坐席多开
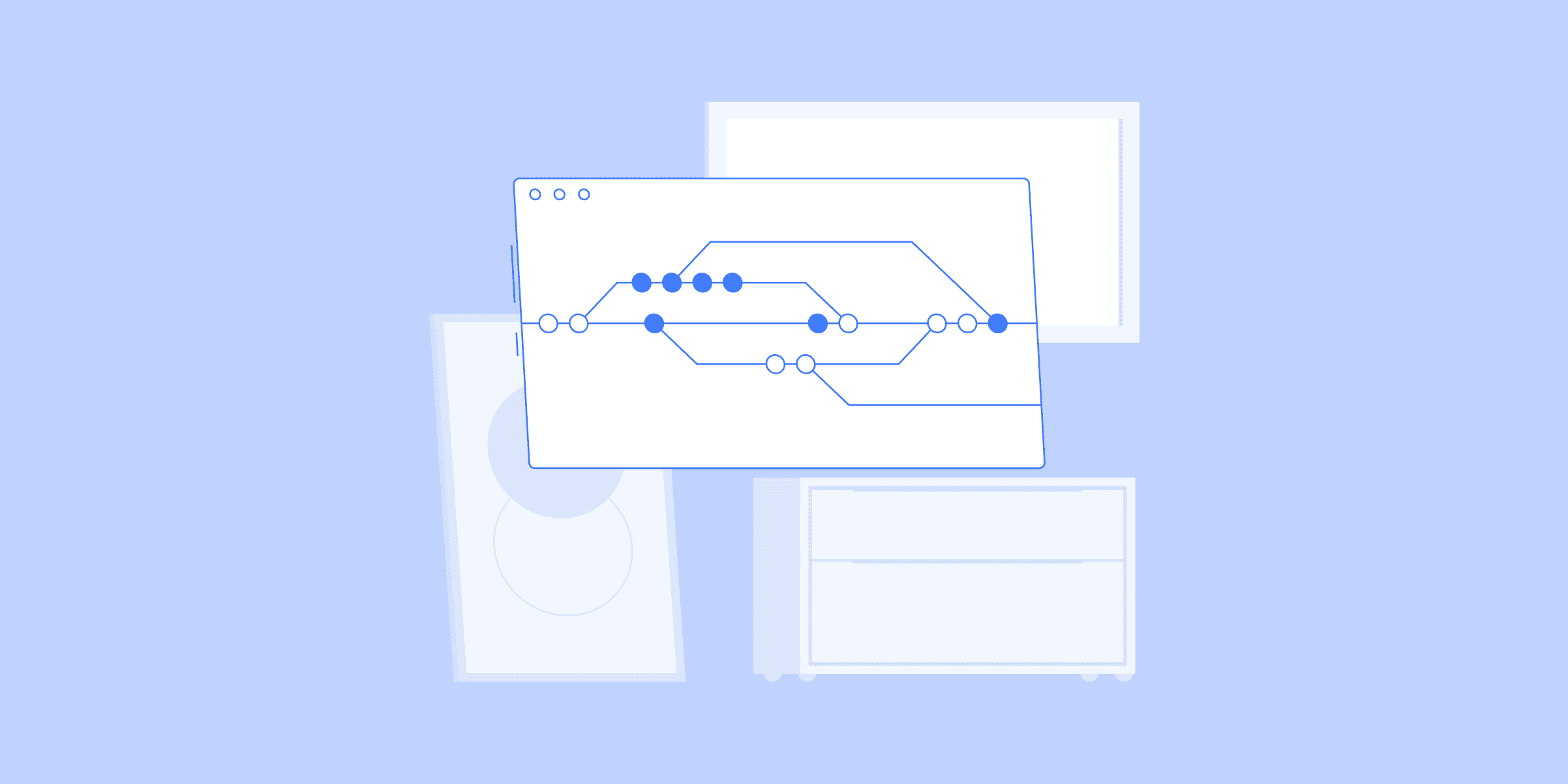
Managerial Accounting – Definition, Objective, Techniques & Limitations
Decision-making holds the key to success within business management. Managerial accounting helps leaders make smart decisions by giving them important financial information. This specialised type of accounting looks closely at a company’s financial data to aid in internal decision-making. Managerial accounting empowers managers with the insights they need to allocate resources effectively, optimise pricing strategies, and navigate the ever-changing business landscape. This article explores the core of managerial accounting, shedding light on its significance, scope, techniques, and limitations, while also highlighting the transformative power of Financial Services CRM with LIKE.TG in revolutionising managerial accounting practices.
What is managerial accounting?
In business management, managerial accounting is essential for making the best decisions. It turns financial data into useful information, helping managers confidently handle business challenges.
Unlike traditional accounting, which focuses on external reporting and compliance, managerial accounting looks into the specifics of an organisation’s financial health. It encompasses the identification, measurement, analysis, interpretation, and communication of financial information that is relevant to internal users, particularly those involved in decision-making, planning, and controlling the organisation’s activities.
Managerial accounting helps management understand the company’s financial performance by providing detailed reports, budgets, and cost analyses. It gives them the tools to find areas for improvement and make smart decisions about resource use, pricing, and business operations. It acts like a guide, helping decision-makers achieve profitability and growth.
Essentially, managerial accounting is the financial backbone of sound decision-making, providing managers with the knowledge and insights they need to steer their organisations towards success. It is the key that unlocks the door to informed decision-making and sustainable business growth.
The importance of managerial accounting
Managerial accounting holds a prominent position as a powerful tool that empowers managers to navigate the complexities of financial decision-making. It serves as a beacon, guiding them through the ever-changing landscape of resource allocation and pricing strategies. By providing managers with accurate and timely financial data, managerial accounting enables them to make informed choices that optimise costs, enhance profitability, and contribute to the overall financial well-being of the organisation.
Managerial accounting plays a major role in risk management and opportunity identification. Through meticulous analysis of financial information, managers can anticipate potential pitfalls and seize emerging opportunities. This proactive approach allows organisations to stay ahead of the curve, adapting swiftly to market dynamics and maintaining a competitive edge in an environment that changes rapidly.
Managerial accounting is also instrumental in facilitating effective planning and budgeting processes. By shedding light on past financial performance and present financial standing, it empowers managers to set realistic and achievable goals for the future. This data-driven approach minimises uncertainties, ensures efficient resource allocation, and aligns the organisation’s strategic objectives with its financial capabilities.
Managerial accounting is necessary for good decision-making in a company. It gives managers the information and tools they need to guide the company towards growth, profitability, and long-term success. Using managerial accounting practices is needed for businesses that want to succeed today.
The scope of managerial accounting
Managerial accounting encompasses a wide range of activities that are essential for effective decision-making within an organisation. These activities include:
Cost Accounting: Managerial accountants play a crucial role in identifying, classifying, and allocating costs associated with various products, services, or departments within the organisation. This involves analysing and interpreting cost data to determine the profitability of different products or services, as well as evaluating the efficiency of various operations.
Budgeting: Managerial accountants assist in the development and implementation of budgets, which serve as financial roadmaps for the organisation. They analyse historical financial data, industry trends, and market conditions to create realistic and achievable budget targets. Budgeting helps managers allocate resources effectively, control costs, and monitor financial performance.
Performance Evaluation: Managerial accountants evaluate the financial performance of the organisation and its various segments by analysing financial statements, ratios, and other relevant metrics. They identify variances between actual results and budgeted targets and investigate the reasons for such deviations. This enables managers to assess the effectiveness of their strategies and make necessary adjustments to improve performance.
Decision Making: Managerial accountants provide valuable insights and analysis to support decision-making processes within the organisation. They evaluate the financial implications of various decisions, such as capital investments, product launches, or market expansion. By presenting relevant financial information and conducting cost-benefit analyses, managerial accountants help managers make informed choices that align with the organisation’s strategic objectives.
Reporting and Communication: Managerial accountants prepare and communicate financial reports and analyses to various stakeholders within the organisation, including top management, department heads, and investors. These reports provide critical financial information that enables managers to monitor the organisation’s financial health, identify trends, and make informed decisions.
The scope of managerial accounting encompasses a diverse range of activities that are essential for effective decision-making, planning, and control within an organisation. Managerial accountants play a vital role in providing accurate and timely financial information, analysing costs, evaluating performance, and supporting management in achieving the organisation’s financial goals and objectives.
Managerial accounting techniques
Managerial accounting employs various techniques to provide managers with valuable insights and information for decision-making. Here are five key managerial accounting techniques:
**Cost-Volume-Profit (CVP) Analysis**: CVP analysis examines the relationship between costs, volume of activity, and profits. It helps managers understand how changes in costs and sales volume impact the organisation’s profitability. By analysing the break-even point and the margin of safety, managers can make informed decisions on pricing, production levels, and cost control strategies.
**Budgeting**: Budgeting is the process of creating a financial plan for a specific period, usually a year. It involves estimating revenues, expenses, and cash flows. Budgets serve as a roadmap for the organisation’s financial activities and help managers allocate resources effectively, control costs, and stay on track with financial goals.
**Standard Costing**: Standard costing assigns predetermined costs to products or services based on historical data and engineering estimates. By comparing actual costs with standard costs, managers can identify variances and investigate the underlying causes. This technique helps control costs, improve efficiency, and set performance targets.
**Marginal Costing**: Marginal costing focuses on the incremental costs associated with producing additional units of a product or service. It helps managers understand the impact of changes in production volume on profitability. By comparing the marginal cost with the marginal revenue, managers can make informed decisions on pricing, product mix, and outsourcing.
**Activity-Based Costing (ABC)**: ABC is a costing method that assigns costs to products or services based on the activities required to produce them. Unlike traditional costing methods, ABC recognises that not all costs are directly related to production volume. By identifying the cost drivers and allocating costs more accurately, ABC helps managers improve product costing, pricing, and decision-making.
These managerial accounting techniques are powerful tools that empower managers to make informed decisions, optimise resource allocation, control costs, and enhance profitability. By leveraging these techniques, organisations can achieve their strategic objectives and gain a competitive advantage in the market.
Cost-volume-profit (CVP) analysis
Cost-volume-profit (CVP) analysis is a cornerstone technique in managerial accounting that enables organisations to understand the intricate relationships between costs, volume, and profits. This powerful tool provides valuable insights into the impact of various scenarios on an organisation’s profitability, enabling managers to make informed decisions about pricing, production, and marketing strategies.
At the heart of CVP analysis lies the concept of the break-even point, a critical threshold where total revenues equal total costs, resulting in zero profit or loss. Calculating the break-even point is essential as it serves as a benchmark against which various scenarios can be evaluated. By analysing the break-even point, managers can determine the minimum sales volume required to cover all costs and achieve profitability.
Another key aspect of CVP analysis is the margin of safety, which represents the excess of sales revenue over the break-even point. This concept is vital in assessing the organisation’s ability to withstand fluctuations in sales volume or unexpected cost increases without slipping into losses. A wider margin of safety indicates a more robust financial position and provides a buffer against potential risks.
CVP analysis is crucial in the decision-making processes. By simulating different scenarios and analysing their impact on profits, managers can evaluate the effects of pricing changes, variations in production levels, or the introduction of new products or services. This enables them to make informed choices that optimise profitability and align with the organisation’s strategic objectives.
CVP analysis empowers managers with the knowledge and insights necessary to navigate the complex business landscape. By understanding the cost-volume-profit relationships, managers can make well-informed decisions that drive growth, profitability, and long-term success for their organisations.
Limitations of managerial accounting
Despite the numerous benefits of managerial accounting, it is essential to acknowledge its limitations. These limitations should be considered when using managerial accounting information for decision-making purposes.
One limitation of managerial accounting is the reliance on estimates and assumptions. Many managerial accounting techniques, such as budgeting and standard costing, require managers to make assumptions about future events. These assumptions may not always be accurate, which can lead to errors in decision-making. For example, if a manager underestimates the cost of a new product, the organisation may end up losing money on the product.
Another limitation of managerial accounting is the potential for inaccuracy. Managerial accounting information is often based on data from various sources, such as financial statements, sales reports, and production reports. If any of these data sources are inaccurate, it can lead to errors in managerial accounting information. For example, if a sales report contains incorrect sales figures, the manager may make decisions based on incorrect information about the organisation’s financial performance.
Managerial accounting also has a limitation in that it primarily focuses on financial data. While financial data is important, it does not provide a complete picture of an organisation’s performance. Other non-financial factors, such as customer satisfaction, employee morale, and market share, are also important for decision-making. However, managerial accounting often does not provide information about these non-financial factors.
To continue, implementing managerial accounting systems can be complex and time-consuming. Organisations need to invest in software, train employees, and develop procedures to collect and analyse data. This can be a significant challenge for small businesses or organisations with limited resources.
Lastly, managerial accounting requires specialised knowledge and skills. Accountants and managers need to have a strong understanding of accounting principles and financial analysis techniques to use managerial accounting information effectively. This can be a challenge for organisations that do not have access to qualified accounting professionals.
Whilst managerial accounting provides valuable information for decision-making, it is essential to be aware of its limitations. These limitations include the reliance on estimates and assumptions, the potential for inaccuracy, the focus on financial data, the complexity of implementation, and the need for specialised knowledge and skills. Managers should consider these limitations when using managerial accounting information to make the best decisions.
Financial Services CRM with LIKE.TG
LIKE.TG Financial Services Cloud is a customer relationship management (CRM) platform that is specifically designed for the financial services industry. It provides a comprehensive suite of tools that can help financial institutions enhance customer experiences, increase operational efficiency, and drive revenue growth.
One of the key benefits of LIKE.TG Financial Services Cloud is its ability to provide a 360-degree view of the customer. This means that financial advisors have access to all of the relevant customer information in one place, including account history, contact information, and interactions with the company. This allows advisors to provide more personalised and relevant service to their clients.
LIKE.TG Financial Services Cloud also offers a variety of tools that can help financial institutions automate their processes and improve operational efficiency. For example, the platform can be used to automate tasks such as lead generation, account management, and compliance reporting. This can free up financial advisors to focus on more strategic tasks, such as providing advice to clients and growing their business.
LIKE.TG Financial Services Cloud can help financial institutions drive revenue growth by providing them with tools to identify and track sales opportunities. The platform also offers a variety of marketing tools that can be used to reach new customers and grow the business.
Overall, LIKE.TG Financial Services Cloud is a powerful CRM platform that can help financial institutions improve customer experiences, increase operational efficiency, and drive revenue growth. By leveraging the power of LIKE.TG, financial institutions can gain a competitive edge and achieve success in the rapidly changing financial services industry.
