can be a valuable addition. Pros: Low-code UI speeds up some tasks. Multiple built-in transformations to work with. Integrates seamlessly with other Oracle products and services. Cons: Users report that Oracle’s customer support is not amongst the best. While it claims to be highly scalable, user reviews highlight performance issues. The UI is not intuitive, which makes troubleshooting difficult. IBM InfoSphere DataStage IBM InfoSphere is a data integration platform that focuses on the design, development, and running of data transformation and movement workflows. It’s primarily used as an ETL tool but also supports ELT. IBM offers multiple versions of InfoSphere DataStage, including Server, Enterprise, and MVS. Pros: Offers capable data mapping functionality. Ability to handle large data volumes. Transformations can be applied without using a lot of code. Cons: IBM’s technical support leaves a lot to be desired. Better suited to users with a technical background. It’s generally pricier than other Talend alternatives. Matillion The last in this list is Matillion, an ETL tool that leverages AI to build and manage pipelines. Matillion’s focus is on making the data available for use as quickly as possible. Pros: Ability to handle both structured and unstructured data. Can be integrated with multiple cloud providers without much trouble. Matillion’s UI is one of the better ones as far as useability is concerned. Cons: Often the updates are not backward compatible. Has a hard limit on the hardware/EC2 instances, meaning it can have scalability issues. Price could be a deterring factor for some businesses. Here’s Why LIKE.TG is the Best Talend Alternative Deciding on a data integration solution requires due diligence. Businesses must consider the platform’s ease-of-use and user-friendliness, transparent and economical pricing, ability to handle high-volume data without performance degradation, ability to combine data via multiple methods, and many other factors. With several vendors available in the market, and the fact that most offer a similar set of features, settling for a solution can be tricky. However, the key is to find the best fit. In this scenario, LIKE.TG stands out from the abovementioned Talend alternatives as it offers an end-to-end data integration and management solution with a consistent UI and UX throughout. Here are more reasons to consider LIKE.TG as an alternative solution to Talend: Simple and straightforward initial setup process significantly reduces the time required for solution deployment. 100% no-code, visual drag-and-drop UI keeps the learning curve short and smooth. Parallel processing ETL/ELT engine delivers superior performance even when dealing with high volume data sets. Pre-built connectors for a breadth of data source types, including file formats, databases, data warehouses, applications, cloud storages, web services, and file providers. The ability to instantly preview data at every step of the process with a couple of clicks. LIKE.TG offers the capability to build an entire data warehouse from scratch without writing a single line of code. The ability to build a custom connector for the required source or destination seamlessly. Proactive customer support that’s available round the clock regardless of geographic location. Download a 14-day free trial to get started. Alternatively, get in touch with us directly at +1 888-77-LIKE.TG. Take the first step to No-Code Data Management with LIKE.TG Whether you want to extract unstructured data in bulk, integrate data from disparate source, or build a data warehouse from scratch, LIKE.TG's 100% no-code platform empowers you to do it all. Download Trial
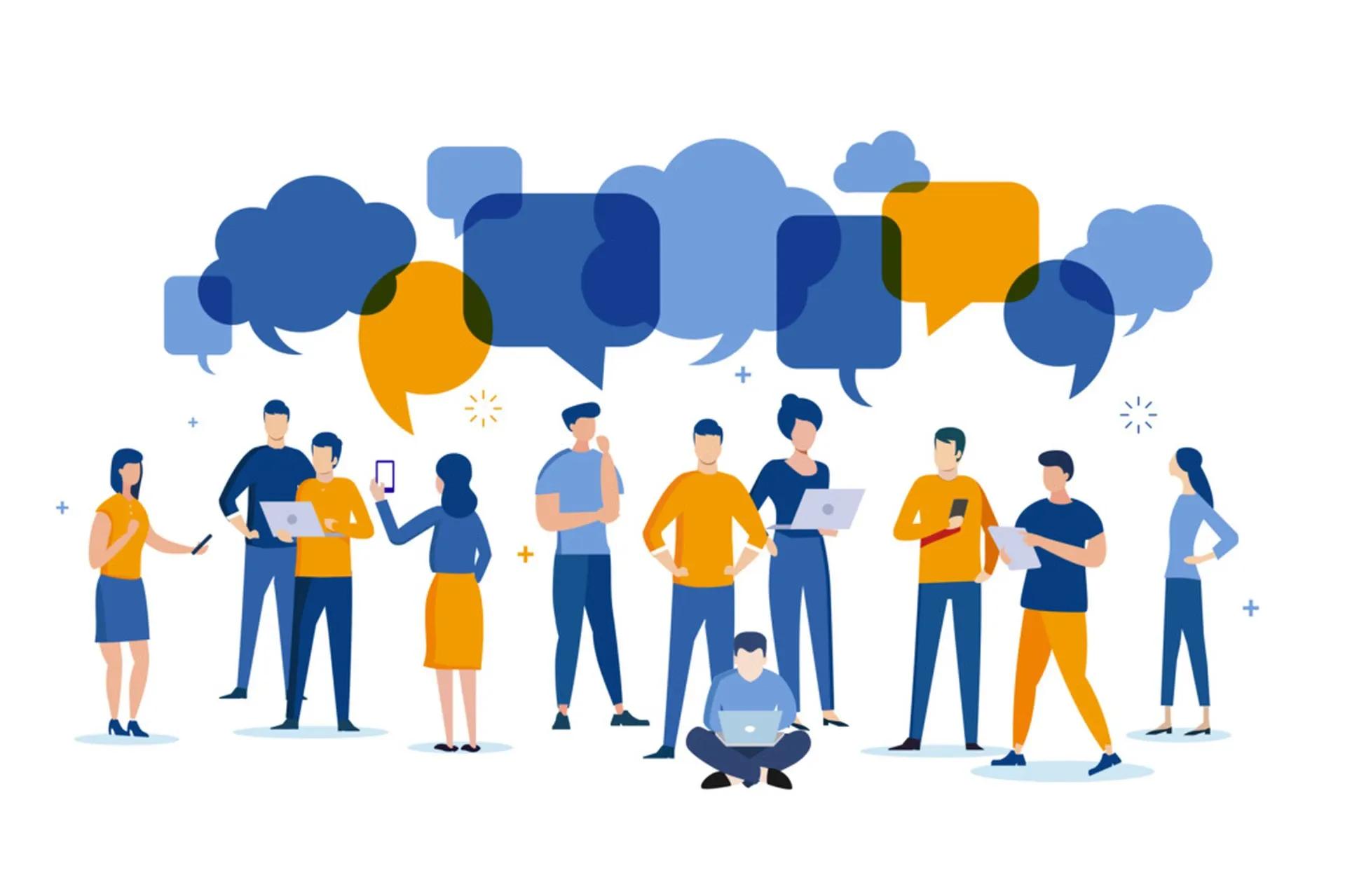
Information Marts: Enabling Agile, Scalable, and Accurate BI
Businesses need scalable, agile, and accurate data to derive business intelligence (BI) and make informed decisions. However, managing evolving data requirements has become more difficult with predefined data models and rigid schemas. Information marts, combined with data vaults can help you adapt to growing data volumes and user demands and deliver insights swiftly and iteratively. What are Information Marts? Information marts (also called data marts) are data structures optimized for reporting and analysis. They are built on top of specialized data warehouses called data vaults, allowing users to customize and modify data and reports. Information marts are designed to meet the needs of specific groups by having a narrow subject of data. For instance, an information mart specialized in sales will contain data related to sales performance, such as revenue, orders, customers, products, regions, and channels. This allows sales teams to access critical insights quickly and easily, without searching the entire data warehouse. According to IBM, information marts can help your team reduce costs, improve efficiency, and enable tactical decision-making. The data vault stores raw data, while the business vault applies business rules, and transformations to the data. Typically, the data vault is not optimized for reporting and needs information marts to transform and aggregate data for analysis. Information Mart Design Information usually follows a star schema or a snowflake schema, which are simple and intuitive structures containing fact tables and dimension tables. Fact tables store quantitative measures or metrics, while dimension tables store descriptive attributes or dimensions. A fact table stores metrics critical to the business operation, such as sales transactions, costs, revenue, and profits. Dimension tables provide additional context to this information. A sales transactions table may be connected to dimension tables that illustrate different aspects of the transactions, such as products, customers, regions, and dates. Therefore, users can aggregate sales transactions by customers, products, regions, or dates. Furthermore, information marts deliver focused, business-centric data to end-users like analysts, managers, and executives. This helps organizations create BI pipelines with access to historical data. This design approach also supports various types of analysis, such as descriptive, diagnostic, predictive, or prescriptive. Analytics teams can also visualize these insights by leveraging reporting and visualization tools, such as dashboards, charts, or graphs. Why are Data Vaults and Information Marts Crucial in the BI Ecosystem? Data vault uses a hub and spoke architecture to simplify the intricacies of data integration and storage. Its versatility enables users to seamlessly manage diverse and ever-changing data sources, formats, and structures, all without disturbing existing data or structures. The data vault architecture ensures both scalability and high performance. Techniques like parallel loading and hash keys optimize the data loading process, improving the efficiency of BI pipelines. Data vault goes a step further by preserving data in its original, unaltered state, thereby safeguarding the integrity and quality of data. Additionally, it allows users to apply further data quality rules and validations in the information layer, guaranteeing that data is perfectly suited for reporting and analysis. Learn whether you really need data vault. Information marts are an extension of the data vault in the information layer. They bridge the gap between the raw data and the business insights by offering a fast, reliable, and user-friendly way to access, analyze, and visualize the data. Moreover, data vault allows users to optimize information marts for reporting and analysis by applying various transformations, aggregations, calculations, and filters to tables. Information marts can also include additional data sources outside the data vault, such as external or unstructured data. Information marts enable analytics teams to leverage historical data for analysis by accessing the full history of changes and transactions stored in the data vault. This allows them to perform time-series analysis, trend analysis, data mining, and predictive analytics. Similarly, information marts can also support different types of analysis, such as descriptive, diagnostic, prescriptive, and exploratory, by providing different levels of detail, granularity, and dimensionality. Information marts are flexible and agile, as they can be easily created, modified, or deleted without affecting the data vault or other information marts. How Information Marts Enable Agile, Scalable and Accurate BI Ecosystems Information marts also play a vital role in enhancing three key aspects of BI: scalability, agility, and accuracy. Here’s how: Scalability through Data Segmentation: Information marts segment data to cater specifically to the needs of different business units or departments. Each mart operates independently, allowing for modular scalability. By dividing data into manageable segments, information marts facilitate scalable BI. As the organization grows, new or existing marts can be added, ensuring scalability without overhauling the entire BI infrastructure. Agility via Tailored Data Delivery: Information marts offer tailored datasets, allowing users to access and analyze information that aligns precisely with their requirements. This tailored approach is central to agile BI practices. Users can rapidly obtain the insights they need without wading through irrelevant data. This user-centric approach, facilitated by information marts, supports agile methodologies like iterative development and continuous delivery, promoting a responsive BI environment. Accuracy through Data Governance: Information marts empower data owners and stewards to control and maintain data quality within their domains. Governance practices, including data quality rules and security policies, are enforced at the mart level. The accuracy of BI is safeguarded by information marts governance mechanisms. Data quality is upheld, and compliance policies ensure accurate and reliable information is delivered to decision-makers, fostering trust in the BI outputs. The Role of Information Marts in BI Imagine a hypothetical healthcare organization, CareOrg, providing care to a vast patient community. With over 20 hospitals, 260 physician practices, 4500 affiliated physicians, and a team of 25,000, CareOrg is all about boosting the health and happiness of the communities it serves. CareOrg stores clinical data in a data vault, collecting data from various sources such as electronic health records, labs, pharmacies, radiology, billing systems, public health agencies, and contact tracing apps. Inside, there is patient info, medical histories, lab results, treatments, and more. However, the data in this vault is raw and not optimized for reporting and analytics. The data vault and information marts work together to enable the organization to monitor and manage the spread of infectious diseases such as dengue, COVID-19, influenza, tuberculosis, measles, etc. The data vault integrates data from different sources and preserves the history and lineage of the data. The information marts provide a tailored view of the data for each disease, focusing on key metrics such as infection rates, mortality rates, vaccination rates, risk factors, and outcomes. This is important because it helps the organization track the trends and patterns of infectious diseases, identify high-risk populations and regions, evaluate the effectiveness of interventions and policies, and improve the quality of care and prevention. For example, the data vault blends information from diverse sources like electronic health records, claims, surveys, social media, and wearable devices in managing an outbreak. On the other hand, information marts help create specialized analytical reports for each disease, showing the current situation and projections of the outbreaks. The dynamic duo of data vault and information marts helps the organization enhance the population health management for various infectious and chronic diseases. This helps the organization detect outbreaks more quickly, manage chronic diseases, and create targeted plans for each group. Think of it like having personalized health plans for different groups, all based on what the data says. Other Real-World Use Cases Information marts have been successfully used by many organizations across various industries and domains for BI purposes: Marketing Analytics: A retail company uses an information mart to analyze its marketing campaigns across different channels, such as email, web, social media, or mobile. The information mart contains metrics such as impressions, clicks, conversions, revenue, cost, ROI, etc., as well as dimensions such as campaign, channel, product, customer segment, location, etc. The company uses this information mart to measure the effectiveness of its marketing strategies, optimize its marketing mix, segment its customers, personalize its offers, etc. Sales Forecasting: A manufacturing company uses an information mart to forecast its sales for the next quarter based on historical trends and current opportunities. The information mart contains metrics such as sales volume, sales value, sales growth, margin, etc., as well as dimensions such as product line, product category, product type, customer industry, customer region, etc. The company uses this information mart to apply various models or scenarios to predict its sales performance, identify potential risks or opportunities, allocate resources accordingly, etc. Risk Analysis: A financial institution uses an information mart to perform a risk analysis on its portfolio of loans across different countries and sectors. The information mart contains metrics such as exposure amount, default probability, loss given default, expected loss, etc., as well as dimensions such as loan type, loan status, loan rating, country, sector, etc. The institution uses this information mart to perform various calculations or simulations to assess its risk profile, manage its capital adequacy, mitigate its credit risk, etc. A Final Word Information marts are indispensable assets in BI. They help organizations leverage data warehouse as a reliable repository for analysis and reporting in the face of increasing volumes of data and evolving business rules. Meanwhile, organizations remain compliance ready and maintain a rich source of historical data for accurate analysis and forecasting. Are you looking for a no-code solution to create and manage your business intelligence (BI) pipelines? LIKE.TG is a unified data management platform that lets you build your own data vault and information marts within minutes. Learn more about LIKE.TG and how it can help you turn data into insights at lightning-fast speed. Click here to start your free trial or schedule a demo.
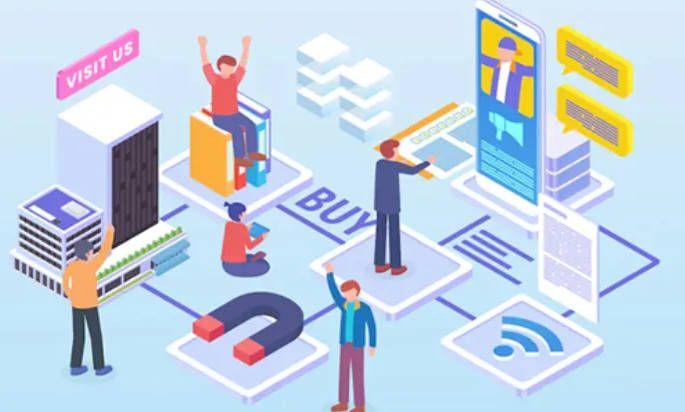
What is Data Modeling?
Data modeling is the process of structuring and organizing data so that it’s readable by machines and actionable for organizations. In this article, we’ll explore the concept of data modeling, including its importance, types, and best practices. What is a Data Model? A data model is a framework that organizes data elements and standardizes how they relate to one another. Data shapes everything from scientific breakthroughs to the personalized experience of streaming services. It informs how businesses grow, influences public policies, and even affects the entertainment that pops up on our screens. But raw data is like an uncut diamond – valuable but needing refinement. Data models help us understand and utilize raw data within any system. They outline the various data elements, such as customer details or transaction records, and illustrate the connections between them. Therefore, a data model is not just a visual aid that brings clarity to vast and complex data but also a strategic tool that shapes the way data is configured for optimal use. A well-designed data model can help organizations improve operations, reduce costs, and make better decisions. What is Data Modeling? Data modeling is the process of creating data models. It acts as the refining process for raw data as it defines its structure and attributes and transforms it into an actionable resource. Data modeling involves creating a detailed visual representation of an information system or its components. It is designed to communicate the connections between various data points and structures. This process encompasses the analysis and definition of the diverse types of data that a business gathers and generates, focusing on their intricate relationships, groupings, and organizational structure. With a combination of text, symbols, and diagrams, data modeling offers visualization of how data is captured, stored, and utilized within a business. It serves as a strategic exercise in understanding and clarifying the business’s data requirements, providing a blueprint for managing data from collection to application. Data Modeling Concepts: Types of Data Models The classification of data models into distinct types is a strategic approach that reflects the evolving stages of data representation and management within an organization. It serves a sequential purpose in the data modeling process; from providing a high-level abstraction to delving into specific details to translating logical structures into tangible implementations. Here are the three types of data models: Conceptual Data Models At the outset, conceptual data models provide a high-level representation of the organizational data, catering to a specific business audience. They focus on the overall structure of data in relation to business objectives without diving into technical specifics. It outlines the general relationships between entities and illustrates how they interact within a system. The conceptual layer is built independently of any hardware specifications, storage capacity, or software limitations. The goal is to represent the data as viewed in the real world. For example, a conceptual model in a hospital information system could outline the relationships between patients, doctors, and appointments. Logical Data Models Following the conceptual model, a logical data model provides a more detailed view of data elements and their interrelationships. This layer is more complex and structured than the conceptual layer. The logical model lists the project’s requirements, but it could also integrate with other data models, depending on the scope. It is designed and developed independently from DBMS, and the data types of the data elements have a precise length. Logical data layer contains information about how the the model should be implemented and outlines specific attributes of data elements. For instance, in a customer relationship management system, logical modeling details how customer records relate to purchase orders and support tickets, including attributes like customer ID, purchase history, and interaction logs. Physical Data Models Finally, physical data models focus on the technical aspects of database design, defining how the data model will be implemented in the database. The physical data model diagram helps visualize the entire structure of the database. It contains the table of relationships between the data, addressing the nullability and cardinality of said relationships. Physical data models are designed and developed specifically for a specific DBMS version, the technology used for the project, required data storage, and location. A physical data model specifies the structure of tables, the relationships between them, and indexes for efficient data retrieval. For example, in a SQL database, physical data modeling defines how tables are structured and how they relate to each other. Together, these data models simplify the way data is organized and understood by data professionals. Discover the Data Model Centric Approach to Data Warehousing Download Whitepaper The Data Modeling Process: How to Create a Data Model Data modeling is a structured process that involves a set of techniques for representing data comprehensively. It includes several sequential tasks conducted repetitively to ensure that the data is organized efficiently and caters to the business requirements. As part of the process, start by engaging the relevant stakeholders to understand the purpose of the data model. It should provide answers to questions like: What data is needed? How will it be accessed? What questions should it answer? What problems will it solve? Define the scope to avoid unnecessary complexity. Identifying Entities The initial step of the data modeling process is to pinpoint the components or main objects, called entities. Entities are representations of actual objects, concepts, or events found in the data set which needs modeling. These could be tangible things like customers or abstract concepts like transactions. It’s important to ensure that each entity is unique and logically distinct from others, as it prevents data confusion and maintains the data model clarity. Defining Attributes After identifying an entity, the next step is to define its unique properties, which are called attributes. For instance, for modeling a product entity, the attributes can be product name, price, manufacturer, and description. Analysis of these attributes can give a deeper understanding of each entity’s inherent characteristics. Specifying the Relationship The next step in the data modeling process is defining the relationships between different entities. Different notations and conventions, such as the Unified Modeling Language (UML), are used to define these relationships and to visually represent and document the connections between entities and their attributes. When defining relationships between entities, it is also important to consider their cardinality, i.e., whether the relationship will be one-to-one, one-to-many, many-to-one, or many-to-many. Additionally, For example, while modeling a student and course entity in a university database, the relationship could be such that each student can be enrolled in multiple courses (one-to-many relationship). This will depict how students and courses are interconnected in a data model. Mapping the Attributes to Entities Mapping the attributes to entities is all about ensuring that the data model clearly reflects how the organization will use the data. Based on their specific requirements, developers can apply formal data modeling patterns, such as analysis patterns or design patterns. Consider, for instance, an online store with entities like customer and order. The customer entity typically has attributes like name and address, while the order entity has attributes like order date and total amount. The attributes must be linked to the appropriate entity to demonstrate how customer information and orders are handled precisely. Depending on the situation, patterns like Customer-Order can be used to optimize the data model. Assigning Keys Assigning keys (numerical identifiers) is carefully selecting the appropriate type of key (primary, foreign, etc.) for each entity based on the nature of the data and the requirements of the database. While assigning keys, it’s important to strike the right balance between reducing redundancy and meeting performance requirements. This is done through normalization, which assigns keys to data groups to represent relationships without repeating information. For instance, while creating a data model for a library database, instead of typing out the author’s name on every book, give each author a unique number, like an author ID. Then, link this ID to each book to indicate the author. This way, the author’s name will not be repeated for every book, making it easier for readers to find books by the same author. Keep in mind that normalization involves a trade-off between reducing redundancy and increasing complexity. In some cases, denormalization might be considered for performance reasons, but it should be done judiciously to avoid introducing data update anomalies. Finalizing the Data Model The data modeling process is constantly evolving to adapt to the changing needs of the business. Therefore, conducting regular reviews through scheduled assessments and feedback from stakeholders is essential. Make refinements as necessary to ensure that the data model aligns with the organization’s objectives. For example, while managing company finances is simple at first, as the business grows, its financial data grows. In this case, updating the financial model regularly is necessary to include newer pieces of data. It ensures that the data model always reflects the complete financial picture and helps in accurate financial planning. Types of Data Modeling Techniques Data modeling techniques have evolved over the years with advancements in technology. The following section delves into prominent types of data modeling techniques, each offering unique perspectives and methodologies for representing the relationships between data entities and their interactions within a given system. There are various types of data modeling: Traditional Data Modeling Techniques These are the techniques used extensively in the early days of data modeling, but they are still in use today. Hierarchal Data Modeling In hierarchical data modeling, a single parent node is connected to multiple child nodes, creating a branching structure that resembles a tree. Each parent node can have multiple children, but each child node has only one parent, creating a clear and organized data hierarchy. At the top level of the hierarchy, there is the root node, which represents the main entity, and each branch represents a different data entity that is connected to the root node. For example, in an organization’s database, the CEO entity is the root, with Department Managers as children, who in turn have Employees as their children. Relational Data Modeling Relational data modeling is a method to design databases based on the principles of relational model. This model organizes data into tables having rows and columns and allows for various types of relationships such as one-to-one, one-to-many, and many-to-many. The key feature of the relational model is that it links data across tables using common data elements or keys. This enables efficient and effective data management and easy access and retrieval of information. The relational model also facilitates data analysis and reporting, making it a valuable tool for businesses and organizations. For example, a database for a bookstore can have separate tables for ‘Books’, ‘Authors’, and ‘Publishers’, with relations established through keys like ISBN for books, author IDs, and publisher IDs. Network Data Modeling The network data model is another common way to structure data in a database. Extending the concepts of hierarchical data modeling, network data modeling allows entities to have multiple parent-child relationships. The resulting web-like structure is not only sophisticated but also highly flexible. Think of it as nodes (data objects) connected by edges (relationships). For instance, in a company database, employees and projects could be nodes, with edges indicating which employee works on which project. This model offers flexibility for managing intricate data relationships. Object Oriented Data Modeling The object-oriented data model combines elements from object-oriented programming and relational databases. Object-oriented data modeling extends other data modeling methods by representing data as objects, thereby encapsulating data and the methods or operations that can be performed on that data. These objects have their own properties (attributes) and actions (methods), and they can be connected to each other. Consider a Car object with properties like color and speed, and actions like start and stop. Now, for a Sports Car, it can be based on the Car object and adds specific features like turbo boost. Widely Used Data Modeling Techniques Today There are a few data modeling techniques which are popular and are most widely used now a days. Dimensional Data Modeling Dimensional data modeling is used to structure data for analytical and reporting purposes. It organizes data into two types of tables: fact tables and dimension tables. Fact tables store numerical measures, while dimension tables store descriptive information. The dimensional modeling approach enables efficient querying and analysis for business intelligence, simplifying complex data for meaningful insights. For example, in a retail sales data model, the fact table might contain sales revenue, quantity sold, and date, while the dimension table could store details about products, customers, and stores. Entity Relationship (E-R) Data Modeling Entity relationship (E-R) is used to design databases by defining entities (objects) and their relationships. Entities represent real-world objects, and relationships depict how these entities interact. For instance, in a university database, student and course are entities, and the relationship enrollment connects students to the courses they are taking. Data Vault Modeling It is a way to design data warehousing that’s optimal for handling large amounts of data, an organization’s changing needs (changing data sources and structures), and keeping track of past data by using hubs, links, and satellites. Data vault modeling focuses on agility and in managing and integrating enterprise data. For instance, a healthcare provider can use it to integrate patient data from multiple sources, maintaining a historical record of patient interactions. Graph Data Modeling Graph data modeling shows data like connected dots and lines, making it ideal for understanding and working with complicated relationships and networks. For example, a social media platform can use graph modeling to analyze and visualize the connections and interactions between millions of users. Specialized Data Modeling Techniques There are certain data modeling techniques that can be used for very specific use cases, each offering unique benefits. For instance, object-relational models display advanced functionalities of their own along with the simplicity of the relational model. This type of data model is considered to be a hybrid model that allows modelers to incorporate new objects in a familiarly structured environment. Multidimensional data modeling is used in online analytical processing systems to model data in multiple dimensions for in-depth analysis. For example, a retail business can use it to view sales data by product, region, and time, offering a comprehensive analysis of sales trends. Temporal data modeling is ideal for handling data that changes over time. A financial institution, for example, can use temporal data modeling to track fluctuating interest rates over years, providing insights into market trends and helping in future strategy planning. Machine learning model design is for predictive analysis. For example, a retail business can use this to predict customer purchasing behavior based on historical purchase data and improve inventory management. Finally, conceptual blending involves combining different concepts for innovation. For instance, a tech firm can use this approach to blend concepts from different industries to create a new product, like using gaming technology in educational software. Benefits of Data Modeling Data modeling structures data in a way that enables business owners and leaders to access and utilize it efficiently. It turns data into a valuable tool for gaining insights and making informed decisions. Integrating Diverse Systems Integrating disparate systems is a challenge for many organizations, especially when data silos exist across multiple platforms. Data modeling acts as a bridge that connects these isolated systems by establishing a common framework and set of rules for how data is structured and related. Implementing a data model standardizes data formats, terminologies, and relationships across different systems. As a result, data teams can address the inconsistencies and discrepancies that hinder data integration. Essentials of Database Design In designing databases, data modeling serves as a fundamental framework that shapes the way databases and repositories are conceptualized and built. The process involves a detailed, multifaceted approach to describing and structuring data tailored to meet a business’s specific needs and objectives. This way, businesses can build databases that support efficient data retrieval and analysis, essential for tasks like customer relationship management or targeted marketing. Additionally, a well-designed data model anticipates future needs and scalability, allowing for adjustments and expansions in the database design as business needs evolve. This foresight in design ensures that the database remains an adaptable asset over time. Effective Business Intelligence Data modeling is a critical step in ensuring that the information a business uses is well-organized and easy for BI tools to handle. It maintains the effectiveness of BI strategies and tools by categorizing data into a format that is easily processed by BI systems. Data modeling keeps the data relevant and logically organized, making it easier to identify patterns, analyze spending trends, and engage in predictive analysis. So, when businesses leverage BI tools, they can quickly uncover meaningful insights and make informed decisions based on a solid and comprehensible data framework. Efficient Development Modeling data reduces the complexity of software development as it provides a clear and detailed roadmap. Data modeling tools automate the process of creating and maintaining database schemas, streamlining the entire development process. Developers can also share data models seamlessly, ensuring everyone is on the same page. This shared understanding is critical when changes need to be made. Whenever an alteration is made in the data model, data modeling tools automatically propagate it to the database, eliminating the risk of inconsistencies or errors. This clarity accelerates the development process and enhances the quality of the product. In fact, systems developed under the guidance of a well-structured data model have fewer bugs and are often delivered faster. Facilitates Cloud Migration Data modeling helps with migrating data to the cloud smoothly by creating a clear and organized blueprint of how the data is structured. The blueprint includes details about the relationships between different pieces of data, making it straightforward to map and adapt the structure to fit the requirements of a cloud environment. In essence, it aligns the database design with the requirements of cloud environments. Streamlines External Communication Data modeling also enhances communication with external stakeholders by providing a visual representation of how data is structured and related with a system. Data models serve as a common language that facilitates discussions about data requirements and overall project understanding. Sharing these models helps convey complex information in a more understandable and standardized format enhances effective communication and understanding between organizations and external stakeholders. Integrating a data model with BI further enhances communication, both internal and external. It ensures that the data-driven insights derived from BI tools are presented in a clear, concise manner that aligns with standardized definitions and structures. This is particularly important when sharing insights with external parties who may not be familiar with the organization’s internal data systems. Data Modeling Best Practices There are a few best practices that can optimize the overall data modeling process andensure that the resulting data model is effective. Align the Process With Business Goals Plan the data modeling process so that the data model is aligned with business objectives. Collaborate with business analysts and stakeholders to ensure the model accurately represents business processes.This way, the data model directly supports business strategies and decisions, enhancing its relevance and effectiveness. Maintain Comprehensive Documentation Adequate documentation of the data model is essential for clarity about the database structure and ensures its usability for developers and stakeholders. It should detail what data is stored, how it’s used, and its relationships.Comprehensive documentation simplifies the understanding and utilization of the data model, facilitating smoother onboarding of new team members and helping in future modifications. Select The Appropriate Modeling Technique Choose a data modeling technique that best suits the data type and use case. For instance, use relational modeling for end-user applications and dimensional modeling for analytics-focused tasks.Opting for the right technique maximizes efficiency and effectiveness, ensuring the data model is optimally structured for specific business needs and use cases. Use Clear Consistent Naming Conventions Using clear and simple naming conventions makes data models easier to read and maintain. It simplifies database development and reduces confusion among team members. For example, using names like customer_id instead of confusing abbreviations like CustID makes it clear what the field means and helps with understanding and collaboration. Strive For Simplicity In data modeling, it is important to focus on what’s essential right now rather than creating an overly complex solution. For example, a small business should model for current scale, which involves managing gigabytes of data with moderate user traffic, instead of over-engineering for the future, which may require handling terabytes and heavy traffic. This practice can save valuable time and resources by concentrating on immediate needs. Additionally, it ensures that the data model is not overly complicated and remains agile enough to adapt as business evolves. Use Flexible Data Models for Future Adaptation Creating flexible data models enables databases to adapt to changing business needs. The additional flexibility makes it easier to add new data sources or make changes to the data structure. For example, using a flexible schema in a NoSQL database allows adding new data attributes easily and without causing issues with existing data. Incorporate Data Governance Security When designing data models, it is important to give data governance and security their due share of importance right from the start. Doing so ensures that data is stored, accessed, and managed in accordance with rules and security guidelines. For instance, by using role-based access control (RBAC) in the data model, access to sensitive data is limited to authorized people, making data more secure and compliant with regulations. Final Word Data modeling is significant in integrating systems and supporting business intelligence. Applying the right data modeling techniques can lead to improved efficiency, clearer communication within teams, and better decision-making. Make the most of this process with LIKE.TG DW Builder. Streamline data modeling and warehousing for seamless data integration with our intuitive and easy-to-use interface. Start your data modeling journey with a free 14-day trial. Build a Data Warehouse in Days - Without Any Coding! Try LIKE.TG DW Builder for free for 14 days! Simplify data integration and get real-time insights effortlessly. Request Free Trial
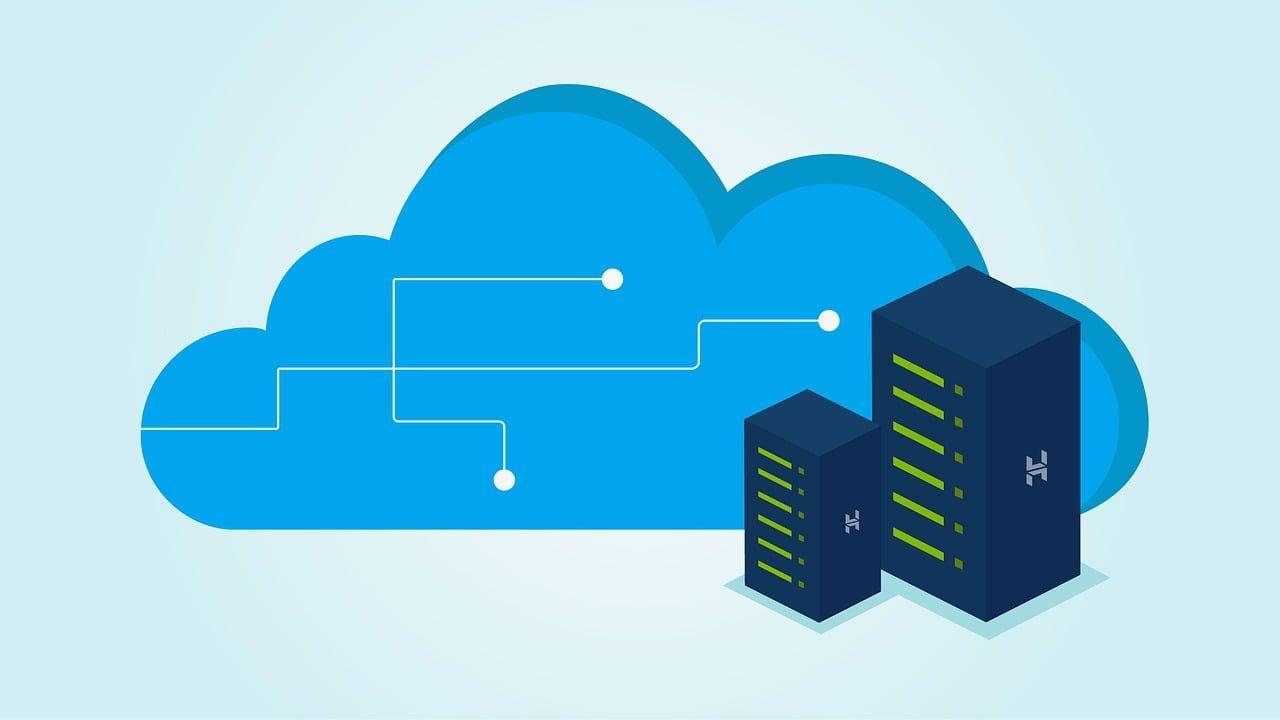
Top 10 Matillion Alternatives In 2024