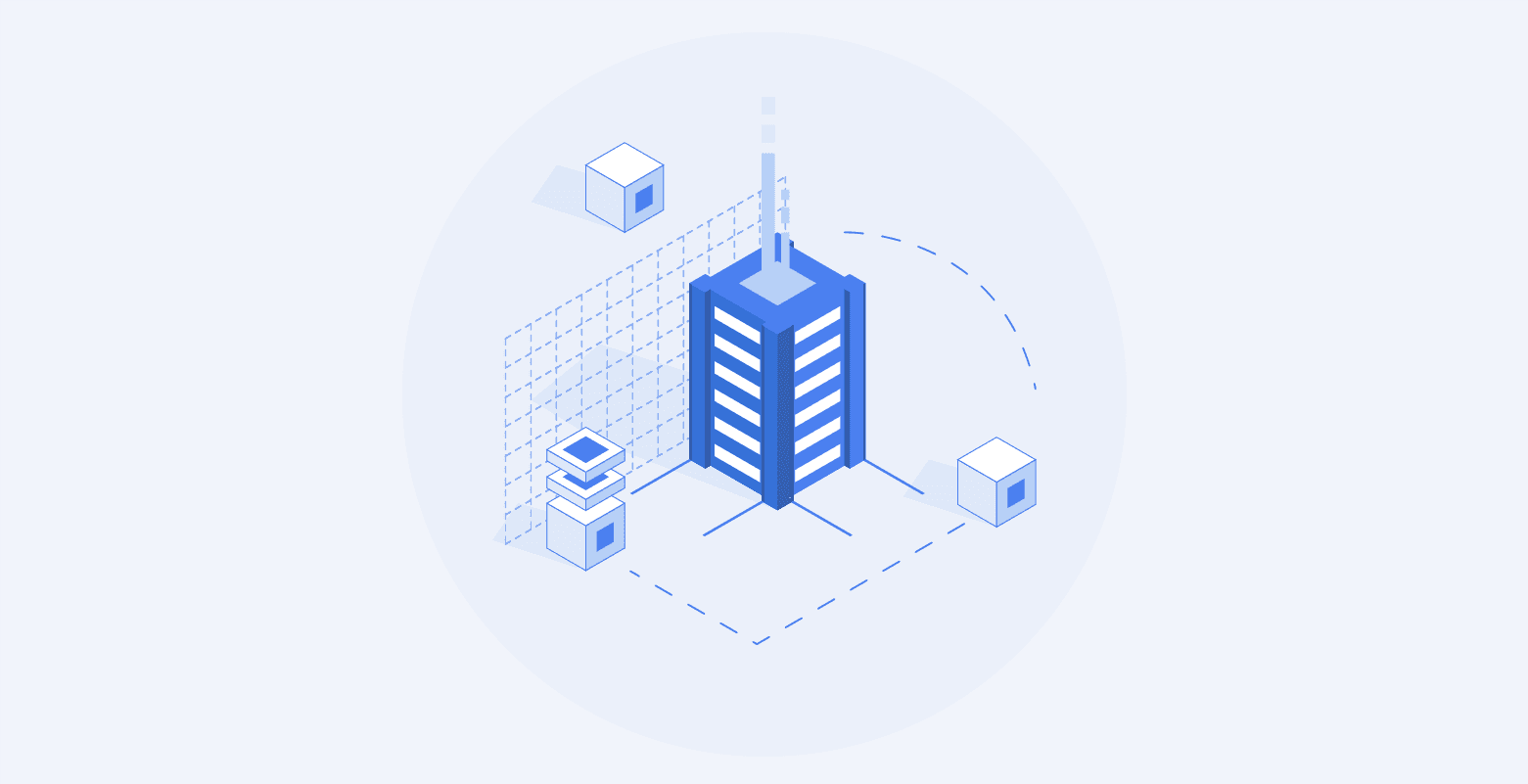
Double-Entry Bookkeeping: What It Is and How It Works
Double-entry accounting is a robust bookkeeping method that has stood the test of time. The double-entry accounting method offers business owners and accountants alike a full financial overview by recording every transaction twice – once as a debit and once as a credit. This time-tested record-keeping system provides an unmatched level of accuracy and transparency, making it the gold standard for businesses seeking to maintain impeccable financial records. We’ll look closer into the intricacies of double-entry accounting, exploring its advantages over single-entry accounting, and uncovering its potential drawbacks. Additionally, we will provide a practical example to illustrate how this accounting method works in real-life scenarios.
What Is Double-Entry Accounting?
Double-entry accounting is a time-honoured bookkeeping system that operates on the principle of recording every financial transaction twice, ensuring that each debit entry or cash account amount is matched with a corresponding credit entry. This meticulous approach provides a holistic view of a company’s financial health, offering business owners and accountants alike a clear and accurate representation of their business and financial standing.
Double-entry accounting, also known as double-entry bookkeeping, stands in stark contrast to single-entry accounting, which only records transactions once. This simplified method, while less complex, is prone to errors and omissions, potentially leading to discrepancies and inaccuracies in financial reporting. In contrast, double-entry accounting’s dual recording system acts as an internal control mechanism, ensuring the integrity and reliability of financial data. Additionally, double-entry accounting complies with generally accepted accounting principles (GAAP), which ensures accurate financial reporting.
The foundation of double-entry accounting lies in the fundamental accounting equation: Assets = Liabilities + Owner’s Equity. This equation serves as the guiding principle for every transaction recorded in the general ledger. When a transaction occurs, it simultaneously affects at least two accounts, with the debit entry increasing one account and the credit entry increasing another. This balancing act maintains the equilibrium of the accounting equation, ensuring that the financial records remain accurate and consistent.
The benefits of double-entry accounting are numerous. It provides an overarching audit trail, allowing accountants and auditors to trace transactions back to their source documentation, enhancing the transparency and accountability of financial records. By capturing both sides of every transaction, double-entry accounting facilitates the preparation of financial statements, including the balance sheet, income statement, and statement of cash flows. These statements serve as vital tools for decision-making, enabling businesses to assess their financial performance, profitability, and cash flow management.
Understanding Double-Entry Accounting
In accounting, double-entry accounting is a key method that changed how financial transactions are recorded and analysed. It operates on the fundamental principle that every business transaction always has two inseparable aspects: a debit and a credit. Debits signify an increase in assets or expenses, while credits represent an increase in liabilities, equity, or revenue. Debits increase asset and expense accounts, while credits decrease them. For example, money paid towards a bank loan is recorded as a debit to the expense accounts, and receiving payment for a service provided is recorded as a credit to the revenue account. This meticulous approach ensures that the accounting equation, a sacred balance of Assets = Liabilities + Owner’s Equity, remains perpetually in harmony.
Picture this: a company embarks on a transaction, purchasing inventory on credit for a substantial sum of $10,000. In the double-entry accounting realm, this transaction unfolds as a debit of $10,000 to the inventory asset account, reflecting the influx of new goods. Simultaneously, a credit of $10,000 graces the accounts payable liability account, acknowledging the debt incurred. This intricate dance of debits and credits ensures that the company’s total assets rise by $10,000, while its liabilities mirror this increase, preserving the equilibrium of the financial accounting equation.
The magic of double-entry accounting is in how it carefully records every financial transaction, tracking all money coming in and going out. It empowers businesses to meticulously track their income and expenses, keeping a watchful eye on their assets and liabilities. Armed with this all-encompassing and more complete financial picture and panorama, decision-makers gain invaluable insights into the company’s overall financial
Double-entry accounting serves as the bedrock for crafting essential financial statements: the balance sheet, a snapshot of a company’s financial position; the income statement, a chronicle of its financial performance; and the statement of cash flows, a detailed account of its cash movements. These statements, meticulously crafted using double-entry accounting principles, form the cornerstone of informed decision-making, financial reporting, and regulatory compliance.
Essentially, double-entry accounting is the linchpin of modern accounting systems, a method that instils confidence in the accuracy, consistency, and reliability of financial records. Its systematic approach empowers businesses to make better decisions, navigate financial complexities, and meet regulatory obligations, ensuring their continued success in the ever-evolving economic landscape.
Types of Business Accounts
The intricate system of double-entry accounting encompasses a variety of business accounts, each meticulously designed to capture and categorise financial transactions. These accounts fall into five broad categories: assets, liabilities, equity, income accounts revenue, and expenses.
Assets are the resources owned by a business and include tangible assets such as cash, inventory, and equipment, as well as intangible assets like patents and trademarks. Liabilities, on the other hand, represent the financial obligations of a business, including loans, accounts payable, and mortgages.
Equity accounts reflect the ownership interest in a business. This includes the initial investment made by the business owners, as well as retained earnings, which in asset accounts are the profits that have been reinvested in the business.
Revenue accounts record the income generated by a business from its primary operations, such as sales of goods or services. Expenses and expense accounts, conversely, capture the costs incurred by a business in generating revenue, including salaries, rent, and utilities.
The meticulous classification of financial transactions into these distinct accounts allows for the preparation of financial statements, including the balance sheet, income statement, and statement of cash flows. These detailed financial statements provide invaluable insights into the financial health of a business, enabling stakeholders to make informed decisions and navigate the ever-changing business landscape.
The Double-Entry Accounting System
The double-entry accounting system is the backbone of double-entry bookkeeping. This method of recording financial transactions ensures that each transaction is recorded twice, once as a debit and once as a credit. This double-entry bookkeeping system creates a system of checks and balances that ensures the accuracy and integrity of the financial records.
In the double-entry accounting system, every transaction affects at least two accounts. When a debit is made to one account, a corresponding credit is made to another account. This ensures that the accounting equation (Assets = Liabilities + Owner’s Equity) is always in balance.
For example, let’s say a company purchases $1,000 worth of inventory on credit. The inventory account would be debited for $1,000, and the accounts payable or credit account, would be credited for $1,000. This transaction increases the company’s assets (inventory) and liabilities (accounts payable), while keeping the accounting equation in balance.
The double-entry accounting system also provides a clear audit trail for all financial transactions. By tracking each transaction from its origin to its final destination, auditors can easily verify the accuracy of the financial records. This helps to protect businesses from fraud and other financial irregularities.
In addition to its accuracy and transparency, the double-entry accounting system also provides valuable insights into a company’s financial performance. By analysing the debits and credits in each account, businesses can identify trends and patterns that can help them make informed decisions about their operations.
The double-entry accounting system is a powerful tool that helps businesses manage their finances effectively. By providing accurate, transparent, and insightful financial records, double-entry and accounting software helps businesses make informed decisions and achieve their financial goals.
Example of Double-Entry
Let’s consider a simple example to illustrate how double-entry accounting works. Suppose a company sells goods worth $1,000 to a customer on credit. In this transaction, the company’s sales revenue increases by $1,000, and its accounts receivable (an asset) also increases by $1,000. To record this transaction in double-entry bookkeeping, the company would make the following journal entries:
Debit: Accounts Receivable $1,000
Credit: Sales Revenue $1,000
Now, let’s assume the company purchases inventory worth $500 from a supplier on credit. This transaction results in two entries: an increase in the company’s inventory (an asset) and an increase in its accounts payable (a liability). The journal entries for this transaction would be:
Debit: Inventory $500
Credit: Accounts Payable $500
Finally, suppose the company pays $200 in cash to a creditor to a bank loan to settle its accounts payable. This transaction decreases the company’s accounts payable and its cash (an asset). The journal entries for this transaction would be:
Debit: Accounts Payable $200
Credit: Cash $200
As you can see from this example, each transaction is recorded twice, once as a debit and once as a credit. This ensures that the credit side of the accounting equation (Assets = Liabilities + Owner’s Equity) remains in balance.
What Is the Difference Between Single-Entry Accounting and Double-Entry Accounting?
Within bookkeeping, there exists a dichotomy of two accounting entry methods: single-entry bookkeeping and its more intricate counterpart, double-entry accounting. While both serve the purpose of recording financial transactions, they diverge in their approach and complexity.
Single-entry bookkeeping, as its name suggests, records each transaction only once. It is a simpler, time-efficient system suitable for small businesses with limited accounting knowledge and resources. This method involves tracking income and expenses without delving into the intricacies of assets, liabilities, and equity. Though straightforward, it lacks the comprehensive nature of its double-entry counterpart.
Double-entry accounting, on the other hand, is a meticulous system that records every transaction twice, ensuring a balanced equation of Assets = Liabilities + Owner’s Equity. This method necessitates a deeper understanding of accounting principles and requires more time and effort to maintain. However, its advantages far outweigh its complexities.
The double-entry accounting system provides a comprehensive and complete picture of a company’s financial health by meticulously categorising transactions into various accounts. These accounts encompass assets, liabilities, equity, revenue, and expenses, each serving a specific purpose in capturing the financial intricacies of a business.
The meticulous nature of double-entry accounting extends to the preparation of financial statements. The balance sheet, income statement, and statement of cash flows are all products of this system, offering invaluable insights into a company’s financial performance and position. Stakeholders rely on these statements to make informed decisions and navigate the ever-changing business landscape.
In contrast, single-entry accounting falls short in providing such comprehensive information. While it may suffice for basic bookkeeping needs, it lacks the depth and accuracy required for businesses seeking a thorough understanding of their financial health.
In essence, double-entry accounting stands as the superior choice for businesses seeking accuracy, transparency, and a comprehensive analysis of their financial performance. Its intricate nature may require more effort, but the benefits it provides are invaluable in the pursuit of financial success and sustainable growth.
The Disadvantage of the Double-Entry Accounting System?
Double-entry accounting is widely regarded as the gold standard for bookkeeping, but it is not without its drawbacks. One significant disadvantage is its complexity compared to single-entry accounting. Double-entry accounting requires a higher level of accounting knowledge and expertise to implement and manage effectively. This can be a challenge for small businesses or organisations with limited accounting resources.
Another disadvantage of double-entry accounting is its time-consuming nature. Recording each transaction twice, along with the need for regular balancing and reconciliation, can be more labour-intensive than single-entry accounting. This can lead to increased operational costs and potential delays in financial reporting.
Furthermore, double-entry accounting is more prone to human error due to its intricate nature. Errors in recording transactions or other bookkeeping errors in balancing accounts can have significant impacts on the accuracy and reliability of financial statements. Detecting and correcting these errors can be a time-consuming and challenging process.
Finally, the complexity of double-entry accounting can make it more difficult to track down errors and irregularities. This can be particularly problematic in cases of fraud or financial mismanagement. Auditors and investigators may require additional time and effort to untangle complex transactions and identify discrepancies.
Despite these disadvantages, double-entry accounting remains the preferred choice for businesses seeking accurate and reliable financial reporting. Its advantages, such as providing a comprehensive view of financial health and ensuring the integrity of financial records, outweigh the challenges associated with its implementation and maintenance.
LIKE.TG’s Financial Services CRM
is a cloud-based platform specifically designed to cater to the unique needs of financial institutions. By leveraging the power of LIKE.TG’s cutting-edge technology, this comprehensive suite of tools empowers financial service providers to effectively manage customer relationships, streamline processes, and significantly enhance overall efficiency.
One of the key strengths of LIKE.TG’s Financial Services CRM lies in its robust client management capabilities. This feature enables financial institutions to centralise and organise all relevant customer information, including personal details, financial profiles, and interaction history, in a single, easily accessible platform. This streamlined approach facilitates efficient customer service, allowing financial advisors to gain a comprehensive understanding of their client’s needs and deliver personalised financial solutions.
Another notable aspect of LIKE.TG’s Financial Services CRM is its effective opportunity management module. This module assists financial institutions in identifying, tracking, and managing sales opportunities throughout the entire sales cycle. By providing a structured framework for managing leads, nurturing prospects, and closing deals, this feature empowers financial advisors to optimise their sales efforts and maximise revenue potential.
Furthermore, LIKE.TG’s Financial Services CRM offers advanced reporting capabilities that enable financial institutions to gain valuable insights into their business performance. Through customisable reports and dashboards, financial institutions can analyse key metrics such as sales performance, customer satisfaction, and operational efficiency. This data-driven approach supports informed decision-making, allowing financial institutions to identify areas for improvement and implement effective strategies for growth.
By leveraging LIKE.TG’s Financial Services CRM, financial institutions can transform their operations and deliver exceptional customer experiences. With its comprehensive suite of tools and powerful functionality, LIKE.TG empowers financial service providers to achieve greater efficiency, enhance customer engagement, and drive business growth in the competitive financial services industry.
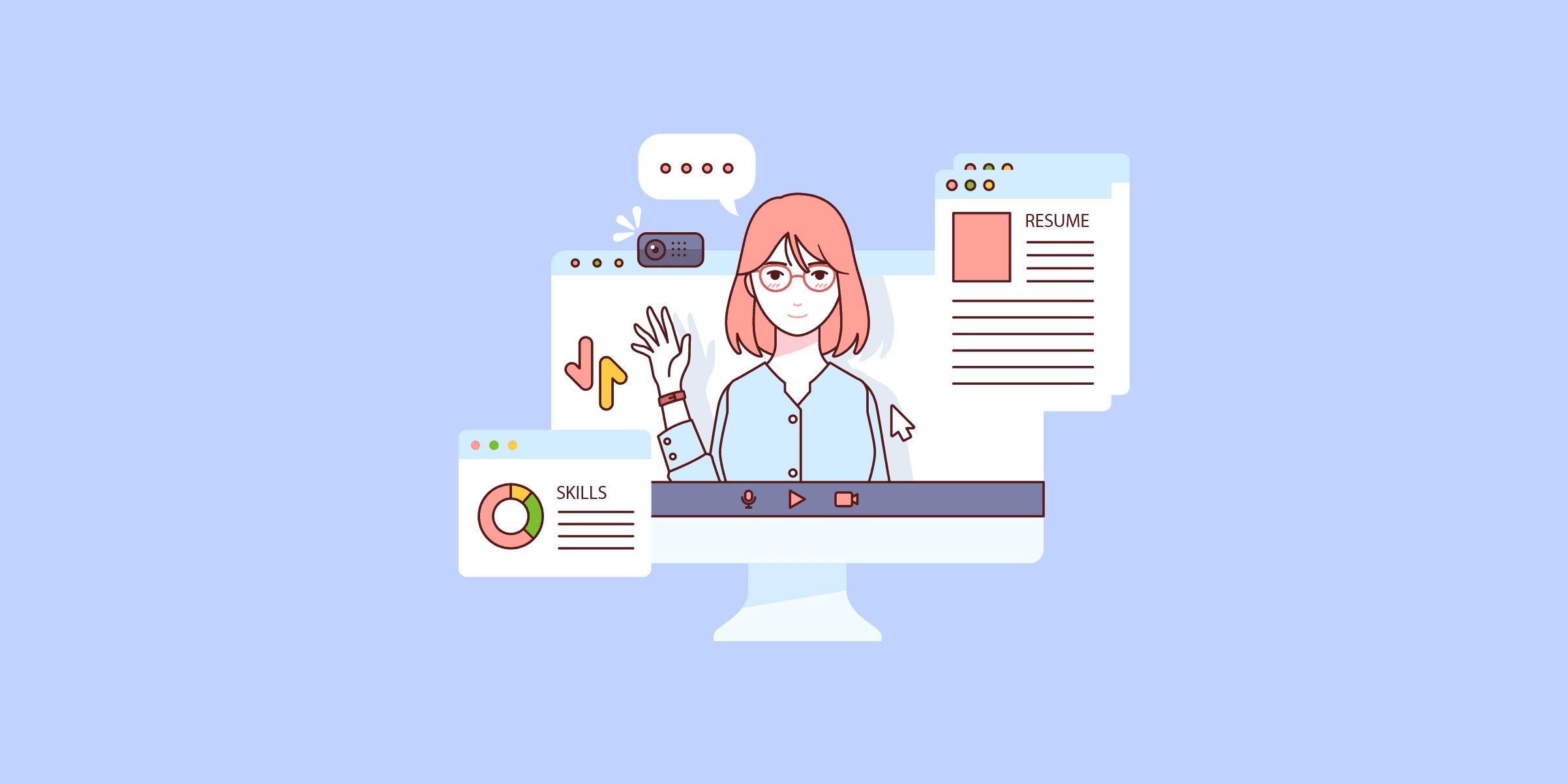
What is Big Data and Why Does it Matter?
Today we are constantly generating and consuming vast amounts of data. From social media posts and online transactions to sensor data and scientific research, the volume, variety, and velocity of data are growing exponentially. This phenomenon is known as big data. In this article, we will explore the concept of big data, its origins, and its significance in the modern world. We’ll also look closely into how big data works, provide real-world examples of its applications, and discuss its impact on various industries and sectors. Finally, we will look ahead to the future of big data and its potential to transform our lives and businesses even further.
What is big data?
There’s an overwhelming influx of data that characterises our daily interactions. This phenomenon, commonly referred to as big data, encompasses a vast and ever-growing collection of information. It extends beyond the traditional structured data found in relational databases to include unstructured data such as social media posts, sensor data, and weblogs. The sheer volume, variety, and velocity of this data present both challenges and opportunities for businesses and organisations.
The term “big data” was coined in the early 21st century to describe the exponential growth and complexity of data. Its defining characteristics are often summarised by the three Vs: Volume, Velocity, and Variety. Volume refers to the immense quantity of data generated daily. Velocity pertains to the rapid speed at which this data is produced and processed. Variety encompasses a diverse range of data formats, including structured, unstructured, and semi-structured data.
The sources of big data are as varied as the data itself. Social media platforms, e-commerce transactions, sensor networks, and scientific research contribute to this ever-expanding pool of information. The proliferation of smartphones, IoT (Internet of Things) devices, and cloud computing has further accelerated the growth of big data.
History of big data
The history of big data is relatively short, but it has already had a profound impact on the way we live and work. In the early days of computing, data was scarce and expensive to store. In the early days, raw data was often collected and stored without much processing, making it challenging to derive meaningful insights. As a result, businesses and organisations were forced to be very selective about the data they collected and stored. However, with the advent of cheaper storage and more powerful computers, it became possible to collect and store vast amounts of data. This led to the rise of big data.
The term “big data” was first coined in 2005 by Roger Mougalas. Mougalas used the term to describe the massive amounts of data that were being generated by the Internet and other digital sources. He argued that this data could be used to gain valuable insights into human behaviour and to improve decision-making.
In the years since Mougalas coined the term, big data has become a major force in business, government, and society. Big data is used to improve customer service, develop new products and services, and make better decisions. It is also used to study human behaviour, track disease outbreaks, and fight crime.
The potential of big data is enormous. However, there are also challenges associated with big data. One challenge is the sheer volume of data that is available. This data can be difficult to store, process, and analyse. Another challenge is the privacy of big data. Big data can be used to track people’s movements, habits, and preferences. This information can be used for good, but it can also be used for malicious purposes.
Despite the challenges, the potential of big data is too great to ignore. Big data is changing the world, and it is important to understand how it works and how it can be used.
Data-driven innovation
Data-driven innovation is the process of using big data analytics to analyse data and derive insights for informed decision-making. This can help organisations improve efficiency and productivity, develop new products and services, and improve customer service.
Data scientists and analysts play a crucial role in analysing data to uncover trends and patterns that can drive business decisions.
One example of data-driven innovation is the use of big data analytics to improve customer service. By analysing customer data, businesses can identify trends and patterns in customer behaviour. This information can then be used to develop targeted marketing campaigns, improve customer service strategies, and develop new products and services that meet the needs of customers.
Another example of data-driven innovation is the use of big data analytics to improve healthcare. By analysing patient data, healthcare providers can identify trends and patterns in patient health. This information can then be used to develop personalised treatment plans, improve patient outcomes, and reduce healthcare costs.
The potential of data-driven innovation is enormous. By harnessing the power of big data, businesses and organisations can improve their operations, develop new products and services, and make better decisions.
However, there are also challenges associated with data-driven innovation. One challenge is the sheer volume of data that is available. Another challenge is the privacy of big data. Businesses and organisations need to be careful about how they collect, store, and use big data. They need to make sure that they are protecting the privacy of their customers and employees.
Despite the challenges, data-driven innovation is a powerful tool that can help businesses and organisations improve their operations and make better decisions. By harnessing the power of big data, businesses and organisations can gain a competitive advantage and achieve success.
How Big Data Works with Structured and Unstructured Data
In order to understand big data, it’s important to know how it works. A data lake is often used to store unstructured big data, allowing for flexible data management and quick access. The big data process can be broken down into five key steps: data collection, data storage, data processing, data analysis, and data visualisation.
The first step in the big data process is data collection. This involves gathering data from a variety of sources, such as sensors, social media, and customer transactions. Once the data has been collected, it needs to be stored in a way that makes it easy to access and analyse. This is where data storage comes in.
The next step is data processing. This involves cleaning and preparing the data to ensure data quality, which may include removing duplicate data and correcting errors. This may involve removing duplicate data, correcting errors, and converting the data into a format that is compatible with the analysis tools that will be used.
Once the data has been processed, it can be analysed to identify patterns and trends. This involves using statistical and machine-learning techniques to identify patterns and trends in the data. This information can then be used to make informed decisions about everything from product development to marketing strategies.
The final step in the big data process is data visualisation. This involves presenting the results of the data analysis in a way that is easy to understand. This may involve creating charts, graphs, and other visual representations of the data.
Big data examples
Big data is being used by businesses across a wide range of industries to improve their operations and deliver better customer experiences. Here are a few examples:
Retail: Big data is used by retailers to track customer purchases, analyse customer behaviour, and develop targeted marketing campaigns. Retailers use big data analysis to uncover customer preferences and optimise inventory management. This information can be used to improve the shopping experience, increase sales, and reduce costs.
Healthcare: Big data is used by healthcare providers to improve patient care, reduce costs, and develop new treatments. Healthcare providers, as business users, leverage big data to enhance patient care and operational efficiency. This information can be used to identify patients at risk for certain diseases, develop personalised treatment plans, and track the effectiveness of treatments.
Finance: Big data is used by financial institutions to detect fraud, assess risk, and develop new financial products. This information can be used to protect customers from financial crime, improve the efficiency of financial transactions, and develop new investment opportunities.
Transportation: Big data is used by transportation companies to improve logistics, reduce costs, and improve safety. Big data helps transportation companies in resource management by optimising routes and reducing fuel consumption. This information can be used to optimise shipping routes, track the location of vehicles, and predict traffic patterns.
Manufacturing: Big data is used by manufacturers to improve quality control, reduce costs, and develop new products. This information can be used to identify defects in products, optimise production processes, and develop new products that meet the needs of customers.
These are just a few examples of how big data is being used by businesses to improve their operations and deliver better customer experiences. As the volume, velocity, and variety of data continue to grow, we can expect to see even more innovative and groundbreaking uses of big data in the years to come.
Big Data Technologies in Today’s World
Big data has become an integral part of our daily lives and has revolutionised the way we interact with technology, businesses, and information. In today’s world, the amount of data created every day is simply mind-boggling. According to recent estimates, the global data creation is a staggering 2.5 quintillion bytes of data every single day, and this number is only expected to grow exponentially in the years to come.
The impact of big data can be seen across various industries and sectors. For instance, in the healthcare sector, big data is used to improve patient care, reduce costs, and develop new treatments. By analysing vast amounts of patient data, healthcare providers can identify trends and patterns, leading to more personalised treatment plans and better patient outcomes. Similarly, in the financial industry, big data plays a crucial role in detecting fraud, assessing risk, and developing innovative financial products.
The retail industry also leverages big data to enhance customer experiences and drive sales. By tracking customer purchases, analysing customer behaviour, and developing targeted marketing campaigns, retailers can gain valuable insights into consumer preferences and provide more personalised services. Big data also plays a significant role in the manufacturing industry, where it is used to improve quality control, reduce costs, and develop new products.
Furthermore, the entertainment industry has embraced big data to create more engaging and personalised experiences for consumers. By analysing user data, content providers can tailor recommendations, improve streaming quality, and develop new content that resonates with their audience.
The growth of the Internet of Things (IoT) has further amplified the significance of big data. With billions of devices connected to the internet, from smartphones and smartwatches to industrial sensors and home appliances, the volume of data generated is immense. This data holds valuable insights into consumer behaviour, operational efficiency, and asset tracking, enabling businesses to make informed decisions and optimise their operations.
The world of big data continues to evolve rapidly, presenting both opportunities and challenges for businesses and organisations. Harnessing the power of big data effectively requires robust data management strategies, advanced analytics capabilities, and a commitment to data privacy and security. By embracing big data and leveraging its potential, businesses can gain a competitive edge, drive innovation, and transform their operations.
Future of Big Data and Machine Learning
The future of big data is bright. As the amount of data in the world continues to grow, so too will the need for tools and technologies to process and analyse it. This growth will create new opportunities for businesses and organisations of all sizes to use big data to improve their operations, develop new products and services, and make better decisions.