ETL tools have become popular due to their automation capabilities and ease of use. They enable non-technical people to create workflows from scratch. However, the multitude of ETL tools available makes it difficult to select the most suitable one. One of the software in this sphere is Matillion. But why do teams select it? What are its key features, and what are some good Matillion alternatives? Key features of Matillion Matillion, established in 2011 in Manchester, UK, is a cloud-based ETL tool. Its core mission revolves around streamlining data integration and enhancing business intelligence. Matillion’s ETL solution emphasizes sturdy transformations and diverse connectivity options. Users benefit from ready-made connectors, community-driven ones, and the ability to craft their custom connectors. Matillion offers compatibility with prominent cloud platforms like Amazon, Google Cloud Provider, and Microsoft Azure. Its pricing model operates on three tiers – basic, advanced, and enterprise – utilizing a credit-based system. Its core features are: Drag-and-drop interface: Matillion features a drag-and-drop interface. Visual job development: You can visually design data pipelines using pre-built components. Live feedback and data previews: As you build pipelines, Matillion provides real-time feedback and data previews. Abundant connectors: Matillion offers a variety of connectors to popular data sources. ELT/ELT hybrid model: Matillion supports both ETL and ELT. Push-down ELT technology: Matillion utilizes push-down ELT technology, which pushes transformations down to the data warehouse for efficient processing. Automation and scheduling: You can automate data pipelines and schedule them to run at specific times. Security and compliance: Matillion offers features like encryption and audit trails. The Need for Alternatives Why look for Matillion alternatives? Although Matillion is a good ETL tool, there are other options that might be better in certain scenarios. Its version control features are limited compared to some other software and the credit-based pricing model isn’t for everyone. It’s also not fully no-code, which might discourage non-technical users and require some learning. Pricing Matillion’s pricing structure, while flexible with different tiers catering to varying needs, might not align with budget constraints for some smaller-scale projects or startups. The credit-based model could become expensive if not managed efficiently, potentially making it less feasible for organizations with stringent financial boundaries. Support According to users on third-party review sites, Matillion adopts a more hands-off approach to customer support. The tutorials are also lacking detail. This increases the learning curve of the tool and time-to-insight. Look for vendors that offer robust documentation and high-quality support, highlighted by industry awards, while adopting an expensive software for sensitive tasks like data migration and ETL. Scope Another important feature of an ETL tool is its scope, like the number of in-built transformations and quality of integrations. Several other Matillion alternatives, for example, offer better Git Integration. For exceptionally large-scale projects or those requiring highly complex data transformations, Matillion’s performance might face challenges. While it’s robust, there are limitations in handling extensive data volumes or extremely intricate transformations efficiently. Top 10 Matillion Alternatives LIKE.TG LIKE.TG is an established solution with award-winning ETL/ELT capabilities and one of the best customer support teams in the market. It is trusted by many Fortune 500 companies and SMEs for their ETL initiatives. LIKE.TG streamlines data integration with a fully no-code approach, empowering business users to execute their data management tasks easily – while saving considerable resources. The pricing is also flexible where you pay only for the modules you use. Plus, LIKE.TG provides no-code unstructured data management, data warehouse building, and API management capabilities. These features come on top of its robust ETL/ELT engine. LIKE.TG’s key features include: No-code data pipeline builder with a drag-and-drop UI. A vast library of pre-built connectors. Built-in transformations for every major use-case. ETL and data mapping automation based on triggers and time intervals. Data quality checks and data profiling. Pushdown optimization. Advanced Git Integration features. Real-time data preview. Simplified collaboration features. No-code API Management. Here’s a high-level product overview of LIKE.TG: End-to-end No-code Data Management Looking for the best Matillion alternative? With LIKE.TG, get data extraction, transformation, integration, warehousing, and API and EDI management in a single platform. Download Your Free 14-dayTrial! Talend Talend Data Fabric is a comprehensive data management platform that aims to unify data integration, data quality, and data governance in a single, easy-to-use solution. It helps organizations break down data silos, improve data quality, and make trusted data available to users across the organization. It also enables users to handle complex data integration tasks across various systems Best Use Case: Talend is versatile and suitable for businesses requiring an open-source or hybrid approach to data integration. It can be an ideal solution for enterprises with diverse data sources and intricate transformation needs. Cons: It can be difficult to use with a steep learning curve. Its important features are locked behind an expensive premium tier. Informatica Informatica PowerCenter is a comprehensive data integration platform that allows businesses to extract, transform, and load data from various sources into target systems, ensuring data quality, consistency, and accessibility. Informatica’s comprehensive suite of tools excels in managing complex data environments, making it a go-to choice for large organizations dealing with vast volumes of structured and unstructured data across different systems. Best Use Case: Informatica is well-suited for large enterprises and industries with stringent regulatory compliance requirements. Its robustness lies in its ability to handle sensitive data and comply with complex data governance and security standards. Cons: It is expensive and might not be suitable for niche use cases. It can also be difficult to use, even for Informatica-certified professionals. SAP Data Services SAP Data Services is an enterprise-level data integration and transformation software offered by SAP. It serves as a comprehensive solution for data integration, data quality management, and data processing tasks within organizations. Best Use Case: SAP Data Services is an excellent fit for businesses heavily invested in SAP systems and enterprise applications. Its integration capabilities with SAP ecosystems are unparalleled, making it ideal for organizations seeking seamless ETL within their SAP landscape. Cons: Being an established solution from a large company, the cost is very high, especially for SMEs. It’s also more geared towards developers, leaving business users at a disadvantage. Additionally, error descriptions are very vague. AWS Glue AWS Glue is a fully managed ETL service provided by Amazon Web Services (AWS). It’s designed to make it easier for you to prepare and load your data for analytics and data-driven insights. Best Use Case: AWS Glue is well-suited for companies leveraging Amazon Web Services (AWS) extensively. Its native integration with AWS services makes it an excellent choice for businesses aiming to benefit from the cloud environment while staying within the AWS ecosystem. Cons: AWS Glue has limited connectivity to non-AWS technologies. Since it’s a serverless architecture, it can be expensive to handle. It also requires knowledge of Apache Spark. Google Cloud Dataflow Google Cloud Dataflow is a fully managed service provided by Google Cloud Platform for stream and batch processing of data. It allows you to design, deploy, and monitor data processing pipelines at scale. It’s built on Apache Beam, an open-source unified programming model for both batch and streaming data processing. Google Cloud Dataflow’s scalability and integration with Google Cloud Platform services make it a preferred choice for companies invested in GCP. Best Use Case: It’s ideal for real-time data processing and streaming. It is suitable for organizations requiring continuous, near real-time data analysis and transformation. Cons: Some users have found the technical support to be inadequate. Performing detailed transformations is also complex. Microsoft Azure Data Factory Azure Data Factory is Microsoft’s cloud-based data integration service that facilitates building, scheduling, and orchestrating complex data workflows. It offers a scalable and flexible platform for seamless data movement and transformation within the Microsoft Azure ecosystem. Best Use Case: It’s well-suited for organizations deeply integrated into the Microsoft ecosystem. It’s a good choice for businesses leveraging Microsoft Azure services and seeking seamless data movement and transformation within Azure environments. Cons: It also faces problems performing complex transformations and workflows. Like AWS Glue, it has limited connectivity with non-Azure services. The monitoring and logging capabilities are also lacking. Pentaho Pentaho is an open-source business intelligence tool that includes data integration, visualization, and analytics capabilities. Its open-source foundation, scalability, and ease of use make it a valuable tool for organizations of all sizes. Pentaho’s visual tools and community support make it a great fit for organizations aiming for quick deployment and ease of use without compromising on functionalities. Best Use Case: It’s particularly advantageous for small to mid-sized businesses looking for cost-effective yet powerful data integration solutions. Cons: Pentaho’s reporting features can be made better according to some users. Its web interface has also received criticism due to a lack of functionality. Some of Pentaho’s customers have also reported frequent time-out errors. Apache NiFi Apache NiFi is an open-source data integration tool that facilitates the automation of data flow between various systems. It provides a visual interface for users to design, control, and manage the movement of data in real-time. Originally developed by the United States National Security Agency (NSA), NiFi became an Apache Software Foundation project in 2014. Best Use Case: It excels in handling data flows and data routing scenarios, making it ideal for IoT (Internet of Things) environments, where it efficiently manages data streaming from numerous devices. Its visual interface and adaptability suit scenarios requiring dynamic and real-time data routing. Cons: Many users face difficulties managing a large number of flows. Apache NiFi also doesn’t efficiently process a large amount of small files. Alteryx Alteryx is a self-service data preparation and analytics platform, ideal for data analysts and business users. Its strength lies in its user-friendly drag-and-drop interface that allows you to perform complex data blending, cleaning, and analysis without extensive coding knowledge. Best Use Case: Alteryx is best suited for organizations seeking to empower business users to independently perform data transformations and analytics tasks. It is also a good option for self-service data preparation. Cons: Alteryx users must get a separate expensive server for automating pipelines. It also has a steep learning curve, and it lacks clarity in terms of error messages and debugging. LIKE.TG – The Best Way to ETL Selecting the right data integration solution can be overwhelming, especially given the multiple options available. It involves weighing factors such as product features, ease-of-adoption, total cost of ownership, and customer support, which takes a lot of time and research. Considering its importance, the choice of ETL tool can make or break an organization’s BI initiatives. With its robust features and support, LIKE.TG is one of the best Matillion alternatives in the market. It provides a very flexible up-front pricing structure. Users also receive full-support during the installation process and extensive product training, if needed. With LIKE.TG, you can also get: A data cleansing and data extraction module – fully no-code. A no-code data warehouse builder. A no-code API Management solution. Try LIKE.TG out now for yourself with a free 14-day trial and experience a simple way to ETL! Build Data Pipelines Effortlessly With LIKE.TG’s Automated Data Integration Solution Building and maintaining ETL/ELT pipelines does not have to be complex and time-consuming. Leverage LIKE.TG's 100% no-code solution for seamless data integration. Get Your Free Trial!
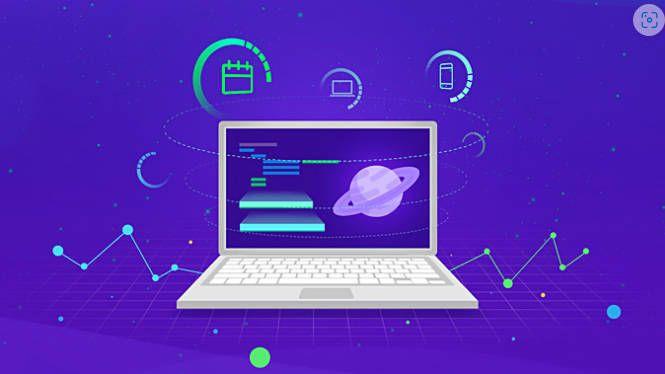
The Best Fivetran Alternatives in 2024
Fivetran is a low-code/no-code ELT (Extract, load and transform) solution that allows users to extract data from multiple sources and load it into the destination of their choice, such as a data warehouse. The fact that it focuses on data movement via ELT only, businesses with broader data integration and management requirements look for Fivetran alternatives. So, in this article, we will explore some of the best alternatives to Fivetran. But first, let’s get some basics out of the way. Fivetran Features Here are some of the key features of Fivetran: Built-in connectors for various files, applications, databases, and data warehouses SQL transformations supported through dbt core Pre-built data models Volume based pricing No-code setup Local data processing Take the first step to No-Code Data Management with LIKE.TG Whether you want to extract unstructured data in bulk, integrate data from disparate source, or build a data warehouse from scratch, LIKE.TG's 100% no-code platform empowers you to do it all. Download Trial Fivetran Limitations While Fivetran is a viable tool for basic ELT projects, it does come with some limitations when it comes to broader data integration projects, especially in large enterprises. Here are some of its limitations that prompt organizations to look for Fivetran alternatives: The first thing that you should know about Fivetran is that it is only meant for ELT. So, in case your data requires extensive transformation or cleaning, Fivetran is not the ideal solution. Fivetran might be a viable solution if your data is already in good shape, and you need to leverage the computing power of the destination system. Fivetran offers volume-based pricing and charges you based on monthly active rows (MARs). While this might be a good start for small enterprises, the costs can quickly go out of control. You need to keep a check on the usage since accurately predicting the incurred costs is a hassle. As far as the destinations are concerned, Fivetran supports data warehouses and databases, but it doesn’t support most data lakes. Fivetran is also not an ideal solution if you are looking for a complete enterprise-grade data management solution as it doesn’t support data governance or offer advanced capabilities to improve data quality. It also offers limited data transformation capabilities and that too through dbt core, which is an open source tool. While it enables you to conduct complex transformations in your destination through SQL statements, the constant switching between different interfaces means it’s not really a unified solution. 7 Best Fivetran Alternatives in 2024 Due to its inherent limitations, many businesses often seek Fivetran alternatives. We have listed some other data integration tools and platforms that can be a great replacement for Fivetran. 1. LIKE.TG LIKE.TG is a 100% no-code data management platform that has been designed with a focus on accessibility for business users. The platform is built on an industrial strength ETL/ELT engine that can easily process large volumes of data, delivering analysis-ready data for decision-making. With LIKE.TG, you get: A visual drag-and-drop interface that allows users to easily build data pipelines within minutes. AI-powered data extraction capabilities that can extract data from PDFs, text files, spreadsheets, and other unstructured data sources, for example, purchase orders and invoices. Built-in connectors for various file formats (Excel, JSON, Parquet, Avro, and more), databases (Amazon Redshift, Oracle, Netezza and more), Cloud storages (SharePoint, Blob storage and more) data warehouses (Snowflake, Amazon Redshift, etc.) and data lakes (Amazon S3 and Azure Data Lake). It also supports various applications natively as well as through its custom connectors. Various advanced transformations and functions that allow you to manipulate data however you like. All you need to do is just drag and drop the transformations in the data flow designer. AI-powered auto mapper that automatically maps fields from source to destination. Powerful data quality features that allow you to easily cleanse, de-duplicate and validate your data based on your business rules. Change data capture (CDC) for all relational databases in one platform. Workflow automation and process orchestration. Award-winning customer support. LIKE.TG also offers components for building a data warehouse from scratch, managing APIs, as well as EDI management. As far as pricing is concerned, LIKE.TG operates on a license-based pricing model, which means you only pay one time annually regardless of the volume of the data or the connectors you are using. It also provides full visibility into how much you will pay. Additionally, LIKE.TG offers a 14-day free trial so that you can see if it fits your use-case before you commit. Together, these features and offerings make LIKE.TG stand out from the rest of the Fivetran alternatives. Take the first step to No-Code Data Management with LIKE.TG Whether you want to extract unstructured data in bulk, integrate data from disparate source, or build a data warehouse from scratch, LIKE.TG's 100% no-code platform empowers you to do it all. Download Trial 2. Talend (acquired by Qlik) Talend, now acquired by Qlik, is a data integration platform. It’s known for its open-source version, Talend Open Studio, as well as its commercial offerings like Talend Data Integration and Talend Cloud. Here are some key features of Talend: Talend Open Studio is an open-source version that allows you to get started with ETL processes at no cost. It can be a viable solution if you work in a small business. However, being a free tool, Talend Open Studio has quite a lot of limitations. As far as the commercial license is concerned, Talend is on the pricier side. Talend includes features for data quality and governance, allowing you to address data quality issues during the ETL process. The tool comes with a broad set of connectors for various databases, cloud platforms, and applications. The platform provides a visual design interface for building data integration jobs. You can easily design and orchestrate complex workflows. Talend provides version control features, so you can manage and track changes to your data integration jobs over time. Users new to ETL tools or those without a strong technical background might find that the learning curve is rather steep. The tool’s extensive capabilities and features can be overwhelming for beginners, prompting them to look for Talend alternatives. 3. Stitch Data Next up in our list of Fivetran alternatives is Stitch that started in 2018 and was later acquired by Talend. It is a cloud-based ELT tool that is a better fit for small enterprises. Here are some key features of Stitch data: Stitch data is good for replicating data from databases and applications. The tool supports basic transformations but for more complex transformations, you need to use Talend, making the process less intuitive. Stitch supports various connectors for popular databases, SaaS applications, cloud platforms, and more. For connectors that are not supported by the tool, you can create custom integrations, but you need to be familiar with Singer, which can complicate the process for business users. It can automatically detect changes in source data schemas and adjust the destination schema accordingly. Stitch is designed to work with cloud-based data warehouses, and its compatibility might be limited if you’re using on-premises data storage solutions. As far as pricing is concerned, Stitch offers three types of data plans: the Standard plan at $100 for a monthly data processing capacity of 5 million rows, and it can scale up to $1250 for a capacity of 300 million rows, however you can still only connect to 1 destination. The Advanced plan costs $1,250/month for only 100 million rows and only 3 destinations. Finally, the Premium plan costs $2,500/month for a billion rows and only 5 destinations. 4. Matillion Matillion, a cloud-based data integration solution, comes in at fourth in our list of Fivetran alternatives. The platform is designed to run on popular cloud platforms such as Amazon Web Services (AWS), Microsoft Azure, and Google Cloud Platform (GCP). Here are some of the key features of the platform: It provides a low-code interface that allows data teams to build and orchestrate data pipelines. Unlike LIKE.TG, it’s not a truly no-code solution. The tool supports various connectors to popular databases, data warehouses and SaaS applications. It also offers version control, allowing you to keep track of all your ETL jobs. Another feature that Matillion offers is that you can save a list of values or variables and use them in another component. Matillion supports post load transformations. You can either create transformation components or you can use SQL statements, which can be tedious. Matillion also offers variable pricing. It offers three different packages: basic, advanced and enterprise costing $2, $2.5, and $2.75 per credit, respectively. And this is where it gets tricky to predict the actual cost that will be incurred. 5. Airbyte Airbyte is an open-source ELT tool that was launched in 2020. The fact that it’s open-source, make it one of the best Fivetran alternatives. Here are some key features of Airbyte: It has a wide range of connectors for sources and destinations including the popular data warehouses, data lakes and databases. What is different about Airbyte connectors is that they are open-source and users can customize them accordingly. However, the users must have a sound technical background. It also comes with a connector builder that allows users to build their own connectors, which is why most of the connectors have been contributed by the community. As Airbyte is an ELT tool, it doesn’t support any transformations. The only way users can transform data is through SQL or through deep integration with dbt. As far as pricing is concerned, Airbyte offers credit-based pricing. Credits are consumed based on volume. 6. Integrate.io Integrate.io is a cloud-based data integration tool with a focus on ETL and data warehouse integration. The company was founded in 2021 and is a result of the merger between Xplenty, FlyData, DreamFactory, and Intermix.io. It is a low-code platform that runs on Amazon Web services, which means it’s not the best solution for organizations that want to empower their businesses users and reduce reliance on IT. Here are some key features of integrate.io: It comes with a visual interface and various transformations such as sort, filter, join etc. The tool supports ELT, ETL, CDC as well as Reverse ETL. It also has inbuilt support for various databases, SaaS platforms, data warehouses, and BI tools. It offers an API generation tool that allows you to connect to data sources that don’t offer APIs. As far as the pricing is concerned, Integrate.io has a different pricing structure for each of its components. For example, there are three packages for ETL and Reverse ETL including starter, enterprise and professional. The starter package starts from $15000. The starting price for ELT and CDC is $159 per month for 5M rows. As for the API generation component, you need to contact the sales team for a custom quote. 7. Hevo Data Hevo Data, the last in our list of Fivetran alternatives, is another ETL/ELT tool that transfers data from source systems to destination. You can use the platform to collect data from various sources and send it to a data warehouse or a database. Here are some key features of Hevo Data: It supports various connectors to popular databases, data warehouses and SaaS applications. It includes 40+ free connectors. With Hevo Data, you can pre-load transformations through Python. The flexibility to execute transformation code for each event within the configured data pipelines. To perform these transformations, you need to edit the properties of the event object received as a parameter in the transform method, which can be complex, and, therefore, time-consuming. Take the first step to No-Code Data Management with LIKE.TG Whether you want to extract unstructured data in bulk, integrate data from disparate source, or build a data warehouse from scratch, LIKE.TG's 100% no-code platform empowers you to do it all. Download Trial Which is the Best Fivetran Alternative? The tool that you opt for depends on your use case and the size and budget of your company. If you are a small enterprise, then you could opt for a tool that offers variable pricing as it can be a viable option to get things going. However, if you are a large enterprise with broader data integration and management needs then you should opt for a complete data management solution such as LIKE.TG. The platform is packed with features and, most importantly, it is easy to use so you can get started with it immediately. LIKE.TG offers an annual license, making it easier to budget. Ready to try LIKE.TG? Download the 14-day free trial today.
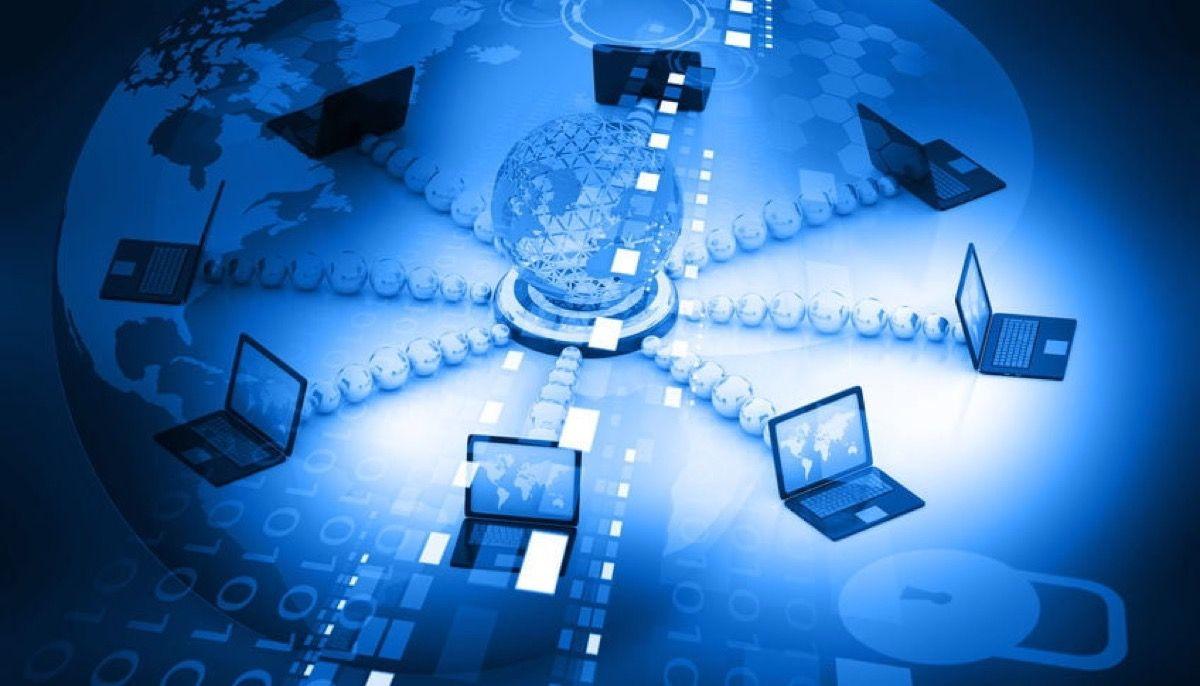
Database Replication 101: Everything You Need To Know
Database replication involves making sure important data is replicated across multiple locations within a company. In the advanced digital age, businesses must take all measures to safeguard their data and ensure its availability at all times. Data availability is important for proactive decision-making and empowering other tech, such as AI. This is where database replication becomes a high priority. But what is database replication exactly? What does it involve, and what are the benefits of replicating databases? Let’s find out. What is database replication? Database replication is the process of creating and maintaining multiple copies of a database across different locations or servers. The main objective of replication is to improve data availability, distribute the workload, and enhance the overall performance of the database system. It also provides redundancy and fault tolerance by ensuring that data is replicated to multiple nodes, whether synchronously or asynchronously. Database replication plays a crucial role in modern data management systems and strategies. It is a fundamental technique used by organizations to ensure data integrity, reliability, and accessibility. It simplifies access to data across multiple teams, and, by making copies, it protects data from tampering and destruction. Database replication is an ongoing process. Continuous replication ensures that changes to the primary database are promptly propagated to replicas, guaranteeing up-to-date data globally. It allows organizations to add new replicas and accommodate evolving schemas. Ongoing replication also facilitates automatic failover and recovery, ensuring seamless transitions during primary database failures. Difference between Data Replication vs Database Replication Before diving further into database replication, it’s important to understand the distinction between data replication and database replication. Data replication refers to copying specific data from one storage device to another, often for backup purposes. This process ensures that in the event of a hardware failure or data loss, the replicated data can be used to restore the original data. On the other hand, database replication involves replicating an entire database, including its schema, tables, and stored procedures, to multiple servers.. Unlike data replication, which focuses solely on copying data, database replication aims to create identical copies of the entire database structure and its contents. Naturally, database replication involves more complexity and intricacies compared to simple data replication. It requires careful consideration of factors such as data consistency, synchronization, and conflict resolution.. Database Replication vs. Mirroring Database replication and mirroring are techniques used to create redundant copies of a database. However, there are some key differences between them. Database replication involves creating multiple copies of a database. As mentioned previously, it distributes the workload and improves performance by allowing applications and users to access data from the nearest replica. The clear advantage of database replication over mirroring lies in the flexibility it offers in terms of data distribution and configuration options. It offers the ability to selectively replicate specific objects or subsets of data within a database. This feature provides more granularity and control over what data is replicated, allowing users to tailor the replication process to meet specific needs.. On the other hand, mirroring involves creating an exact copy of the database on another server, known as the mirror server. The mirror server remains synchronized with the primary server through continuous data transfer. In the event of a failure on the primary server, the mirror server can take over seamlessly. One thing to keep in mind is that while mirroring “mirrors” the database, it cannot be queried unless snapshots are created. Why do Organizations Replicate Databases? One of the key benefits of database replication is improved data availability. With multiple copies of the database spread across different locations, or servers, organizations can ensure that the data is always accessible, even in the event of a server failure or network outage. This high availability of data is essential for real-time access to up-to-date information. Furthermore, database replication helps distribute the workload among multiple nodes, resulting in improved performance. By spreading the read and write operations across different replicas, organizations can handle a higher number of concurrent requests, reducing the chances of bottlenecks and improving response times. The distributed nature of replication also enables organizations to scale their databases horizontally by adding more replicas as the workload increases. Database replication also plays a vital role in disaster recovery strategies. By having replicated copies of the database in different geographical locations, organizations can recover from disasters such as natural calamities, hardware failures, or human errors. In the event of a disaster, the replicated database can be quickly activated, ensuring minimal downtime and data loss. How does database replication work? Database replication works by employing a set of techniques to copy and synchronize data across multiple databases or servers. Change Data Capture (CDC) is one such technique. It captures changes in the source database and updates the replica in real-time. In general, replication involves a primary database that serves as the source of truth and one or more replicas that maintain copies of the primary database. When a change occurs in the primary database, it is captured by the replication mechanism and propagated to the replicas. This ensures that the data in the replicas is consistent with the primary database. Types of Database Replication Full-table Replication Full-table replication replicates entire tables from a source database to one or more replica databases. In this approach, any changes made to the source table, including inserts, updates, and deletes, are replicated entirely to the corresponding table(s) in the replica database(s). Full-table replication is straightforward and ensures that the replica contains an exact copy of the source table at any given point in time. However, it may result in higher data transfer and storage requirements, especially when dealing with large tables or when only a small portion of the data has changed. This method is commonly contrasted with more granular approaches, such as CDC, where only the modified data is replicated, leading to more efficient use of resources. Key-Based Incremental Replication In this type of database replication strategy, changes to a database are identified and replicated based on specific key values within the data. Instead of replicating entire tables, key-based incremental replication selectively captures and replicates only the rows that have been inserted, updated, or deleted, based on certain key columns or fields. Key-based incremental replication is particularly useful for minimizing data transfer and improving efficiency in scenarios where only a subset of data changes frequently. Log-Based Replication Log-based replication relies on capturing and replicating changes directly from the transaction log (or database log) of the source database. Instead of monitoring and tracking individual data modifications (as in key-based or full-table replication), log-based replication extracts the changes recorded in the database’s transaction log, which is a sequential record of all database transactions. Synchronous Replication Synchronous replication ensures that all changes made to the database are immediately replicated to all replicas before the transaction is considered complete. While it guarantees data consistency, it may introduce latency as the transaction has to wait for the replication process to finish. Asynchronous Replication Asynchronous database replication is used to copy and synchronize data between databases in a way that doesn’t require the primary database to wait for the replica to acknowledge receipt of the data changes. In this scenario, the replication process doesn’t happen in real-time or synchronously with each transaction on the primary database. Instead, changes are transmitted and applied to the replica at a delay, often referred to as “replication lag”. Master-slave Replication In master-slave replication, also called Single Leader Replication, there is a single primary database, known as the master, which processes write operations. The replicas, known as slaves, receive the changes from the master and update their data accordingly. This technique is widely used in scenarios where read scalability and fault tolerance are important. Master-master Replication Master-master replication, also known as bi-directional replication, allows both databases to act as the primary database and accept write operations. Changes made in one database are replicated to the other, ensuring that both databases are in sync. This technique provides better fault tolerance and enables load balancing between databases. Snapshot Replication Snapshot replication is a type of database replication where a copy of the entire database is taken at a specific point in time and then transferred to the replicas. This initial snapshot is then followed by incremental updates to keep the replicas synchronized with the primary database. Snapshot replication is commonly used when the data changes infrequently or when the replicas are located in remote locations with limited bandwidth. Transactional Replication Transactional replication is a type of database replication that captures and propagates individual transactions from the primary database to the replicas. This means that every change made to the primary database, such as insertions, updates, or deletions, is replicated to the replicas in the same order they occurred. Transactional replication is often used in scenarios where data consistency and low latency are critical, such as in financial systems or real-time applications. Merge Replication Merge replication is a type of database replication that allows multiple replicas to independently modify the data and then merge the changes back to the primary database. This type of replication is commonly used in scenarios where the replicas are frequently disconnected from the network or when conflicts between changes made at different replicas need to be resolved. Merge replication requires a more complex conflict resolution mechanism to ensure data integrity. Peer-to-Peer Replication Peer-to-peer replication, also known as multi-master replication, is a type of database replication where each replica can act as both a source and a target for data changes. This means that changes made at any replica are propagated to all other replicas in the network. Peer-to-peer replication is often used in distributed systems where multiple replicas need to be updated simultaneously and where high availability and scalability are essential. Common Database Replication Challenges Implementing database replication comes with its own set of challenges. It’s important to factor in these challenges to ensure smooth and effective database replication: Data consistency: Ensuring data consistency across replicas can be challenging, especially in scenarios where data changes frequently. Synchronizing and propagating changes requires sophisticated algorithms and techniques to avoid conflicts and maintain consistency. Replication lag: As replication often involves propagating changes to remote replicas, there can be a slight delay between the primary database and the replicas. Minimizing replication lag may be a high priority, especially in scenarios where real-time data is critical. Using synchronous database replication can help keep replication delays to a minimum. Network latency: Replicating data across geographically distributed locations can be impacted by network latency. Ensuring efficient data transfer and minimizing latency becomes criticalto maintain a responsive and reliable replication system. Scalability: As the volume of data and the number of transactions increase, ensuring the scalability of the replication system becomes a challenge. Scaling to accommodate a growing workload, like during a holiday sale, while maintaining performance and responsiveness is a delicate balance. Conflict resolution: When concurrent updates happen on both the primary and replica databases, a mechanism is needed to determine which update prevails. Choosing the wrong strategy can lead to lost data or corrupted information. How to evaluate modern Database Replication Platforms? With numerous database replication platforms available in the market, choosing the right one requires due diligence. Here are some key factors to consider when evaluating modern database replication platforms: Performance and scalability: Assess the platform’s ability to handle the workload and scale as your data grows. Look for features such as load balancing, data sharding, and automatic failover. Latency and Replication lag: Assess the platform’s ability to minimize replication lag, especially if your use case requires real-time or near-real-time data synchronization. Look for features that enable monitoring and management of replication lag. Data consistency and integrity: Ensure that the database replication platform provides mechanisms to maintain data consistency and integrity across replicas, such as conflict resolution, transactional guarantees, and data validation. Monitoring and management: Consider the ease of monitoring and managing the replication process. A good database replication solution provides functionalities like real-time monitoring, performance metrics, and intuitive management interfaces to streamline the process. Integration and compatibility: Check if the platform supports integration with your existing database systems and applications. Evaluate compatibility with different database management systems and programming languages. No-code tools, like LIKE.TG, can integrate with a range of databases, ensuring a complete data management process. By evaluating these factors, organizations can make an informed decision and select a modern and efficient database replication tool tailored to their unique requirements. Conclusion Database replication plays a crucial role in ensuring data availability, performance, and fault tolerance for organizations of all sizes. Understanding the concepts and techniques associated with database replication is essential for building resilient and scalable data systems. Implementing a robust database replication solution and choosing the right replication platform, enables you to deliver high data availability, keeping it ready to meet your ever-growing business needs. Make Database Replication Easy and Fast Streamline and automate the entire database replication process – without using any code! Centerprise does it all for you. Give it a try – 14 days free!