One of the most important developments in the future of big data will be the continued growth of artificial intelligence (AI) and machine learning (ML). These technologies are already being used to automate many of the tasks associated with big data processing and analysis, and they will become even more powerful in the years to come. As AI and ML become more sophisticated, they will be able to identify patterns and trends in data that are invisible to the human eye. This will allow businesses and organisations to make even better decisions and to develop new products and services that are tailored to the needs of their customers.
Another important development in the future of big data will be the increasing use of data visualisation tools. These tools make it possible to present big data in a way that is easy to understand and interpret. This will allow businesses and organisations to communicate the results of their big data analyses to their stakeholders in a way that is clear and concise.
Finally, the future of big data will also see an increasing focus on data privacy and security. As more and more data is collected and stored, it is important to ensure that it is protected from unauthorised access and use. Businesses and organisations will need to invest in data security measures to protect their data from cyberattacks and other threats.
The future of big data is full of potential. As the amount of data in the world continues to grow, so too will the opportunities for businesses and organisations to use it to improve their operations, develop new products and services, and make better decisions.
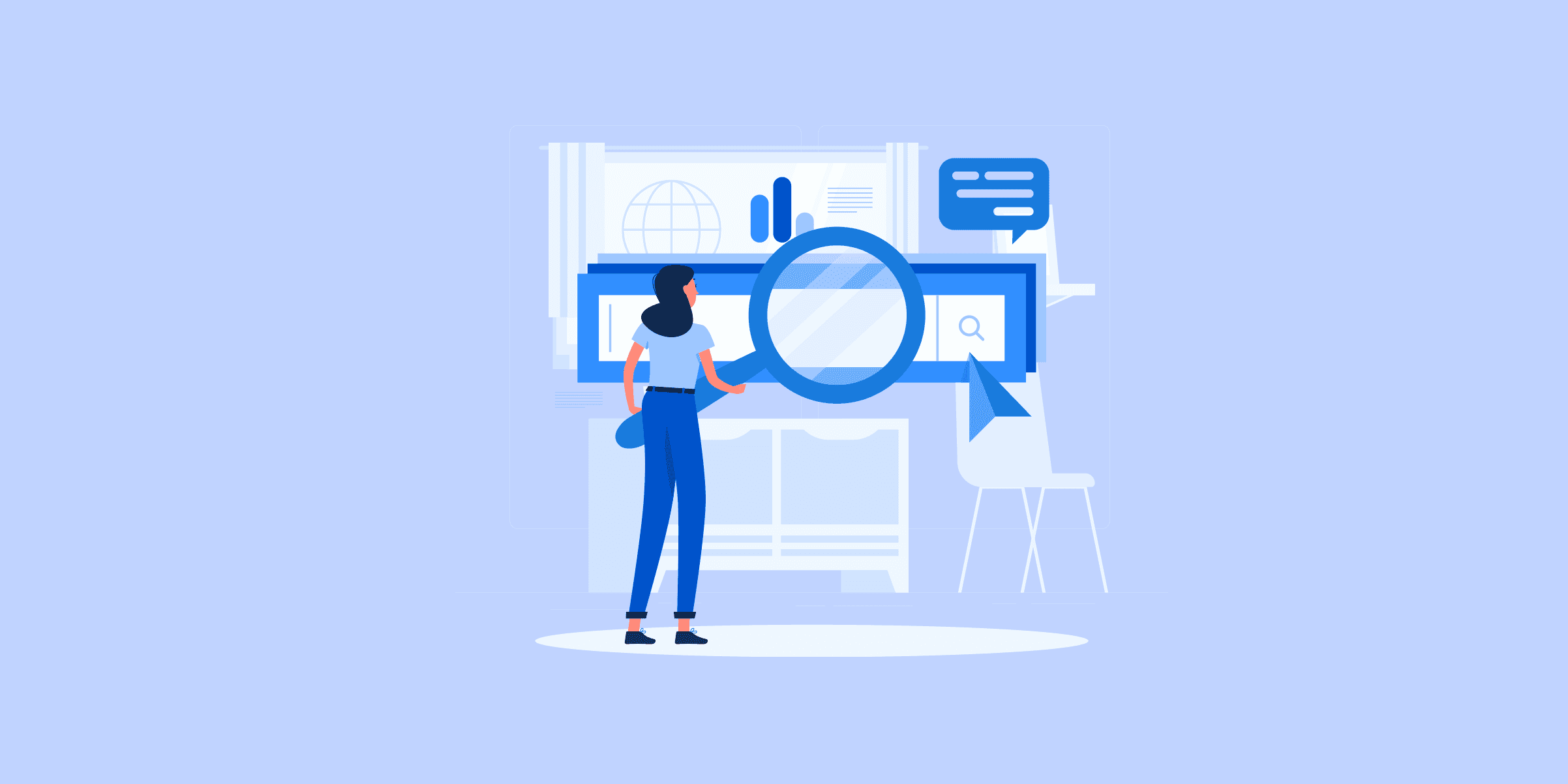
What are Wealth Management Services?
In personal finance, the concept of wealth management often arises as a crucial aspect of securing financial stability and achieving long-term goals. It encompasses a comprehensive range of advisory services tailored to individuals and families, aiming to effectively manage and grow their financial resources. We’ll look into the intricacies of wealth management, exploring its diverse services, advantages, and strategies employed by wealth managers to help clients navigate the complexities of financial planning, investment management, and estate planning. From understanding the types of wealth management services available to gaining insights into the selection process of a wealth manager, this overarching guide provides valuable information to empower individuals in making informed decisions about their financial wellbeing.
What is wealth management?
Wealth management services are meticulously tailored to each client’s unique circumstances and aspirations. Investment management lies at the core of this service, where experts strategically allocate financial assets to optimise returns while mitigating risks. Through careful portfolio construction and ongoing market monitoring, wealth managers ensure that investment strategies remain aligned with evolving financial goals.
Financial planning looks into the intricacies of an individual’s financial life, encompassing cash flow analysis, debt management, education planning, retirement planning, and insurance planning. This approach ensures that every financial situation and aspect is meticulously considered and optimally managed, creating a roadmap towards financial stability and prosperity.
Estate planning, an essential component of wealth management, focuses on preserving and distributing an individual’s assets during their lifetime and beyond. Through the creation of wills, trusts, and other legal instruments, wealth managers ensure that an individual’s wishes are meticulously documented and executed, safeguarding the financial wellbeing of their loved ones and preserving their legacy for generations to come.
Wealth management is an invaluable service for individuals and families seeking to cultivate, preserve, and grow their financial resources. By partnering with experienced wealth managers, clients gain access to personalised financial strategies, expert guidance, specialist advice, and unmatched proficiency in navigating the complexities of financial planning, investment management, and estate planning. This collaborative endeavour empowers individuals to confidently pursue their financial aspirations, ensuring a secure financial foundation for themselves and their loved ones.
Types of wealth management services
Wealth management services provide a thorough array of financial solutions designed to cater to the unique needs and aspirations of individuals and families. These services encompass four primary domains: financial planning, investment management, estate planning, and tax planning.
The Financial Industry Regulatory Authority (FINRA) plays a crucial role in overseeing professional standards and credentials in wealth management.
Financial planning is essential in wealth management as it offers a structured approach to achieving financial objectives. It entails a thorough assessment of an individual’s current financial standing, identification of short-term and long-term goals, and the formulation of a customised roadmap to realise those aspirations. This financial planner also encompasses budgeting strategies, debt management, retirement planning, education funding, and risk mitigation strategies.
Investment management plays a pivotal role in wealth management, focusing on the strategic allocation and management of assets to foster wealth growth. Wealth managers construct diversified investment portfolios tailored to each client’s risk tolerance, investment horizon, and financial goals. They exercise constant vigilance over market dynamics, making judicious adjustments to the portfolio to optimise returns while minimising potential risks.
Estate planning is an essential component of wealth management, ensuring the orderly transfer of assets during a client’s lifetime and beyond. It involves the creation of legal instruments, such as wills, trusts, and powers of attorney, to delineate the distribution and management of assets. Estate planning also addresses crucial matters like minimising estate taxes, preserving family wealth, and safeguarding the financial security of heirs.
Tax planning is an integral aspect of wealth management, aimed at minimising tax liabilities and maximising after-tax income. Wealth managers meticulously analyse clients’ tax situations and devise strategies to optimise tax efficiency. This may involve leveraging tax-advantaged investment vehicles, maximising deductions and credits, and anticipating the potential tax implications of various financial transactions.
By seamlessly integrating these services, wealth managers empower clients with a holistic approach to managing their financial resources and achieving their long-term financial aspirations. They serve as trusted advisors, providing personalised guidance, profound expertise, and unwavering support assisting clients to navigate the complexities of wealth management and make informed financial decisions.
Advantages of wealth management
Wealth management offers a range of advantages that can significantly benefit individuals and families seeking to achieve their financial goals. Firstly, a wealth management team provides clients with personalised financial advice tailored to their unique circumstances and aspirations. Through extensive financial planning, wealth managers assess clients’ financial situations, identify their goals, and develop customised strategies to help them achieve those goals. This personalised approach ensures that clients receive guidance that is aligned with their specific needs and priorities.
Secondly, wealth management services provide clients with access to professional expertise and knowledge in managing complex financial matters. Wealth managers possess extensive knowledge of financial markets, investment strategies, and tax regulations, enabling them to make informed decisions on behalf of their clients. This expertise is particularly valuable for individuals who may lack the time, knowledge, or experience to effectively manage their own finances.
Thirdly, wealth management offers clients peace of mind and financial security. By entrusting their financial affairs to experienced wealth managers, individuals and families can alleviate the stress and anxiety associated with managing their finances. Wealth managers continuously monitor clients’ portfolios, ensuring that they remain aligned with their financial goals and risk tolerance. This ongoing monitoring and management provide clients with the assurance that their financial future is in capable hands.
Fourthly, wealth management services can help clients achieve greater financial efficiency and optimisation. Wealth managers employ sophisticated financial planning techniques and strategies to maximise clients’ returns while minimising risks. They also assist clients in optimising their tax liabilities, ensuring that they retain more of their hard-earned income. This financial efficiency can significantly contribute to clients’ overall financial wellbeing and long-term financial success.
In conclusion, wealth management offers a multitude of advantages for individuals and families seeking to achieve their financial goals. Through personalised advice, professional expertise, peace of mind, and financial efficiency, wealth management services provide clients with the support and guidance needed to navigate the complex world of finance and secure their financial future.
How to choose a wealth manager
When selecting a wealth manager, it’s crucial to consider several key factors to ensure you find a professional who aligns with your financial needs and goals. One important aspect to consider is the wealth manager’s fiduciary status. A fiduciary is legally obligated to act in the best interests of their clients, prioritising their financial wellbeing above all else. This level of trust and responsibility is essential when entrusting your financial future to a wealth manager.
Experience and qualifications are also vital factors to evaluate. Look for a wealth manager with a proven track record of success in managing and growing wealth. Consider their educational background, professional certifications, and years of experience in the financial industry. A wealth manager with a strong track record and relevant qualifications can provide valuable insights and strategies to help you achieve your financial objectives.
Another important consideration is the wealth manager’s investment philosophy and risk tolerance. Understand their approach to investing and managing risk, and ensure it aligns with your own financial goals and risk appetite. A wealth manager or financial advisor who shares your investment philosophy and understands your risk tolerance can tailor their strategies accordingly, maximising the potential for success.
Fees are another crucial aspect to consider when choosing a wealth manager. Be clear about the fee structure and ensure you understand all associated costs, including management fees, performance fees, and any additional expenses. Transparent fee structures and reasonable rates are essential to ensure your wealth manager’s interests are aligned with yours.
Finally, don’t underestimate the value of references and recommendations. Seek referrals from friends, family, or business associates who have worked with wealth managers in the past. Personal recommendations can provide valuable insights into the wealth manager’s professionalism, expertise, and client service.
By carefully considering these factors, you can select a wealth manager who possesses the experience, qualifications, and values to effectively guide you towards achieving your financial aspirations.
Wealth management fees and costs
Wealth management services typically come with associated fees and costs that vary depending on the complexity of the financial plan, the level of service provided, and the wealth manager’s experience and qualifications. It is crucial for individuals and families to understand these fees and costs before selecting a wealth manager to ensure that they are making an informed decision.
Common types of fees charged by wealth managers include:
Advisory fees: These fees are typically based on a percentage of assets under management (AUM) and cover the ongoing investment advice, and management provided by the wealth manager. The percentage can vary depending on the size of the portfolio and the services included.
Transaction fees: These fees are charged each time a trade is executed on behalf of the client. They may include commissions, bid-ask spreads, and other related costs.
Custodian fees: These fees are an advisory fee charged by the financial institution that holds the client’s assets in custody. They cover the costs of safeguarding and administering the assets.
Performance fees: Some wealth managers charge performance fees based on the investment returns achieved. These fees are typically structured as a percentage of the profits generated above a predetermined benchmark.
Hourly fees: In some cases, private wealth managers may charge an hourly fee for specific services, such as financial planning or estate planning.
It is important to note that fees and costs can vary significantly between wealth managers, so it is essential to compare and understand the fee structures before making a decision. Some wealth managers may offer bundled services that include a combination of fees, while others may charge separately for each service.
Additionally, some wealth managers may require a minimum investment amount or a minimum annual fee, which can limit accessibility for individuals with smaller portfolios. It is advisable to seek clarification on all fees and costs, including any potential hidden charges, before committing to a wealth management service.
Examples of wealth management
Wealth managers provide a wide range of services to help clients manage their wealth effectively. Some common examples of wealth and asset management and services include:
Creating and maintaining an investment portfolio: Wealth managers can help clients build diversified investment portfolios that align with their risk tolerance, investment goals, and time horizon. They can also provide ongoing monitoring and management of the portfolio to ensure it remains aligned with the client’s objectives.
Developing strategies for tax-efficient investing: Wealth managers can help clients develop strategies to minimise their tax liability and maximise their after-tax returns. This may involve utilising tax-advantaged investment vehicles, such as retirement accounts and tax-efficient mutual funds, as well as implementing tax-loss harvesting strategies.
Providing advice on estate planning: Wealth managers can help clients create estate plans that ensure their assets are distributed according to their wishes and that their loved ones are financially secure. This may involve drafting wills, trusts, and powers of attorney, as well as providing guidance on issues such as probate and estate taxes.