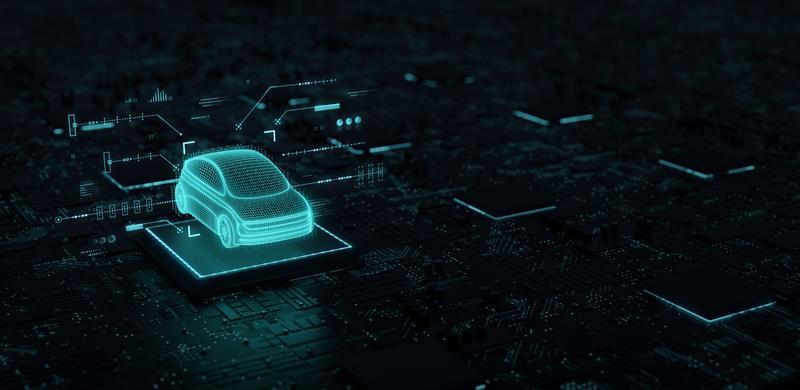
Top 6 Mulesoft Alternatives & Competitors in 2024
ETL platforms play a pivotal role in the seamless functioning of modern businesses, enabling the interconnection of various applications, data sources, and systems. MuleSoft has long been a prominent player in the integration platform arena, renowned for its powerful integration capabilities. However, as the technological landscape continues to diversify in 2024, businesses are exploring MuleSoft alternatives that cater to their unique needs and requirements. Mulesoft and Its Key Features MuleSoft provides a unified data integration platform for connecting applications, data, and devices on-premises and in the cloud. Built on Java, its Anypoint Platform acts as a comprehensive solution for API management, design, monitoring, and analytics. Some of Mulesoft’s key features include: API-Led Connectivity: Mulesoft facilitates the creation, management, and scaling of APIs, allowing organizations to improve connectivity with applications. Through its API-led approach, Mulesoft emphasizes modularity and reusability, simplifying the construction and upkeep of integrations. Anypoint Platform: Serving as a centralized hub, the Anypoint Platform allows for the design, construction, and management of APIs, integrations, and applications. Organizations can collaborate, streamline development processes, and gain insights into their integration landscape. Automated Data Mapping: Anypoint DataGraph by Mulesoft supports automatic data mapping, ensuring precise data synchronization. Error Handling and Monitoring: Mulesoft provides error handling and monitoring capabilities for quick issue identification and resolution. Real-time monitoring and alerting allow organizations to ensure the reliability and performance of their integrations. Limitations: Why Should You Consider a Mulesoft Alternative? Here are some potential reasons why businesses consider Mulesoft alternatives: Steep Learning Curve: MuleSoft has a steep learning curve, and its Anypoint Studio, which is used for designing Mule applications, may require training for developers who are new to the platform. Dependency on Skilled Developers: Requires an experienced MuleSoft developer with a Java background in the team. Limited Design Environment Support: Interaction with MuleSoft support directly from the design environment is currently unavailable. Customer support: MuleSoft offers different levels of customer support based on the subscription or licensing tiers, which means not all customers get 24/7 support and dedicated support engineers. Also, support hours and number of incidents supported are also dependent on the support tier purchased. Shortcomings in Complete Data Management: While MuleSoft excels in integration and connectivity, it falls short of being an end-to-end data management platform. Notably, MuleSoft lacks built-in capabilities for AI-powered data extraction and the direct construction of data warehouses. Mulesoft Pricing MuleSoft’s Anypoint Platform is an integration tool with a notably high cost, making it one of the more expensive options in the market. The pricing structure is linked to the volume of data being extracted, loaded, and transformed, resulting in monthly costs that are challenging to forecast. The Anypoint platform from MuleSoft features three pricing tiers—gold, platinum, and titanium—each offering distinct processing capabilities, functionality levels, and corresponding pricing. The cost associated with each tier is determined by the processing power required for data extraction and loading, increasing with growing API and integration requirements. Top 6 Mulesoft Alternatives 1. LIKE.TG LIKE.TG is an enterprise-grade unified end-to-end data management platform that enables organizations to build automated data pipelines easily in a no-code environment. With its extensive library of connectors, built-in data transformations, and data quality and security features, LIKE.TG stands out as one of the best alternatives to Mulesoft. Key Features: Unified platform for AI-powered data extraction, preparation, integration, warehousing, edi mapping and processing, and API lifecycle management. Supports a broad range of connectors for popular databases, data warehouses, and file formats, facilitating seamless integration into the ETL/ELT process. Build API-based connectors for any integration apart from various native connectors. Extensive library of pre-built records and set-level transformations. Provides robust data profiling and quality features. Provides workflow automation, CDC, real-time data preview, and parallel processing Pricing: Fair pricing structure with quote available on request. Avail a 14-day free trial to experience the solution firsthand. 2. Dell Boomi Dell Boomi is a cloud-based integration platform encompassing application and data integration, API management, and master data management, among other data management capabilities. Its visual interface and pre-built connectors allow for rapid integration. Key Features: Drag-and-drop user interface. Repository of pre-built integration processes, connectors, and reusable assets. Support for various integration patterns such as event-driven web services, batch, streaming, and ETL integration. Unified reporting console for streamlined monitoring. API-centric platform allowing the creation of low-code applications. Pricing Structure: Choose from 4 pricing plans – Professional, ProPlus, Enterprise, and Enterprise Plus – each tailored to cover specific features and different levels of customer support. 3. IBM App Connect IBM App Connect is a cloud-based Integration Platform as a Service (iPaaS) tool that allows seamless connections among various software applications such as SaaS, ERPs, CRMs, HRMs, and data stores. It has AI capabilities that simplify the process of mapping and transforming data. Additionally, IBM’s dashboard and integrated management tools allow users to oversee and control integrations, ensuring data integrity and security. Key Features: No-code user interface. AI-driven connectors, and customizable templates. Many deployment options, including on-premises, in-cloud, and hybrid environments. Support for establishing automated CI/CD pipelines for continuous integration and delivery. Pricing: Enterprise Integration Software pricing is available upon request. 4. SnapLogic SnapLogic offers a low-code/no-code cloud-based integration platform that caters to both application and data integration needs. The platform provides a range of pre-built connectors (known as Snaps) and comprehensive end-to-end integration templates tailored for various common business processes. Its feature set encompasses data automation and integration functions, allowing the efficient delivery of data to data lakes and cloud data warehouses through visual ETL and ELT processes. Key Features: Data streaming architecture. AI-powered integration assistant for constructing workflows and pipelines. Equipped with pre-built Snaps for connecting to a wide array of common systems such as ERPs, CRMs, databases, IoT devices, among others, with the additional capability of creating custom Snaps through an SDK. Pricing: The pricing details are available upon request. 5. Jitterbit Jitterbit’s Harmony is a low-code iPaaS solution designed to connect data, applications, and business processes, whether on-premises or in the cloud. Functioning as a cloud-native integration platform, it automates workflows and undertakes comprehensive API lifecycle management tasks while upholding enterprise-grade security and governance. Key Features: Ready-made recipes, process templates, and connectors featuring reusable integration components Compatibility with hybrid environments, including SaaS, legacy systems, and on-premise setups Developer-friendly platform for API creation, publication, execution, and analysis Pricing: Jitterbit Harmony offers Standard, Professional, and Enterprise pricing plans, tailored based on features, connectivity, API platform, and support and training. 6. Informatica Informatica Integration Cloud offers a comprehensive suite of integration solutions, including data integration, application integration, and API management. With its user-friendly interface and extensive connectivity options, Informatica poses a strong alternative to Mulesoft. Informatica supports connectivity to a broad range of data sources and targets, including databases (both relational and non-relational), applications, cloud platforms, and more. This enables organizations to integrate data from diverse sources and formats. Key Features: Data transformation, allowing users to cleanse, enrich, and transform data during the integration process. Workflow management system Metadata Management for data lineage, and impact analysis Data profiling, cleansing, and standardization capabilities. Pricing: Consumption-based pricing. Quote available upon request Related: Learn about the best Informatica Alternatives for ETL/ELT Why is LIKE.TG the Best Mulesoft Alternative? LIKE.TG stands out as a superior alternative to Mulesoft due to its distinct flexibility, ease of use, and cost-effectiveness advantages. LIKE.TG offers a more intuitive and user-friendly interface, allowing organizations to streamline their integration processes without the need for extensive technical expertise. Its agile and adaptable architecture enables seamless integration with various systems and applications, facilitating quicker implementation and reducing time-to-market. While Mulesoft employs a tiered customer support model, wherein different plans dictate the level of assistance users receive, LIKE.TG is known for its award-winning customer support. Mulesoft’s approach may limit accessibility for users on lower-tier plans, potentially impacting the quality and speed of support they receive. On the other hand, Asters offers exceptional customer support and assistance through a ticket system, responsive email support, live chat for real-time resolutions, prompt phone support, and comprehensive documentation. LIKE.TG also has its Data Academy for training and a collaborative community forum. This diverse range of support channels ensures users have efficient access to help across different needs and preferences. So what are you waiting for? Try LIKE.TG’s powerful data management solution today to extract, transform, secure, streamline, and synchronize data effortlessly. Sign up for a demo or a 14-day free trial now! LIKE.TG does it all – but better! Enjoy increased flexibility, award-winning customer support and a highly user-friendly interface – all in a reasonable price. Book FREE Demo Today!
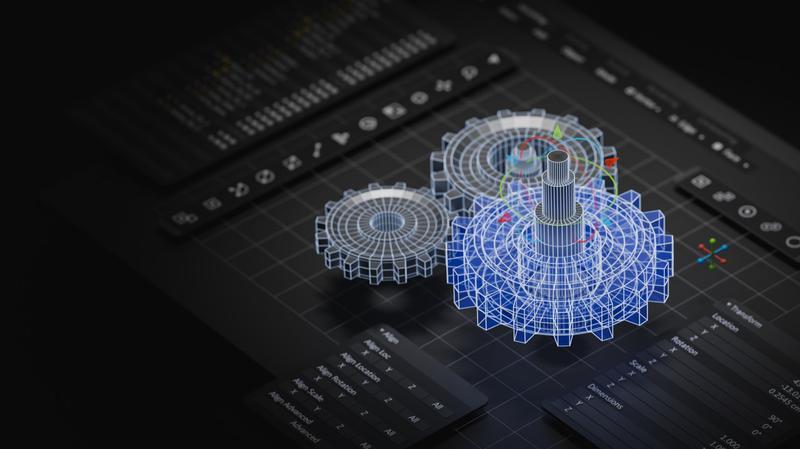
Delivering Personalized Experiences with a Single Customer View
Every interaction a customer has with a business, be it an online purchase, a visit to a physical store, a customer service call, or a social media engagement, is a valuable piece of a larger puzzle. Individually, these pieces provide a fragmented understanding of the customer’s story. But the true value lies in assembling these pieces together to reveal the complete picture. A Single Customer View (SCV) is a consolidated, holistic view of each customer which integrates data from various touchpoints. It’s the key to unlocking the full potential of customer data, driving effective customer engagement, and maintaining a competitive edge in an increasingly customer-centric market. What is a Single Customer View? A Single Customer View (SCV) is a comprehensive view of customer data across all interactions and touchpoints with a business or organization. It brings together information from various sources, channels, and departments within an organization to create a cohesive and accurate profile of an individual customer. The primary objective of a Single Customer View is to provide businesses with a complete understanding of their customers’ needs, preferences, and behaviors. This enables businesses to deliver personalized customer experiences, improve customer satisfaction, and enhance customer loyalty. Furthermore, an SCV aids in making informed business decisions, optimizing marketing strategies, and identifying opportunities for cross-selling and up-selling. Essentially, SCV is creating a unified and centralized snapshot of a customer’s interactions with a company. Types of Customer Data Collected in a Single Customer View Customer data comes from diverse sources, each providing unique insights into customer interactions and behaviors. The consolidation of these key data sources from various channels contributes to a comprehensive understanding of individual customers. The key types of customer data typically include: Transactional Data: Information about the customer’s purchases, transactions, and order history, such as product details, quantity, price, and date of purchase. This data helps businesses understand what products or services the customer has bought and their spending patterns. Interaction Data: Details about the customer’s interactions with the business across different channels. This may include website visits, social media interactions, emails, and other forms of engagement. Understanding how a customer interacts with the company helps tailor communication and marketing strategies. Demographic Information: Basic personal details such as the customer’s name, address, contact information, age, gender, and other relevant demographic data. This information provides a foundational understanding of who the customer is. Preferences and Behavior: Insights into the customer’s preferences, behaviors, and choices. This data may include product preferences, communication channels, and specific interests. Understanding customer preferences enables businesses to offer more personalized experiences. Customer Service History: Records of the customer’s interactions with customer support, including inquiries, issues raised, and resolutions provided. This data helps improve customer service and ensures a consistent and positive experience. Feedback and Reviews: Information on customer opinions, including feedback surveys, ratings, and reviews. This data may include comments, ratings on a scale, and testimonials. It is valuable for sentiment analysis, identifying areas for improvement, and shaping future interactions. Marketing Responses: Data on how customers respond to marketing efforts, including metrics like open rates for emails, click-through rates on advertisements, and conversion rates from promotional campaigns. Understanding how customers engage with marketing efforts helps refine future campaigns for better effectiveness. Social Media Data: Insights from the customer’s social media presence include data such as the number of followers, engagement metrics (likes, shares, comments), and information from linked social media profiles. This provides insights into the customer’s online presence and can be valuable for targeted marketing and engagement strategies. Benefits of Single Customer View Building a SCV transforms the way organizations operate, reducing costs, saving time, and elevating profitability. Let’s explore how SCV streamlines processes and enhances outcomes for the benefit of businesses and all stakeholders involved. Personalized and Targeted Marketing: A 360-degree customer view, enriched with insights into preferences, behaviors, and historical interactions, empowers marketers to create highly personalized campaigns. By segmenting customers based on specific criteria and updating profiles in real-time, businesses tailor content to meet unique needs. Customers are more likely to respond positively to messaging that resonates with their specific preferences, contributing to overall profitability, as affirmed by 90% of top marketers. Improved Audience Segmentation: By consolidating data from diverse sources into a centralized repository, SCV creates a comprehensive and up-to-date profile for each customer. This 360-degree customer view allows businesses to categorize their customer base into distinct segments based on various criteria such as demographics, behavior, and preferences. Marketers can leverage this segmentation to create more focused and relevant campaigns for specific customer segments. Precision in targeting ensures that messages reach the right audience, optimizing marketing spend and improving overall effectiveness of marketing efforts. Enhanced Cross-Sell and Upsell Opportunities: The ability to identify and capitalize on cross-sell and upsell opportunities is a valuable outcome of a unified view of a customer’s interactions, purchases, and preferences. By understanding the customer’s history, businesses can strategically recommend products that the customer typically purchases together, leading to increased average transaction values. Leveraging insights gained from customer history for cross-selling and upselling contributes to maximizing the lifetime value of each customer, as businesses can tailor their offerings based on a comprehensive understanding of individual customer needs. Proactive Issue Resolution: A comprehensive customer view equips customer support teams with a holistic perspective of a customer’s history and interactions across channels. This insight allows organizations to identify potential issues before they escalate and take preemptive measures to address concerns. Anticipating customer needs and resolving issues promptly enhances overall customer satisfaction. Quick and proactive issue resolution mitigates potential negative experiences and contributes to positive brand perception, fostering customer loyalty and retention. Customer Journey Optimization: Understanding the complete customer journey is essential for effective marketing. SCV provides insights into every touchpoint and interaction a customer has with the brand. Marketers can use this information to map out and optimize the entire customer journey, ensuring a seamless and engaging experience. This optimization contributes to increased customer satisfaction and loyalty as customers move smoothly through each phase of their interactions with the brand. How to Create a Unified Customer View The following steps outline the process of creating a unified customer view, providing a roadmap for businesses to effectively consolidate and leverage their customer data. Each step plays a critical role in ensuring the accuracy, completeness, and usability of the customer profile. Data Collection: The first step is data collection, which involves gathering customer data from various sources. These sources could include sales records, website interactions, customer service communications, and customer surveys. The goal is to capture a wide range of data that reflects all aspects of the customer’s interactions and experiences with your business. Data Integration: The next step involves consolidating data from different sources and channels into a single source of truth. The aim of data integration is to create a unified, coherent set of data that provides a comprehensive view of each customer. This process may involve cleansing data to remove errors or inconsistencies and transforming data to convert it into a common format that can be easily analyzed and interpreted. Identity Resolution: Identity resolution refers to linking various identifiers like email addresses or phone numbers to create a singular customer profile. This process ensures that data from different interactions is correctly attributed to the same customer, providing a comprehensive, rather than disjointed view of their behavior. The goal is to ensure that all data points related to a single customer are connected, providing a complete picture of that customer’s interactions with your business. Create a Centralized Customer Database: This step involves establishing a centralized repository or database for storing unified customer data. Various platforms can be leveraged for this purpose, including traditional relational databases, modern NoSQL databases, data warehouses, or cloud-based storage solutions. The choice of platform depends on factors such as data volume, real-time processing needs, security requirements, and budget. It is important to ensure that the database’s structure facilitates easy access and efficient data analysis. Share Data Across the Organization: The final step is to share the data across the organization in a secure and compliant manner. This involves making the central customer data hub accessible to all relevant departments within your business, such as sales, marketing, customer service, and product development. By having access to the unified customer view, teams can gain a better understanding of the customers. This helps them to tailor their strategies based on the insights gained from the data and work together to provide a consistent and personalized customer experience. Conclusion As enterprises navigate through ever-evolving customer needs and preferences, the importance of building a SCV becomes increasingly evident. It serves as a strategic tool, consolidating data from various touchpoints to provide a summary of each customer’s journey. This holistic understanding enables businesses to create experiences that resonate with customers, making them feel valued and understood. In this context, solutions like LIKE.TG, with its advanced data integration and management capabilities, are instrumental. It aids in creating an effective SCV, allowing businesses to seamlessly consolidate and analyze customer data. This empowers businesses to deliver personalized experiences, fostering customer loyalty and driving growth. Download our eBook now and take a step towards a customer-centric approach.
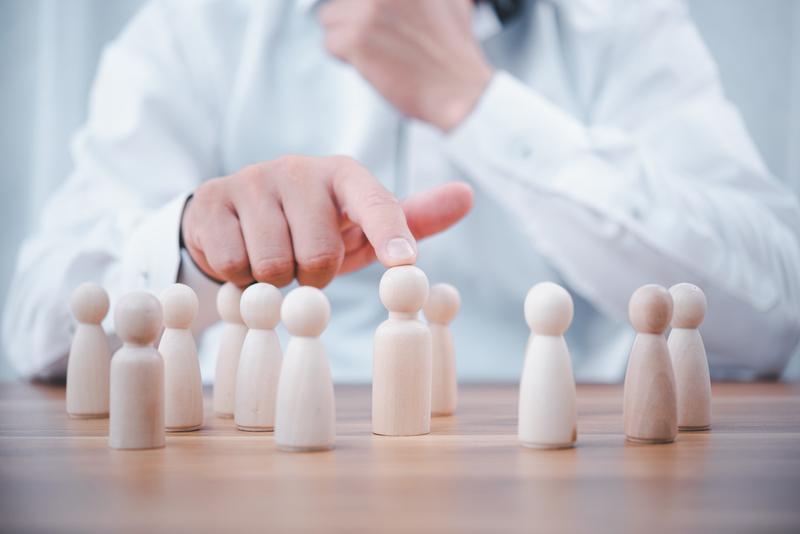
Your Introduction to Marketing Data Analytics
Did you know that leaders at high-performing companies are 57% more likely to adjust long-term strategies based on data and analytics than their peers at low and average performing companies? It’s not just about numbers; it’s about understanding your audience and making decisions that resonate with them. Your CRM, SaaS applications, ERP systems, and other digital assets contain a wealth of insights. Every click, view, and interaction tell a story about your audience and are full of valuable insights. That’s where marketing data analytics comes into play. What is Marketing Data Analytics, and Why is it Important? Simply put, “marketing data analytics” is the process of collecting, analyzing, and interpreting data related to your marketing efforts. This data includes everything from website traffic and social media engagement to email campaign performance. Why does it matter? Well, marketing analytics, fueled by data collected from diverse sources plays a crucial role in optimizing marketing strategies. By employing smart data analysis tools and models, you can extract actionable insights from this data. This approach allows you to: make data-driven decisions. refine targeting strategies. allocate resources efficiently. ultimately enhance the overall impact of marketing campaigns. Moreover, marketing analytics also makes it easy to understand your audience. No more shooting in the dark; you’ll know which channels deliver the best results, allowing you to allocate resources wisely and optimize your marketing budget. Related: Learn about data analytics as a whole. How to Use Data Analytics to Maximize Marketing ROI Here’s how you can leverage marketing data analytics to maximize your ROI: Understanding Customer Behavior Marketing data analytics provides a deep dive into customer behavior. You can track website interactions, analyze click-through rates, and understand the customer journey. This insight helps tailor messaging and offerings to align with customer preferences. For example, you can see what customers click on most—maybe a particular product category—on your website. Knowing this, you can tweak your messages and improve the display of related products to match what they like. This will increase engagement and satisfaction, optimizing conversions. Personalized Marketing Campaigns Gone are the days of generic mass marketing. With analytics, you can create personalized campaigns based on customer demographics, preferences, and past interactions. This approach enhances the customer experience and significantly boosts the chances of conversion. In retail, analytics can tap into customer demographics, past interactions, and preferences to craft personalized campaigns. You can track what categories customers have demonstrated interest in and showcase new arrivals in those categories online. This strategy enhances their experience and boosts the chances of conversion. Here’s one example: Amazon utilizes the Collaborative Filtering Engine (CFE), a specialized software for personalized recommendations, following behavioral analytics principles and contributing to 35% of annual sales on the Amazon Web Store. Predictive Analytics for Planning Predictive analytics uses historical data to forecast future trends, helping you stay ahead of the curve and plan your marketing strategies accordingly. This foresight allows you to allocate resources efficiently, plan inventory, and optimize marketing well in advance. Again, Amazon is on top of its analytics game. The company recently patented a cutting-edge predictive ordering technology, allowing them to order desired products on behalf of customers before actual purchases. This showcases the remarkable advancement of their predictive AI in anticipating consumer preferences. Optimizing Ad Spend Marketing data analytics gives you a clear picture of which channels are driving the most engagement and conversions. This information empowers you to optimize your ad spend by focusing on the most effective channels. For example, an ad performing well on Instagram may not deliver equivalent results on Facebook, impacted by audience demographics and content format within each platform. A robust marketing analytics pipeline consolidates these diverse data points, providing valuable insights for optimized targeting, content tailoring, and improved ad performance. Real-time Campaign Monitoring In digital marketing, real-time monitoring tracks campaign performance as it happens, enabling you to make quick adjustments to capitalize on what’s working and rectify what’s not. Real-time monitoring will tell that a particular ad variant is generating high engagement, enabling you to decide upon allocating more budget to that specific element for immediate impact. By leveraging these strategies, you’ll not just gather data but transform it into actionable insights that drive higher conversion rates. Important Marketing Data Analytics Metrics Metric Description Example Customer Acquisition Cost (CAC) Calculate the cost of acquiring a new customer. $800 spent on marketing, acquiring 40 customers, CAC = $20. Churn Rate Measure the rate at which customers stop doing business. Start with 2500 customers, lose 1250 in a month, Churn Rate = 50%. Cart Abandonment Rate Track the number of customers who abandon their online shopping carts. Out of 1200 initiated sales, 840 were abandoned, Abandonment Rate = 70%. Customer Lifetime Value (CLV) Measure the total value a customer is expected to bring over their relationship. The customer purchases $120 products 4 times a year for 2 years, CLV = $960. Brand Mentions Monitor the frequency of brand mentions on various platforms. Count mentions in social media, news articles, and product reviews. Impression Share Quantify the brand’s performance on a channel compared to its potential audience. For 4800 ad impressions out of 10000 potential, Impression Share = 48%. Customer Satisfaction (CSAT) Gauge average customer satisfaction through ratings. 240 out of 1200 customers rated 4 or 5, CSAT = 20%. Customer Effort Score (CES) Measure how much effort customers put forth in their interactions. 480 responses with a sum of 2160, CES = 4.5. Bounce Rate Calculate the ratio of visitors who leave a page without further engagement. 720 of 1800 visitors leave, Bounce Rate = 40%. Time Spent on Site Observe the duration of site visits to evaluate engagement. Users spend an average of 15 seconds, indicating a need for content evaluation. Return on Investment (ROI) Measure the efficiency of a marketing campaign by dividing profit by cost. $1200 spent, $600 profit, ROI = 50%. Email Open Rate Determine the proportion of recipients who opened an email. 3600 opened out of 6000, Email Open Rate = 60%. Click-Through Rate (CTR) Evaluate campaign performance across various channels. 24 clicks out of 1200 impressions, CTR = 2%. Cost per Lead Calculate the cost to attract potential customer attention. $600 spent to acquire 12 leads, Cost per Lead = $50. Repurchase Ratio Provide insight into the number of customers making repeated purchases. 120 repurchases out of 1200 customers, Repurchase Ratio = 10%. Return on Advertising Spend (ROAS) Calculate revenue generated for every dollar spent on PPC advertising. $1200 spent, $2400 revenue, ROAS = 2.0. Several additional metrics can help you determine the performance of your website, such as: Web Traffic: Track volume and source of visits to assess targeted marketing success. Mobile Traffic: Monitor the number of users accessing the site through mobile devices. Screen Flow: Chart a user’s journey around the website to optimize the user experience. Unique Visitors: Measure individuals who accessed the website within a specified time. Referral Traffic: Indicate the source of website traffic to evaluate marketing strategy effectiveness. These extra metrics help you understand how users interact with your website. As a result, you can improve the site, enhance user experience, and assess the effectiveness of your marketing strategies. Final Word Marketing data analytics isn’t a luxury; it’s a necessity to get good results. Leveraging timely insights allows you to transform your marketing strategies from guesswork to precision. But how do you gather all of this customer data? You have to first collect the necessary customer data before using it for analysis. You can either do it manually through coding, or you can use dedicated no-code data integration tools. These integration tools connect to your CRM, ERP, and OLTP system to extract customer data and consolidate it into a central repository. These tools also allow you to automate the entire data collection process. Learn more about integrating data and how LIKE.TG’s Data Pipeline Builder helps companies combine their data seamlessly! Try our ETL tool for free with a 14 day trial!