Offering guidance on charitable giving: Wealth managers can help clients develop charitable giving strategies that align with their philanthropic goals and maximise their tax benefits. This may involve establishing donor-advised funds, charitable trusts, or private foundations, as well as providing guidance and tax advice on selecting charities and structuring gifts.
Assisting with business succession planning: Wealth managers and financial advisers can help business owners develop plans for the orderly transfer of their business to the next generation or to other owners. This may involve developing buy-sell agreements, creating family-limited partnerships, or implementing other succession planning strategies.
Wealth managers can also provide guidance on specialised areas of wealth management, such as retirement planning, education funding, and insurance planning. By working with a qualified wealth manager, individuals and families can gain access to the expertise and resources they need to achieve their financial goals and secure their financial future.
Strategies of a Wealth Manager
Within wealth management, meticulous strategies are implemented to assist clients in accomplishing their financial aspirations. Diversification stands as a central strategy, aiming to disperse investments across asset classes, industries, and geographic boundaries. This prudent approach to investment strategy seeks to mitigate the impact of market fluctuations, paving the way for sustained long-term growth.
Risk management plays an equally important role, with wealth managers meticulously assessing clients’ risk tolerance and crafting personalised investment strategies aligned with their comfort levels. This may entail employing stop-loss orders, strategic hedging, or incorporating alternative investments like real estate or commodities to fortify against potential losses. Regular vigilance and proactive adjustments ensure that clients’ portfolios remain harmonised with their risk preferences.
Rebalancing emerges as another essential strategy, ensuring that asset allocation remains optimal amidst dynamic market conditions. As investments traverse through varying performance cycles, periodic adjustments are made to restore the portfolio’s balance, aligning it with the target allocation. This prudent approach not only mitigates risk but also seizes emerging growth opportunities.
Tax efficiency holds a prominent position in wealth management, with strategies designed to minimise clients’ tax liabilities. Wealth managers leverage techniques such as tax-loss harvesting, judicious investment placement, and leveraging tax-advantaged accounts. By optimising tax efficiency, clients are empowered to maximise their after-tax returns and preserve a greater portion of their wealth.
Estate planning constitutes an integral component of wealth management, ensuring that clients’ wealth is transferred to heirs in a tax-efficient manner and their wishes are honoured after their passing. This includes crafting trusts, wills, and other legal instruments, as well as collaborating with estate attorneys and other professionals. Through comprehensive estate planning, clients can safeguard their legacies and provide for their loved ones.
These strategies coalesce to form a holistic framework for wealth management, encompassing financial guidance, risk management, and future security. Wealth managers serve as trusted financial advisors throughout, diligently guiding clients toward the realisation of their financial goals and securing their financial wellbeing.
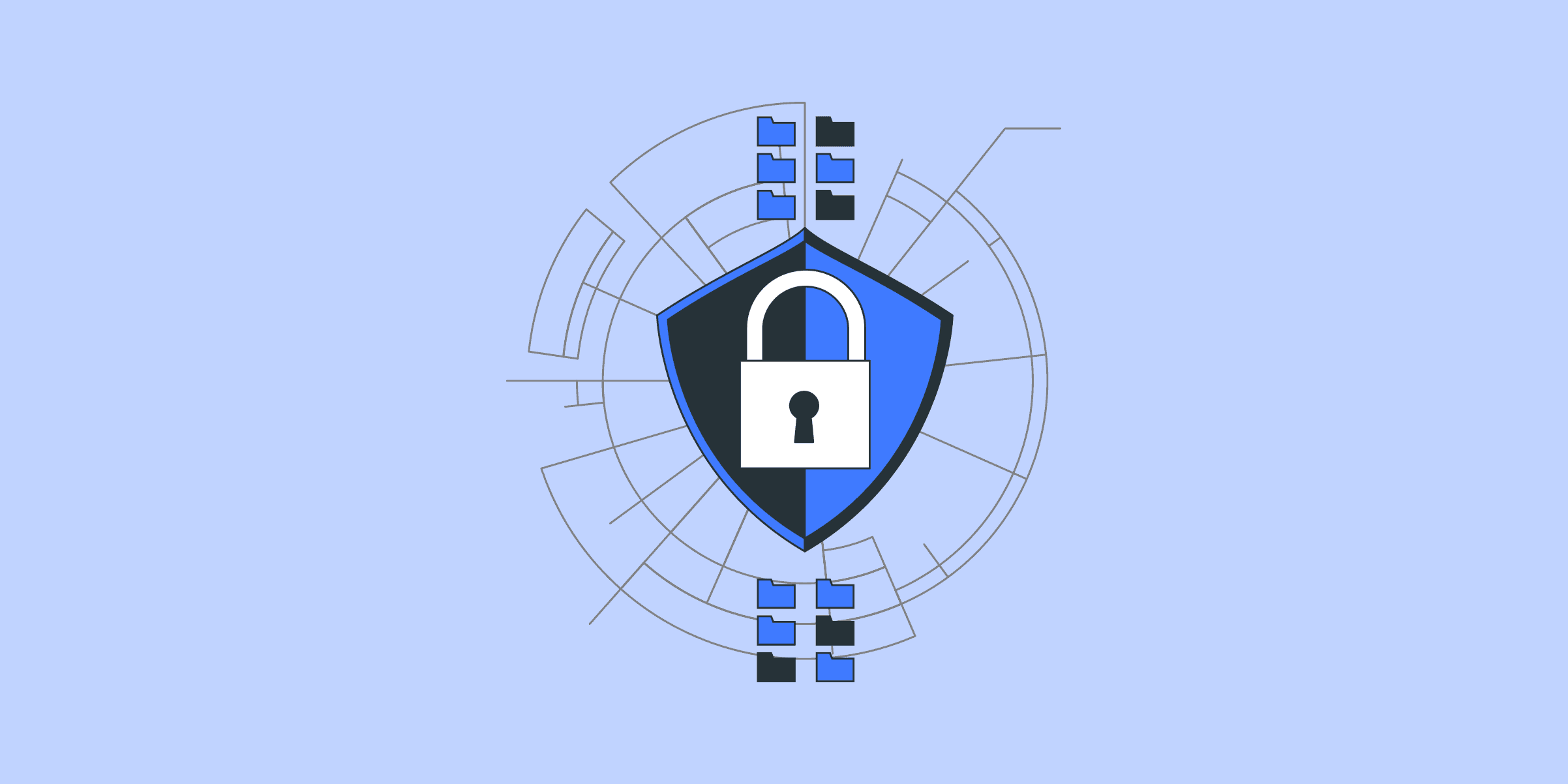
What does asynchronous mean?
Asynchronous communication is quickly becoming the new normal in the digital age. This type of communication allows for more flexibility and convenience, as participants can send and receive messages at their own convenience. It also fosters deeper and more thoughtful responses, as participants have time to reflect and compose their messages. In this article, we will explore the concept of asynchronous communication, its benefits, and how it is used in telecommunication, computer programming, and workplace communication. We will also provide some examples of asynchronous communication and compare it to synchronous communication. Finally, we will discuss how LIKE.TG can help you with the term asynchronous communication.
What is asynchronous communication?
Asynchronous communication is a mode of digital communication, in which the sender and receiver are not engaged in simultaneous, real-time interaction. Unlike synchronous communication, such as phone calls or face-to-face conversations, asynchronous communication does not require the immediate presence or availability of both parties.
In an asynchronous form of communication, the sender can transmit a message without expecting an immediate response from the recipient. This allows for greater flexibility and convenience, as participants can engage in communication at their own convenience, regardless of their location or schedule. Asynchronous communication can take place through various channels, including email, text messaging, instant messaging, social media, and online forums. In contrast, other forms of real-time communication, like texting, e-mail, and Twitter, require immediate or near-immediate responses.
One significant advantage of asynchronous communication is the opportunity it provides for more thoughtful and well-crafted responses. Since participants have time to reflect on their messages before sending them, asynchronous communication often leads to more detailed and insightful conversations. It allows individuals to express their thoughts and ideas more clearly and comprehensively, fostering deeper and more meaningful discussions.
Furthermore, asynchronous communication can enhance productivity by enabling individuals to multitask and prioritise their tasks. It allows them to engage in multiple conversations simultaneously without the pressure of immediate response, leading to more efficient use of time and resources.
However, it’s important to acknowledge the potential drawbacks associated with asynchronous communication. The lack of immediate feedback can sometimes lead to misunderstandings or delays in resolving issues. Additionally, the absence of non-verbal cues, such as body language and tone of voice, can make it challenging to convey emotions and intentions accurately.
Overall, asynchronous communication offers a flexible and convenient alternative to synchronous communication, allowing for more thoughtful and in-depth interactions. While it may have certain limitations, asynchronous communication remains a valuable tool in today’s fast-paced digital landscape, facilitating effective collaboration and knowledge sharing among individuals and teams.
Benefits of asynchronous communication
Asynchronous communication offers a host of advantages over synchronous communication, making it a valuable tool for businesses and individuals alike.
Improved productivity
Asynchronous communication allows employees to work at their own pace and on their own time, which can lead to increased productivity. Employees are not constrained by the need to be available for real-time conversations and can instead focus on completing tasks without distractions. This flexibility can be especially beneficial for employees who have other commitments, such as caregiving or remote work.
Greater flexibility
Asynchronous communication offers greater flexibility than synchronous communication, as it allows employees to communicate from any location and at any time, on their own schedule. This can be a major advantage for businesses with employees who work remotely or travel frequently. Asynchronous communication also makes it easier for employees to collaborate with colleagues in different time zones or countries.
Increased inclusivity
Asynchronous communication can make the workplace more inclusive for employees with disabilities or who are neurodivergent. For example, employees with social anxiety or autism may find it easier to communicate via text or email than in person or over the phone. Asynchronous communication can also level the playing field for employees who are not native speakers of the majority language in the workplace.
Deeper and more thoughtful responses
Asynchronous communication allows participants to take their time and craft thoughtful, well-considered responses. This can lead to deeper and more meaningful conversations, as participants have the opportunity to reflect on their thoughts and feelings before responding. Asynchronous communication can also be beneficial for resolving conflicts, as it allows participants to avoid getting caught up in the heat of the moment.
Asynchronous in telecommunication
Within telecommunication, asynchronous communication refers to the exchange of information between devices or systems without the requirement for real-time interaction. Unlike synchronous communication, where participants engage in a continuous, back-and-forth exchange, asynchronous communication allows for a more flexible and time-independent exchange of messages.
A typical example of asynchronous communication in telecommunication is the exchange of text messages or emails. In this scenario, the sender composes and sends a message, and the recipient retrieves and reads it at their convenience. In contrast, video conferencing is a synchronous communication method where events need to happen in order and over a fixed span of time, unlike asynchronous methods where events can happen at irregular intervals and recipients can respond at their convenience. There is no need for both parties to be available simultaneously, providing greater flexibility in communication.
Asynchronous communication also finds applications in various telecommunication technologies and services. For instance, voicemail systems allow callers to leave recorded messages for the intended recipients to retrieve and listen to later. Similarly, fax machines enable the transmission of documents without requiring the sender and receiver to be online simultaneously.
One key advantage of asynchronous communication in telecommunication is its ability to transcend geographical boundaries and time zones. It enables seamless communication between individuals or entities located in different parts of the world, regardless of their respective time zones. This flexibility makes asynchronous communication particularly suitable for global business operations and remote collaboration.
However, it’s important to acknowledge certain drawbacks associated with asynchronous communication in telecommunication. The absence of real-time client interaction can sometimes lead to delays in resolving urgent matters or addressing time-sensitive issues. Additionally, the lack of immediate feedback or response can hinder effective problem-solving or decision-making processes.
Despite these limitations, asynchronous communication remains a valuable tool in the telecommunication landscape, offering convenience, flexibility, and the ability to bridge geographical distances. It complements synchronous communication methods by providing an alternative means of interaction that suits various communication needs and preferences.
Asynchronous in computer programming
Asynchronous programming is a paradigm that allows different parts of a computer program to run concurrently. In traditional synchronous programming, each statement is executed in sequence, and the next statement cannot start until the current statement has finished executing. This can lead to inefficiencies, especially when dealing with long-running tasks or when multiple tasks need to be coordinated and performed simultaneously.
Asynchronous programming overcomes these limitations by allowing multiple tasks to execute concurrently. This is achieved by using a technique called “event-driven programming,” which involves setting up event handlers that are triggered when certain events occur. For example, if a user clicks a button on a web page, an event handler can be triggered to handle the click event and perform the necessary actions.
Asynchronous programming can significantly improve the performance and responsiveness of computer programs, especially when dealing with user interfaces, network communication, and other I/O-intensive tasks. It also allows for more efficient use of resources, as multiple tasks can be executed concurrently without having to wait for each other to complete.
Some popular examples of asynchronous programming languages include JavaScript, Python, and Ruby. These languages provide built-in support for event-driven programming and offer various libraries and frameworks that facilitate asynchronous programming.
Overall, asynchronous programming is a powerful paradigm that enables efficient and responsive computer programs by allowing multiple tasks to execute concurrently. It is widely used in various applications, including web development, mobile development, and real-time systems.
Asynchronous in workplace communication
Asynchronous work is a valuable tool for workplace communication. It can enable employees to work flexibly, collaborate more effectively, reduce stress and burnout, and improve communication between employees and managers.
One of the key benefits of asynchronous communication is that it allows employees to work on their own schedules. This can be especially beneficial for employees who have other commitments, such as family or school, or who simply prefer to work at their own pace. Asynchronous communication also allows employees to collaborate more effectively, as they can easily share ideas and documents with each other without having to be in the same place at the same time. This can be especially helpful for teams that are spread across different locations or time zones.
In addition, asynchronous communication can help to reduce stress and burnout in the workplace. When employees are not constantly interrupted by phone calls or emails, they can focus better on their work and take breaks when they need them. This can help to improve employee morale and productivity. Finally, asynchronous communication can improve communication between employees and managers. When managers are not able to communicate with their employees in real-time, they have more time to think about their responses and provide more thoughtful feedback. This can help to build trust and rapport between managers and employees.
Of course, asynchronous communication also has some drawbacks. One potential drawback is that it can be difficult to resolve urgent matters or address time-sensitive issues. Additionally, asynchronous communication can sometimes lead to misunderstandings or miscommunications, as employees may not be able to clarify their words or meaning in real-time. However, overall, asynchronous communication can be a valuable tool for workplace communication when used effectively.
Asynchronous examples
Examples of asynchronous communication include sending an email, posting on a message board, leaving a voicemail, sending a text message, and using Slack or Microsoft Teams.