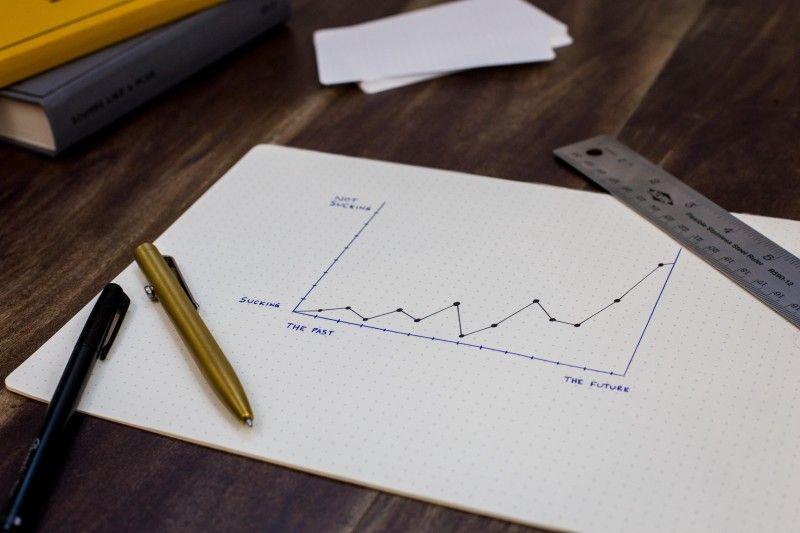
The Change Data Capture (CDC) Guide for PostgreSQL
This article serves as a comprehensive guide to change data capture (CDC) in PostgreSQL, also known as Postgres. It will take you through the different ways of implementing Postgres CDC, including the pros and cons, as well as an automated alternative to all the manual methods. It will also touch upon the importance of PostgreSQL CDC. Before we start, let’s get some basics out of the way. What is PostgreSQL? PostgreSQL is an open-source relational database management system (RDBMS). Its versatility allows for its usage both as a database and as a data warehouse when needed. PostgreSQL is also completely free, and its users consistently enjoy extensive open-source development and reliable support. These are some of the major reasons for its impressive longevity—PostgreSQL has been around for over two decades and continues to rank among the most widely used relational databases for data management today. Features and Applications of PostgreSQL Besides being free, PostgreSQL has also earned a great reputation for its adaptability and extensibility. It integrates seamlessly with your existing systems and adheres to SQL standards, so you know what to expect. With built-in support for change data capture, Postgres provides a robust mechanism for tracking and capturing changes to the database. It is ACID-compliant, highly secure, and capably handles processing faults, so you can count on data validity. It supports both JSON and SQL queries. As a relational database, PostgreSQL stores data elements in the form of tables where the rows are also called tuples, and each tuple is identified with a unique key. The columns store the attributes for each corresponding data element. These features make PostgreSQL the right choice for many applications, a few of which include: Transactional Database: When you frequently need quick access to information to use in a product or an application, PostgreSQL is the right choice of database since its relational structure fetches relevant data at high speeds. Data Warehousing: A database works well for transactional data operations but not for analysis, and the opposite is true for a data warehouse. The two complement each other so you can leverage your data more easily. PostgreSQL’s compatibility with Business Intelligence tools makes it a practical option for fulfilling your data mining, analytics, and BI requirements. Location-Based Services: Using the PostGIS extension, you can use PostgreSQL to store, index, and query geospatial data as needed. This makes it a smart choice for location-based services and Geographic Information Systems (GIS). OLTP Transactions: PostgreSQL is commonly used for Online Transaction Processing (OLTP) transactions in many industries, including e-commerce (online purchases and stock updates), banking (funds transfers, ATM withdrawals, and balance checks), sales (retail transactions, invoice generation, and loyalty points), and services (booking appointments, service updates, and payments for services rendered). Why Do You Need Postgres CDC? Let’s say you require the most up-to-date data for reporting purposes right now, except you cannot have it yet since the next sync is scheduled for hours from now. Manual sync is one option, but if yours is a massive enterprise dealing with vast volumes of data, batch processing can quickly become a hurdle. It can lead to mistakes, the use of outdated information, and incorrect reporting. Ultimately, your decision-making will be affected as you won’t have the updated data you need to take the steps you should. This is exactly the kind of scenario that you can avoid with Postgres CDC. Postgres CDC methods help you track and handle changes in your databases. The most common action in such cases is the replication of changes in the source to a destination data store. This lets you keep your data synchronized between multiple databases. How Does PostgreSQL CDC Work and What Does It Do? Postgres CDC ensures that all systems have consistent access to the most updated version of your data, so you are always working with up-to-date information. Postgres change data capture also has some additional benefits, such as: Postgres CDC can help you lower your network usage costs since only the latest changes will be processed during each sync instead of the entire dataset. Analytics and similar tasks require more resources to execute, so frequent batch processing impacts the Postgres database’s performance over time and disrupts its functionality. Postgres CDC initially makes copies of the database and then incrementally updates them with changed data. This process is much lighter than batch processing, keeping your database faster and more efficient. Your Master Data Management (MDM) System will operate more smoothly with Postgres CDC in effect. With changed data from disparate sources continually updated in the MDM system, all your teams will use the same updated data. This can improve collaboration and coordination and speed up better business decisions. You can even use change data capture with Postgres as a disaster recovery mechanism for your data. Real-time CDC helps you back up critical databases and create redundancies that can be useful in instances of system failure, malware attacks, human errors, and other similar situations. Methods to Implement PostgreSQL Change Data Capture As discussed above, Postgres CDC will track and replicate any data changes across multiple databases. Your CDC method of choice can be either batch or real-time since CDC does not have any time-related requirements. You can implement Postgres CDC in a few distinct ways based on your operational requirements, and we will take a closer look at them below: Triggers Trigger-based Postgres CDC is also known as “event sourcing.” In this method, a dedicated event log is created to serve as the primary source of information. As its name suggests, this method relies heavily on triggers, which are crucial in each database transaction and capture events in real-time. A trigger programs the database to behave in a particular way whenever a specified event occurs. This event could be the introduction of new data, updates to existing data, or the removal of existing data from the database. Postgres CDC triggers are highly customizable. You can configure them to run before or after the events mentioned above, to run for every individual change, or to run once for a group of changes. You can even impose operating conditions on triggers—having them run only when a specific tuple is modified or run only as a response to certain actions. Triggers in Postgres CDC work well for tracking changes in tables, recording them in a different table, and creating a log of every change. To implement trigger-based Postgres change data capture, you can make audit triggers on your Postgres database that will track all events related to actions like INSERT, UPDATE, and DELETE. Since this method operates at the SQL level, you can refer to the Change Data Capture table and identify all changes. Here is an example of a trigger function: This code will create a table named ‘users_cdc’ for storing change data capture information, capturing information such as the user ID, operation type (INSERT, UPDATE, DELETE), timestamp of the change, and the user’s pre- and post-change information. This code defines a PL/pgSQL function (‘capture_changes’) triggered after INSERT, UPDATE, OR DELETE operations on the ‘users’ table. The ‘CASE’ statement determines the operation type based on the value of ‘TG_OP’ (trigger operation). This code creates a trigger named ‘users_trigger’ on the ‘users’ table that will fire following any INSERT, UPDATE, or DELETE operations. In the above Postgres CDC example, any time a change occurs in the ‘users’ table, the corresponding trigger will activate the ‘capture_changes’ function, which will log the changes to the ‘users_CDC’ table. The CDC table will capture the operation type, timestamp, and relevant data before and after the change. Together, these elements will help you track all modifications in the original table over time. Pros of Trigger-Based Postgres CDC Trigger-based Postgres CDC is reliable and comprehensive. All change captures and record-keeping occur within the SQL system. Instantaneous change capture enables the real-time processing of events. You can create triggers for diverse types of events. Cons of Trigger-Based Postgres CDC: Since any triggers you create run on your primary Postgres database, they can slow the database down. Like any other operation, executing Postgres CDC via triggers also requires resources and increases the pressure on the database. Minimizing the impact on the database resources involves creating another table mirroring the primary table and using this secondary table for trigger implementation. However, you will also need to make a separate pipeline to mirror any changes in any destination that lies outside of the trigger’s applicable Postgres instance. Queries Queries-based Postgres CDC requires more manual effort than using triggers. You must actively query your Postgres database to identify any changes instead of relying on pre-configured triggers. You need a timestamp column in your table to use this custom method. Whenever a record is added or modified, the timestamp column will be updated to include the date and time of the change. Any query you make to your Postgres database will use this timestamp column to obtain all modified records since your last query and then display those captured changes. You can also use scripts to monitor your Postgres database for changes and record them in a destination database, but this option is even more labor-intensive than simply querying the database. Continuing the Postgres change data capture example above, here is how you will query a ‘users’ table: This query fetches all records from the ‘users‘ table where the ‘last_updated‘ timestamp is greater than ‘2024-01-01’. It is used to retrieve user records that have been updated since the specified date. This code will create the table ‘users_changes‘ with information on each change—such as the type of operation (INSERT, UPDATE, or DELETE), its timestamp, and relevant data before and after the change. Pros of Queries-Based Postgres CDC It’s easier than setting up Postgres change data capture via triggers. It gives you more control over the CDC process. You don’t need any external tools for query-based CDC. Cons of Queries-Based Postgres CDC Requires a more proactive approach than the set-it-and-forget-it trigger-based Postgres CDC. You will need to regularly query the database to ensure accurate and on-time change tracking. The query layer is crucial for data extraction in this method, which can put an additional load on the Postgres database. PostgreSQL Logical Replication Postgres CDC with logical replication is also called Logical Decoding. Think of it as a streaming representation of a Write-Ahead Log (WAL). Since WAL captures and records all data changes in the Postgres database, these changes are considered logical decoding streams and are categorized as a logical replication slot at the database level. In other words, a replication slot is nothing more than a stream of changes occurring in a database. Each database can have multiple slots or streams of changes. Implementing PostgreSQL logical replication requires a logical decoding plugin. Postgres versions 10 and later feature the default ‘pgoutput’ plugin. It allows Postgres database changes to be processed as streams. However, if you are using a version older than 10, you will need to manually install a plugin such as ‘decoderbufs‘ or ‘wal2json‘. The ‘pgoutput‘ plugin is useful for replicating data between two or more PostgreSQL instances. Still, it can be difficult to transfer Postgres change stream data into another platform or database. If you want to move change stream data to a non-Postgres platform, you can use the ‘wal2json‘ plugin to transform the change stream data into JSON. This will allow your destination platforms to read it in JSON format—which is easier than reading pgoutput’s binary output. Besides a plugin, the other vital component in CDC via PostgreSQL logical replication is a subscription model with publishers and subscribers. This subscription model allows one or more subscribers to subscribe to one (or more than one) publications using the publisher node. Subscribers pull data from the publications, and they can republish it for replication or further reconfigurations. Follow the steps below to implement Postgres CDC with logical replication from a source database (we will use the ‘users’ table from the previous examples) to a destination database, which we will call the ‘users_changes’ table. Remember to replace placeholders such as ‘source_db’ and ‘replication_user’ with your actual database information. First, enable logical representation in the Postgres configuration file ‘postgresql.conf‘. Use the above settings and restart Postgres once these changes are made. This section will create a table named ‘users’ and a publication named ‘my_pub‘ for the ‘users‘ table. This publication is the source of the changes to be replicated. This section will make a table named ‘users_changes‘ in the destination database to store the changes from the source. This code will establish the subscription ‘my_sub‘, which will connect to the source database and subscribe to the ‘my_sub‘ publication. This code defines a trigger function ‘capture_changes‘ to capture changes in the ‘users’ table. It inserts relevant information into the ‘users_changes‘ table depending on the operation type (INSERT, UPDATE, DELETE). It also creates the trigger ‘users_trigger‘ to execute this function after each row-level change in the ‘users’ table. This is an SQL statement for monitoring changes in the logical replication slot named ‘my_sub‘ and fetching them. Replace ‘my_sub‘ with your specific subscription name. Pros of Postgres CDC with Logical Replication: Log-based CDC allows real-time data change capture using an event-driven mechanism. This enables downstream applications to access updated data from a Postgres database consistently. This CDC method can identify all kinds of change events in a Postgres database. Since this method accesses the file system directly, it puts less strain on the database. Cons of Postgres CDC with Logical Replication: Logical replication is not available for PostgreSQL versions older than 9.4. Depending on the use case, the complex logic required to process these events and their eventual conversion into statements for the target database can potentially affect project completion. Postgres CDC Using the Write-Ahead Log (WAL) Both triggers-based and queries-based Postgres CDC can create latency and affect your database’s performance over time. If,you’drather leverage Postgres’ built-in features and repurpose them for CDC processes instead of using the techniques discussed above, you can use the WAL. The WAL is a transaction log that notes all changes in the database. Its primary purpose is recovery and ensuring data integrity, making it useful for event-based CDC. Since this is a built-in feature, you will mainly be working with the Postgres database’s own settings to set it up for CDC. Below are the steps you need to take to implement Postgres change data capture using transaction log: First, enable WAL in your Postgres configuration. While this is typically the default setting, check the ‘postgresql.conf’ file to confirm. Postgres allows users to examine the WAL’s contents. As an example, we will use the ‘pg_waldump’ tool. Replace the placeholder ‘<path_to_wal_file>’ with the actual path of your WAL file when you use this code. Next, query the WAL contents using SQL queries. The ‘pglogical’ extension package includes the ‘pg_decode’ extension, which is the most frequently used extension for this purpose. ‘CREATE EXTENSION’ will create and install the ‘pglogical’ extension which provides logical replication capabilities for Postgres. The ‘SELECT’ SQL statementcreates a logical replication slot named ‘my_slot‘ using the ‘pg_create_logical_representation_slot‘ function. ‘pgoutput‘ specifies the output plugin to use for decoding changes and here it’s a built-in output plugin for logical replication. ‘pg_logical_slot_peek_changes’ is used to examine the changes captured in a logical replication slot ‘my_slot‘ is the logical replication slot being queried. This name is a placeholder and you should replace it with the name of the actual slot you want to query ‘NULL, NULL‘ is where you can place parameters specifying the range of changes to retrieve. Using ‘NULL, NULL‘ here means retrieving all available changes without any specific range. Note that you may need to do some coding, particularly if you are planning to automate change extraction and handling. Pros of Using WAL for Postgres CDC While some coding is still involved in using the WAL, overall it requires less coding than the other Postgres CDC methods we have discussed. Third-party solutions and platforms such as ‘pglogical’ are available to simplify the more complex steps in the process. Cons of Using WAL for Postgres CDC The data you extract from the WAL may be in a raw format. Transforming it to align with your application’s data structure requires additional work. Monitoring changes in the WAL could require further scripting or automation. Comprehension and interpretation of the WAL records require an in-depth understanding of your Postgres database’s internal workings. Automating Postgres CDC with LIKE.TG The following example explains how you can automate trigger–based Postgres CDC using LIKE.TG. Let’s assume you’re working with a PostgreSQL database and have configured a Database Table Source to read information from this database. First, you’ll enable CDC on this database by selecting Enable Change Data Capture on table. Then, select which fields you want to enable the CDC on, through the Select Columnsdialog box. While you can select one or all the fields in a database, it’s mandatory to choose a primary key. In this case, you can choose EmployeeID. Once you’ve chosen the fields, click ‘OK’. You’ll see the dialog box indicating that you have successfully enabled CDC on this database. Next, set up the destination table for storing the updated data from the source table. Add a database destination object from the Toolbox to your left. Configure the destination object by opening its properties. In the Define Input Ports for Mappingsection, select the Upsert checkbox with a CDC source as the incoming data will likely contain both new and updated records. In Select Fields for Matching Database Record, choose EmployeeID since it’s the primary key and unique for each record in the source database. Next, use drag-and-drop to map all the fields from the database source object to the destination object. The dataflow to implement Postgres CDC is now complete. When you run the dataflow and check the job progress window, you’ll find that LIKE.TG has read and written the entries from the source table to the destination table. Incremental Postgres CDC It’s easy to set up Incremental CDC in a PostgreSQL database using LIKE.TG, enabling you to load the data from your database table incrementally instead of complete loads with every run. Let’s assume that we’re working with shipping companies’ data in this use case and want to store this data in a new database table. We want tobe able to update the new table any time there’s a change in the source, without having to load the source table completely. We’ll use a pre-configured database table source with the pertinent information. Access the properties of the source object by right-clicking its header and selecting Properties. Connect with the database and click ‘Next’ to proceed. On the next screen you’ll see the Incremental Read Optionssection. Choose Incremental Load Based on Audit Fieldsas the Read Strategywhich will display further options. Audit Fields are updated when a record is created or modified, such as created date and time, modified date and time, and auto number. Incremental read tracks the highest value for any audit fields that you specify. During the next run, only records that have a higher value than the saved value are retrieved. Add a file path for the Incremental Transfer Information File, which LIKE.TG creates to store information on the database table’s last entry. It will compare this file with the database table on each run to check for new entries. Set up a destination table by dragging and dropping Database Table Destination from the Toolbox. Once configured, map the table source to the table destination object. You’ll see that the destination table is empty. You can check its contents as shown below, and this will open an SQL query for viewing the table data. When you execute the dataflow, check the Job Progress window and you will see that the entries from the source table have been written to the destination table. You can check this by previewing the destination table. Automate Postgres CDC in LIKE.TG and Keep Your Databases Synchronized Effortlessly Combine Postgres CDC techniques with LIKE.TG's impressive data management features and make the most of your always up-to-date databases. Discover the LIKE.TG difference today! Start Your FREE Trial Choosing The Right PostgreSQL CDC Method for Your Use Case There are multiple methods for implementing CDC in a PostgreSQL database, and you need to consider several factors when deciding which method to choose. Each method has its pros and cons, which we have briefly outlined above. Additionally, here are some more points to think about: Data Volume and Change Frequency: In environments with moderate data changes requiring real-time tracking, trigger-based CDC is your best bet Logical replication is suitable for scenarios with high data change rates as it provides real-time replication capabilities. If there is infrequent extraction of data changes in your workflows, choose queries-based Postgres CDC. Performance and Overhead: Triggers-based Postgres CDC can add additional overhead, especially if high transaction rates are involved. Logical replication is low-impact and easy on the source system, making it the right choice for high-performance scenarios. Queries-based CDC typically does not consume too many resources, but it can affect performance when there is intensive querying. Use Case Complexity: Triggers-based CDC is useful for complex cases that require customization and detailed change tracking. Logical replication is suitable for cases requiring simplicity and real-time replication. Queries-based CDC is a hassle-free option for simple use cases that don’t need complex triggers. Integration and Compatibility: Triggers-based CDC can integrate seamlessly with your current applications and databases Logical replication is ideal for scenarios where there is a need for compatibility between different Postgres instances. Queries-based CDC involves custom queries. As such, it’s the right option for meeting tailored integration needs. Simplicity and Functionality: Triggers-based CDC is a robust solution offering detailed change tracking, but this adds to its complexity. Good for customization-heavy environments. Logical replication strikes the right balance here, making it a practical choice for a variety of scenarios and ideal for catering to real-time replication requirements. Queries-based CDC is quite simple and flexible, but this means it can potentially need more manual intervention. It’s the right technique for occasional change extraction. Conclusion In this blog, we took an in-depth look at various options you can use for implementing CDC in PostgreSQL. We also discussed each method’s advantages and disadvantages and highlighted the factors you should consider before choosing a CDC method for your enterprise. While there is no one-size-fits-all solution when it comes to change data capture, automating the process should be in your list of top priorities. Ultimately, how you implement Postgres CDC depends on your performance requirements, customization preferences, and individual use case. At LIKE.TG, we believe in providing a simplified end-to-end data management solution. Our intuitive, drag-and-drop interface with built–in connectors and transformations does away with coding and democratizes data operations, making them equally accessible and insightful for non-technical and technical stakeholders alike. Our suite enables you to simplify your data integration processes, build robust data warehouses, and streamline your EDI and API management, all without writing a single line of code. Experience the LIKE.TG difference. Start your free trial today or request a quote to get started.
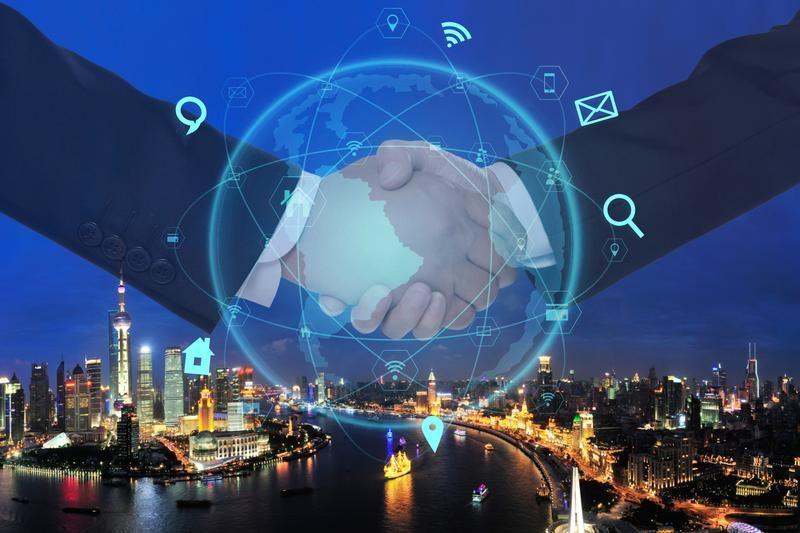
Top 9 Reverse ETL Tools and How to Choose The Right Tool for Your Organization
Modern data management relies heavily on ETL (extract, transform, load) procedures to help collect, process, and deliver data into an organization’s data warehouse. However, ETL is not the only technology that helps an enterprise leverage its data. Reverse ETL does the opposite of the standard ETL process, moving the processed data from storage back into various business applications and tools. In doing so, Reverse ETL (or rETL) processes enable non-technical stakeholders to utilize formatted data for multiple purposes—including forecasting, operational decision-making, customer behavior analysis, and more. All this is achieved with the help of Reverse ETL tools, and we’ll be discussing them in detail. What is a Reverse ETL Tool? Simply put, Reverse ETL lets you turn informative facts and figures into actionable steps, and various Reverse ETL tools are available to help you do so. All Reverse ETL tools serve the same primary purpose of transferring data from your storage solutions into downstream systems, so you will need to look carefully at other factors when choosing one for your enterprise. Let’s take an in-depth look at Reverse ETL tools and highlight some of 2024’s best ones. Types of Reverse ETL Tools Note that data transfer—the core function of reverse ETL tools—can be achieved using different methods. Here are some ways you can do so: Full-Fledged Reverse ETL Tools Reverse ETL tools are purpose-built for transferring processed data from your data warehouse, lake, or other storage system to various business tools. They work with your existing data storage mechanism to push formatted data where it’s needed. You can consider this the purest form of reverse ETL since there are no workarounds or hacks involved. Customer Data Platforms (CDPs) A Customer Data Platform (CDP) ingests data from various sources and consolidates it into a persistent, unified database. Different business teams can access this centralized database easily and benefit from comprehensive customer profiles. CDPs are different from true Reverse ETL tools, yet they can function similarly when needed and send data to downstream locations based on activation requirements. integration Platform as a Service (iPaaS) An iPaaS tool serves as a data integration platform and is designed for easier point-to-point integrations. Using them with APIs, you can create workflows and trigger events to share data between systems. Just like CDPs, an iPaaS can offer the utility of a Reverse ETL tool without being one itself. It can serve as a Reverse ETL tool while having a different primary function. The Best Reverse ETL Tools in 2024 These are the best Reverse ETL tools on the market in 2024: 1. LIKE.TG LIKE.TG caters effectively to your Reverse ETL requirements using a no-code interface. It has a user-friendly design that you can quickly get comfortable with, regardless of your technical proficiency. Key Features of LIKE.TG It offers customized data quality rules so you can get to your required data faster and remove irrelevant entries more easily. It makes it easy to maintain a log of all warnings and errors to identify potential issues. It supports all data formats and features an extensive library of connectors. Its cluster-based architecture distributes jobs across multiple nodes for faster completion. Considering cloud-first data management? LIKE.TG connects easily with cloud sources and destinations, such as Google BigQuery, Google Drive, Dropbox, Microsoft Azure, Amazon S3, Amazon Redshift, etc. Simplify your ETL and Reverse ETL processes! LIKE.TG Centerprise makes ETL and reverse ETL processes super easy using its drag-and-drop interface, cutting significant amounts of time and money investments – all while using zero code! Try now – 14 days free! 2. Matillion Matillion is a cloud-native, GUI-based ETL pipeline tool. While it’s mainly used for data integration purposes and delivers ETL solutions, it also features limited Reverse ETL capability. Key Features of Matillion The tool supports multiple data sources. It offers batch data loading and universal data connectors. Gives users a simple, code-free environment. Matillion’s Change Data Capture (CDC) processes enable rapid synchronization of data updates. Allows the automation of data pipeline creation and accelerates data delivery 3. Stitch Stitch was originally a self-service cloud data integration solution and was acquired by Talend in 2018. While it operates primarily as a cloud-based ETL platform, Stitch also has Reverse ETL functionality. Key Features of Stitch Supports numerous data warehouses such as Snowflake, Amazon RedShift, Google BigQuery, and Microsoft Azure Synapse Analytics, and data sources such as Shopify, MongoDB, and Salesforce. Offers ready-to-query schema and a simple interface. Provides enterprise-grade security integration for HIPAA and SOC 2 compliance. The tool offers open-source extensibility. It has orchestration features for scheduling, logging and monitoring, and error handling. 4. Airbyte Airbyte is an open-source data integration platform focusing on ELT. The platform focuses heavily on community-building and open-source development. Key Features of Airbyte Allows users to build connectors in different languages, in addition to the connectors built by its team. It offers support through GitHub, Slack, and forums. Features a low-code design and a user-friendly interface Implements standardized data transfer protocols. Airbyte offers built-in scheduling, orchestration, and monitoring. 5. Dataddo Dataddo has not spent as long on the Reverse ETL scene as the other tools on this list, and is a no-code, fully managed data integration platform with Reverse ETL capabilities. Key Features of Dataddo Dataddo offers support for Reverse ETL integration involving data lakes, data warehouses, SQL databases, and a variety of other sources. It provides multiple security measures for data protection. Features built-in data quality tools, such as the Data Quality Firewall, and error detection. Offers bi-directional data flows and compatibility between sources and destinations. Besides Reverse ETL, the tool also provides database replication and allows online services to be directly integrated with dashboarding applications. 6. Hevo Activate Hevo Activate is one of Hevo Data’s two primary data integration productsand is a dedicated Reverse ETL tool. Key Features of Hevo Activate Features Automated Schema Management to ensure synchronicity between the destination schema and source datasets. Offers pre-load and post-load transformations. The tool enables quick integration into existing workflows using the Hevo API It provides ease of use and scalability. 7. Census Census is a fully-managed, no-code Reverse ETL tool that has been in the industry since 2018. Key Features of Census Census offers high-speed connectors and supports large-volume data transfer. Features incremental diffing for API rate management. Offers a simple UI, segment builder, and visual field mapper. The tool provides automated data quality measures, connector extensibility, detailed observability and logging, and programmatic sync management. 8. Hightouch Hightouch is a scalable Reverse ETL tool that comes with various features for efficient data synchronization. Key Features of Hightouch Offers effective management of Reverse ETL processes through granular permissions, SSO, and access control. Features a simple 3-step process for data activation with a secure, SOC 2 Type 2 compliant platform. Hightouch has a live debugging feature for easy identification of bad or irrelevant data. The tool offers observability, configurable alerts, and integration with tools such as Datadog, Datafold, and Monte Carlo. 9. Grouparoo Grouparoo is an open-source, code-optional, partially managed Reverse ETL platform aimed primarily at developers. Key Features of Grouparoo Grouparoo offers a permission system to meet security considerations. Features external tool compatibility for data quality procedures. Although mainly designed for developers, Grouparoo provides some functionality for non-technical users through its web UI. Allows users to schedule automated syncs. How to Choose The Right Reverse ETL Tool in 2024 There are some critical factors to consider when picking a Reverse ETL tool for your organization. Here is what you should keep in mind: 1. Connector Selection A Reverse ETL tool’s functionality depends on the quality and number of its connectors. The more connectors a tool has, the more sources and destinations it will be able to support. This is why the first thing you should do when looking for a Reverse ETL tool is to examine its connectors. Consider the following: Connector breadth: these are the features that are available for all connectors Connector depth: features that are specific to the connectors you require Prioritization: Connectors should work seamlessly with the business programs and tools that your organization uses today. Extensibility: If you require custom integrations for a particular workflow, connector extensibility can make the process easier, whether it’s through a custom API or Webhook connector. Having a higher number of connectors means the Reverse ETL tool you choose is likelier to support your current data-related processes, while being scalable for future changes. 2. Rule-Based Pipeline Support Creating conditions for your Reverse ETL pipelines gives you control over which datasets are processed and updated by your Reverse ETL tool. Instead of doing full syncs each time, which can be time-consuming and require more resources, rule-based pipelines can perform incremental syncs and only focus on the data specified in the sync rules. This specificity can streamline your updates and make them faster, turning data into insights and making it available for you to use in less time. 3. Hybrid Data Management Your preferred Reverse ETL tool should support hybrid integration. The ability to work with both on-premises and cloud-based applications leads to greater flexibility and scalability, which will prove useful as your organization evolves over time. 4. Event-Based Integrations Event-driven architecture helps you ensure that your pipelines are updated in real-time. This is an essential feature because despite the effectiveness of scheduled updates, real-time integrations are more reliable for eliminating any gaps in your data, maintaining consistent data quality, and always having the most up-to-date data available. 5. Sync Reliability What makes sync reliability so important is the peace of mind it offers. If your Reverse ETL tool prioritizes sync reliability and robustness, you can rest easy knowingthat your data will be synchronized regardless of any technical difficulties or other issues that may arise. Your Reverse ETL tool should automatically reattempt syncs in case of interruption or failure, seamlessly process any changes in your data models, and notify you if there are any problems. Additionally, data validation capabilities, automated and trigger-based sync scheduling, and high sync speeds are also vital considerations. 6. Security Data privacy is a significant consideration, not just for compliance purposes but also because you have a duty to your customers to keep their information safe. Your Reverse ETL tool needs to complement your organization’s data security measures, not pose a challenge for regulatory and security compliance. The Reverse ETL tool you choose should meet up-to-date safety standards, such as the Health Insurance Portability and Accountability Act (HIPAA) and the Payment Card Industry Data Security Standard (PCI-DSS). Also look for data protection measures such as encryption for optimum security. 7. Cost of The Tool Evaluate the costs involved with using the tool. You will want to look at pricing models (not every tool comes with a conventional monthly plan) and determine whether the Reverse ETL tool you are interested in has a pricing model that works for you. Remember that the tool’s pricing not only needs to be feasible for right now, but it should also continue to be so as you scale your business. 8. Customer Support Buying a tool is not the end-all-and-be-all of your Reverse ETL process. From setup and configuration to demonstration, tutorials, and eventual troubleshooting, you’ll be working closely with your vendor’s support teams for as long as you continue using the tool. As such, prompt and reliable customer support can go a long way in determining your overall experience with a product. You need to be able to count on your vendor for quick responses, short turnaround times, and consistent availability so any issues that come up can be dealt with as soon as possible. 9. The Standalone vs. Unified Question One significant consideration for organizations is whether to invest in a standalone Reverse ETL solution or a unified data integration platform that comes with Reverse ETL capabilities. To make the right choice, first consider the volume of data that your organization is dealing with. If your workflows routinely involve large data volumes, then a unified data integration platform with built-in Reverse ETL functionality is a better option. The alternative to using a data integration platform is scripting or point-to-point data integration. While quick, these aren’t reliable in instances where there is a lot of data or a need for automation. Having a unified data integration platform—complete with Reverse ETL capabilities—lets you get the most use out of your data without worrying about data quality, the coding effort required by your developers for scripting, or other concerns. Not only will you be able to obtain useful insights that much faster, but the other functions of the platform will help streamline all your data processes, too. On the other hand, if your organization has smaller operations and its datasets aren’t extensive, then scripting can meet your basic integration requirements, and—once your data is integrated and formatted—a standalone Reverse ETL tool can move it from your data storage to your applications for downstream usage. Note that this is only suitable if your datasets are small enough to be manually validated and changes in your data are infrequent enough not to require automated integration.If you plan to scale, operate in a dynamic environment, or just want to be better prepared for your evolving data needs, it’s better to invest in a unified data integration platform from the outset. Why LIKE.TG is The Right Choice for Your Organization’s Reverse ETL Needs LIKE.TG’s unified platform enables you to perform various data-related tasks and glean valuable insights using a single interface. Our zero-coding framework, paired with rapid onboarding, ensures you can quickly incorporate LIKE.TG into your workflow and benefit from personalized solutions for your use case with minimal downtime. The drag-and-drop interface makes it easy to create a dataflow and schedule it to run depending on your needs. LIKE.TG’s comprehensive data management suitetackles various data-related challenges. With our range of modules, you can simplify your Reverse ETL processes, make sense of unstructured data, upgrade your data integration, build data warehouses, and improve your EDI and API management—all while using the samehighly intuitive UI and experiencing consistent ease-of-use. With an established presence in the data management sector and experience in the finance, retail, government, healthcare, and insurance industries, we can help you develop better Reverse ETL processes and enjoy hassle-free end-to-end data management. Conclusion Investing in the right Reverse ETL tool leads to better decision-making, increased operational efficiency, and the quick movement of enriched data to the required destinations. Discover what LIKE.TG can do for you with a free 14-day trial or request a quote today to get started.
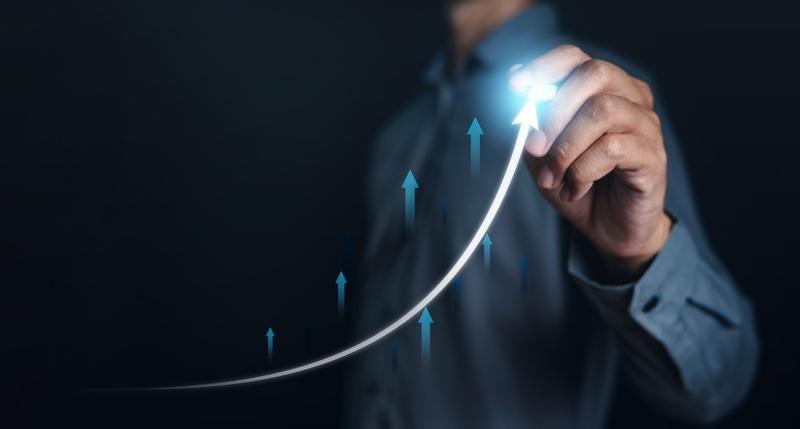
5 Best Claims Automation Software in 2024
IBM estimates that the insurance industry contributes significantly to the creation of 2.5 quintillion bytes of data every day, with claims data being a major contributor to this massive volume. Manual processing of this data is no longer practical, given the large data volume. Instead, automated claims processing software can handle this data efficiently and accurately. The right software can transform the efficiency and accuracy of claims handling, and directly impact operational productivity and customer satisfaction. In this article we will discuss the best claims automation software for businesses that can meet varying requirements. The 5 Best Automation Claims Processing Software LIKE.TG ReportMiner LIKE.TG ReportMiner is an advanced data extraction tool designed to automate and enhance the process of extracting information from unstructured documents. This makes it particularly useful in industries like insurance, where claims processing involves dealing with a variety of document types and formats. Key Features ReportMiner excels at extracting data from a range of unstructured sources such as PDFs and text documents, which are commonly used in insurance claims. Creates custom templates for data extraction, which can be reused for processing documents of a similar layout. It enhances efficiency in handling recurring claims. Uses OCR to extract data from extractable (with text) and non-extractable (with images or scans) PDFs. Uses advanced AI algorithms to identify and extract relevant data fields from unstructured files automatically. This reduces the need for manual efforts in creating extraction templates and speeds up the claims processing. ReportMiner extracts data and offers features for cleansing and transforming the extracted data. This ensures the accuracy and consistency of data before the claims processing. Exports data in various formats such as CSV, Excel, XML, and more. It makes it adaptable to different downstream processing requirements. Enables the automation of complete workflows, from data extraction to delivery, streamlining the entire claims process. Docsumo Docsumo is an AI-driven platform that leverages machine learning and intelligent OCR technology to streamline insurance claims processing tasks. Key Features Uses OCR to extract data from unstructured documents, converting them into an analyzable and processable format. Utilizes machine learning for data extraction, adapting to different document layouts and formats commonly encountered in insurance claims. Docsumo has automated cloud backup and data recovery. This ensures data security and availability in claims processing. Works with multiple document types, like forms or invoices. Nanonets Nanonets is an AI and ML-powered platform designed to automate various aspects of document processing in insurance claims. Key Features Employs machine learning algorithms to achieve accuracy in extracting information from unstructured data. Nanonets leverages AI to classify and categorize claims based on their content. Uses AI and ML to automate the extraction of information from a variety of documents such as invoices, medical reports, and claim forms. Through machine learning models, it helps in detecting fraud and identifying fake documents. Abby FlexiCapture It is a data capture solution designed to automate and streamline health and medical insurance claims processing. Key Features Simplifies the claims entry process by automating it with various validation rules. Abby FlexiCapture supports all standard health insurance claim forms, including claim attachments. Accurately extracts data from health and medical insurance claims. Provides organizations with greater visibility into their documentation, enhancing workflow management. Skyvia Skyvia is a cloud-based integration platform that offers solutions to enhance the automation of claims processing. Key Features Offers scalable and accessible data management from any location, enhancing the flexibility of claims operations. Skyvia’s no-code approach to data management simplifies the process of maintaining data integrations. Allows customized connections between different software systems through API integration. Gives insights from claims data through advanced analytics and reporting tools. How to Choose the Right Automated Claims Processing Software When choosing processing software, it is important to select a tool that complements and enhances the entire claims process. This means picking a tool that easily fits in and helps do things like handle data, automate tasks, and make operations smoother. Different claims processes may need different tools, so it is essential to understand this to make the best choice. There are a few key factors for selecting the ideal processing software to improve your claims process. Integration Capabilities Seamless integration with the existing business systems, like HR, accounting, and CRM, allows data to flow smoothly between systems. It minimizes the disruption and reduces the need for manual data entry and removes errors. Centralized Access Integrating the automated claims software with different data repositories allows the system to aggregate and present all relevant information in a unified dashboard. It centralizes data from multiple sources and makes it easily accessible in one place. This approach ensures that users can retrieve all necessary data, such as policy details, claim history, and customer interactions, from a single point. It reduces the time spent switching between different systems and databases. Accurate Data Management Such software utilizes automated validation and cross-referencing algorithms to identify inconsistencies in data entries, to ensure the consistency and accuracy of data. This feature maintains the integrity of the claims process, as accurate data is essential for fair and timely claim resolutions. User Friendly Interface Simple navigation and a clear logical layout of features ensure that both insurers and users can use the software with minimal training. This ease of use speeds up the claim submission and processing. It also reduces the potential frustration and errors associated with complex or poorly designed interfaces. Customization Flexible claims processing software should offer customization options to suit specific business needs. Customization can range from configurable fields and forms to adaptable workflow rules. It allows the software to adapt to various claim types or specific operational requirements, thereby enhancing efficiency and relevance in the claims handling process. Scalability Software designed for scalability can handle increasing workloads and adapt to growing claim volumes without the need for significant system changes. It ensures that your investment remains effective and relevant as your business expands. Analytics Select software that offers comprehensive analytics for tracking performance and identifying improvement areas. Advanced analytics tools should be capable of generating detailed reports and real-time data visualizations. These are instrumental in monitoring the effectiveness of the claims process and guiding continuous optimization strategies. Future Proofing An ideal claims processing software is flexible and capable of integrating with evolving technologies. Such futureproofing involves ensuring compatibility with emerging tech trends, like AI and machine learning. It enhances the software’s capabilities and keeps your claims processing at the forefront of industry developments. Why Invest in Claims Automation Software Investing in claims processing software can be a strategic decision for companies, like the insurance and health sectors. Automated systems offer several benefits. Automated claims processing software can significantly reduce the time it takes to process claims. It does this by streamlining workflows, automating data entry, and categorizing claim-related data. Automated systems minimize human error, leading to more accurate claims processing. It utilizes precise data extraction algorithms which ensure consistency and accuracy in data handling. Adoption of automated claim processing software can reduce the cost of a claims process by as much as 30%. It frees up resources for more strategic tasks, thereby enhancing overall productivity and service quality. Efficient claims processing software is proficient at handling high volumes of claims without compromising on quality or efficiency. It leverages automated workflows to ensure consistent quality even under increased workload. Automated claims processing software can effectively detect and prevent fraud with advanced analytics and pattern recognition. Conclusion Investing and choosing efficient automated claims processing software leads to faster claims resolution and higher accuracy. Opting advanced claims processing software like LIKE.TG ReportMiner can streamline operations by efficiently handling unstructured data. With features like data extraction, automation, and AI algorithms, it ensures accuracy and speed in claims processing. Try LIKE.TG for free with a 14-day trial or request a quote today. Take a step forward in addressing the complexity of modern claims processing. Streamline the Process of Handling Claims Processing Looking to simplify the complex process of Data Extraction? Try LIKE.TG Reportminer for free for 14 days and optimize your workflow. Request a Demo
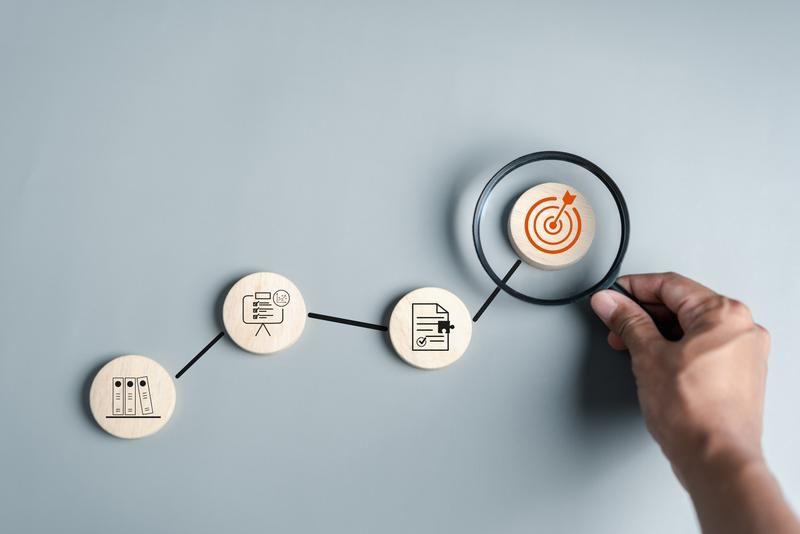
Ensuring Enterprise Data Privacy: 2024 & Beyond | Tips From 6 Data Experts
As we approach Data Privacy Day on January 28th, it’s crucial to recognize the significance of enterprise data privacy in our increasingly digital world. Data privacy is a fundamental aspect that businesses, especially those dealing with vast amounts of data, must ensure to protect sensitive information. To better understand this important topic, we’ve gathered insights from industry experts who navigate the complex landscape of data privacy daily. Their perspectives offer valuable guidance for enterprises striving to safeguard their data in 2024 and beyond. These insights touch upon: The growing importance of protecting data. The role of data governance. The impact of industry regulations. Balancing the benefits and risks of AI. Resolving data security issues. Emergence of new technologies. Going beyond compliance. The Growing Importance of Data Privacy Merrill Albert, an experienced Enterprise Data Leader and Governance Officer, emphasizes that data privacy is not a fleeting concern but a persistent issue that can have far-reaching consequences if neglected. “Data privacy is becoming more and more important as our data resides with so many companies. Those data privacy issues still exist and will become more important if they don’t pay attention to what happens with the data,” Albert asserts. In a world where our personal and sensitive information is dispersed among countless organizations, it’s not hyperbole to say that data privacy affects us all. Our data isn’t just a digital asset; it’s a piece of ourselves that deserves protection. Neglecting data privacy isn’t an option; it’s a risk that can lead to unintended consequences. The Role of Governance in Data Privacy Data privacy is a discipline that requires robust governance. “Data privacy is a data discipline that needs to be governed. If the people working on governance handle privacy, they’ll work on identifying where private data lives, understand privacy rules, communicate to data users, and monitor adherence to privacy rules,” Albert adds. Enterprises need to clearly understand where private data resides, be well-versed in privacy regulations, and ensure effective communication about data usage policies. Data privacy isn’t an abstract concept; it’s a fundamental aspect of our digital lives that should be governed with care, understanding, and respect for individuals. It’s about creating a digital world where privacy isn’t a luxury but a right and where trust is built on a foundation of responsible data handling. The Impact of Regulations like GDPR Vincent Rainardi, a Data Architect and Author, highlights the impact of regulations like the GDPR on enterprises. GDPR has revolutionized how customer data is handled, mandating that personal data, whether it’s a name or a bank balance, be stored securely and accessed judiciously. Rainardi emphasize this by stating, “Access to personal data must be limited… This means that we need to classify information, which one is PII (Personally Identifiable Information) and which one is SPII (Sensitive PII).” The ripple effects of GDPR extend beyond mere data storage and access. It challenges organizations to rethink their entire data lifecycle, especially within data warehouses and during data migration projects. Rainardi highlights a critical operational aspect: the retention period of personal data. He points out, “Data warehouses cannot store personal data more than the specified period (say 7 years), which means that there must be a facility within the data warehouse or data lake to identify PII data which has been stored for more than 7 years and destroy them. Not only in the data lake/warehouse but also in all other data stores.” This requirement compels organizations to implement sophisticated data identification and retention management mechanisms. It’s not just about adhering to regulations; it’s about embedding the principles of privacy and respect for personal data into the very fabric of data infrastructure. The need for robust data governance and comprehensive data catalogs has never been more pressing. They are not just tools or processes but are foundational to ensuring that organizations respect individual privacy rights while harnessing the power of their data assets. Balancing AI Benefits and Data Privacy Risks Antonio Grasso, Author of Toward a Post-Digital Society, Technologist, and Sustainability Advocate, sheds light on the delicate balance enterprises must maintain in the AI-driven business era. Grasso’s insights are especially pertinent as we delve deeper into an era where AI’s capabilities are not just luxuries but necessities for staying competitive and innovative. “Enterprise data privacy is a critical and complex issue – especially in the current AI-driven business era. Organizations must balance the benefits of AI, such as enhanced analytics and automation, with the risks of data breaches and privacy violations.” This statement opens a dialogue about the dual-edged nature of AI. On the one hand, AI serves as a catalyst for breakthroughs in analyzing vast datasets, automating repetitive tasks, and even forecasting market trends, which can lead to significant growth and operational efficiency. On the other hand, the intensification of data usage brings forth a range of privacy concerns. The risk of data breaches, unauthorized access, and misuse of personal information are not just hypothetical scenarios; they are real challenges that organizations grapple with daily. Grasso believes that the essence of addressing these challenges does not lie in avoiding the adoption of AI but in fostering a culture of responsible AI utilization and data handling. He articulates a clear roadmap: “I believe the key lies in implementing robust data governance policies, employing encryption and anonymization techniques, and ensuring compliance with evolving regulations – like GDPR in Europe.” This perspective emphasizes not just the implementation of technical safeguards but also the establishment of comprehensive governance structures that oversee the ethical use of data and AI.” However, his insights go beyond technical fixes. He reinforces the dynamism of regulations like GDPR, which are not static but evolve to address emerging privacy concerns in an AI-driven ecosystem. Hence, staying compliant is not a one-time task but a continuous journey of adaptation and vigilance. Furthermore, Grasso touches on a crucial aspect often overlooked in the race to harness AI: the human element. He asserts, “Many agree that as AI technologies evolve, so does the need for transparent and ethical data handling practices, making continuous adaptation and employee training essential for safeguarding sensitive information.” This statement highlights the importance of cultivating an organizational culture where every stakeholder is aware of and committed to data privacy principles and ethical AI use. Regular training, open communication, and a transparent approach to data use and protection are fundamental to building this culture. Addressing Data Sharing and Security Challenges Jay Mishra, COO of LIKE.TG, draws attention to the intricacies of data sharing in our interconnected corporate world, fraught with privacy challenges. He emphasizes the necessity of secure data sharing, stating, “Data, even when anonymized, can sometimes be traced back, leading to potential privacy breaches. Securing data is not just about avoiding risks; it’s about building confidence.” Mishra advocates for robust data management as a fundamental defense against unauthorized access. “The key to any solid data security approach lies in effective data management by overseeing data from its initial collection right through to its final deletion. Done well, this management is a powerful shield against unauthorized access,” Mishra adds. The Emergence of Privacy-Enhancing Technologies (PETs) in Data Management Henry Orlando Clavo, a Data Professional and Technology Consultant, predicts that 2024 will be a significant year for data privacy, especially with the adoption of Privacy-Enhancing Technologies (PETs). Clavo believes, “These tools and technologies are crucial for businesses that strive to use the power of Artificial intelligence while respecting the privacy of the users and complying with regulations like GDPR and CCPA.” His insight sheds light on the evolving landscape of data management, where respect for privacy and technological advancement go hand in hand. Elevating Data Privacy Beyond Compliance William Mcknight, President of Mcknight Consulting Group and a globally recognized thought leader in the data space, emphasizes the evolution of data privacy from a mere compliance requirement to a core element of consumer trust. Mcknight highlights the paradigm shift in consumer expectations and the role of corporate leadership in championing data privacy. He says, “Data privacy is no longer just a box-ticking exercise, but a fundamental trust builder. Consumers are becoming more data-savvy, demanding transparency and control. C-suite leaders must champion data privacy within their organizations, dedicating resources, building awareness, and fostering a culture of data responsibility.” In essence, data privacy is not a technicality or a buzzword; it’s about earning and maintaining the trust of the people whose data we handle. It’s a shift from mere compliance to a deeper commitment to respecting the privacy and expectations of consumers in this data-driven world. As we observe Data Privacy Day, it’s clear that the journey toward robust data privacy is ongoing and multifaceted. Understanding complex regulations like GDPR and embracing advanced technologies like PETs are essential. The insights from these experts highlight the need to create a privacy-focused culture where data protection is embedded in every action and decision. In the dynamic landscape of data privacy, staying informed and agile is not just beneficial; it’s indispensable.
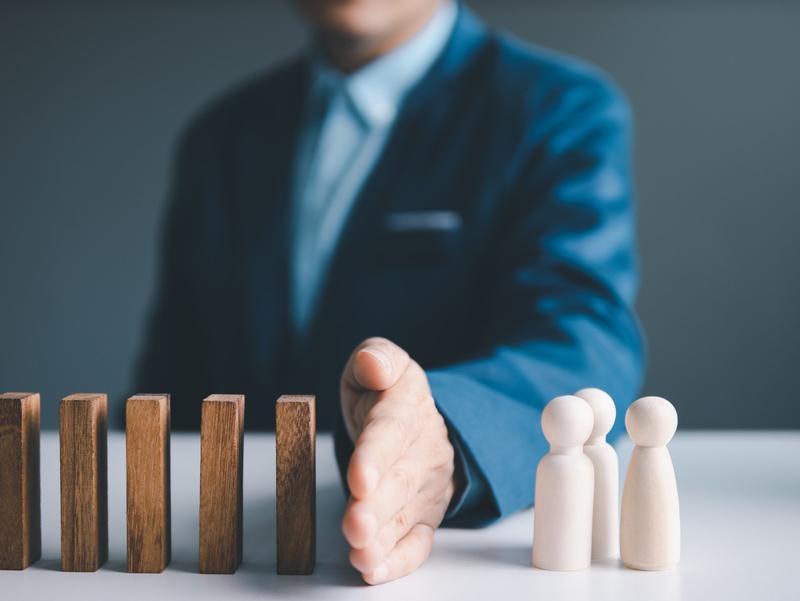
How Automated Financial Data Integration Streamlines Fraud Detection
Do you know proactive fraud detection and prevention could save organizations up to a staggering $5.9 billion annually? However, gathering relevant data for this purpose is no easy task. Financial data integration plays a crucial role in the fight against fraud, enabling organizations to merge data from various sources and formats into a unified, consistent view.
However, gathering relevant data for this purpose is no easy task. Financial data integration faces many challenges that hinder its effectiveness and efficiency in detecting and preventing fraud.
Challenges of Financial Data Integration
Data Quality and Availability
Data quality and availability are crucial for financial data integration project, especially detecting fraud. Fraudsters often exploit data quality issues, such as missing values, errors, inconsistencies, duplicates, outliers, noise, and corruption, to evade detection and carry out their schemes.
According to Gartner, 60% of data experts believe data quality across data sources and landscapes is the biggest data management challenge.
Additionally, some data sources may be hard to access, unreliable, or outdated, which may compromise the completeness and timeliness of the financial data integration process.
Therefore, data quality management is essential to ensure that the data is accurate, consistent, and reliable. Data quality management involves various techniques, such as data cleansing, validation, verification, and reconciliation, to identify and resolve data quality problems. Data quality management can have significant benefits for organizations, such as:
Reducing wasted resources lost revenue, and increased risk. According to a survey by Experian, 95% of organizations see negative impacts from poor data quality, such as increased costs, lower efficiency, and reduced customer satisfaction.
Saving money and boosting the economy. According to a report by IBM, poor data quality costs the US economy $3.1 trillion a year, which is equivalent to 17% of the US GDP. Improving data quality can help reduce these losses and increase productivity and innovation.
Enhancing data governance and customer insights. According to a study by SAS, only 35% of organizations have a well-established data governance framework, and only 24% have a single, integrated view of customer data. Data governance is the process of defining and implementing policies, standards, and roles for data management. Data governance can help improve data quality, security, and compliance, as well as enable better decision-making and customer service.
Data Integration and Transformation
The financial data integration process consists of two core tasks: extracting data from multiple sources and converting it into a unified and consistent view. These tasks are challenging, as they involve various issues, such as:
Data heterogeneity: Data sources may have different structures, formats, and semantics, which need to be reconciled and aligned.
Data mapping: Data sources may have different identifiers, values, and units, which need to be translated and standardized.
Data transformation: Data sources may have different quality, granularity, and complexity, which to be cleaned, validated, aggregated, filtered or transformed in any other way.
Data consolidation: Data sources may have redundant, conflicting, or missing data, which need to be resolved and integrated.
Data integration testing: Data sources and transformations may have errors, bugs, or anomalies, which need to be detected and corrected.
These tasks also require high performance and efficiency, as they may deal with large volumes and varieties of data. According to a report by Gartner, data integration and transformation account for 60% of the time and cost of data warehouse projects.
How Automated Data Pipelines Assist in Financial Data Integration for Fraud Detection
Automated data pipelines enable the creation, execution, and management of financial data integration workflows without requiring extensive coding or manual intervention. They offer many features that make financial data integration for fraud detection easier:
Drag-and-drop interface: Automated data pipelines provide a user-friendly and intuitive drag-and-drop interface that allows users to design and configure financial data integration workflows with ease and flexibility. Users can simply drag and drop pre-built data sources, transformations, destinations, and other components onto a graphical canvas and make mappings to create customized data pipelines. Users can also customize the properties and parameters of each component and preview the results of each step.
Connectivity to a diverse range of sources: Automated data pipelines support connectivity to a diverse range of data sources, such as databases, structured and unstructured files, web services, cloud platforms, and applications. Users can easily access and extract data from various sources regardless of their location, format, or structure. Users can also use pre-built connectors or create custom connectors to integrate with any data source.
Pre-built transformations: Automated data pipelines offer a rich set of pre-built transformations that can perform various data manipulation and processing tasks, such as data cleansing, data validation, data conversion, data aggregation, data filtering, data sorting, data joining, data splitting, data pivoting, and data enrichment. These transformations help address errors, ensure conformity, facilitate interoperability, provide summaries, focus on relevant subsets, organize data, integrate diverse sources, extract specific information, restructure for different perspectives, and augment datasets with additional context. Users can also create custom transformations, write expressions, or use scripting languages to perform complex or specific data transformations.
Data quality management: Automated data pipelines facilitate data quality management, which is the process of ensuring that the data is accurate, complete, consistent, and reliable. Data quality management involves various tasks, such as data profiling, data cleansing, validation, verification, reconciliation, and auditing.
Workflow automation: Automated data pipelines enable workflow automation. This allows users to not only create self-regulating data pipelines, but automate tasks that typically require manual interference.
Change data capture (CDC): Automated data pipelines support change data capture (CDC), which is the process of capturing and transferring only the changes made to the data sources rather than the entire data sets. CDC is useful for financial data integration for fraud detection, as it enables the detection of fraud in near real-time and reduces the data volume and latency.
Managed file transfer (MFT): Automated data pipelines support managed file transfer (MFT), which is the process of securely and reliably transferring files between different systems and locations. MFT is useful for financial data integration for fraud detection, as it enables the exchange of data with various stakeholders, such as customers, partners, vendors, and regulators.
Security: Automated data pipelines ensure security, allowing you to protect the data and the data integration workflows from unauthorized access, use, modification, disclosure, or destruction. Security is vital for financial data integration, as it involves sensitive and confidential data that may have legal or regulatory implications.
Protect Yourself Against Fraud using LIKE.TG’s Automated Data Pipeline Builder
LIKE.TG’s Automated Data Pipeline Builder simplifies the Financial Data Integration process using a super-simple no-code drag-and-drop interface.
Book Your Free Trial Now!
Best Practices and Tips for Optimizing and Troubleshooting Financial Data Integration
Optimize Data Sources
You must take some strategic measures to improve your data sources. First, you should refine your selection process and opt for only relevant data fields. Second, you should use filters to exclude irrelevant information and reduce overall data volume.
For instance, in the analysis of credit card transactions, you should focus on essential data fields like transaction amount, date, time, location, merchant, and customer. To further streamline, you should consider excluding transactions falling below a specific threshold or those from trusted sources through the application of targeted filters.
This not only trims unnecessary data but also directs attention to transactions with a higher likelihood of being fraudulent. Third, you should employ techniques such as indexing and partitioning the data tables based on transaction date, time, or location. This can boost data retrieval performance and expedite fraud detection.
Leverage Data Transformations Efficiently
You need to make thoughtful choices in both method and mode to efficiently leverage data transformations. You can consider in-memory, pushdown, bulk, and parallel processing options. To streamline the process, you should avoid unnecessary or redundant transformations and enhance performance by implementing caching and buffering techniques during data processing.
For instance, if you’re consolidating data from multiple sources, it’s advisable to conduct transformations within the source or target system, opting for pushdown/ELT processing. Doing so minimizes data movement, reduces latency, and boosts overall processing speed.
Moreover, if your data types, formats, or units are already consistent across sources, you should skip unnecessary transformations. To further enhance performance and prevent redundant operations, you should store intermediate transformation results in memory or on disk using caching and buffering techniques.
Automate Data Mapping
Automating data mapping can help you save considerable time and effort and create complex expressions for data mapping. One of these features is the auto-mapping feature, which can automatically map your data elements if they have the same or similar names. This feature helps you reduce human errors and speed up the process.
You can also leverage the expression mapping feature to create complex expressions for data mapping, such as concatenating, splitting, or calculating data elements. This can help you create new and useful data elements. For example, you can create a unique identifier for the customer by concatenating their first name and last name.
In addition, you can use some features that can help you map your data elements based on a lookup table or a similarity score. One of these features is the lookup mapping feature, which can map your data elements based on a reference table that contains the list of valid or invalid merchants or customers. This can help you identify and flag transactions that involve suspicious parties.
Another feature is the fuzzy mapping feature, which can help match similar strings. This can help you deal with data elements that are not the same but close enough, such as misspelled or abbreviated names.
Enhance Data Quality
Data quality is important for fraud detection, as it affects the accuracy, consistency, and reliability of the data. To optimize the data quality, you can use various tools and techniques, such as:
Data quality rules: These are rules that check and enforce the data quality standards, such as completeness, uniqueness, validity, consistency, and accuracy. You can use the pre-built data quality rules or create your own data quality rules by using the data quality wizard or the data quality editor. For example, you can create a data quality rule that checks if the transaction amount is within a reasonable range and, if not, rejects or flags the transaction as potentially fraudulent.
Data quality reports: These reports illustrate the data health of a particular dataset. You can use the data quality reports to visualize and communicate the data quality status and trends and to support data quality analysis and decision-making.
Data quality alerts: These are alerts that receive notifications and alerts when the data quality rules are violated and take appropriate actions, such as correcting or discarding the data or notifying the data owners or stakeholders. You can use the data quality alerts to ensure data quality compliance and accountability and to prevent or mitigate data quality risks.
Load and Sync Data Optimally
Data destination is important for fraud detection, as it affects the delivery and storage of the data. To optimize the data destination, you can choose the most suitable and efficient options, such as:
Destination type and format: These are the type and format of the data destination, such as the database, the file, web services such as APIs, the cloud platform, or the application. You can choose the destination type and format depending on the data usage and consumption. For example, you can consume APIs as a data source to access real-time data required for fraud detection.
Load mode: This is the mode of loading the data to the data destination, such as the full load, the incremental load, the upsert, or the insert. You can choose the load mode depending on the data volume and frequency. For example, if you want to load the complete data from the source to destination, you can choose full load. If you want to load it incrementally since the last load, you can choose incremental load. If you want to insert new records in the destination database, choose insert. If you want to insert new records in case they don’t exist in the database and update records in case they do exist, we would choose upsert. Note that incremental-load/full load are not alternatives of upsert/insert. The choice between incremental and full load is separate from that of insert and upsert.
Change data capture (CDC): This is a feature that captures and transfers only the changes made to the data sources rather than the entire data sets. You can use the CDC feature to reduce the data transfer and storage costs, and to improve the data freshness and timeliness for fraud detection.
Configure Workflow Automation
Enhancing workflow automation ensures your data pipeline workflow is optimized for fraud detection. You can configure workflow triggers based on pre-defined frequencies or events, such as data arrivals, changes, quality violations, or fraud alerts. This feature helps you activate and respond to changes that affect fraud detection processes. For instance, you can use this feature to start a workflow when a new file arrives in a folder, when a data quality rule is violated, or when a fraud alert is generated by another system or application.
Once the workflow is established, you must monitor the pipeline. You need to set up metrics to review your pipeline’s performance. For instance, you should monitor how long your data pipeline workflow takes to run, how many records it processes, how many errors or warnings it encounters, and how many actions it performs. You can also use this feature to optimize your data pipeline workflow by changing the parameters, variables, or settings, as needed, to improve the data processing speed, accuracy, and reliability for fraud detection.
Configure Workflow Log
If you’re creating a fraud detection workflow, it’s essential to configure, view, and export the log of your data pipeline using filters, preview, and export functions. This helps you review and analyze the details and history of your data pipeline workflow, and to support your data pipeline workflow audit and evaluation. For instance, you can use this feature to see the start and end time of your data pipeline workflow, the parameters and variables used, the input and output data sets, the data quality rules applied, and the data destination details.
You can also use this feature to optimize your data pipeline workflow by checking the data pipeline workflow execution and results and by finding and correcting any data quality issues for fraud detection.
Set Up Alerts
Your data pipeline needs to send you notifications and alerts when the workflow is completed or failed, using email, SMS, or push notifications. This makes sure that your data pipeline workflow is finished and successful, and to take appropriate actions, such as correcting or discarding the data or notifying the data owners or stakeholders in case of any failures or errors.
For instance, you can use this feature to receive an email, an SMS, or a push notification when your data pipeline has executed successfully or run into an error and to see the summary and status of your data pipeline.
You can also use this feature to optimize your data pipeline by reducing the data pipeline latency and downtime and by improving the data pipeline responsiveness and reliability for fraud detection.
Optimize Exception Handling
Exception handling helps you resolve errors and exceptions that occur during your data pipeline using error codes, error messages, and error actions.
You can use this feature to troubleshoot and fix the errors and exceptions and to prevent or reduce the data pipeline risks and impacts. For example, you can use this feature to see the error codes and error messages that explain the cause and type of the errors and exceptions and to perform the error actions that tell you the steps and solutions to resolve the errors and exceptions.
You can also use this feature to optimize your data pipeline by avoiding or minimizing the data pipeline failures and errors and by enhancing the data pipeline quality and security for fraud detection.
Workflow Recovery
This feature lets you recover and resume your data pipeline workflow from the last successful point using checkpoints, snapshots, and rollback functions. You can use this feature to restore and continue your data pipeline workflow and to avoid losing or repeating any data or work. For example, you can use this feature to see the checkpoints and snapshots that save the state and progress of your data pipeline workflow and to use the rollback function to go back to the last successful point. You can also use this feature to optimize your data pipeline workflow by preserving and recovering the data pipeline workflow data and work and by improving the data pipeline workflow efficiency and continuity for fraud detection.
How LIKE.TG Enables Financial Data Integration
Financial data integration for fraud detection requires careful planning and execution. With the help of automated data pipelines, such as LIKE.TG Automated Data Pipeline Builder, you can achieve data integration for fraud detection with ease and efficiency.
Automated data pipelines assist in financial data integration for fraud detection in several ways, such as a drag-and-drop interface, connectivity to a diverse range of sources, pre-built transformations, data mapping, data quality management, workflow automation, CDC, MFT, security, and analytics add-on.
LIKE.TG automated data pipeline builder is a powerful tool that can help you streamline financial data integration. With LIKE.TG, you can:
Connect to various data sources and destinations, such as databases, files, APIs, cloud services, and more without writing any code.
Transform and enrich your data with built-in functions, expressions, and business rules using a drag-and-drop interface.
Automate and schedule your data pipelines to run on-demand or at regular intervals with advanced error handling and monitoring features.
Monitor and manage your data pipelines with real-time insights and alerts.
Whether you need to integrate your financial data for reporting, analysis, compliance, or other purposes, LIKE.TG can help you achieve your goals faster and easier.
If you want to see how LIKE.TG can work for your specific use case, you can sign up for a free 14-day trial or schedule a custom demo with our experts. Don’t miss this opportunity to take your financial integration to the next level with LIKE.TG!
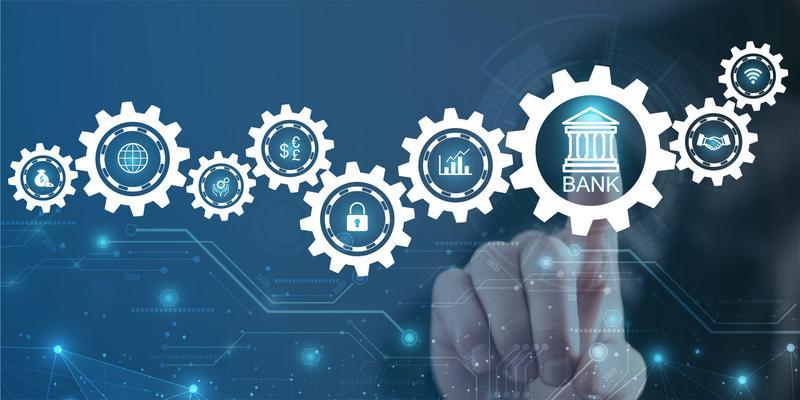
What Is Reverse ETL? The Complete Guide
What is Reverse ETL? Reverse ETL is the process of moving data from the central data warehouse to operational and analytic tools, therefore, it’s essentially an ETL process, except the data moves in the opposite direction. Reverse ETL is essential for businesses that use various tools, such as CRMs, marketing automation platforms, and sales tools, as it enables seamless data flow and empowers informed decision-making for growth, marketing, sales, and support. Reverse ETL ensures that the most up to date data is available in Business Intelligence (BI) and visualization tools, and data visualization, making them more effective and efficient in their operations. Put simply, reverse ETL is an important element in the modern data stack and helps businesses get the right data to the right tools at the right time, making their work easier and more productive. How Does Reverse ETL Fit in Your Data Infrastructure Reverse ETL helps bridge the gap between data warehouse and operational applications and systems. It fits into your data infrastructure to deliver up-to-date data to business teams, enabling them to make informed decisions. By integrating with pre-built connectors, reverse ETL eliminates complex configuration and simplifies the process of moving data out of the warehouse. This facilitates the real-time flow of data from data warehouse to reporting dashboards and operational analytics tools, accelerating data processing and providing business leaders with timely information. Reverse ETL, used with other data integration tools, like master data management (MDM) and change data capture (CDC), empowers employees to access data easily and fosters the development of data literacy skills, which enhances a data-driven culture. This accessibility allows teams to experiment with data, iterate their strategies, and drive innovation. Additionally, reverse ETL supports data governance by providing visual representations of data lineage, versioning support, and historical data tracking, enabling organizations to maintain policies and adapt strategies rapidly when necessary. ETL vs Reverse ETL ETL Reverse ETL Purpose Aggregates and organizes data from various sources for analytical processing. Distributes processed data from the data warehouse to operational systems for action. Direction of Data Flow From source systems (like databases, CRM, ERP) to a centralized data warehouse. From a centralized data warehouse to operational systems and applications. Primary Users Data engineers and analysts who prepare and analyze data. Business users in marketing, sales, customer service, and operations who execute data-driven actions. Data Movement One-way data flow into a data warehouse for storage and analysis. Cyclic data flow, enabling operational systems to be updated with insights from the warehouse. Primary Focus Integrating, cleansing, and storing data for reporting and analysis. Operationalizing insights from stored data and making them actionable in day-to-day business operations. Use Cases Data warehousing, business intelligence, reporting, and data analytics. Data enrichment for CRM, targeted marketing campaigns, real-time customer interaction, and personalized experiences. Impact on Business Facilitates data-driven decision-making through historical analysis and reporting. Enhances operational efficiency and customer experiences by leveraging insights in real-time business processes. Simplify your ETL and Reverse ETL processes! LIKE.TG Centerprise makes ETL and reverse ETL processes super easy using its drag-and-drop interface, cutting significant amounts of time and money investments – all while using zero code! Try now – 14 days free! Functionalities of Reverse ETL Reverse ETL makes stored information accessible to the people who need it. This means that teams like Sales, Marketing, and Customer Support can use this information to make informed decisions and provide more personalized experiences for customers. Instead of just having a bunch of complicated datasets sitting around, businesses can use this data to improve their day-to-day operations. First, reverse ETL can operationalize data by turning abstract information into something measurable and useful for businesses. For example, sales teams can use Reverse ETL to import customer data from the data warehouse into a CRM system like Salesforce. This data could include customer purchase history and interaction logs, enabling the sales team to tailor their pitches and follow-ups to individual customer needs and preferences. Reverse ETL tools help access data from different departments within the company. This means that teams can easily find relevant information without having to ask someone else to create a report. With these tools, it’s easy to break down data silos and access related information. For example, HR teams can use Reverse ETL to pull data from employee surveys stored in a data warehouse into HR management systems. This could help in identifying patterns in employee feedback related to workplace satisfaction or areas for improvement. Reverse ETL combined with data warehouse helps data analysts save time allowing them to focus on more complex tasks such as making sure their data is high quality, keeping it secure and private, and identifying the most important metrics to track. Guide to the Workflow of Reverse ETL There are four main aspects to reverse ETL: Data Source: It refers to the origin of data, like a website or a mobile app. Data Models: These define the specific sets of data that need to be moved. For-example a set of customers purchased behaviors compiled for analysis. Destinations: These are the places where the data set is directed, like data sent to a marketing automation tool. Data Mapping: Mapping is the process of aligning your data from the warehouse to fit specific fields in target destinations. For instance, aligning customer ID fields in the data warehouse with the corresponding fields in a Salesforce CRM. The process basically starts with data extraction and ends with flawless data activation. Extraction It is the process of extracting data from data warehouse using an automated data extraction tool and data integration platforms, specifically designed for handling and transferring large volumes of data efficiently. 2. Transformation After obtaining data it needs to be transformed, to fit the format of its destination. In this case, data mapping comes in handy as it can trace the movement of data between storage systems and tools and put it into specific fields. 3. Loading Next, load the transformed data into its target destination using various methods. For example, using an API for a direct connection, batch processing for handling large volumes of data at once, etc. 4. Activation Once the data is loaded into downstream tools and applications, it can be used by internal teams and even trigger specific actions automatically, like sending personalized emails to customers based on their behavior. 5. On-going Monitoring The final step is to keep an eye on the process. Organizations employ holistic data integration and management tools alongside Reverse ETL tools for monitoring. This ensures a smooth and reliable data flow between their centralized data repository and operational systems. These tools can spot issues like errors or failed data transfers, maintaining data quality and reliability. Reverse ETL Use Cases To determine if reverse ETL is the right fit for your business, it’s important to understand its practical applications. Let’s explore a few examples of how companies can benefit from implementing reverse ETL. Data Activation When it comes to SSA or analytics enablement, businesses tend to focus on the human aspect of it, emphasizing the user’s ability to easily understand and interact with the data. But the way data is presented is equally important, as it directly impacts how users comprehend, interpret, and make decisions based on the data. By providing data insights, businesses can make their data warehouse more accessible and usable for their employees. With Reverse ETL, businesses can automate the flow of data between their data warehouse and operational systems, making it easier for employees to access the data they need to make informed decisions. For example, analysts can feed lead ratings from the data warehouse into a custom field in Salesforce, which can then be used in any operational analytics use case to obtain new business. This eliminates the need for sales staff to use BI reports and allows them to focus on closing deals. Data Automation As organizations grow, their process flows become increasingly complex, making it difficult to identify which processes are causing problems in both internal and external operations. Reverse ETL automates the transfer of data from centralized data warehouses to various operational systems. It uses SQL to extract and synchronize data to external tools, making the process straightforward and efficient. This automation ensures that any changes in the data warehouse are instantly reflected in other tools. It can also trigger automated actions in business applications based on the synced data. It reduces errors and ensures all systems in the organization use the most current data, enhancing overall data quality management. Personalizing Customer Experience Reverse ETL serves as a data infrastructure option that can generate personalized in-app consumer experiences by synchronizing data from your warehouse to production databases via social media or e-commerce platforms. It establishes a data pipeline that extracts relevant data from the centralized data warehouse. This data is transformed and loaded into production databases that power the application. By leveraging social media or e-commerce platforms as intermediaries, this process ensures that real-time and up-to-date information, such as user preferences or purchase history, is readily available within the application. This, in turn, increases customer happiness and loyalty, benefiting businesses through Customer Lifetime Value and revenue generation. Financial Data Integration Reverse ETL is an important component in the financial sector for streamlining transaction processing. It integrates data from analytics platforms into accounting or financial management systems, ensuring that financial records are always up-to-date and accurate. With this approach, transactional data like sales or expense details can be seamlessly transferred from a data warehouse to financial systems. For instance, retail businesses can use this to sync daily sales data from an analytics tool to their accounting software. This means that every transaction recorded in the sales system is automatically reflected in the financial records, reducing manual data entry errors and ensuring financial data integrity. The real-time updating feature is particularly useful for financial reporting, budgeting, and planning. It enables financial teams to make informed and timely decisions, leading to better outcomes. Inventory Management Reverse ETL is beneficial to inventory management by ensuring that product availability and inventory levels are accurately reflected on e-commerce platforms. Businesses can synchronize inventory data from a centralized data warehouse to their e-commerce platform, providing real-time information about product availability to their customers. For instance, an online fashion retailer can use this method to update inventory levels from their warehouse management system to their online store. This way, if a particular item is selling quickly, the website’s inventory data gets promptly updated, preventing over-selling. Similarly, when new stock arrives, it is instantly available, maximizing sales opportunities. This accurate inventory management not only enhances customer experience by providing reliable information but also optimizes stock levels, reducing the probability of excess inventory or stockouts. Final Word Reverse ETL is a necessity for businesses looking to utilize the power of their data. To make the most of this transformative process, it’s essential to have the right tools in place, and LIKE.TG’s data integration tool is the perfect solution. With its seamless integration and efficient ETL capabilities, LIKE.TG empowers businesses to unlock the full potential of their data and drive real-time decisions. So why wait? Start your journey today with a free 14-day trial of LIKE.TG’s ETL tool and experience data-driven success firsthand.