Email is one of the most common forms of asynchronous communication. It allows you to send messages to others at any time, and they can read and respond to them at their convenience. Email is a good way to communicate when you need to share a lot of information or when you want to get feedback from others.
Message boards are another popular form of asynchronous communication. They allow users to post messages and questions on a public forum, and others can read and respond to them. Message boards are a good way to get help from others or to share information with a large group of people.
Voicemail is a good way to communicate when you need to leave a message for someone who is not available to answer the phone. Voicemail messages can be listened to at the recipient’s convenience.
Text messaging is a popular form of asynchronous communication that allows short messages to be sent to others. Text messages are a good way to stay in touch with friends and family or to communicate with co-workers about work-related matters.
Slack and Microsoft Teams are two popular collaboration tools that allow teams to communicate asynchronously. These tools allow users to send messages, share files, and collaborate on projects. Slack and Microsoft Teams are good ways to stay connected with your team and get work done.
Asynchronous communication can be a valuable tool for workplace communication. It can enable employees to work flexibly, collaborate more effectively, reduce stress and burnout, and improve communication between employees and managers. By using asynchronous communication tools, you can improve the productivity and efficiency of your team.
Asynchronous workflows are particularly beneficial for remote teams, as they allow for seamless collaboration across different time zones and locations, transforming parts of the creative process into an asynchronous one.
Asynchronous vs. synchronous
Asynchronous communication is a type of communication in which the sender and receiver are not engaged in real-time communication. It can take place over a variety of channels, including email, text messaging, and social media. Asynchronous communication allows for more flexibility and convenience in communication, as participants can send and receive messages at their own convenience. It can also foster deeper and more thoughtful responses, as participants have time to reflect and compose their messages.
In the context of online education, asynchronous learning offers similar flexibility, allowing students to access instructional materials and complete tasks at their chosen time within a designated time frame. This contrasts with synchronous learning, where students attend class sessions at specific times, engaging in live instruction and participating in real-time discussions and activities. Online classes can be either asynchronous or synchronous, each with its own set of benefits. Asynchronous online classes provide flexibility and the opportunity for immediate feedback, while synchronous online classes offer real-time engagement and interaction.
In a virtual setting, synchronous learning takes place in a digital space where students and instructors interact in real-time, akin to a physical classroom setting.
In contrast, synchronous communication requires both communicating parties to be available at the same time. This type of communication is often used for meetings, phone calls, and video chats. Synchronous communication can be more efficient for time-sensitive matters, as participants can interact and respond to each other immediately. However, it can also be more disruptive, as participants may need to interrupt their other activities to attend to the communication.
The choice between asynchronous and synchronous communication depends on several factors, including the urgency of the matter, the complexity of the information being communicated, and the preferences of the participants. For example, if a message is time-sensitive or requires immediate feedback, synchronous communication may be more appropriate. On the other hand, if the message is not urgent and allows for more thoughtful consideration, asynchronous communication may be a better option.
Asynchronous communication can also be more convenient for participants who are in different time zones or have different work schedules. It allows participants to communicate on their own time, without the need to coordinate schedules. Additionally, asynchronous communication can be more accessible for individuals with disabilities or who prefer written communication.
Overall, asynchronous and synchronous communication have their own advantages and disadvantages, and the best choice depends on the specific operating situation and preferences of the participants.
How LIKE.TG can help you with Asynchronous communication
LIKE.TG provides a comprehensive set of tools and services to facilitate asynchronous communication, enabling businesses to enhance their communication efficiency and productivity. These tools include:
Asynchronous Programming: LIKE.TG offers robust support for asynchronous programming, allowing developers to create applications that can process tasks and operations in the background without hindering the user interface. This enhances application performance and responsiveness, particularly for complex and data-intensive tasks.
Messaging Services: LIKE.TG provides a range of messaging services that enable asynchronous communication between users, customers, and applications. These services include email, chat, and SMS messaging, allowing for seamless and convenient communication.
APIs for Asynchronous Communication: LIKE.TG offers a comprehensive set of APIs that enable developers to integrate asynchronous communication into their custom applications. These APIs include the Messaging API, which facilitates the sending and receiving of messages, and the Streaming API, which enables real-time updates and notifications.
Workflow Automation: LIKE.TG’s workflow automation capabilities allow businesses to automate repetitive and time-consuming tasks, such as sending follow-up emails or generating reports. This automation enhances efficiency and frees up employees to focus on more strategic and value-added activities.
By leveraging these tools and services, LIKE.TG empowers businesses to embrace asynchronous communication, fostering collaboration, productivity, and customer engagement while optimising resource utilisation and reducing costs.
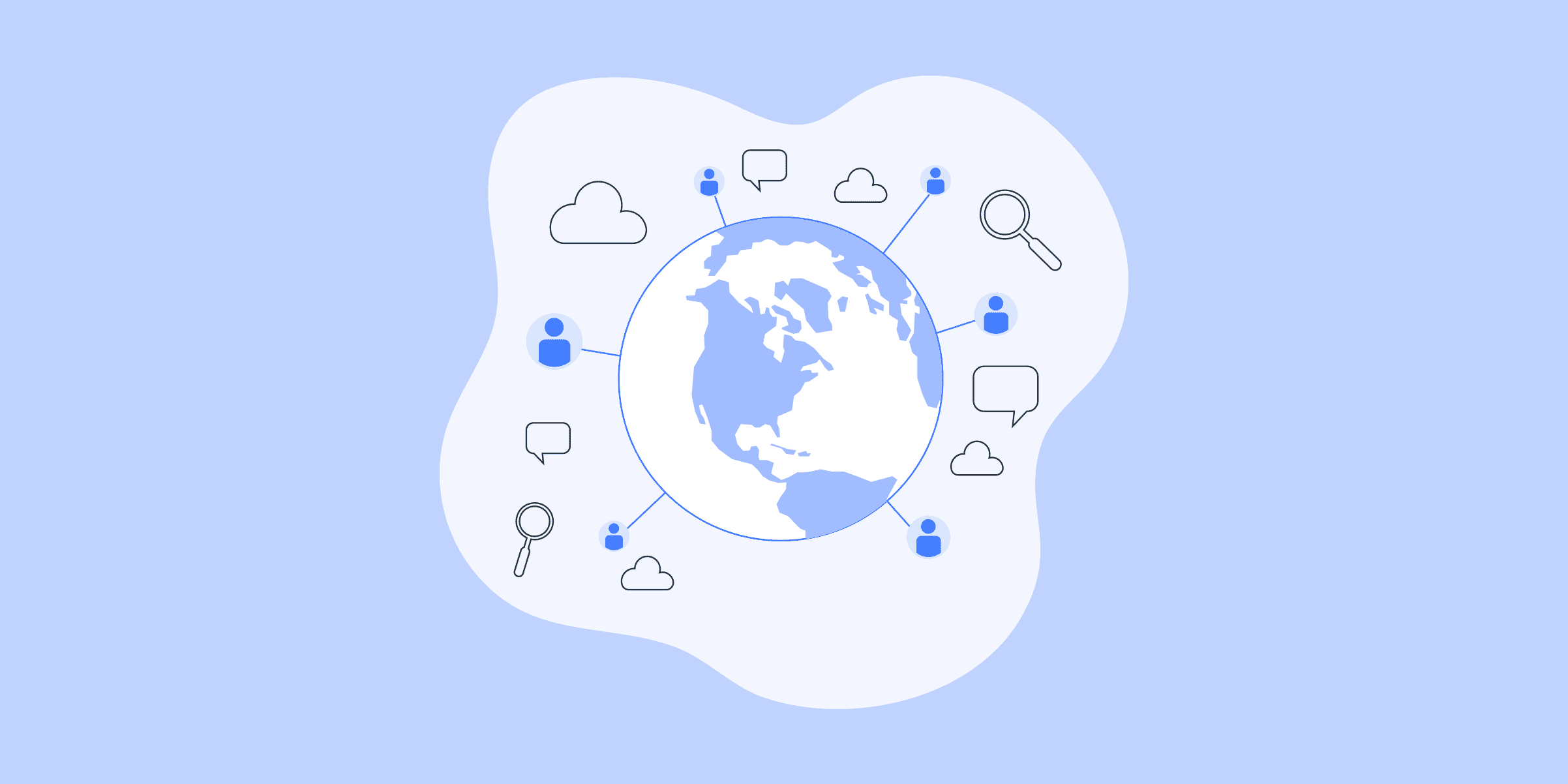
Gross Profit Margin: Formula and What It Tells You
Gross profit margin (GPM) is a key financial metric that measures a company’s profitability. It represents the percentage of net revenue made that exceeds the cost of goods sold (COGS). GPM provides valuable insights into a company’s operational efficiency and pricing strategies.
This blog post takes a closer look into the intricacies of gross profit margin, exploring its formula, calculation, and interpretation. We will also explain how to calculate gross margin, determine the percentage of gross profit in relation to revenue, and its significance for assessing a company’s financial performance. We will also analyse the significance of GPM in evaluating a company’s financial performance and discuss effective strategies to improve it. By understanding gross profit margin, businesses can make informed decisions to optimise their profitability and drive sustainable growth.
What is the Gross Profit Margin?
Understanding Gross Profit Margin: A Key Indicator of Profitability
Gross profit margin (GPM) is a key financial metric that provides insights into a company’s profitability and operational efficiency. It measures the percentage of revenue that exceeds the cost of goods sold (COGS). In simpler terms, GPM reveals how much profit a company generates for each dollar of revenue after deducting the direct costs associated with producing or purchasing its goods.
The formula for calculating GPM is straightforward:
GPM = (Revenue – COGS) / Revenue
Expressed as a percentage, the gross margin percentage offers a clear picture of a company’s ability to generate profit from its sales. A higher GPM indicates that the company is effectively managing its production costs and pricing strategies, allowing it to retain a larger portion of its revenue as profit. Conversely, a lower GPM suggests that the company may be facing challenges in controlling costs or setting competitive prices.
GPM serves as a valuable tool for businesses to assess their financial performance and make informed decisions. It enables companies to compare their profitability against industry benchmarks, identify areas for cost optimisation, and evaluate the effectiveness of their pricing strategies. By closely monitoring and analysing GPM, businesses can gain actionable insights to drive profitability and sustainable growth.
Gross Profit vs. Gross Profit Margin
Gross profit and gross profit margin are two closely related but distinct financial metrics. Gross profit represents the total amount of money a company has left after deducting the direct costs associated with producing its goods or services, while gross profit margin expresses this amount as a percentage of total revenue.
To calculate gross profit, you simply subtract the cost of goods sold (COGS) from the revenue generated by sales. COGS includes expenses such as raw materials, direct labour, and manufacturing overhead. The resulting figure is the gross profit, which represents the amount of money the company has left to cover other expenses, such as operating costs, interest payments, and taxes.
However, gross profit margin is calculated by dividing gross profit by total revenue and multiplying the result by 100. This calculation expresses the gross profit as a percentage of the total sales revenue, allowing for easy comparison between companies and industries. A higher gross profit margin indicates that the company is more efficient at managing its production costs and is able to generate more profit for each dollar of revenue. The perception of a good gross profit margin depends heavily on the industry; for example, a 40% margin might be excellent in retail but considered low in the software industry.
Understanding the difference between gross profit and gross profit margin is essential for financial analysis and decision-making. Gross profit provides a direct measure of the company’s profitability, while gross profit margin allows for benchmarking and comparison with industry peers. By monitoring and analysing these metrics, businesses can gain insights into their cost structure, pricing strategies, and overall operational efficiency, enabling them to make informed decisions to improve their financial performance.
Formula and Calculation of Gross Profit Margin
To calculate the gross profit margin, you need to first determine the gross profit and then divide it by the total revenue. The formula for gross profit margin calculation is:
Gross Profit Margin = (Gross Profit / Revenue) x 100
Here’s an example to illustrate the calculation:
Imagine a company has the following financial data for a specific period:
Revenue: $100,000
Cost of Goods Sold: $60,000
To calculate the gross profit margin from the gross profit, subtract the cost of goods sold from the revenue:
Gross Profit = Revenue – Cost of Goods Sold
Gross Profit = $100,000 – $60,000
Gross Profit = $40,000
Now, to calculate the gross profit margin, divide the gross profit by the revenue and multiply by 100:
Gross Profit Margin = (Gross Profit / Revenue) x 100
Gross Profit Margin = ($40,000 / $100,000) x 100
Gross Profit Margin = 0.4 x 100
Gross Profit Margin = 40%
In this example, the company’s gross profit margin is 40%, which means that for every dollar of revenue, the company generates $0.40 in gross profit. This indicates that the company is efficient at managing its production costs and is able to generate a significant amount of profit for each dollar of revenue.
How Gross Profit Margin Works
The gross profit margin reveals how much of each sales dollar a company retains after accounting for the direct costs of producing or delivering its products or services. A higher gross profit margin generally indicates better cost management and pricing effectiveness. Alternatively, a lower gross profit margin may signal challenges in controlling production costs or subpar pricing strategies.
To illustrate the gross margin calculation, let’s consider a hypothetical company called XYZ Corp. Suppose XYZ Corp generates $100,000 in revenue during a specific period and incurs $60,000 in direct costs associated with producing its goods. In this case, the gross profit would be $40,000 ($100,000 – $60,000).
To determine the gross profit margin, we divide the gross profit by the total revenue and multiply by 100:
Gross Profit Margin = ($40,000 / $100,000) x 100 = 40%
XYZ Corp’s gross profit margin for the period is 40%, indicating that for every dollar of revenue, it retains 40 cents after covering direct production costs.
Variations in gross profit margins across industries can be significant. Companies in industries with high production costs, such as manufacturing or construction, tend to have lower gross profit margins compared to industries with lower production costs, such as technology or software services.
Gross profit margin can also fluctuate within the same industry due to factors such as changes in commodity prices, shifts in consumer demand, or variations in production efficiency. Companies strive to maintain or improve their gross profit margin by optimising production processes, controlling costs, and implementing effective pricing strategies.
Gross Profit Margin vs. Net Profit Margin vs. Operating Profit Margin
While gross profit margin focuses solely on production costs, net income statement or profit margin takes into account all expenses incurred in the course of doing business, including operating expenses, interest, and taxes. As such, net profit margin provides a more comprehensive measure of a company’s overall profitability.
Operating profit margin, on the other hand, narrows the focus to the income generated from a company’s core operations, excluding non-operating income and expenses such as interest and taxes. It provides insights into the efficiency of a company’s core business processes and how effectively it converts revenue into operating profit.