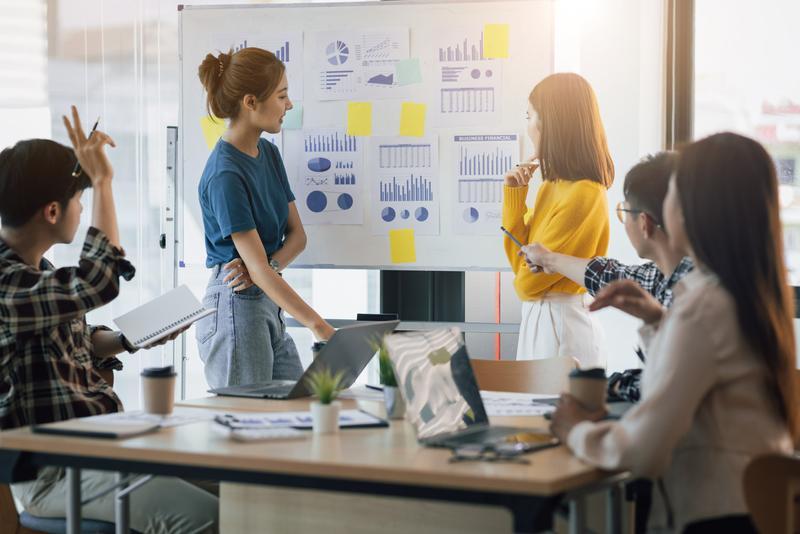
The 10 Best Tools for Microsoft SQL ETL in 2024
Businesses need to integrate data before they can use it to inform their strategic decisions. It is a process that involves consolidating information from various sources, transforming it into a unified format, and loading it into a centralized repository. To streamline and accelerate this process, organizations leverage ETL tools and solutions. A common example is using SQL ETL tools to combine data into a Microsoft SQL Server database. However, with so many tools and solutions to choose from, organizations must do their due diligence to ensure that their SQL ETL solution of choice best satisfies their needs. So, in this article we will explore some of the most capable SQL ETL tools for data integration. But first, let’s get some basics out of the way. Streamline ETL with LIKE.TG—A 100% No-Code Solution Learn More What is Microsoft SQL Server? Microsoft SQL Server is a relational database management system (RDBMS). It’s one of the most popular databases used by businesses of all sizes across industries. Its popularity stems from its ability to efficiently handle structured data, providing a secure and scalable environment for data storage and retrieval. SQL Server’s comprehensive suite of tools and services caters to the diverse needs of businesses. Its extensibility is evident through components, such as SQL Server Integration Services (SSIS) for ETL, SQL Server Reporting Services (SSRS) for creating and managing reports, SQL Server Analysis Service (SSAS) for analytical data models, and so on. While SSIS is Microsoft’s own ETL service, it’s not the only player in the data integration landscape that enables users to implement ETL in SQL Server, as we’ll see later in the article. ETL in SQL ETL SQL scripts allow developers to facilitate the data movement and transformation between various systems. In the data extraction phase, developers retrieve data from source systems such as databases, flat files, APIs, or other data repositories. Example ETL SQL code to obtain data: The transform phase is greatly facilitated by SQL’s rich set of data manipulation and transformation functions, allowing developers to clean, aggregate, and restructure the extracted data to meet the desired format and business logic. Additionally, they can join tables, filter data, and perform calculations. The goal is to prepare the data for optimal loading into the target system. Here’s an example of ETL SQL code to transform data: In the Load phase, ETL SQL scripts enable data professionals to insert, update, or merge the transformed data into the destination tables. Example ETL SQL code to load data: While this manual approach provides developers with a flexible solution for orchestrating the ETL workflow in SQL environment, real world scenarios call for advanced SQL ETL tools and technologies to achieve efficient and scalable data integration. The Need for Microsoft SQL ETL Tools Traditional data integration methods involve extracting information from various sources, transforming it to meet specific business requirements, and loading the refined data into the target database, such as a SQL Server database, using ETL SQL scripts. The demand for SQL ETL tools arises from the need for efficient data management processes within organizations. Businesses need a more streamlined and user-friendly environment to manage their entire ETL workflows. As such, the traditional means of extracting, transforming, and loading data into a centralized repository for decision-making are no longer capable of delivering the requisite time-to-insight. In recent years, there has been a notable shift towards using no-code SQL ETL tools. These tools play a pivotal role in enabling seamless integration and synchronization of data from various sources, ensuring a cohesive and accurate data ecosystem. Particularly, SQL ETL tools provide a user-friendly and intuitive platform that empowers users with diverse backgrounds to design and implement automated data pipelines effortlessly. As a result, they have become indispensable tools for businesses of all sizes. The Best SQL ETL Tools in 2024 As businesses continue to accumulate and integrate vast amounts of data, the demand for robust and efficient tools has never been higher. In this section, we delve into the top-performing SQL ETL tools and explore their features. LIKE.TG LIKE.TG is an automated data management platform with powerful ETL, ELT, and change data capture (CDC) capabilities. It features a 100% no-code UI that’s incredibly user-friendly, making it simple and easy to use even for users without a technical background. Whether you need to manage unstructured data, create fully automated data pipelines, build a data warehouse, manage APIs, or enable frictionless B2B communication via EDI, everything is a matter of drag-and-drop and point-and-click. LIKE.TG’s suite of products includes: ReportMiner to handle unstructured data extraction in bulk Data Pipeline Builder to integrate data via both ETL and ELT Data Warehouse Builder to design a custom data warehouse from scratch API Management to design, publish, and monitor APIs EDI Management to communicate with your trading partners LIKE.TG also offers embedded data quality features and built-in connectors, enabling users to streamline ETL in SQL Server without writing a single line of code. Users can also leverage LIKE.TG’s SQL Server CDC to capture and track changes and ensure up-to-date SQL Server replication. ETL Data to and from SQL Server at Lightning Speeds Whether you want to ETL data into or from SQL Server database, LIKE.TG offers a truly no-code approach to build powerful data pipelines effortlessly. Try for free today. Download Trial Talend (acquired by Qlik) Talend is a data integration platform featuring a drag-and-drop UI and built-in connectors. It offers multiple products, such as Talend Open Studio—an open-source offering—and Talend Data Fabric. While not completely code-free, Talend’s low-code approach is still better than writing ETL SQL scripts manually to integrate data into SQL Server. Given its recent acquisition and the fact that it requires users to have a background in Java, users seek various Talend alternatives for data integration and management. SSIS SSIS is Microsoft’s service for data integration and can be particularly useful in scenarios where an organization is already invested in its ecosystem. It offers seamless integration with other Microsoft products and services, such as a SQL data warehouse, SQL Server databases, Azure services, and Excel, making it a natural choice for organizations already using Microsoft technologies. While it offers a visual interface, it can still be overwhelming for beginners, and there is a learning curve for those who are not familiar with SSIS concepts and practices. Then there’s the issue of limited support for non-Microsoft databases when compared to other ETL tools. Additionally, SSIS is not the best choice for streaming data since it’s largely optimized for batch-processing ETL. Apache NiFi Apache NiFi is another possible SQL ETL tool well-known for its workflow capabilities. Its focus is on processing and moving data reliably. Like other solutions, NiFi has a visual UI to design ETL flows. It offers built-in processors specifically designed for interacting with SQL Server databases. This makes it easy to establish connections, extract data, and load it into SQL Server. Apache NiFi can be a viable choice for ETL with SQL Server given that it offers a balance between usability and visual design. However, mastering all its features requires considerable learning, especially for new users. NiFi’s primary focus is on data movement, and while it does offer basic transformation capabilities, it is not as feature rich as other dedicated ETL tools, such as LIKE.TG, for complex data transformation. This means that users will most likely need to augment NiFi with custom scripts or additional tools to transform the data as needed. Pentaho Pentaho is primarily a business intelligence (BI) tool, but it provides several features for data integration, including ETL. It offers a visual UI and native connectors for SQL Server, simplifying the process of extracting and loading data to and from SQL Server databases. Pentaho supports batch processing well; however, its real-time processing capabilities might be limited compared to some specialized tools. For use cases requiring extremely low-latency data processing, additional tools may be needed, for example, integrating the platform with Apache Spark. Some advanced features and functionalities are only available in the enterprise edition of Pentaho, which requires a commercial license. Informatica Informatica is an enterprise-grade data management tool that offers several products and services to integrate data for BI and analytics. It also offers ETL that allows users to move data to and from several databases, including SQL Server. Informatica is well-known for its complex UI that, in some cases, requires professional certifications to master certain modules. Additionally, its products and services have vastly different interfaces that make it challenging for users to navigate. While it does provide the ability to transform data, complex transformations consume considerable amounts of memory and computing resources. These are some reasons why consumers look for Informatica alternatives. Azure Data Factory Azure Data Factory (ADF) is another one of Microsoft’s ETL services. It’s a cloud ETL service for data integration and transformation. It can be integrated with SSIS, enabling users to run SSIS packages on ADF with full compatibility. And like other SQL ETL tools, Azure Data Factory also offers a visual UI. Users report that ADF’s visual interface quickly becomes cluttered, especially when working with workflows and multiple activities simultaneously. Additionally, even though it does support incremental data loads, the setup is mostly manual and, therefore, complex. Matillion Matillion is another tool that can ETL data to and from a SQL Server database with its native connector. As is the case with data integration solutions these days, Matillion also offers a visual UI with drag-and-drop functionality. Given its focus on cloud data warehouses, Matillion can be a viable option for ETL tasks in cloud environments—even more so if SQL Server is hosted in a cloud environment (e.g. Azure SQL Database). While Matillion does feature a visual design, users typically need additional training and expertise to fully utilize its capabilities. Moreover, with its focus on the cloud, its feature-set is not as optimized for on-premises settings. Finally, Matillion’s pricing is based on concurrent users and data volume, which can be expensive for smaller organizations with limited use cases. All these nuances prompt data teams to look for Matillion alternatives. Stich Stitch is a cloud-based ETL tool with a focus on making the data available for analytics as quickly as possible. Stitch offers a user-friendly interface and pre-built connectors for a wide range of data sources and destinations, including SQL Server. Its integrations are powered by Singer, which is an open-source standard for building data integrations. The use of Singer ensures that Stitch’s integrations are extensible and customizable, providing users with the flexibility to tailor data pipelines to their specific needs. Stitch was acquired by Talend, which in turn was acquired by Qlik, and even though the parent company has publicly committed to supporting Stitch as an independent offering, the overall strategic direction is still unclear, prompting existing customers to consider other SQL ETL tools. Additionally, the users have reported that Stitch’s UI is not as user-friendly when compared to other similar tools. Integrate.io Integrate.io is a cloud-based data integration tool offering multiple solutions including ETL, reverse ETL, and API management. Like all the MS SQL ETL tools discussed in this list, Integrate.io also features a visual, drag-and-drop based UI to set up and build data pipelines. Integrate.io users report that the error messages are not intuitive or self-explanatory, making it difficult to understand the actual problem and, consequently, troubleshoot it. Additionally, it mostly works with cloud-based data sources, which means users cannot work with local files (excel or csv) stored on their local system. Go from low-code to 100% no-code with LIKE.TG Looking for a hassle-free yet robust ETL solution with automation and AI capabilities? Sign up for a free demo and see how LIKE.TG simplifies and accelerates data integration. See it in action Choosing the Right SQL ETL Tool Given the widespread availability of different tools and platforms to ETL data to and from SQL Server, choosing the best can feel like navigating a sea of options. The key is to find a solution that best fits the company’s most immediate and long-term requirements. As such, one must ask the right questions. For example: What are the specific needs and the nature of data workflows? Are robust, built-in transformations a must-have or a nice-to-have? Overall, how tech-savvy is the data team? Are the tool’s ease-of-use and flexibility important factors? Is scalability going to be a prohibiting factor in the future? The fact that more and more business users, or citizen integrators, are taking charge in the data integration landscape, the “best” SQL ETL tool will ultimately be the one that: Caters to the business use case Is economical with transparent pricing Empowers business users with an intuitive UI Backed by a proactive customer support team Wrap Up Leveraging data integration tools instead of writing ETL SQL scripts to integrate data into a SQL Server database offers multiple benefits. First, they provide a visual and intuitive interface that simplifies the overall ETL process, allowing users to design and manage data workflows through a visual representation of the integration pipeline. Second, SQL Server ETL tools typically provide advanced functionalities such as data validation, error handling, and job scheduling. Finally, these tools often support a variety of data sources and formats which further facilitates seamless integration of diverse data sets into the SQL Server database. Do you have a unique use case and are looking for a simple yet powerful SQL ETL solution? Get in touch with one of our solutions experts today. ETL Data to and from SQL Server at Lightning Speeds Whether you want to ETL data into or from SQL Server database, LIKE.TG offers a truly no-code approach to build powerful data pipelines effortlessly. Try for free today. Download Trial
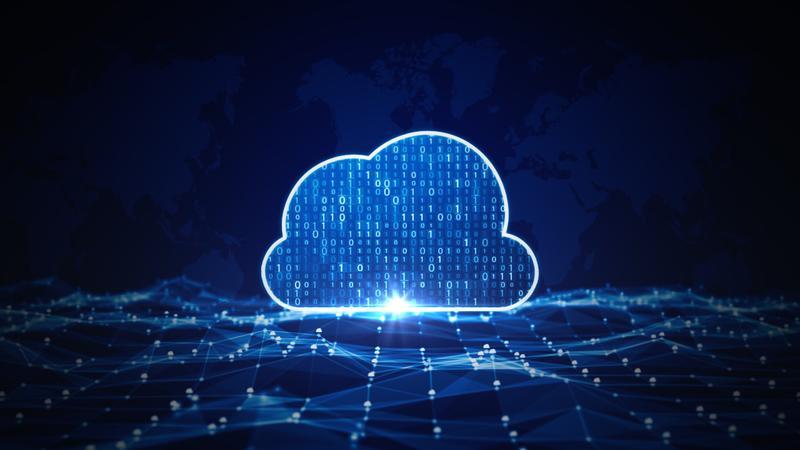
Your Complete Guide to Form Processing
The global data extraction market is predicted to reach a value of $4.90 billion by 2027, boasting a significant CAGR of 11.8%. This growth is largely due to the crucial role played by Form Processing, a technology that has emerged as a fundamental element in the efficient extraction and processing of valuable insights from both structured and unstructured data. What is Form Processing? Form processing refers to the systematic method of extracting, organizing, and storing data from completed forms. It’s an essential process for businesses and organizations, allowing them to collect valuable information and make informed decisions. Companies receive hundreds or even thousands of forms daily. Without a streamlined process in place, handling this influx of data can lead to errors, delays, and inefficiencies. Form processing eliminates these problems by automating the data extraction and storage, ensuring quick and accurate results. Key Components of Form Processing Form processing consists of three major components: 1. Data Capture: This process captures data entered on forms, including text, numbers, and dates. Data capture is a critical step in form processing as it ensures that all the relevant information provided by the form filler is accurately recorded. This process can be achieved through various methods, such as manually extracting data or using optical character recognition (OCR) to extract text from scanned forms. Form processing enables businesses to gather comprehensive and diverse information by capturing a wide range of data types. 2. Data Entry: Data entry writes the captured data into a structured destination, such as a database or an Excel file, that can be easily accessed and analyzed. Data entry operators can perform this process manually, or the process can also be automated using software tools. Manual data entry requires careful attention to detail to ensure accuracy, while automated data entry can significantly reduce the time and effort required. Regardless of the method used, data entry lays the foundation for subsequent data analysis and decision-making. 3. Data Validation: This step ensures that the entered data is accurate, complete, and within specified parameters. It helps identify errors or missing information, minimizing the chances of inaccuracies in data. Data validation ensures the integrity and reliability of the collected data. This process involves performing checks on the entered data to verify its accuracy and consistency. For example, a user can validate a phone number field to ensure it follows the correct format or check the range of values entered in a numerical field. By validating the data, businesses can identify and correct errors or inconsistencies, ensuring that the information is reliable and trustworthy. Diverse Applications of Form Processing in Various Industries Form processing is used in a variety of different scenarios across different industries. Some common examples include: Online Registration Forms: Educational institutions, conferences, and events often use online registration forms to collect participant information. Form processing can automate data extraction like names, addresses, and contact details. Job Application Forms: Companies receive numerous job applications with standardized forms. Form processing can automate the extraction of candidate details such as education history, work experience, and skills from these applications. Insurance Claims: Insurance companies deal with a large volume of claim forms. Form processing can extract relevant information like policy details, incident descriptions, and supporting documentation, streamlining the claims processing workflow. Surveys and Feedback Forms: Businesses and research organizations often use surveys and feedback forms to gather opinions and insights. Form processing can extract and analyze responses, making it easier to understand trends and patterns. Bank Loan Applications: Banks and financial institutions receive loan applications with standardized forms. Form processing can automate the extraction of financial details, employment history, and other relevant information for quick and accurate evaluation. Healthcare Forms: Patient intake forms, medical history forms, and insurance claims in healthcare involve a lot of unstructured data. Form processing extracts patient details, medical history, and insurance information to improve the efficiency of healthcare processes. Tax Forms: Government agencies and tax authorities process large volumes of tax forms to extract income details, deductions, and other relevant information to ensure accurate tax assessment. Manual vs. Automated Form Processing There are two main types of form processing: manual form processing and automated form processing. Manual Form Processing Traditionally, form processing was done manually, with employees reading and transcribing data from forms into tabular formats. This process was time-consuming, prone to errors, and lacked scalability. However, manual form processing remains applicable in specific scenarios, such as when processing handwritten or complex forms that demand human judgment. Complex forms involving subjective decision-making or human expertise may also require manual processing. Automated Form Processing Automated form processing, on the other hand, involves leveraging technology to extract data from electronic forms automatically. This approach has become popular due to its efficiency, accuracy, and scalability. Automated form processing offers several advantages over manual processing. For starters, it eliminates the need for human intervention in routine form processing, freeing up valuable time and resources. Also, it minimizes the chances of errors that can occur during manual data entry, ensuring data integrity. In addition, automated form processing allows for seamless integration with existing systems, enabling efficient data management. Businesses can customize automated form processing to suit their specific needs. For instance, they can program it to extract specific data fields, validate the information, and perform various data manipulation tasks. This level of automation saves time and enhances data accuracy and consistency. Although, forms with complex layouts, non-standard formats, or unusual data structures may require additional customization or human intervention to ensure accurate data extraction. Comparison Table: Manual vs Automated Form Processing Criteria Manual Form Processing Automated Form Processing Speed Slow, as it relies on human data entry. Faster, as it utilizes automated tools and algorithms. Accuracy Prone to errors due to human mistakes. More accurate, with reduced chances of human error. Cost Expensive due to labor costs. Initial setup costs are higher, but ongoing operational costs are lower. Efficiency Less efficient as it depends on human speed and availability. More efficient, providing quick and consistent results. Scalability Limited scalability due to reliance on human resources. Highly scalable, able to process large volumes of forms with ease. Flexibility Limited flexibility, especially for handling complex forms. More flexible, with the ability to adapt to various form structures. Data Validation Relies on manual validation, which may lead to oversight. Automated validation ensures data accuracy and compliance. Integration with Systems May require manual data entry into other systems. Can be seamlessly integrated with other systems for data transfer. Security Potential security risks due to manual handling of sensitive information. Can be more secure with encryption and access controls in place. Human Resources Requires a significant workforce for data entry and validation. Requires minimal human intervention, allowing employees to focus on higher-value tasks. Error Handling Relies on human detection and correction of errors. Automated error detection and handling can be built into the system. What Makes Form Processing Challenging? Variability in Formats: Forms come in various formats and layouts. They can be in a tabular or narrative layout. The fields may be organized differently, and the structure of the form can vary. Inconsistent Data Quality: Forms may have missing or incomplete information, illegible text in case of scanned forms, or errors. Ensuring data accuracy and completeness becomes a challenge when dealing with inconsistent data quality. Sensitive Information Handling: Many forms contain sensitive or personally identifiable information. Ensuring the security and privacy of the data during the processing stage is a significant challenge. Scalability: Handling large volumes of forms efficiently and in a timely manner is crucial for many applications. Ensuring that the form processing system can scale to meet increasing demands is a significant challenge. The Evolution of Form Processing Solutions As technology continues to advance, the integration of AI and machine learning in form processing has become more prevalent. These technologies enable intelligent form recognition, automated data extraction, and advanced data analysis. Advanced optical character recognition (OCR) technology is one of the prominent technologies, allowing these form-processing systems to accurately extract data from scanned documents. The integration of AI extends beyond OCR, providing the capability to understand and process unstructured data within forms automatically. Real-time data extraction and AI’s adaptability to handle complex forms mark the forefront of contemporary form processing solutions. The emphasis on AI not only enhances accuracy and efficiency but also aligns with broader industry trends, contributing to the ongoing digital transformation by reducing manual labor and optimizing workflow processes. LIKE.TG for All Your Form Processing Needs LIKE.TG, equipped with cutting-edge AI technology, stands out as a powerful solution for all your form processing needs, delivering a specialized toolkit tailored to extract meaningful data seamlessly from various form types. Whether dealing with customer surveys, application forms, or compliance documents, LIKE.TG excels in transforming unstructured form data into organized, usable information through advanced AI algorithms. Its intuitive interface simplifies extraction, allowing users to capture and validate data fields effortlessly. With support for diverse form formats, including PDFs and scanned documents, LIKE.TG ensures adaptability to different layouts. Beyond extraction, the solution also offers robust data cleansing and validation features, ensuring the accuracy and reliability of the processed information. For organizations seeking an efficient, user-friendly tool, LIKE.TG is the answer. Experience its power with a 14-day free trial and a personalized demo with our experts—unlock the potential of AI-driven form processing today!
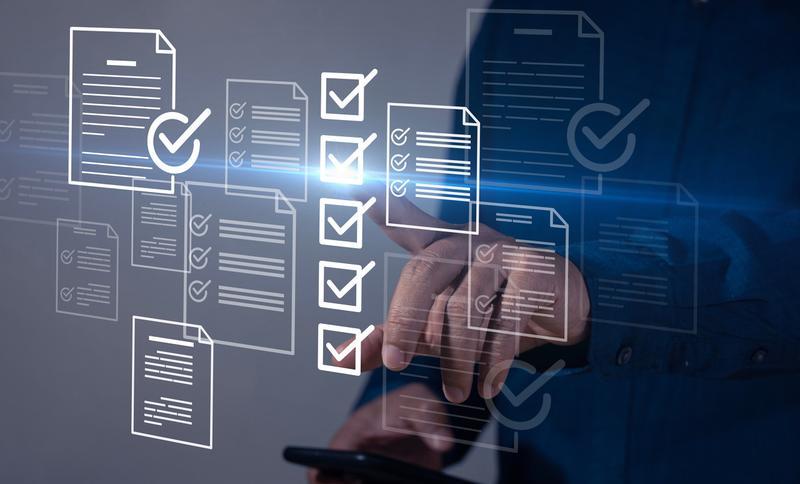
What is Zero ETL? Components, Benefits & How Does it Work
Zero ETL is a data integration technique where data is transferred from source to destination without the need for a traditional ETL (Extract, Transform, Load) process. Zero–ETL simplifies data accessibility by enabling seamless querying across disparate data sources without necessitating extensive data movement. Zero-ETL is a relatively newer approach to data integration and management—a move away from the traditional ETL paradigm. In a zero-ETL architecture, data integration take place in real-time or near-real-time, minimizing the latency between data generation and its availability for analysis. With zero-ETL, the idea is to carry out transformation during the data querying process. It eliminates time-consuming steps and allows businesses to analyze and query data directly from the source in real time. This process also eradicates the need for intermediate data storage in a staging area. So, let’s dig further and see how zero-ETL works and how it can be beneficial in certain data management use cases. How does Zero-ETL Work? Unlike conventional ETL that revolves around time-consuming data transformation before loading it into the target repository, zero-ETL takes a different approach as it eliminates the reliance on scheduled batch processing in ETL. It uses technologies like data lakes and schema-on-read (a data storage and processing approach where data is stored in its raw format without needing upfront schema definition) that allow organizations to extract insights from unstructured data sources instantly. Moreover, zero-ETL also employs data virtualization and federation techniques to provide a unified view without physically moving or transforming it. All this significantly reduces the time spent on data preparation and allows for immediate insights from raw data. It’s important to consider the key components of zero-ETL to understand how it works. Keep in mind that zero-ETL is not a technology but rather a philosophy and approach to data integration. Therefore, the term “components of zero-ETL” refers to key elements and strategies that contribute to achieving its goals. So, let’s explore them in detail: Zero ETL Components Real-Time Data Replication It is a fundamental component of zero-ETL. Organizations use real-time data integration technologies to facilitate the continuous flow of data from source systems to destination repositories. This differs from conventional batch processing methods of ETL, which operate on predefined schedules. Adopting real-time data streaming technologies can also minimize the latency associated with data processing. It also further reduces the dependence on preprocessing and staging of data before analysis. Data Lakes and Object Storage Data Lakes represent another vital component of the zero-ETL framework. They store raw, unstructured, and untransformed data, including diverse types like videos, texts, images, and more. This makes the data immediately available for analysis. On the other hand, object storage systems allow organizations to directly analyze data stored in its original format and eradicate the need for upfront data transformation. It provides an efficient and scalable way to store and retrieve data as discrete objects, each having the data itself along with associated metadata. Data Management Technologies Data federation and data virtualization are important data management technologies that facilitate the functionality of zero-ETL. They both involve creating a virtual layer that integrates data from diverse sources and provides a unified and abstract view for easier access and querying. All this can be done without the need for physically moving data or transforming it. Skilled Employees Without a skilled team, managing the tools and technologies associated with zero-ETL will be difficult. Therefore, analysts and data scientists require proper training to navigate ETL tools and be able to understand data integration pipelines. Proficiency in SQL is also essential for querying and manipulating data stored in databases or warehouses. All of this is necessary to consider before employing staff on zero-ETL strategy. Data Source Diversity Another component of zero-ETL is the accommodation and integration of a wide range of data sources. Organizations aim to handle diverse data sources without the need for upfront standardization. Data source diversity includes different kinds of information, including structured, semi-structured, and unstructured data. Zero ETL and Other Non-ETL Data Integration Techniques Like other non-ETL techniques, Zero ETL prioritizes minimal data movement and transformations for real-time data access and simplicity. However, it’s distinct from other non-ETL methods given their specific approach, level of data processing, and complexity. Let’s discuss how zero-ETL compares with some of the data integration techniques that don’t primarily involve ETL. ELT (Extract, Load, Transform): Similarities: Both minimize data movement by transforming data after loading. Differences: Staging area: ELT typically uses a staging area for data transformation in the target database, while zero-ETL aims for direct source access. Complexity: ELT handles complex transformations efficiently in the staging area, while zero-ETL does not involve any transformation. Latency: ELT introduces some latency due to staging, while zero-ETL prioritizes real-time access. CDC (Change Data Capture): Complementary: CDC can be used with zero-ETL to capture real-time changes efficiently. Differences: Scope: While CDC can be part of an ETL pipeline, it’s not inherently ETL as CDC only captures changes in data. Zero-ETL, on the other hand, aims to eliminate all data movement and transformations. Initial data: CDC doesn’t handle initial data loads, requiring additional solutions in a zero-ETL context. Data Virtualization: Similarities: Both avoid the physical movement of data, offering virtual access to data. Differences: Performance: Zero-ETL might offer better performance with direct source access compared to virtualization overhead. Control: Virtualization grants granular control over data access, while zero-ETL relies on source system permissions. Transformations: May include basic transformations, while zero-ETL minimizes them. API Integrations: Complementary: APIs can be used within zero-ETL for specific data access needs. Differences: Scope: APIs are targeted access points, while zero-ETL aims for broader data integration. Complexity: Building and maintaining APIs might be more complex than zero-ETL solutions. Security: APIs require robust security measures compared to zero-ETL’s reliance on source system controls. Data Federation: Similarities: Both enable querying data across multiple sources without centralizing it. Differences: Control: Zero-ETL focuses on simplicity, while federation offers more granular control over data access and transformations. Complexity: Setting up and managing federation can be more complex than zero-ETL solutions. Performance: Depending on the implementation, the data federation process can introduce performance overhead compared to zero-ETL’s direct access. Zero ETL Benefits Zero ETL has many benefits that can enhance data integration processes and analytics. Three advantages of zero-ETL are: Speed Since there is no data transformation or manipulation involved in the zero-ETL approach, this process tends to be faster than other conventional processes like ETL. In zero-ETL, the emphasis is on direct data movement that enables swift data migrations. Speedy data transfer proves crucial when real-time data delivery is needed, particularly for prompt decision-making. Streamlined Data Analytics With zero-ETL, it’s possible to access and analyze data as it flows. Since there is no need for batch transformations and extensive preprocessing, there is reduced latency. Zero ETL ensures the streamlining of data analytics, enabling timely insights and enhancing the overall experience. Real-time Insights Zero ETL enables organizations to access and analyze data as it is generated. The data becomes available in real time provided there’s that extensive transformations are not required. For instance, cybersecurity firms can adopt zero-ETL for real-time threat detection. Since conventional ETL processes introduce delays in processing and analyzing security event logs, firms may experience delays in identifying potential threats. But with zero-ETL, firms can instantly analyze log data as it’s generated and proactively address cybersecurity issues. Zero ETL Use Cases ETL is an indispensable approach to data integration, especially when complex data transformation is a requirement. However, there are some scenarios where an organization would be much better off with zero-ETL. Some of these use cases are: Quick Replication It refers to the rapid duplication of data from a source to a target system, keeping the target continuously synchronized with the changes in source data. Zero-ETL is well-suited to this scenario as it focuses on real-time data processing and schema-on-read principles. The schema-on-read principles enable on-the-fly interpretation and structuring of data during analysis, thus aligning with the need for quick updates without extensive preprocessing. Real Time Monitoring Altering Zero-ETL proves to be highly effective in cases where continuous monitoring and alerting are required, such as fraud detection applications and network monitoring. Since it streams data in real-time from different real time sources, zero-ETL allows for instant identification of deviations from expected patterns or suspicious activities. The swift and responsive nature of zero-ETL is advantageous in cases like these where proactive response and timely detection are important. Customer Behavior Analysis Customer behavior analysis is another situation where zero-ETL proves more effective than traditional ETL processes. This is due to zero-ETL’s capability of enabling immediate insights without delays. The real-time data streaming feature of zero-ETL ensures that engagement data, customer interactions, and transactions are available for analysis as soon as they occur. This allows businesses to respond instantly to emerging patterns, personalized recommendations, and customer support interactions accordingly. Zero ETL does not Replace ETL Zero ETL’s popularity stems from the belief that it is a futuristic approach or even an alternative to traditional data integration processes. The traditional ETL processes have been used in organizations for decades, setting the foundation for many deeper analytics tasks. Since zero-ETL is known for its exceptional speed considering its direct data transfer approach, it still does not completely replace ETL. Therefore, it’s important to discuss the prevailing misconceptions concerning zero-ETL. Here are some reasons why zero-ETL cannot replace ETL: Lack of Integration Outside the Ecosystem Similar to Snowflake with its Unistore, Amazon has been pushing its zero-ETL approach quite a lot lately. For starters, consider its announcement about launching AWS zero-ETL integrations for Amazon Aurora PostgreSQL, Amazon RDS, and Amazon DynamoDB with Amazon Redshift. Accordingly, other players including Google and Microsoft have been following suit. However, the important thing to note here is that organizations will only be able to realize the gains of zero-ETL as long as they stay within the ecosystem. Since zero-ETL is all about transferring data as quickly as possible, integration with systems outside the ecosystem of, say AWS, can be challenging, if not impossible. Unable to Handle Complex Transformations Since the zero-ETL approach depends on in-place processing, it is not ideal for scenarios demanding data transformations or combining data from different sources. Moreover, highly complex data require more development and maintenance resources to maintain zero-ETL solutions. Compared to zero-ETL, traditional ETL is well-suited for complex data transformations and extensive preprocessing. It allows firms and organizations to structure and clean data before leading it into a target repository. Lack of Data Quality Assurance Though zero-ETL is highly responsive and offers an advantage in terms of speed, it may not provide the same level of data quality assurance as ETL. Quality checks must be integrated into the analytics process, introducing the challenge of maintaining data integrity during on-the-fly transformations. This shift in timing and nature of quality checks tells about the need for careful consideration. Conventional ETL processes include comprehensive data quality checks, like competence consistency, data accuracy, etc., and transformations during the staging phase. This ensures that only accurate data is loaded into the target. Cost Effectiveness and Batch Processing Batch processing is often more cost-effective using ETL and it is more practical for handling large volumes of data that can be processed periodically. This is true particularly when real-time or near-real-time data processing is not a restrictive requirement and delays don’t affect important decisions. Real-time data streaming in zero-ETL incurs higher costs for quick data processing. This makes it less cost-effective in cases where batch processing is ideal for business needs and resource constraints. Conclusion Zero ETL can be a viable approach in certain cases due to its swift response and direct transfers. However, it also requires considerable investment in data management technologies and an expert staff to derive actionable insights efficiently. Both traditional ETL and Zero ETL have their merits and the preference depends on specific use and requirements.When managing data at enterprise level traditional ETL offers a centralised system to not only integrate data from diverse sources but also transform it and improve its quality. For businesses prioritizing performance and a resource efficient approach, LIKE.TG’s merges as a reliable solution for your traditional ETL needs. Streamline your data handling now with LIKE.TG! Take the Next Step Towards Streamlined Data Operations For efficient data management, LIKE.TG is your go-to choice. Simplify your processes and get reliable results with LIKE.TG’s solutions! Signup Now!
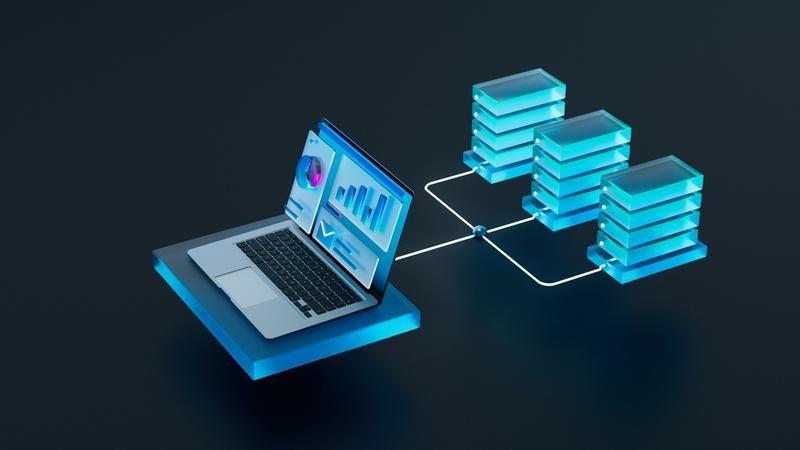
A Comprehensive Guide to SQL Server Replication: Setup, Types, and Components
SQL Server replication is a specialized form of data replication that plays a crucial role in ensuring the seamless transfer and synchronization of data across multiple instances of Microsoft SQL Server databases. Data replication, in the broader sense, is a process in which data is copied from one database or storage system to another, ensuring that the same information is consistently available in multiple locations. The primary purpose of data replication is to enhance data availability, reliability, and fault tolerance. By maintaining multiple copies of data across different servers or locations, organizations can mitigate the risk of data loss due to hardware failures, disasters, or other unforeseen events. As a vital element of data management strategies, data replication aids essential functions like disaster recovery, load balancing, and distributed computing environments. It ensures data uniformity and synchronization across all systems, meaning that all copies of the data are kept up-to-date and consistent, thereby enhancing decision-making and operational efficiency. For instance, a company might replicate its data across servers in different locations to ensure that employees in all locations have access to the same, most recent data. What is SQL Server Replication? SQL Server Replication is a feature provided by Microsoft SQL Server that enables the transfer and synchronization of data and database objects from one database to another. While it shares the fundamental concept of data replication, SQL Server Replication is specifically designed to work within the SQL Server environment, providing a robust, flexible, and efficient solution for managing data distribution and synchronization. SQL Server Replication is highly configurable, allowing it to be tailored to meet specific business requirements. It provides a reliable and efficient mechanism for managing data distribution, making it essential for businesses that rely on SQL Server for data management. Types of SQL Server Replication SQL Server Replication is categorized into four main types. Each of these serve different needs and scenarios. The choice of among them depends on the specific requirements of the data environment. They include: 1. Snapshot Replication Snapshot Replication creates a full copy or ‘snapshot’ of the entire database or a portion of it, which is then transferred to the subscriber. When changes made to data are infrequent, this is the most effective approach. It is a straightforward SQL Server replication method as it simply involves copying data from one database to another. However, it can be resource-intensive for large databases due to the volume of data transferred. Think of a university or college that updates its course catalog once every semester. The university can use snapshot peplication to distribute the updated catalog to its various departments. Since the catalog is updated infrequently, it’s practical to copy the entire catalog each time, ensuring that all departments have the same course information. 2. Transactional Replication Transactional Replication begins with an initial snapshot of data. Following this, only the transactions or changes made to the publisher database are sent to the subscriber. The goal is to ensure that the publisher and subscriber databases are synchronized in near real-time. In scenarios where data changes are frequent and high throughput is required, transactional replication is ideal. For example, an online ticket booking system where ticket availability needs to be updated in real time can utilize transactional replication to duplicate SQL Server data. As tickets are booked or canceled, these changes are replicated across all servers, ensuring that all users see the most current ticket availability. 3. Merge Replication Merge Replication is a more sophisticated type of replication that allows changes to be made at both the publisher and subscriber databases. After the first snapshot of data is created and sent to the subscriber, changes made at both ends are tracked and then merged together. This type of replication is useful in distributed server environments where connectivity may not be consistent. A retail chain with multiple stores, each having its own database, can use merge replication with SQL Server to manage its inventory. If a product is sold or restocked at one store, this change can be made in the local database and then merged with the databases of the other stores. Consequently, all stores have accurate and up-to-date inventory information, improving the efficiency of inventory management across the retail chain. 4. Peer-to-Peer Replication Peer-to-peer replication is a type of transactional replication that allows multiple servers (peers) to hold identical copies of data. In this model, each server acts both as a publisher and a subscriber, meaning any change made in any server is replicated to all other servers. This ensures that all servers contain the most recent data, providing a system that is highly available and fault-tolerant. For example, consider a multinational corporation with offices in New York, London, and Tokyo, each having its own server. The corporation uses peer-to-peer replication to ensure that any update made in any office (like updating client information in the New York office) is immediately replicated to the servers in the other offices. Major Components of SQL Server Replication SQL Server Replication comprises several key components that work together to facilitate the replication process. These components include: 1. Publisher The Publisher in SQL Server Replication refers to the source database where the original data is stored. It’s the database that is being replicated. The Publisher can have multiple publications, each containing a logically related set of objects and data that are replicated as a unit. The Publisher is responsible for tracking changes in the data and transmitting these changes to the Distributor. 2. Distributor The Distributor is a crucial component of SQL Server Replication. It is a database that stores metadata and history data for all types of replication and transactions for transactional replication. The Distributor can be located on the same server as the Publisher, known as a local Distributor, or on a different server, known as a remote Distributor. The Distributor’s primary function is to distribute the data changes from the Publisher to the Subscribers. 3. Subscriber The Subscriber is the destination database. It is where the replicated data from the Publisher is received and stored. A Subscriber can subscribe to multiple publications from various Publishers. The Subscriber applies the changes received from the Publisher to its local data. 4. Publication A Publication is a collection of database objects and data from a Publisher database that is made available to Subscribers. The content of a publication is typically defined by one or more articles. Publications are created at the Publisher and then propagated to the Subscribers by the Distributor. 5. Article An Article is a specific database object that is included in a publication. It could be a table, view, stored procedure, or function. A publication can consist of one or more articles. Each article represents a unit of data that can be replicated independently of other articles. 6. Subscription A Subscription in SQL Server Replication is a request by a Subscriber to receive a publication. The Subscription defines where the publication’s data and database objects are sent. Subscriptions can be either push, where updates are automatically sent from the Publisher, or pull, where updates are requested by the Subscriber. 7. Agents Agents are specialized processes or services in SQL Server Replication that are responsible for moving data between the Publisher and Subscribers. The three main types of agents are the Snapshot Agent, which creates snapshots of data and schema; the Log Reader Agent, which monitors the transaction log; and the Distribution Agent, which moves replicated data from the distribution database to Subscribers. These agents work in unison to ensure the smooth and timely transfer of data. These components interact with each other to ensure that data is accurately replicated from the Publisher to the Subscriber(s), maintaining data consistency and integrity across the databases. How To Set Up SQL Server Replication To demonstrate how an organization can set up SQL Server Replication, let’s consider a use case: A retailer sells via two channels: online and in-store. The retailer maintains distinct database tables, namely Orders_Online and Orders_Store, each residing in separate SQL Server databases on different machines (servers). Crucially, the replication process ensures that orders placed through one channel are mirrored in the other, creating a synchronized data ecosystem. Traditionally, organizations would need to navigate the complexities of configuring SQL Server components to achieve this replication. However, LIKE.TG simplifies the entire task by providing an intuitive, user-friendly solution. By eliminating the intricacies associated with manual installation and configuration, LIKE.TG streamlines the replication process. Here’s a closer look at how LIKE.TG accomplishes this: Objective: Achieve synchronization or replication of data between the Orders_Online and Orders_Store tables. Step 1: Replication from Orders_Online to Orders_Store a. Begin by specifying the necessary information for connecting to the Shop_Online database using SQL Server. b. Select the ‘Order’s’ table and enable Change Data Capture (CDC) by choosing the “Incremental Load Based on Audit Field” option. This involves specifying a designated audit field, typically Order ID, to facilitate tracking changes. c. Configure the Database Diff Processor to detect variations between the source (Orders_Online) and destination (Orders_Store) and write them onto the Orders_Store database table. d. Define a structured mapping that clearly outlines how the columns in the Orders_Online table correspond to those in the Orders_Store table. e. Repeat steps a-d for the Orders_Store table, ensuring bidirectional synchronization. Step 2: Establish Continuous Two-Way Replication The first time the dataflow is run, any differences in the two tables will be written to both tables. On each subsequent run, CDC on the source database, using the audit field as Order ID, will pick up any new orders present in the source table since the last run and write them to the destination if they are not already present there. To set up continuous automated runs of the above dataflow, we can schedule it as a job using the Job Scheduler. Here, the job is configured to run continuously on the LIKE.TG server, with a minimum wait time of 5 seconds and a maximum of 30 seconds before a rerun. This setup ensures near real-time synchronization between the two database tables. Results: Once the job is scheduled, it is run continuously in the background, synchronizing changes between the two tables in near real-time. When a new order is placed in Orders_Online, and When a new order is placed in Orders_Store, These changes are instantly reflected across both databases. Conclusion SQL Server Replication is essential for organizations managing and distributing data across multiple databases. It ensures data consistency, availability, and reliability, which are crucial for informed decision-making and smooth business operations. LIKE.TG is designed to enhance these benefits by simplifying the SQL Server replication process. With its user-friendly interface and advanced features, it reduces the complexity of data replication and ensures that data is always synchronized and readily accessible. Its seamless integration with SQL Server databases and the ability to connect to diverse data sources make it a comprehensive solution for efficient data management across various platforms. Moreover, LIKE.TG’s automated ETL processes and data transformation capabilities simplify the setup and management of replication tasks, allowing for the customization of data during replication. Ready to enhance your SQL Server Replication process? Start your journey by downloading LIKE.TG’s 14-day free trial today. Experience Hassle-Free SQL Server Replication LIKE.TG's user-friendly, drag-and-drop interface makes the replication process easy and straightforward, even for non-technical users. Plus, with customizable features, LIKE.TG can meet your specific business needs, making it the ideal solution for SQL Server replication. Download Free Trial
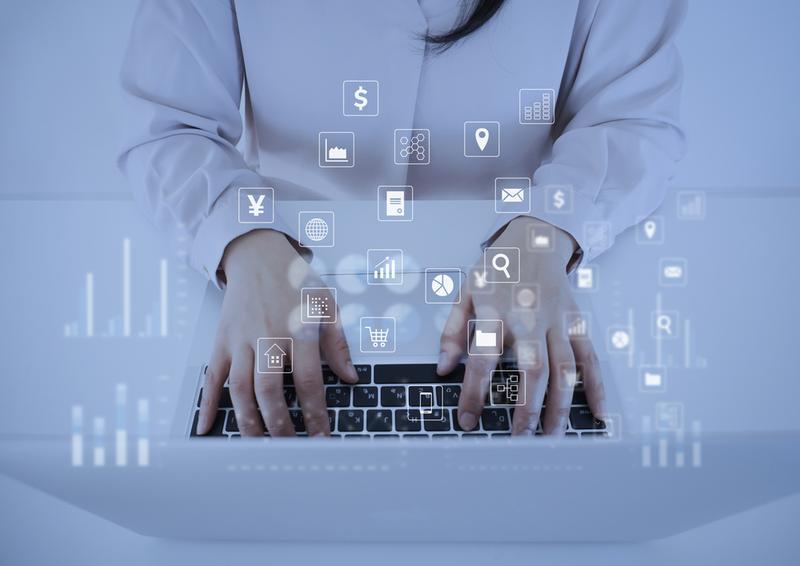
Automated Form Processing: A Practical Guide
Paper-based forms are slowly being replaced by their digital counterparts, but filling out forms is still a big part of doing business. It’s typically the first step in a company’s data collection and facilitates multiple business functions. The ubiquitous nature of forms in today’s business environment necessitates the implementation of automated form processing. Manually collecting, processing, and using forms from various sources is resource-intensive and prone to human errors—issues that automated form solutions can resolve easily. With the increasing use of automation to save time and boost efficiency, a growing number of enterprises are realizing the value of automating their form-processing tasks and how it can improve their data entry and management. This blog takes an in-depth look at automated form processing, explores its benefits, and discusses how LIKE.TG can be the right solution for you. What is Automated Form Processing and How It Works Automated form processing uses software to streamline how your organization handles its forms and documents. By using dedicated applications, your business can eliminate the time and manual effort spent on performing associated tasks—such as extraction, validation, and data entry. The automation process follows these steps: Document Capture and Upload Electronic document capture ensures form information is quickly uploaded where you want it. Instead of manually entering information from filled-out forms into your system, you can use electronic document capture tools to scan hard copies or ingest electronic documents from a specified source. Data Extraction Data extraction software obtains pertinent information from the form or document using techniques such as PDF document parsing, data querying, reusable pattern-based extraction templates, AI-based algorithms, natural language processing (NLP) and Optical Character Recognition (OCR). Data Validation This extracted data will be validated using your specified data quality rules or other conditions. Data Integration and Analysis Data extracted from your forms and documents is integrated with other datasets. You can then use this compiled information for record keeping, analysis, report generation, and obtaining insights for more informed decision-making. Workflow Automation Based on your requirements, you can configure automated workflows to route the forms for further processing, review, or approval. These workflows will seamlessly move the form to the next specified step based on the outcome of the previous process. Benefits of Automated Form Processing These are some of the most substantial benefits of automated form processing: Greater Efficiency: Automation results in a significant boost in your efficiency. Form processing jobs are completed without manual intervention or effort, and your personnel’s focus can be redirected to tasks that benefit from it. Increased Accuracy: Automated processes minimize the possibility of human errors affecting data quality. As a result, you can have more confidence in your extracted data and its accuracy. Time Savings: Quicker processing through automation decreases the time each form takes to go from data to actionable information. On its own, a form is just a compilation of text and figures. However, it becomes a valuable part of a larger picture once extracted and integrated with other data. The sooner you have the insights you need to help with your decision-making, the faster you can determine the next steps you need to take. More Streamlined Workflows: Automation removes the back-and-forth and redundant steps from your organization’s form management. The result is a smoother and more streamlined workflow. Cost Reduction: Automated form processing lowers the costs associated with form management by doing away with manual labor and decreasing the chances of mistakes—and the steps involved in rectifying them. Industries That Can Leverage Automated Form Processing Any industry that uses forms can benefit from automated form processing. W2 form automation is a great example applicable in every sector, big or small. You can easily maintain accurate records of your employees’ critical financial information by automating W2 form data extraction. As a result, it becomes more convenient to review and analyze their salaries, tips, wages, additional compensation, and the taxes withheld from their salaries. [Source: 2024 Form W-2 (irs.gov)] Automated form processing also offers specific advantages in the following domains: Education Automated form processing can help the education industry in a variety of ways, some of which include: A Simplified Admissions Process: Admission applications are packed with documents such as personal information, transcripts, essays, personal statements, and the like. Automated form processing can separate items like essays and personal statements for manual review and automate the extraction and compilation of other data. This can enable educational institutions to handle more applications with less effort. More Accurate Records: Automated validation and data entry processes allow educational institutions to maintain accurate student records. This can reduce errors in administrative tasks and facilitate error-free record-keeping. Easier Compliance: Educational institutions can stay compliant with various regulatory requirements more easily with automated form processing. They can generate more up-to-date reports for audits, accreditation, and other objectives. [Source: transcript-sample-scaled.jpg (1978×2560) (iahe.net)] Healthcare In the healthcare sector, automated form processing assists with various aspects of the patient experience such as: Rapid Patient Registration: Automated form processing accelerates patient registration protocols in medical facilities. Patients can be triaged more quickly in emergencies, experience shorter wait times in regular visits, and start receiving necessary treatment sooner with less red tape in the way. [Source: SOS Free Medical and Dental Clinic (squarespace.com)] Medical Paperwork: Through automated form processing, medical facilities can maintain accurate health records and up-to-date patient histories for more effective care. They can also ensure that consent forms are readily accessible for ethical and legal compliance. On the administrative side, form-heavy processes such as billing, invoicing, and insurance claims processing can all be improved through automation. Data Collection for Clinical Research: Clinical researchers can utilize automated form processing to collect research data more easily and ensure its accuracy and compliance with the applicable study protocols. Government In the government sector, automated form processing streamlines interactions between government agencies and citizens for better performance and outcomes. Here are a few examples: Citizen Service Provision: Automated form processing shortens the processing time required for common services such as license renewals, benefits claims, and permit applications. [Source: VEHICLE REGISTRATION/TITLE APPLICATION (ny.gov)] Quicker Response Times: Faster form processing enables government agencies to respond more quickly to citizen complaints, applications, requests, and inquiries. Prompt service delivery can boost citizens’ satisfaction and improve how they perceive an agency’s performance. Auditable and Transparent Processes: Automation promotes transparency in government processes, and the digital trail that automated systems create during their operations makes them easier to audit. This ensures accountability and regulatory compliance and increases public confidence in a government body. Finance Automated form processing also serves several purposes in the finance sector, including: Expedited Loan Processing: Business and consumer loans form a major component of a financial institution’s operations. Automated form processing accelerates loan application and approval procedures, allowing loan providers to assess and approve requests and disburse funds quickly. [Source: Tiers 3, 4, 5 6 Loan Application for Members Covered by Articles 14, 15 or 22 (RS5025-A) (ny.gov)] Fraud Prevention: The finance sector is vulnerable to fraud and other instances of white-collar crime. Automated analysis and validation of the information included in financial forms can help mitigate the chances of fraud more easily. Compliance Management: Automated form processing aids financial institutions in adhering to financial regulations and other compliance requirements, minimizing their exposure to fines, penalties, and legal consequences. How to Choose an Automated Form Processing Platform Ready to choose an automated form processing platform for your organization? Here are a few factors to keep in mind: Data Extraction Accuracy This is a platform’s ability to correctly extract data from different types of forms. Verify the accuracy of its extraction processes and assess how precisely it processes data. Seamless Integration Easy integration with the systems, tools, databases, and applications you currently use. High compatibility ensures you can have the platform up and running with minimal disruptions to your workflow. Customizability A customizable platform can easily accommodate your current requirements. You can also modify it to keep up with your organization’s evolving demands. Security and Compliance Features Look for security features such as encryption in your automated form processing platform and ensure its adherence to all applicable data protection and privacy regulations. Your platform may need to comply with federal and state data privacy laws, depending on which state you are based in. There can also be additional industry-specific regulations to consider. User-Friendliness An intuitive and user-friendly interface will reduce training requirements and make the platform more accessible for administrators, end users, and non-technical teams alike. Scalability Scalability ensures a platform’s long-term usage and viability for your organization. As your business expands and your form processing requirements grow, your platform needs to be able to keep up easily. Customer Support Your vendor’s customer support staff will significantly affect your user experience, as you will work closely with them to integrate the platform into your processes. Check for seamless onboarding processes and comprehensive tutorials that can prepare your teams for all aspects of usage. However, there’s more to a reliable vendor than good training and comprehensive documentation. Verify the accessibility of their customer support infrastructure. When issues arise, you should get the help you need to sort them out quickly. Cost and ROI The costs of acquiring a new platform are not limited to its purchase. Consider any additional applicable fees, the pricing structure, and setup and training costs. Additionally, factor in the potential return on investment you expect to see in resource conservation and time savings. The LIKE.TG Advantage LIKE.TG combines AI-powered functionality with a user-friendly, no-code interface and an enterprise-grade ETL engine. The result? A powerful, scalable end-to-end document processing solution that readily ingests data from structured, semi-structured, and unstructured data sources. Fulfilling customer requests 15 times faster? Cutting purchase order processing time from hours to 2 minutes? Processing an invoice in less than a minute when previously it used to take 30 minutes to a couple of hours? It’s possible with LIKE.TG. Automate your data’s extraction, validation, and transformation—then send it to downstream destinations, such as databases, BI tools, and ERP/CRM systems, without manual intervention for analytics, reporting, and other uses. Using LIKE.TG for Automated Form Processing LIKE.TG can help your automated form processing with: Adding a Document: LIKE.TG works with PDF, TXT, DOC, and XLS formats. You can add these documents from email, cloud storage, web services, FTP/SFTP, and other sources. Data Extraction: LIKE.TG allows both manual and AI-based extraction template creation. After a document is added, these extraction templates capture the relevant information. Data Preparation: LIKE.TG’s Data Prep feature lets you preprocess the extracted data. You can cleanse it, transform it based on your requirements, and validate it using your specified rules. Data Export: You can then move the processed data to a destination of your choice, depending on your objectives. Export it to a database for integration, a BI tool for analytics, or a CRM system for compilation. Automation and Scheduling: Once you have configured the document source, processes, and desired destination, use LIKE.TG’s automation and scheduling capabilities to decide these extraction jobs’ frequency. For example, you can set them up to run when there is a new document detected in the system or at a given time of the day. Automate Your Organization's Form Processing with LIKE.TG's Intelligent Data Solutions Whether you're in finance, education, healthcare or government—count on our end-to-end data management platform. Become one of our satisfied clients today! Start Your FREE Trial Today Conclusion Automated form processing can transform form management in a variety of sectors, especially education, healthcare, government, and finance. By choosing the right automated form processing platform for its needs, an organization can save time, minimize delays, mitigate human errors, and redirect its resources to where they’re needed the most. Start using an automated form processing platform designed to grow alongside your operations. Contact us for a quote or start your free trial today!
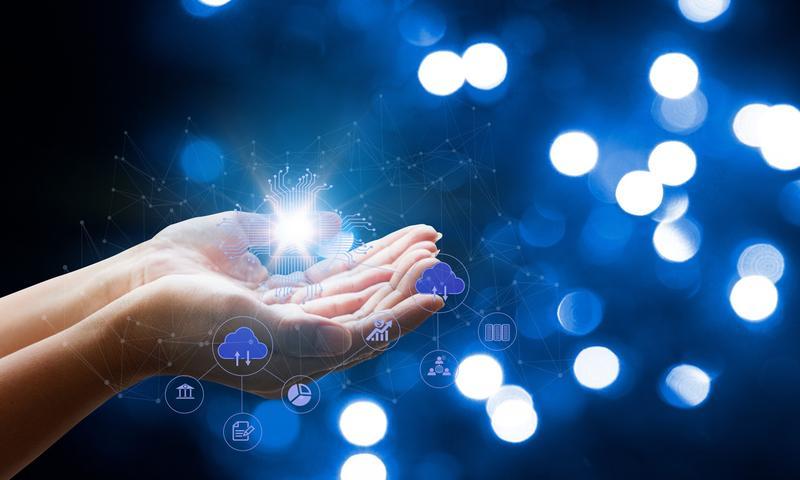
Modernizing Unstructured Data Processing With AI
AI’s integration into data analytics and processing is a natural progression for an industry marked by rapid innovation and growth. The big data analytics market is moving toward an expected valuation of $655 billion in the next five years, and unstructured data processing tools will be responsible for a major chunk of this revenue. With technological advancements and the incorporation of AI, these tools enable organizations to make sense of vast, previously untapped data stores. This blog will discuss how data processing has evolved, examine unstructured data processing, and highlight the role of LIKE.TG’s AI-driven solutions in transforming how businesses handle unstructured data. Unstructured Data and Its Unique Challenges Dedicated unstructured data processing solutions have gained traction recently, but many organizations are still struggling to fully leverage this resource due to its unique nature and features. Unstructured data represents around 80 to 90 percent of all new enterprise data. It comprises a variety of formats, lacks a predefined structure, and is typically complex and heterogeneous. These characteristics make unstructured data unsuitable for generic solutions and standardized data processing methods. Modernizing Unstructured Data Processing AI is being increasingly integrated into data management and processing platforms. It can also solve the most common unstructured data issues. When enterprises leverage AI-driven tools for modernizing their unstructured data processing methods, they benefit in three key ways: Richer Insights: The valuable insights obtained from analyzing unstructured data can give companies a competitive advantage. When different kinds of data sources are collated and analyzed, the results are more comprehensive and paint a more detailed picture. For example, analyzing a customer’s purchases, reviews, and call recordings with support staff—all in different formats—will reveal more about them than just looking at the customer’s purchase history. More Effective Decision-Making: Better insights lead to better decisions. Working with unstructured data, organizational leadership can predict market trends more accurately, understand customer preferences, recognize operational gaps, and identify potential risk factors. Together, these factors can contribute to more well-informed strategizing and direction-setting, helping to secure an organization’s position in its industry. Improved Personalization: The deeper an organization’s understanding of its customers, the better it can cater to their needs. With a keen awareness of customer behavior, organizations can work on boosting customer satisfaction through personalized services, products, and marketing efforts. In this way, unstructured data improves how an enterprise executes its primary role of catering to its customers. By yielding powerful insights, unstructured data supports a business in performing better at the macro and micro levels. Five Applications of AI in Unstructured Data Processing 1. Natural Language Processing (NLP): NLP techniques can be implemented on unstructured text-based datasets to enable named entity recognition, summarization, and topic modeling. Other NLP applications include AI-powered language translation solutions and text-generation platforms. 2. Computer Vision AI models can analyze images and classify the patterns, scenes, and objects contained therein. This facilitates applications such as facial recognition, object detection, and image tagging. AI algorithms can similarly analyze video content, enabling data extraction from video streams. 3. Machine Learning (ML) An ML algorithm identifies patterns, outliers, and trends in unstructured datasets. It can also predict potential outcomes by reviewing historical data and crucial factors such as market trends, customer behavior, and sales. 4. Contextual Understanding Instead of analyzing unstructured data in a vacuum, AI models can perform contextual interpretation. They can incorporate additional factors such as location, user behavior, and browsing patterns to provide a more nuanced understanding. 5. Extraction Templates Template-based extraction allows organizations to capture unstructured data from large volumes of documents. Manual template creation can be time-consuming and complicated, forcing users to build, test, and then use their required extraction template. AI-powered tools simplify and accelerate the template creation process, reducing the time it takes enterprises to implement automated extraction on unstructured data. Advantages of AI-Powered Unstructured Data Processing Organizations actively incorporating AI-based unstructured data processing into their workflows can benefit in multiple ways: Increased Efficiency AI algorithms process unstructured data more rapidly than humans. This enables an enterprise to analyze unstructured data in a fraction of the time that manual processes would take. Greater Accuracy AI models can perform analytical tasks while maintaining a high degree of accuracy. Regardless of the complexity of the data, the risk of errors is minimal, and the results are reliable. Adaptability Using machine learning techniques, AI models can learn and self-improve through feedback and new data to maintain reliability in dynamic environments. Innovation and Development AI offers plenty of opportunities for enterprises to think outside the box and develop innovative solutions. With so much potential still untapped, AI can push companies to try new approaches for dealing with data-related challenges. Minimizing The Common Risks Associated with Overreliance on AI As with all new technology, AI in unstructured data processing comes with certain risks. However, an organization can mitigate these risks with the right systems in place. Here are two examples: 1. Non-Deterministic Results AI models maintain great accuracy most of the time. However, due to their probabilistic nature, there can be instances where these models won’t be as accurate in their recommendations or solutions. To counter a potential lack of accuracy, organizations can implement AI during the design stage, when manual intervention is easier, and mistakes can be quickly rectified. In contrast, mistakes during runtime by a fully automated AI model are more difficult to catch. 2. Lack of Explainability It can be tempting to overuse AI as a catch-all solution for every unstructured data issue an organization faces. By simply generating a solution, AI can take away explainability, which is essential for understanding how a problem is solved and the steps involved. To counter this, enterprises can craft a specific role for AI in their unstructured data processing methods. With a well-defined problem and clear expectations for the outcome, AI solutions become easier to review, document, and explain. Experience AI-Powered Unstructured Data Processing At Its Finest Ready to optimize unstructured data processing for better insights that give you a competitive edge? Discover LIKE.TG's AI-powered unstructured data solutions for yourself. I Want to Start My FREE Trial How LIKE.TG’s AI-Driven Solutions Can Help LIKE.TG uses a combination of AI and template-based extraction processes to accelerate unstructured data processing. Users can extract, cleanse, prepare, and export unstructured data from multiple sources to their specified downstream destinations for further use. They can automate their workflows to run at certain times or when certain conditions are met. Best of all, they can do all this without having to write a single line of code. The result is a seamless, hassle-free process for unstructured data processing and management. At LIKE.TG, our aim is not just to democratize and simplify data operations. We also enable our clients to meet their data management requirements with strategic AI integration. Hear from our COO Jay Mishra about the intersection of AI and data management and where he thinks things in this sector are headed. Check out his EM360 Podcast episode today! It’s also available on Spotify, Google Podcasts, and Apple Podcasts.