To illustrate the differences between these three profit margin metrics, consider the following example:
– A company generates $100,000 in revenue.
– The cost of goods sold is $40,000.
– Operating expenses amount to $20,000.
– Interest expense is $5,000.
– Tax expense is $10,000.
The gross profit to gross margin ratio for this company would be:
Gross Profit Margin = ($100,000 – $40,000) / $100,000 = 0.6 or 60%
The net profit margin would be:
Net Profit Margin = ($100,000 – $40,000 – $20,000 – $5,000 – $10,000) / $100,000 = 0.25 or 25%
The operating profit margin would be:
Operating Profit Margin = ($100,000 – $40,000 – $20,000) / $100,000 = 0.4 or 40%
In this example, the gross profit margin indicates that the company retains $0.60 for every dollar of revenue after covering production costs. The net profit margin shows that for every dollar of revenue, the company has $0.25 left after accounting for all expenses, including operating expenses, interest, and taxes. Lastly, the operating profit margin reveals that the company generates $0.40 in operating profit for every dollar of revenue.
By analysing these three profit margin metrics together, businesses gain a more nuanced understanding of their financial performance and can identify areas for improvement.
Examples of Gross Profit Margin
Here are some examples of gross profit margin for different companies:
Apple: In 2021, Apple reported a gross profit margin of 37.6%. This means that for every dollar of revenue Apple generated, it kept $0.376 after accounting for the direct costs of producing its products.
Amazon: In 2021, Amazon reported a gross profit margin of 41.9%. This means that for every dollar of revenue Amazon generated, it kept $0.419 after accounting for the direct costs of fulfilling orders.
Walmart: In 2021, Walmart reported a gross profit margin of 24.9%. This means that for every dollar of revenue Walmart generated, it kept $0.249 after accounting for the direct costs of purchasing and transporting merchandise.
Nike: In 2021, Nike reported a gross profit margin of 44.5%. This means that for every dollar of revenue Nike generated, it kept $0.445 after accounting for the direct costs of manufacturing its products.
Costco: In 2021, Costco reported a gross profit margin of 14.7%. This means that for every dollar of revenue Costco generated, it kept $0.147 after accounting for the direct costs of purchasing merchandise.
These examples illustrate the wide range of gross profit margins that can exist across different industries. Companies with high gross profit margins are often able to generate more profit for each dollar of revenue they generate, which can lead to increased profitability and shareholder value.
What Does Gross Profit Margin Indicate?
Gross profit margin is an indicator of a company’s financial health and operational efficiency. It provides insights into various aspects of a business, including pricing strategy, cost management, and competitive positioning. By analysing gross profit margin, stakeholders can gain a deeper understanding of a company’s ability to generate profits and its overall financial performance.
A higher gross profit margin generally signifies that a company is effectively managing its production costs and expenses. It suggests that the company is able to generate more revenue for each dollar spent on producing or delivering its products or services. This reflects well on the company’s pricing strategy and its ability to control costs. A higher gross profit margin can also indicate that the company has a competitive advantage in terms of pricing or cost structure, allowing it to capture a larger share of the market.
A lower gross profit margin may indicate challenges in managing costs or inefficiencies in the production process. It could be a sign that the company is facing intense competition, which forces it to lower prices or increase discounts to maintain market share. Alternatively, it may suggest that the company’s cost structure is not optimised, leading to higher expenses and reduced profitability.
By monitoring and analysing gross profit margins over time, businesses can identify trends and patterns that help them make informed decisions. For instance, a declining gross profit margin may prompt a company to review its pricing strategy, cost structure, or operational processes to identify areas for improvement. On the other hand, a consistently high gross profit margin can serve as a validation of the company’s effective management practices and competitive positioning.
Gross profit margin is a valuable metric that provides insights into a company’s profitability, cost management, and competitive position. It helps stakeholders assess the financial performance of a business and identify areas for improvement. By analysing gross profit margin, companies can make informed decisions to enhance their profitability and long-term success.
Difference Between a High and Low Gross Profit Margin
This section discusses the difference between a high and low gross profit margin. A high gross profit margin indicates that a company is generating more revenue relative to its cost of goods sold, while a low gross profit margin indicates that a company is spending more on its cost of goods sold compared to its revenue. A high enough gross profit margin ratio is generally preferable as it indicates that a company is able to generate more profit from its sales.
There are several factors that can contribute to a high gross profit margin. These include:
– Efficient production processes that minimise waste and maximise output.
– Strong pricing power that allows a company to charge premium prices for its products or services.
– A focus on high-margin products or services.
– Effective cost management practices.
However, a low gross profit margin can be caused by several factors, such as:
– Inefficient production processes that result in high costs.
– Weak pricing power due to competition or other market factors.
– A focus on low-margin products or services.
– Poor cost management practices.
It is important to note that a high or low gross profit margin is not necessarily good or bad. The ideal gross profit margin for a company will vary depending on its industry, business model, and competitive landscape. By understanding the factors that affect gross profit margin, companies can make informed decisions to improve their profitability.
How Does a Company Increase Its Gross Profit Margin?
A company can increase its gross profit margin by reducing the cost of goods sold (COGS), increasing net sales by volume, improving product mix, implementing lean manufacturing techniques, and investing in marketing and promotions.
Reducing COGS involves finding ways to produce goods at a lower cost without compromising quality. This can be achieved by negotiating better deals with suppliers, optimising production processes, and eliminating waste. Increasing sales volume means selling more products or services, which can be done by expanding into new markets, offering discounts or promotions, and improving customer service.
Improving product mix refers to selling more of the products or services that have a higher gross profit margin. This can be done by discontinuing low-margin products, upselling higher-margin products, and bundling products or services together. Implementing lean manufacturing techniques involves streamlining production processes to reduce waste and improve efficiency. This can be done by using automation, reducing inventory levels, and improving quality control. Investing in marketing and promotions can help increase sales volume and improve product mix by creating awareness of the company’s products or services and generating leads.
By implementing these strategies, a company can increase its gross profit margin and improve its overall profitability.
How LIKE.TG can help your Gross Profit Margin
LIKE.TG is a powerful customer relationship management (CRM) platform that can help businesses improve their gross profit margin in a number of ways. Firstly, LIKE.TG provides real-time insights into business performance through its comprehensive reporting and analytics capabilities. This allows businesses to identify areas where they can reduce costs and improve efficiency, such as by optimising inventory levels or reducing the cost of goods sold.
Secondly, LIKE.TG can automate sales and order management processes, freeing up employees to focus on more value-added activities. As a result, this can assist businesses to reduce labour costs and improve productivity, leading to an increase in gross profit margin. Thirdly, LIKE.TG can improve customer service by providing a centralised platform for managing customer interactions. Helping businesses to resolve customer issues more quickly and efficiently, leading to increased customer satisfaction and repeat business. Finally, LIKE.TG can help businesses optimise their pricing strategies and promotions by providing insights into customer behaviour and preferences. This can help businesses set optimal prices for their products or services, and target their promotions more effectively, leading to increased sales and higher gross margins and profit margin.
LIKE.TG is a valuable tool that can help businesses improve their gross profit margin in a number of ways. By providing real-time insights into business performance, automating sales and order management processes, improving customer service, and optimising pricing strategies and promotions, LIKE.TG can help businesses reduce costs, increase sales, and ultimately improve their bottom line.
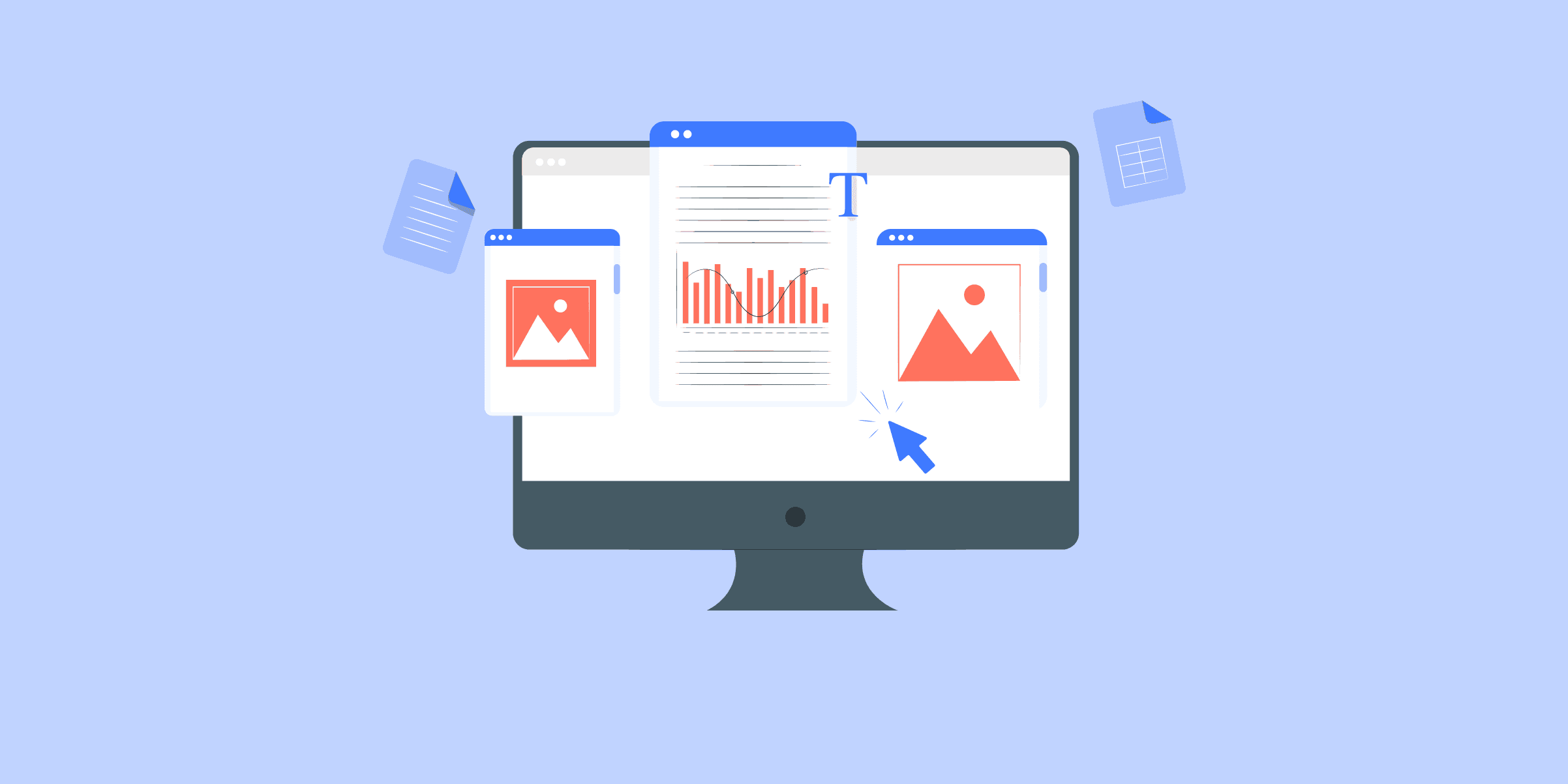
Statutory Compliance: Guide to Payroll Compliance
Within payroll management, statutory compliance is essential for responsible business practices. Adhering to federal and state employment laws is not merely a legal obligation; it’s an ethical commitment to employees and a safeguard against costly penalties. This broad guide looks into the specifics of statutory compliance in payroll, exploring its significance, various facets, and how LIKE.TG’s financial services CRM empowers businesses to streamline compliance and elevate their payroll operations.
What is statutory compliance in payroll?
In payroll management, following federal and state employment laws is very important. It’s not just a legal requirement but also a moral duty that protects employees’ rights and keeps businesses out of trouble. Statutory compliance in payroll encompasses a diverse spectrum of responsibilities, including the provision of fair compensation, adherence to minimum wage standards, and the meticulous calculation and remittance of taxes, social security contributions, and other deductions. Each of these elements plays a pivotal role in ensuring that employees receive the remuneration and benefits they rightfully deserve, as stipulated by law.
The significance of statutory compliance in payroll cannot be overstated. It’s an imperative element of ethical business practices, fostering a harmonious work environment where employees feel valued and respected. Compliance safeguards businesses from the potential repercussions of non-compliance, such as hefty fines, legal penalties, and the erosion of their hard-earned reputation.
The legal environment is notoriously ever-changing, so keeping up with the latest employment laws is essential to ensure payroll follows the rules. Businesses must demonstrate unwavering commitment to continuous learning and adaptation, diligently monitoring regulatory changes and incorporating them into their payroll practices. Only through such vigilance can they navigate the complexities of payroll compliance and fulfil their obligations to their employees, the government, and society at large.
Importance of statutory compliance
Statutory compliance in payroll is of paramount importance as it ensures that businesses adhere to the legal obligations set by government regulations and labour laws. This adherence is crucial for several reasons. Firstly, it ensures that employees are treated fairly and in accordance with the law. By complying with statutory regulations, businesses safeguard the rights and well-being of their employees, fostering a positive work environment. Secondly, statutory compliance helps businesses avoid legal penalties and fines that may arise from non-compliance.
These penalties can be substantial and can have a significant financial impact on a business. Thirdly, compliance contributes to building trust and credibility with employees, customers, and stakeholders. When a business demonstrates its commitment to statutory compliance, it establishes itself as a responsible and ethical organisation, enhancing its reputation and fostering trust among its stakeholders. Overall, statutory compliance is not just a legal requirement but also a fundamental aspect of responsible business practices that promotes fairness, protects employees’ rights, and contributes to the success and sustainability of a business.
Types of statutory compliance in payroll
Minimum wage complianceThe federal minimum wage is $7.25 per hour. However, many states and cities have their own minimum wage laws that are higher than the federal minimum. Businesses must comply with the highest minimum wage law that applies to their employees.
Overtime pay compliance
Employees who work more than 40 hours a week are entitled to overtime pay at a rate of 1.5 times their regular pay rate. There are some exceptions to this rule, such as for employees who are exempt from overtime pay.
Child labour laws compliance
Child labour laws restrict the types of jobs that children can do and the hours that they can work. Businesses must comply with all applicable child labour laws.
Equal pay laws compliance
Equal pay laws prohibit employers from paying women less than men for equal work. Businesses must conduct regular pay audits to ensure that they are not violating equal pay laws.
Family and medical leave laws compliance
Family and medical leave laws allow employees to take unpaid leave from work for certain family and medical reasons. Businesses must comply with all applicable family and medical leave laws.