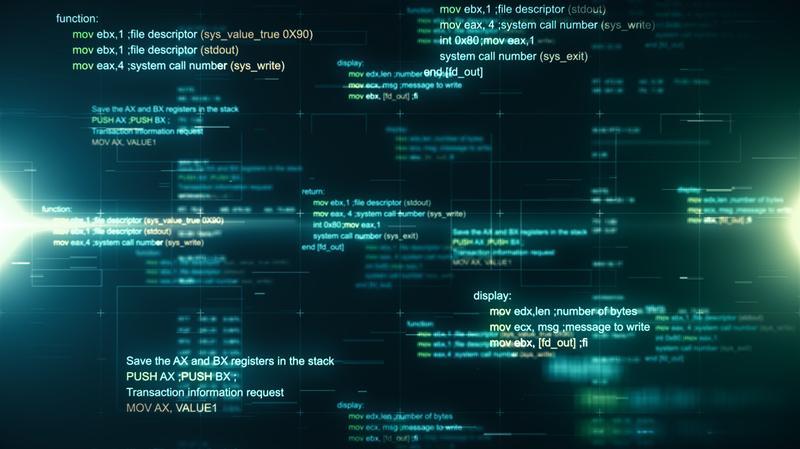
Data Vault 101: A Comprehensive Guide to Scalable Data Warehousing
As businesses deal with larger and more diverse volumes of data, managing that data has become increasingly difficult. Only 5% of businesses feel they have data management under control, while 77% of industry leaders consider growing volume of data one of the biggest challenges. Data vault is an emerging technology that enables transparent, agile, and flexible data architectures, making data-driven organizations always ready for evolving business needs. What is a Data Vault? A data vault is a data modeling technique that enables you to build data warehouses for enterprise-scale analytics. It allows you to adapt to fast-changing business requirements with an agile and incremental approach, avoiding the need for extensive re-engineering. It also ensures historical tracking and auditing of data, as it stores all the data from the source systems without any updates or deletions. Data Vault Architecture A data vault follows a three-layered architecture containing the raw, business, and information vault. This design allows you to build agile and scalable data warehouses resilient to changes in the source systems and business requirements. Raw Vault The raw vault is the most granular level, and it stores data as close to the source format as possible. It also keeps track of all the audit information from the source. Since the raw vault is insert-only, no data is ever deleted or updated. This ensures that the raw vault preserves the full history and traceability of the data.The raw vault composed of hubs, links and satellites. These tables capture business keys, relationships and attributes of your data. Hubs represent core business concepts, such as customers, products, or vehicles. They store the business keys that uniquely identify these concepts, along with some metadata information, such as load date and sequence ID. Users can use the business keys to query information about a hub. For instance, a Customer hub has CustomerID as the business key, and a Product hub will have a ProductID as the business key. Links represent the relationships between hubs. Links store the combinations of business keys, showcasing how those hubs are connected. For example, a link between Customer and Product hubs can be a PurchaseTransactions link table. It will contain data relevant to both these hubs, such as PurchaseDate, Quantity, TotalPrice. To make sure each transaction can be uniquely identified, the data vault will concatenate the CustomerID and ProductID, then generate a hash key based on the concatenated string. Satellites store the descriptive information about hubs and links. They contain the attributes that describe the characteristics or states of the hubs and links over time. For instance, the satellite for the Customer hub may store the customer’s FirstName, LastName, Address, PhoneNumber, etc. Likewise, the satellite for the PurchaseTransactions link table may store information like PaymentMethod, DeliveryStatus, LoadDate, RecordSource. In this table, PaymentMethodandDeliveryStatusprovide additional information about each transaction.LoadDateandRecordSource are metadata fields. Business Vault The business vault is another layer derived from the raw vault. It applies selected business rules, calculations, data cleansing and data quality functions to the data.The business vault can also contain hubs, links and satellites, but they are usually created based on business needs and are usually not a direct copy of those in the raw vault. The business vault is useful for providing query assistance and facilitating user access to the data. Here are some data transformations and data quality rules that might be applied in the Business Vault in our example: Data Transformation – Customer Lifetime Value Calculation:You might have a transformation rule that calculates the lifetime value of each customer based on their purchase history. This could be implemented as a new Satellite table in the Business Vault that associates eachCustomerIDwith a calculatedLifetimeValueattribute. TheLifetimeValuecould be calculated as the sum ofTotalPricefor all transactions associated with eachCustomerID. Data Transformation – Product Category Sales:You might want to track the total sales for each product category. This could be implemented as a new Hub and Satellite in the Business Vault that associates eachCategorywith a calculatedTotalSalesattribute. Data Quality Rules:You might have business rules that enforce data quality standards. For example, you might have a rule that flags any transactions where theTotalPriceis less than zero, or where theCustomerIDorProductIDdoes not exist in the respective Hub tables. These rules can be implemented as checks in the data loading process or as constraints in the database schema. Information Vault The information vault (also known as information marts) is a presentation layer built off the raw vault and business vault to support reporting and analytics. It is composed of user-friendly structures like star schemas that representor data marts. The information vault can apply further transformations and aggregations to the data to make it ready for consumption by end users or business intelligence tools. Data Vault Architecture Learn how to use information marts to deliver customized and relevant data to your end-users. The combination of raw vault, business vault, and information marts allows for better data integrity, near-real-time loading, and better accommodation of new business needs without affecting existing structures. Benefits of Data Vault Flexibility and Adaptability Data vault can handle multiple source systems and frequently changing relationships by minimizing the maintenance workload. This means that a change in one source system that creates new attributes can be easily implemented by adding another satellite to the data vault model. Similarly, new and changing relationships can be handled by closing one link and creating another one. These examples show the high level of flexibility and adaptability provided by data vault. Scalability As the data volume grows or more source systems are added, data vault scales easily. You can introduce new hubs, links, and satellites to incorporate additional data sources or entities without disrupting the existing structure. The data warehouse allows you to accommodate more data and more users without compromising performance or quality. Historical Data Preservation The use of satellite tables in data vault ensures that historical data is preserved. This is critical for tracking changes over time, analyzing trends, and meeting regulatory compliance requirements. For instance, in a clinical data warehouse, it’s important to store historical data understanding for how patient diagnoses or provider specialties have evolved over time. Data Lineage and Auditability Data vault embeds data lineage and auditability in the data vault model. In other words, the data vault stores the load date and data source for every new record, telling us when and where the data came from. Additionally, you can analyze hash keys and hash differences to quickly compare row values and identify changes. These features help to ensure data quality, traceability, and accountability. Consistency Data vault ensures data consistency by capturing data in a consistent manner even when the source data, or its delivery, is inconsistent. This means that the data warehouse can provide reliable and accurate information for business decisions. Moreover, data vault enables parallel loading of data with the use of hash values, which improves data access speed and user satisfaction. Agility Data vault supports agile development and evolution of data warehouse requirements by following a methodology that includes several principles of agile work processes. This means that data vault projects have short, scope-controlled release cycles that allow the development team to work closely with the business needs and create a better solution. Data Vault vs Traditional Data Warehouse Techniques Data vault is a modeling technique to build data warehouses but differs from traditional techniques such as dimensional model and 3NF. It has some key differences in terms of data loading, data modeling, and data agility. Data Loading Data vault loads data differently compared to traditional data warehousing techniques. Typically, data warehouses follow Extract-Transform-Load (ETL) workflow where data is transformed and validated before being loaded into the warehouse. In this technique, you must update or delete existing records in the warehouse based on the changes in the source systems. In contrast, data vault leverages the Extract-Load-Transform (ELT) workflow where the data is directly stored in the raw vault from the source system. Business rules, transformations, or validations are applied later in the process, typically inside the business vault. This approach allows you to append new records to the vault without updating or deleting existing records. You can apply business rules, transformations, and validations only when you need to use the data for reporting or analysis. Data Modeling A traditional data warehouse typically uses a dimensional modeling or a normalized modeling (3NF) to organize the data into facts and dimensions or entities and relationships, respectfully. Data vault uses a hybrid modeling technique that combines the best practices of both dimensional and normalized modeling. It leverages a hub-and-spoke schema to represent the data in a historical and flexible way. Whether data vault is the ideal data modeling for you depends on your requirements. Data Agility A Data Warehouse typically has a rigid and stable structure that is designed to meet the current and anticipated business requirements. The structure of the warehouse may change over time due to changes in business requirements, source systems, or data quality standards. However, such changes may require significant efforts and resources to modify the existing ETL processes, warehouse schemas, and reports. Data vault adapts to fast-changing business requirements by separating a data model’s stable and temporal parts.This means that the core business concepts and their relationships are stored in hubs and links, which rarely change. In contrast, the descriptive attributes and their changes over time are stored in satellites, which can be easily added or modified. This way, data vault avoids the need for extensive re-engineering of the data warehouse when new sources or attributes are introduced or existing ones are modified. Capacity to Handle High Volumes of Data Data vault supports parallel loading of data from different sources, which increases the speed and efficiency of data integration. Data vault is based on agile methodologies and techniques, allowing you to deliver value continuously and iteratively, and collaborate actively with business users. Data Vault Best Practices Data vault can handle complex and dynamic data environments. However, to ensure a successful data vault implementation, following best practices and avoiding common pitfalls is important. Here are some tips and recommendations from experts in the field: Plan and define business model and requirements clearly before designing the data vault. This helps identify the key business concepts, relationships, and attributes that will form the basis of hubs, links, and satellites. Use automation tools to speed up and simplify the development and maintenance of the data vault. Automation tools can help generate code, data models, documentation, and tests based on predefined patterns and metadata. Follow the data vault 2.0 standards and best practices to ensure consistency, quality, and scalability of the data vault. Data vault 2.0 is an updated version of the original methodology incorporating new features such as hash keys, parallel loading, audit columns, and business vault. Avoid overloading the data vault with unnecessary or redundant data. Data vault is designed to store raw data from source systems without applying any transformations or business rules. However, this does not mean one should load everything into the data vault without filtering or validating the data. Users should only load the relevant, accurate, and useful data for their business needs. Monitor and test data vaults regularly to ensure their performance, reliability, and accuracy. Data vault is a dynamic and evolving data structure that can accommodate changes in data sources and business requirements. However, this also means that users must keep track of the changes and their impacts on the data vault. Build a Data Warehouse with LIKE.TG Data vault offers many benefits, such as scalability, auditability, parallelism, and adaptability, making it a great choice for modern data warehousing needs.LIKE.TG DW Builder is a code-free and automated data warehouse design and ETL/ELT tool that allows users to build data vaults in minutes. To learn more about LIKE.TG DW Builder and its data vault features, visit the website or request a demo today.
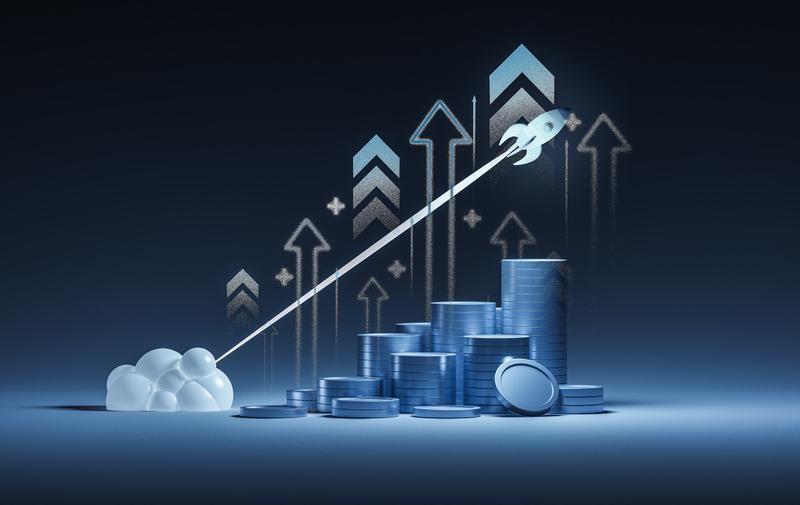
Managed File Transfer in Banking: A Complete Guide
As businesses deal with larger and more diverse volumes of data, managing that data has become increasingly difficult. Only 5% of businesses feel they have data management under control, while 77% of industry leaders consider growing volume of data one of the biggest challenges. Data vault is an emerging technology that enables transparent, agile, and flexible data architectures, making data-driven organizations always ready for evolving business needs. What is a Data Vault? A data vault is a data modeling technique that enables you to build data warehouses for enterprise-scale analytics. It allows you to adapt to fast-changing business requirements with an agile and incremental approach, avoiding the need for extensive re-engineering. It also ensures historical tracking and auditing of data, as it stores all the data from the source systems without any updates or deletions. Data Vault Architecture A data vault follows a three-layered architecture containing the raw, business, and information vault. This design allows you to build agile and scalable data warehouses resilient to changes in the source systems and business requirements. Raw Vault The raw vault is the most granular level, and it stores data as close to the source format as possible. It also keeps track of all the audit information from the source. Since the raw vault is insert-only, no data is ever deleted or updated. This ensures that the raw vault preserves the full history and traceability of the data.The raw vault composed of hubs, links and satellites. These tables capture business keys, relationships and attributes of your data. Hubs represent core business concepts, such as customers, products, or vehicles. They store the business keys that uniquely identify these concepts, along with some metadata information, such as load date and sequence ID. Users can use the business keys to query information about a hub. For instance, a Customer hub has CustomerID as the business key, and a Product hub will have a ProductID as the business key. Links represent the relationships between hubs. Links store the combinations of business keys, showcasing how those hubs are connected. For example, a link between Customer and Product hubs can be a PurchaseTransactions link table. It will contain data relevant to both these hubs, such as PurchaseDate, Quantity, TotalPrice. To make sure each transaction can be uniquely identified, the data vault will concatenate the CustomerID and ProductID, then generate a hash key based on the concatenated string. Satellites store the descriptive information about hubs and links. They contain the attributes that describe the characteristics or states of the hubs and links over time. For instance, the satellite for the Customer hub may store the customer’s FirstName, LastName, Address, PhoneNumber, etc. Likewise, the satellite for the PurchaseTransactions link table may store information like PaymentMethod, DeliveryStatus, LoadDate, RecordSource. In this table, PaymentMethodandDeliveryStatusprovide additional information about each transaction.LoadDateandRecordSource are metadata fields. Business Vault The business vault is another layer derived from the raw vault. It applies selected business rules, calculations, data cleansing and data quality functions to the data.The business vault can also contain hubs, links and satellites, but they are usually created based on business needs and are usually not a direct copy of those in the raw vault. The business vault is useful for providing query assistance and facilitating user access to the data. Here are some data transformations and data quality rules that might be applied in the Business Vault in our example: Data Transformation – Customer Lifetime Value Calculation:You might have a transformation rule that calculates the lifetime value of each customer based on their purchase history. This could be implemented as a new Satellite table in the Business Vault that associates eachCustomerIDwith a calculatedLifetimeValueattribute. TheLifetimeValuecould be calculated as the sum ofTotalPricefor all transactions associated with eachCustomerID. Data Transformation – Product Category Sales:You might want to track the total sales for each product category. This could be implemented as a new Hub and Satellite in the Business Vault that associates eachCategorywith a calculatedTotalSalesattribute. Data Quality Rules:You might have business rules that enforce data quality standards. For example, you might have a rule that flags any transactions where theTotalPriceis less than zero, or where theCustomerIDorProductIDdoes not exist in the respective Hub tables. These rules can be implemented as checks in the data loading process or as constraints in the database schema. Information Vault The information vault (also known as information marts) is a presentation layer built off the raw vault and business vault to support reporting and analytics. It is composed of user-friendly structures like star schemas that representor data marts. The information vault can apply further transformations and aggregations to the data to make it ready for consumption by end users or business intelligence tools. Data Vault Architecture Learn how to use information marts to deliver customized and relevant data to your end-users. The combination of raw vault, business vault, and information marts allows for better data integrity, near-real-time loading, and better accommodation of new business needs without affecting existing structures. Benefits of Data Vault Flexibility and Adaptability Data vault can handle multiple source systems and frequently changing relationships by minimizing the maintenance workload. This means that a change in one source system that creates new attributes can be easily implemented by adding another satellite to the data vault model. Similarly, new and changing relationships can be handled by closing one link and creating another one. These examples show the high level of flexibility and adaptability provided by data vault. Scalability As the data volume grows or more source systems are added, data vault scales easily. You can introduce new hubs, links, and satellites to incorporate additional data sources or entities without disrupting the existing structure. The data warehouse allows you to accommodate more data and more users without compromising performance or quality. Historical Data Preservation The use of satellite tables in data vault ensures that historical data is preserved. This is critical for tracking changes over time, analyzing trends, and meeting regulatory compliance requirements. For instance, in a clinical data warehouse, it’s important to store historical data understanding for how patient diagnoses or provider specialties have evolved over time. Data Lineage and Auditability Data vault embeds data lineage and auditability in the data vault model. In other words, the data vault stores the load date and data source for every new record, telling us when and where the data came from. Additionally, you can analyze hash keys and hash differences to quickly compare row values and identify changes. These features help to ensure data quality, traceability, and accountability. Consistency Data vault ensures data consistency by capturing data in a consistent manner even when the source data, or its delivery, is inconsistent. This means that the data warehouse can provide reliable and accurate information for business decisions. Moreover, data vault enables parallel loading of data with the use of hash values, which improves data access speed and user satisfaction. Agility Data vault supports agile development and evolution of data warehouse requirements by following a methodology that includes several principles of agile work processes. This means that data vault projects have short, scope-controlled release cycles that allow the development team to work closely with the business needs and create a better solution. Data Vault vs Traditional Data Warehouse Techniques Data vault is a modeling technique to build data warehouses but differs from traditional techniques such as dimensional model and 3NF. It has some key differences in terms of data loading, data modeling, and data agility. Data Loading Data vault loads data differently compared to traditional data warehousing techniques. Typically, data warehouses follow Extract-Transform-Load (ETL) workflow where data is transformed and validated before being loaded into the warehouse. In this technique, you must update or delete existing records in the warehouse based on the changes in the source systems. In contrast, data vault leverages the Extract-Load-Transform (ELT) workflow where the data is directly stored in the raw vault from the source system. Business rules, transformations, or validations are applied later in the process, typically inside the business vault. This approach allows you to append new records to the vault without updating or deleting existing records. You can apply business rules, transformations, and validations only when you need to use the data for reporting or analysis. Data Modeling A traditional data warehouse typically uses a dimensional modeling or a normalized modeling (3NF) to organize the data into facts and dimensions or entities and relationships, respectfully. Data vault uses a hybrid modeling technique that combines the best practices of both dimensional and normalized modeling. It leverages a hub-and-spoke schema to represent the data in a historical and flexible way. Whether data vault is the ideal data modeling for you depends on your requirements. Data Agility A Data Warehouse typically has a rigid and stable structure that is designed to meet the current and anticipated business requirements. The structure of the warehouse may change over time due to changes in business requirements, source systems, or data quality standards. However, such changes may require significant efforts and resources to modify the existing ETL processes, warehouse schemas, and reports. Data vault adapts to fast-changing business requirements by separating a data model’s stable and temporal parts.This means that the core business concepts and their relationships are stored in hubs and links, which rarely change. In contrast, the descriptive attributes and their changes over time are stored in satellites, which can be easily added or modified. This way, data vault avoids the need for extensive re-engineering of the data warehouse when new sources or attributes are introduced or existing ones are modified. Capacity to Handle High Volumes of Data Data vault supports parallel loading of data from different sources, which increases the speed and efficiency of data integration. Data vault is based on agile methodologies and techniques, allowing you to deliver value continuously and iteratively, and collaborate actively with business users. Data Vault Best Practices Data vault can handle complex and dynamic data environments. However, to ensure a successful data vault implementation, following best practices and avoiding common pitfalls is important. Here are some tips and recommendations from experts in the field: Plan and define business model and requirements clearly before designing the data vault. This helps identify the key business concepts, relationships, and attributes that will form the basis of hubs, links, and satellites. Use automation tools to speed up and simplify the development and maintenance of the data vault. Automation tools can help generate code, data models, documentation, and tests based on predefined patterns and metadata. Follow the data vault 2.0 standards and best practices to ensure consistency, quality, and scalability of the data vault. Data vault 2.0 is an updated version of the original methodology incorporating new features such as hash keys, parallel loading, audit columns, and business vault. Avoid overloading the data vault with unnecessary or redundant data. Data vault is designed to store raw data from source systems without applying any transformations or business rules. However, this does not mean one should load everything into the data vault without filtering or validating the data. Users should only load the relevant, accurate, and useful data for their business needs. Monitor and test data vaults regularly to ensure their performance, reliability, and accuracy. Data vault is a dynamic and evolving data structure that can accommodate changes in data sources and business requirements. However, this also means that users must keep track of the changes and their impacts on the data vault. Build a Data Warehouse with LIKE.TG Data vault offers many benefits, such as scalability, auditability, parallelism, and adaptability, making it a great choice for modern data warehousing needs.LIKE.TG DW Builder is a code-free and automated data warehouse design and ETL/ELT tool that allows users to build data vaults in minutes. To learn more about LIKE.TG DW Builder and its data vault features, visit the website or request a demo today.
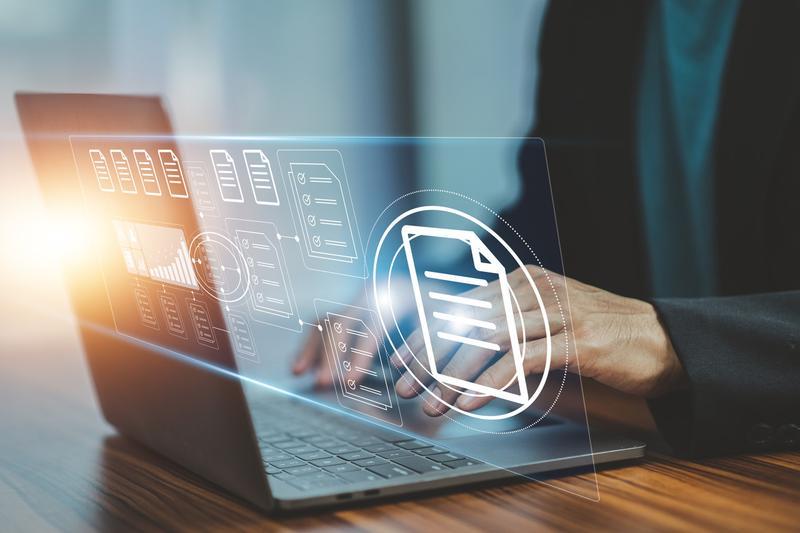
ETL Using Python: Exploring the Pros vs. Cons
Are you looking to automate and streamline your data integration process? ETL (extract, transform, and load) collects data from various sources, applies business rules and transformations, and loads the data into a destination system. Today, you will learn how to build ETL pipelines using Python – a popular and versatile programming language. Is It Possible to Build ETL Using Python? Yes! Python has a rich set of libraries and frameworks that can handle different aspects of the ETL process, such as data extraction, manipulation, processing, and loading. Python makes it easy to create ETL pipelines that manage and transform data based on business requirements. There are several ETL tools written in Python that leverage Python libraries for extracting, loading and transforming diverse data tables imported from multiple data sources into data warehouses. Python ETL tools are fast, reliable, and deliver high performance. Some top tools that build ETL using Python are: Apache Airflow Luigi petl Spark pandas Advantages of Configuring ETL Using Python Easy to Learn Python has a simple and consistent syntax that makes writing and understanding ETL code easy. Python also has a REPL (read-eval-print loop) that allows interactive ETL code testing and debugging. Moreover, Python has a “batteries included” philosophy that provides built-in modules and functions for everyday ETL tasks, such as data extraction, manipulation, processing, and loading. For instance, you can use the CSV module to read and write CSV files, the JSON module to handle JSON data, the SQLite3 module to connect to SQLite databases, and the urllib module to access web resources. Therefore, if you are looking for a simple way to build data pipelines, configuring ETL using Python might be a good choice. Flexibility Python has a flexible and dynamic typing system allows ETL developers to work with different data sources and formats, such as CSV, JSON, SQL, and XML. Python supports multiple paradigms and styles of programming, such as object-oriented, functional, and procedural, that enable ETL developers to choose the best approach for their ETL logic and design. Python also has a modular and scalable structure that allows ETL developers to organize their ETL code into reusable and maintainable components, such as functions, classes, and modules. For instance, you can use the Pandas library to create and manipulate DataFrames, the NumPy library to perform numerical computations, the SciPy library to apply scientific and statistical functions, and the Matplotlib library to generate and display data visualizations. Therefore, if you are looking for a flexible and adaptable way to build data pipelines, ETL using Python is the way to go. Power Python has a robust and diverse set of third-party libraries and frameworks that can handle different aspects of the ETL process, such as data extraction, transformation, loading, and workflow management. Some standard Python tools and frameworks for ETL are Pandas, Beautiful Soup, Odo, Airflow, Luigi, and Bonobo. These tools and frameworks provide features and functionalities that can enhance the performance and efficiency of the ETL process, such as data cleaning, data aggregation, data merging, data analysis, data visualization, web scraping, data movement, workflow management, scheduling, logging, and monitoring. For instance, you can use the Beautiful Soup library to extract data from HTML and XML documents, the Odo library to move data between different formats and sources, the Airflow framework to create and run ETL pipelines, the Luigi framework to build complex data pipelines, and the Bonobo framework to build ETL pipelines using a functional programming approach. Drawbacks of Configuring ETL Using Python Performance Python is an interpreted language that runs slower than compiled languages, such as C or Java. Python also has a global interpreter lock (GIL) that prevents multiple threads from executing Python code simultaneously, limiting the concurrency and parallelism of the ETL process. Python also has a high memory consumption and garbage collection overhead, which can affect the scalability and stability of the ETL process. Therefore, if you are dealing with large and complex data sets, configuring ETL using Python may affect your system’s performance. Compatibility Python has multiple versions and implementations, such as Python 2 and 3 or CPython and PyPy, which can cause compatibility issues and inconsistencies in the ETL code and environment. Python also has a dependency management system that can be complex and cumbersome to manage, especially when dealing with multiple libraries and frameworks for ETL. Moreover, Python lacks standardization and documentation for some ETL tools and frameworks, making learning and using them challenging. For instance, there are many different ways to connect to a database using Python, such as psycopg2, SQLalchemy, pyodbc, and cx_Oracle, but each has syntax, features, and limitations. Therefore, building ETL pipelines using Python can be difficult when you’re working with different data sources and formats. Complexity Configuring ETL using Python is complex and challenging to design, develop, and debug, especially when you’re dealing with large and diverse data sources and formats, such as CSV, JSON, SQL, and XML. Python ETL developers need to have a good understanding of the data sources, the business logic, and the data transformations, as well as the Python libraries and frameworks that can handle them. Python ETL developers also need to write many custom codes and scripts to connect, extract, transform, and load data, which can be prone to errors and bugs. For instance, if you want to extract data from a web page using Python, you may have to use a library like Beautiful Soup to parse the HTML, a library like Requests to make HTTP requests and a library like LXML to handle XML data. Therefore, you might have to spend a lot of time and effort configuring ETL using Python and debugging data pipelines. Maintenance Maintaining and updating ETL using Python can be difficult and costly to, especially when the data sources, the business requirements, or the destination systems change. Python ETL developers must constantly monitor and test the ETL pipelines, handle errors and exceptions, log and track the ETL process, and optimize the ETL performance. Python ETL developers also need to ensure the quality and accuracy of the data, as well as the security and compliance of the data transfer. For instance, if you want to load data into a data warehouse using Python, you may have to use a library like sqlalchemy to create and manage the database schema, a library like Pandas to manipulate and validate the data, and a library like pyodbc to execute the SQL queries. Therefore, you may have a messy and unreliable ETL pipeline that can compromise your data quality and integrity if you are not careful and diligent. Scalability As your data increases in volume and variety, Python code can increase in length and complexity, making it harder to maintain. Building ETL using Python can also be challenging with large and complex data sets, as it can exhaust the memory or have long execution times. To improve the scalability and efficiency of the ETL, users can leverage distributed computing frameworks, such as Spark or Hadoop, which can utilize multiple nodes and parallel processing to handle large and complex data sets. However, integrating Python with these frameworks can also pose challenges, as it can require additional configuration and coding, increasing the ETL’s complexity and overhead. Discover LIKE.TG Centerprise’s Benefits for Finance 360 LIKE.TG's user-friendly ETL automates data pipelines for Finance 360. Boost efficiency gain a single source of truth. Learn More ETL Using Python vs. LIKE.TG Aspect LIKE.TG Python Data Integration Supports various data sources and destinations with ease. Supports multiple data types and formats but requires additional libraries for different sources. Data Quality Provides advanced data profiling and quality rules. Lacks built-in quality framework, requiring external libraries for checks and validations. Data Transformations Supports visual design for data transformations and mappings. Requires coding for transformations, potentially slower iterations. Data Governance Offers a robust governance framework for compliance. Lacks built-in governance, necessitating external libraries for encryption and security. Customizability Offers a code-free interface for ETL pipeline design. Provides a versatile language for custom logic but requires extensive coding. Performance Utilizes parallel processing for efficient handling. Slower due to interpretation, limited concurrency, and high memory consumption. Maintenance Provides a visual interface for debugging and optimizing. Requires constant monitoring, error handling, and performance optimization. Complexity Simplifies ETL pipeline management with intuitive UI. Demands extensive coding and rigorous maintenance processes. Scalability Accelerates reading large datasets from databases and files by partitioning data, breaking tables into chunks, and reading them simultaneously High memory consumption and complex dependency management hinder scalability. Security Offers advanced security features compliant with industry standards. Relies on external libraries for security and may lack compliance with specific regulations. Cost Savings Significant long-term cost savings The need for skilled, high-end developers and ongoing maintenance offsets lower upfront costs. Self-Regulating Pipelines Provides features for automated monitoring, alerts, and triggers. Requires custom implementation for automated pipelines. Workflow Automation Offers built-in workflow orchestration and scheduling features. Relies on external libraries or frameworks for workflow automation. Time to Market Rapid development with intuitive UI and pre-built connectors. Longer development time due to coding and integration requirements. How LIKE.TG Streamlines ETL Python and LIKE.TG are powerful and popular tools, but LIKE.TG has some clear advantages and benefits over Python that you should know about. LIKE.TG is a no-code ETL platform that lets you create, monitor, and manage data pipelines without writing code.It has a graphical user interface, making it easy to drag and drop various components, such as data sources, destinations, transformations, and workflows, to build and execute ETL pipelines. You can also see the data flow and the results in real time, which helps you validate and troubleshoot your ETL logic. LIKE.TG supports various data types and formats, such as CSV, JSON, databases, XML, unstructured documents and can integrate with multiple systems and platforms, such as databases, data warehouses, data lakes, cloud services, and APIs. LIKE.TG further improves ETL performance thanks to parallel processing. LIKE.TG supports parallel and distributed processing, which can leverage the power of multiple cores and nodes to handle large data processing tasks. Likewise, LIKE.TG offer low memory consumption and an intelligent caching mechanism, which can improve scalability and stability. Moreover, LIKE.TG has a standardized and documented platform that can make it easy to learn and use effectively. LIKE.TG ETL pipelines can also be simple and easy to design, develop, and debug, especially when dealing with large and diverse data sources and formats, such as CSV, JSON, SQL, and XML. You don’t have to write complex, lengthy code or scripts to transform and load your data. You can use the built-in components and functions LIKE.TG provides or create custom ones if necessary. You can easily reuse and share your ETL pipelines across different projects and teams, increasing productivity and collaboration. Ready to experience the power and potential of no-code ETL tools like LIKE.TG for your data integration projects? If so, you can take the next step and request a free 14-day trial or schedule a custom demo today.
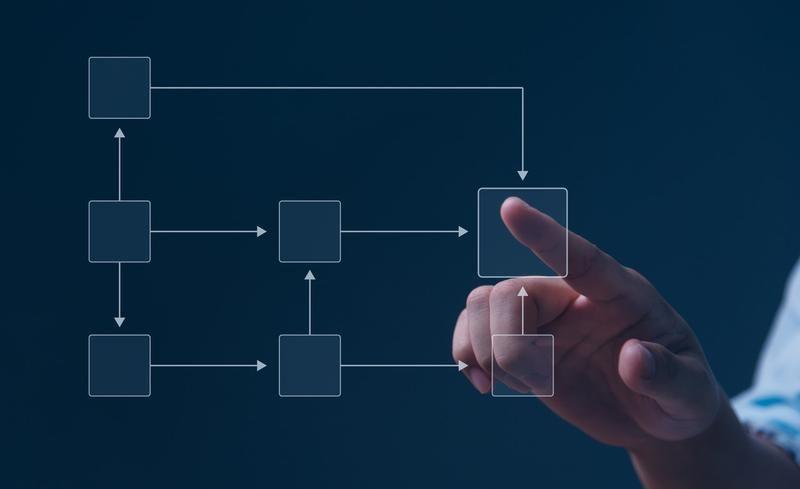
Car Insurance Claims Processing With A Data-Driven Approach
A shift from manual to digital processing in the insurance sector, especially for handling unstructured data, is defining the industry’s future. Digital transformation is a key for insurers to efficiently manage the increasing volumes of complex data. McKinsey Company show a potential 60% rise in operating profits through effective data utilization. The digital shift streamlines claim processing and boosts customer satisfaction, as faster claim resolutions directly correlate with improved customer experiences. The evolution in car insurance claims management reflects an industry-wide commitment to operational excellence and heightened customer service. This blog is part one of two, focusing on how to process car insurance claims using data. We’ll discuss the importance of using data analytics and technology in the insurance industry and the benefits it brings. Part two will focus on LIKE.TG ReportMiner, a tool that helps process claims better by extracting and analyzing data from reports and documents, making handling car insurance claims more efficient and accurate. The Evolution of Car Insurance Claims Processing The car insurance industry has changed its operations by shifting from manual to digital methods for claims processing. Traditionally, processing claims involved a lot of paperwork and manual labor, which was time-consuming and prone to errors. Now, with digital tools, the focus is on handling the vast unstructured data that comes with each claim, like photos from an accident scene or handwritten notes. For instance, many insurers are now using digital platforms that can quickly scan and extract information from photos and PDF documents. This means claims can be processed much faster and more accurately than before. A practical example is the use of digital tools to assess car damage through uploaded photos, speeding up the assessment process. According to a Deloitte study, this technological shift can lead to a 30% reduction in processing costs, highlighting efficiency and cost-effectiveness. This digital transformation in car insurance claims processing allows insurance companies to provide quicker and more reliable service to their customers. It is about adapting technology to the evolving needs of the customer and improving the overall experience in the event of a claim. The Role of Unstructured Data in Car Insurance Claims Car insurance claims generate a lot of unstructured data, such as emails, photos, and PDFs. Handling this data properly is crucial to avoid mistakes in claims and delays. The main task is to sort out and extract the relevant information from these different formats efficiently. Why is Unstructured Data Important? Unstructured data holds insights that can help insurers understand the context and details of each claim. Unstructured data, found in documents like PDFs and emails, sheds light on the details of each claim. This includes understanding the full scope of damage, identifying all parties involved, and piecing together the sequence of events leading to the incident. When insurers analyze detailed breakdowns of repair costs or read written descriptions of an accident, they gain insights that go beyond mere numbers. This analysis helps pinpoint the specific parts of a vehicle affected and assess the accident’s impact, enabling a more informed evaluation of the claim. The detail within unstructured data can also help insurers spot potential fraud. Discrepancies or anomalies in the repair estimates that do not match the described damage can be red flags, prompting further investigation. Access to details through unstructured data allows insurers to process claims with a higher degree of empathy and accuracy. Knowing the complete information behind each claim means insurers can respond more thoughtfully and personally to each customer, improving satisfaction and trust. The Role of Automation Software Claims automation software uses technologies like Optical Character Recognition (OCR) to transform data from PDFs and scanned documents into machine-readable formats, making it ready for data extraction. This is especially useful in car insurance claims where critical details like damage assessments are often hidden in unstructured data. For example, AI-driven systems can extract and classify specific damage details from scanned claim forms, improving the accuracy and speed of the claims assessment process. This ensures that all crucial information, regardless of its original format, is used to develop a well-informed and efficient claims resolution strategy. The effective handling of unstructured data directly impacts the speed and accuracy of claim resolutions. It ensures that all relevant information is considered, leading to fairer outcomes, and reducing the risk of disputes. Moreover, quick and accurate claims processing significantly enhances customer satisfaction, aligning with the insurance industry’s goal to provide exemplary service. Leveraging Technology to Effectively Handle Unstructured Data in Car Insurance Claims AI and machine learning are reshaping the processing of unstructured data in car insurance claims by enhancing efficiency and accuracy while propelling the insurance industry towards a more data-driven, customer-centric approach. Insurers can now offer quicker and more accurate claim settlements, meeting the demands of today’s policyholders. For example, when processing a PDF document that contains a detailed repair estimate, AI-powered pattern recognition can quickly spot and sort each item. This precision enables insurers to quickly process and make informed decisions, resulting in efficient claim resolutions. Machine learning algorithms learn from every document processed, by analyzing patterns and outcomes. This constantly refines their data identification and classification capabilities. This learning curve improves the system’s ability to accurately identify and classify data with time. It is also essential in adapting to new complexities and formats within car insurance claims, enabling insurers to remain agile and responsive in a dynamic environment. Benefits of Using a Data-Driven Approach to Car Insurance Claims Processing When car insurance claims are processed using a data-driven approach, every decision and action throughout the claim lifecycle is based on accurate and real-time data. Enhances Operational Efficiency Data-driven strategies enhance operational efficiency, allowing insurers to process claims faster and more accurately. Machine learning algorithms play a crucial role in this process by analyzing vast datasets, learning from them, and predicting outcomes. As a result, the claims handling process is continually refined based on evolving data patterns, leading to faster resolution of claims and a higher level of accuracy in evaluating each case. Handles Complex Unstructured Data Insurers can use advanced analytics and AI to navigate the complexities of unstructured documents in claims processing. This approach provides up-to-date and comprehensive data to make informed decisions. The large volume and diversity of documents, including digital and scanned forms, pose significant challenges. Manual processing can lead to errors due to the diverse data within each document. Using technology to manage these complexities streamlines the process and improves claims handling accuracy. Improves Customer Satisfaction In addition, this approach improves customer satisfaction. By speeding up the claims process and making data-backed decisions, policyholders experience quicker and more transparent claim resolutions. Data-driven methods can adapt to new data formats and challenges, enabling insurers to stay ahead and provide personalized and efficient service to their customers. Final Word Managing unstructured data in car insurance claims is crucial to building an efficient, accurate, and customer-centric claims processing system. The next part of this series will explore specific solutions that make the car insurance claims process smoother. One of these solutions is LIKE.TG ReportMiner. This tool helps extract and process data in the insurance industry more effectively. Get ready to learn how LIKE.TG ReportMiner improves operations, makes things faster, and changes the way car insurance claims are handled. Stay tuned to discover how technology like LIKE.TG ReportMiner is shaping the future of insurance! Optimize Car Insurance Claims Processing With LIKE.TG Ready to Simplify Your Claims Process? Try LIKE.TG's Data Extraction for Free! Get started with a 14-Day Free Trial and see how easy and efficient your claims processing can be. Learn More