These are just a few of the many types of statutory compliance that businesses must adhere to in order to stay compliant with federal and state laws. Businesses that fail to comply with statutory compliance requirements can face serious consequences, including fines, penalties, and legal action.
Statutes on employee salaries and benefits
Several federal and state statutes govern employee salaries and benefits. These laws set forth requirements for minimum wage, overtime pay, equal pay, family and medical leave, and other benefits. Employers must comply with these laws to ensure fair treatment of their employees and avoid legal penalties.
One of the most important federal laws governing employee salaries and benefits is the Fair Labour Standards Act (FLSA). The FLSA sets the minimum wage for non-exempt employees and requires employers to pay overtime pay to employees who work more than 40 hours per week. The FLSA also includes provisions for child labour laws and equal pay laws.
The Equal Pay Act (EPA) is another important federal law that prohibits employers from discriminating against employees based on gender in terms of compensation. The EPA requires employers to pay men and women equally for equal work.
The Family and Medical Leave Act (FMLA) provides employees with the right to take unpaid, job-protected leave for certain family and medical reasons. The FMLA covers employees who have worked for their employer for at least 1,250 hours in the 12 months preceding the leave.
The Worker Adjustment and Retraining Notification Act (WARN) requires employers to provide advance notice to employees of plant closings or mass layoffs. The WARN Act covers employers with 100 or more employees.
The Consolidated Omnibus Budget Reconciliation Act (COBRA) allows employees who lose their health insurance coverage due to job loss or other qualifying events to continue their coverage for a limited period. COBRA covers employees who have worked for their employer for at least 20 hours per week for the 12 months preceding the loss of coverage.
These are just a few of the many federal and state statutes that govern employee salaries and benefits. Employers must comply with all applicable laws to ensure fair treatment of their employees and avoid legal penalties.
Statutes on social security
Must be written for each region
Statutes on tax liabilities
Must be written for each region
LIKE.TG’s financial services CRM
LIKE.TG’s financial services CRM offers a comprehensive suite of tools and features designed specifically to help businesses in the financial services industry streamline compliance, simplify reporting, automate calculations, enhance security, and improve customer service.
Streamlined Compliance
With LIKE.TG’s financial services CRM, businesses can centralise and manage all compliance-related data in a single platform. This enables easy access to relevant information, facilitates efficient reporting, and ensures compliance with industry regulations. The CRM’s robust compliance management capabilities help businesses stay up-to-date with changing regulations, automate compliance tasks, and mitigate risks associated with non-compliance.
Simplified Reporting
LIKE.TG’s financial services CRM simplifies the complex task of generating reports. The platform’s pre-built templates and customisable reporting tools enable businesses to quickly and easily create reports that meet their specific needs. With real-time data and comprehensive analytics, businesses can gain valuable insights into their operations, identify trends, and make informed decisions. The CRM’s advanced reporting capabilities empower businesses to demonstrate compliance, optimise processes, and enhance overall performance.
Automated Calculations
LIKE.TG’s financial services CRM automates various calculations related to payroll, commissions, bonuses, and other financial transactions. This eliminates manual calculations and reduces the risk of errors. The CRM’s powerful automation engine ensures accurate and consistent calculations, saving businesses time and resources while enhancing overall efficiency. By automating calculations, businesses can streamline their operations, improve accuracy, and focus on delivering exceptional customer service.
Enhanced Security
LIKE.TG’s financial services CRM provides robust security measures to protect sensitive financial data. The platform employs industry-standard encryption protocols, access controls, and authentication mechanisms to safeguard data from unauthorised access. LIKE.TG’s commitment to security ensures that businesses can confidently manage and store financial information, reducing the risk of data breaches and maintaining customer trust.
Improved Customer Service
LIKE.TG’s financial services CRM empowers businesses to deliver exceptional customer service. The platform’s customer relationship management (CRM) capabilities enable businesses to centralise customer interactions, track customer history, and provide personalised experiences. With LIKE.TG’s CRM, businesses can streamline customer communications, resolve inquiries efficiently, and build strong customer relationships. By leveraging LIKE.TG’s financial services CRM, businesses can enhance customer satisfaction, increase loyalty, and drive business growth.
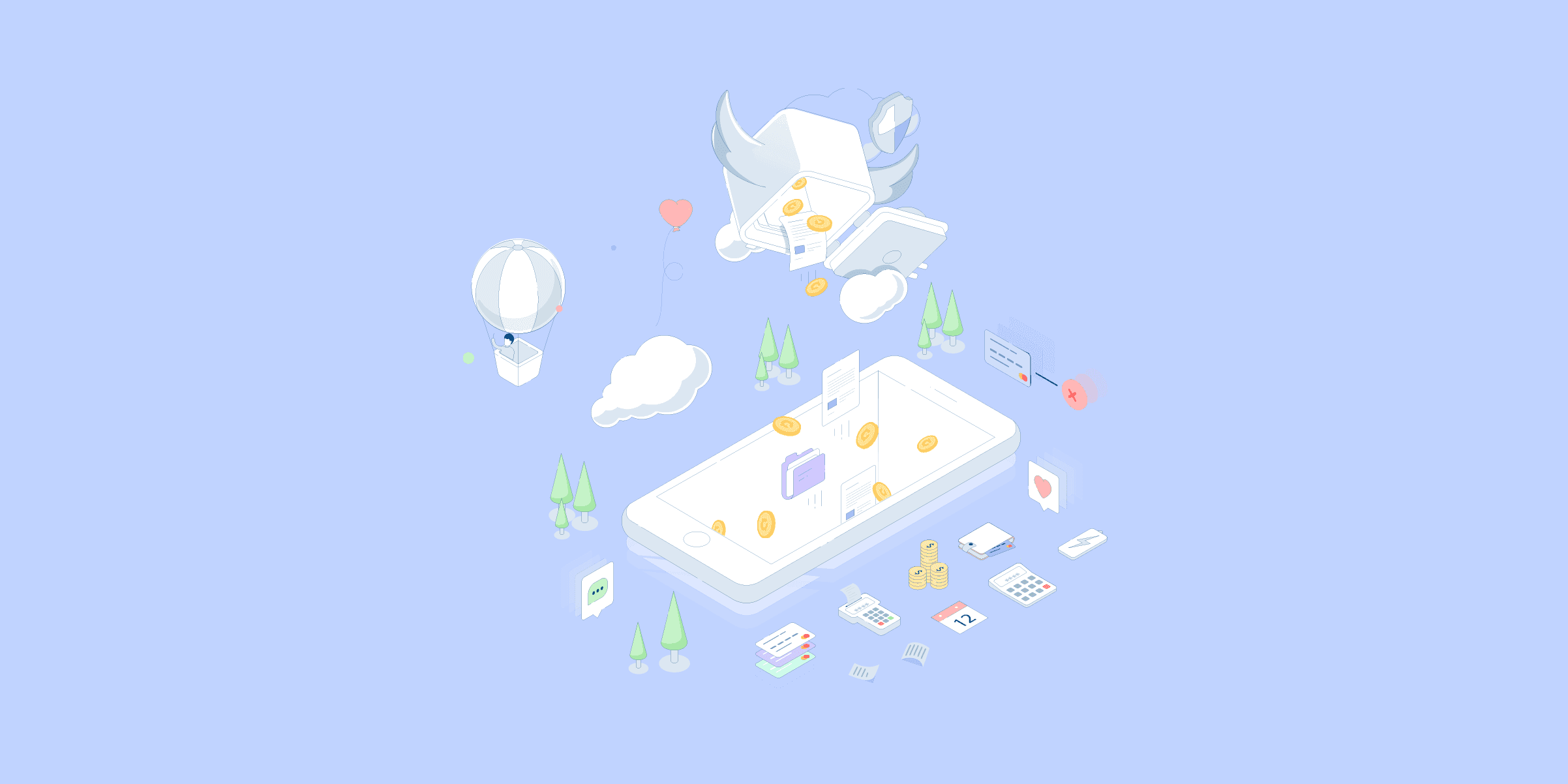
CRM Finance: Choose the Best CRM for Financial Services
The financial services industry is constantly evolving, and businesses need to keep up with the latest technology to stay competitive. One important tool that can help financial services businesses succeed is a customer relationship management (CRM) system. Throughout this blog, we will explore the importance of CRM for financial services businesses, and how a CRM can help improve customer service, streamline sales, and enhance collaboration.
What Is a CRM for Financial Services?
The financial services and insurance industry is one that constantly changes, so having the right tools to manage customer relationships and interactions is paramount to success. This is where CRM for financial services comes into play. CRM stands for Customer Relationship Management and refers to the technology and strategies employed by businesses to manage and analyse customer interactions and data throughout the customer lifecycle.
Specifically designed for financial institutions, a CRM system for financial services is a software platform that centralises customer data, sales, marketing, customer service, and support activities. This centralised platform allows financial institutions to gain a holistic understanding of their customers and deliver exceptional client service. By leveraging the capabilities of a CRM system, financial institutions can streamline their operations, enhance collaboration among teams, and ultimately, drive business growth.
Integrating CRM with other tools like Outlook and LIKE.TG can save time and reduce human error, ensuring that data is accurately copied and managed across platforms.
CRM systems for financial services address the unique needs of the industry, ensuring compliance with regulatory requirements, data security, and customer privacy. With a CRM system in place, financial institutions can efficiently manage customer information, track interactions, and make informed decisions based on data-driven insights. This leads to improved customer experiences, increased operational efficiency, and a competitive edge in the market.
How Can a Finance CRM Help Your Business?
A CRM system can help financial services businesses improve customer service by providing a centralised platform for managing all customer interactions. This allows businesses to track customer history, preferences, and interactions, and to provide personalised and efficient service. This reduces customer frustration when contacting their service provider. For instance, a financial advisor can use a CRM to quickly access a client’s account information, view their past transactions, and make notes about their financial goals. This enables the advisor to provide tailored advice and recommendations that meet the client’s specific needs. Additionally, a CRM can help automate tasks such as sending appointment reminders and following up on leads, freeing up advisors to focus on providing excellent customer service.
A CRM can also help financial services businesses streamline sales by providing tools for managing leads, tracking opportunities, and forecasting revenue. This can help businesses identify and prioritise high-value leads, allocate resources effectively, and close deals faster. For example, a CRM can automatically score leads based on their likelihood to convert, allowing sales teams to focus their efforts on the most promising opportunities. Additionally, a CRM can provide insights into the sales process, such as which marketing channels are generating the most leads or which sales tactics are most effective. This information can help businesses optimise their sales strategies and improve their bottom line.
Finally, a CRM can help financial services businesses enhance collaboration by providing a central platform for sharing information and communicating with colleagues. This can help break down silos between departments and improve teamwork. For instance, a customer service representative can use a CRM to quickly get in touch with a subject matter expert to resolve a customer issue. Additionally, a CRM can be used to track and manage projects, ensuring that everyone is on the same page and that projects are completed on time and within budget.
In conclusion, a CRM system can provide numerous benefits for financial services businesses, including improved customer service, streamlined sales, and enhanced collaboration. By leveraging the capabilities of a CRM, financial institutions and clients can gain a competitive advantage and achieve long-term success.
How Can a Finance CRM Help Your Customers?
A finance CRM can provide customers with a secure and convenient way to manage their finances. It can help them track their spending, set financial goals, make informed financial decisions, and stay up-to-date on their financial accounts and transactions. By providing customers with a centralised platform for managing their finances, a CRM can help them improve their financial literacy and make better financial decisions.
For example, a finance CRM can help customers track their income and expenses, create budgets, and set savings goals. It can also provide customers with insights into their spending habits and help them identify areas where they can cut back. Additionally, a finance CRM can help customers stay up-to-date on their financial accounts and transactions by providing them with real-time notifications and alerts.
Overall, a finance CRM can be a valuable tool for customers looking to manage their finances more effectively. It can provide them with the information and tools they need to make informed financial decisions and improve their financial literacy.
The Features You Need From a CRM for Client Management in Financial Services
A CRM for financial services should have robust contact management capabilities. This includes the ability to store and manage customer contact information, such as name, address, phone number, and email address. It should also allow users to create and manage contact groups, such as customers, prospects, and vendors.
Lead management is another important feature for a CRM for financial services. This includes the ability to track leads from the initial point of contact through the sales process. It should also allow users to qualify leads, assign them to sales representatives, and track their progress.
Opportunity management is also essential for a CRM for financial services. This includes the ability to track sales opportunities from the initial contact through the close of the sale. It should also allow users to create and manage sales forecasts, see interest rates, track win rates, and identify trends.
Integration with other business systems is also important for a CRM for financial services. This includes the ability to integrate with accounting systems, ERP systems, and other business applications. This allows users to access all of their customer data in one place, which can help companies improve efficiency and productivity.
Reporting and analytics are also important features for a CRM for financial services. This includes the ability to generate reports on customer activity, sales performance, and other key metrics. It should also allow users to create custom reports and dashboards, so they can track the metrics that are most important to them.
How to choose the best financial CRM for your business?
When selecting the most suitable CRM for your financial services business, there are several factors to consider. First, it is essential to identify your specific CRM requirements. Determine the key features and functionalities that are essential for your business, such as customer data management, sales tracking, and reporting capabilities. This will help narrow down your options and select a CRM that aligns with your specific needs.
Next, it is important to set a budget for your CRM investment. CRM systems can vary significantly in cost, so it is crucial to determine how much you are willing to spend before beginning your search. Consider the long-term return on investment (ROI) when making your decision, as a well-chosen CRM can lead your company to increased efficiency, improved customer service, and ultimately, increased revenue.
The size of your business is also an important factor to consider when choosing a CRM. Smaller businesses may have different needs and requirements compared to larger enterprises. Look for a CRM that is scalable and can grow with your business as it expands. Consider the number of users who will need access to the CRM’s ability, and ensure that the system can accommodate your current and future needs.
The industry in which you operate can also influence your CRM selection. Different industries have specific requirements and regulations, so it is important to choose a CRM that is tailored to your industry’s needs. For example, a banking or financial services CRM should have features that support compliance with industry-specific regulations and provide the necessary security measures to protect sensitive customer data.
Finally, it is advisable to read reviews of different CRM systems before making a decision. Customer reviews and feedback can provide valuable insights into customer satisfaction and the pros and cons of various CRM solutions. Look for reviews that highlight the strengths and weaknesses of each CRM and consider how they align with your business requirements. By carefully evaluating these factors and conducting thorough research, you can select the best financial CRM for your business and reap the benefits of improved customer service, streamlined sales, and enhanced collaboration.
Key features of CRM for financial services
Contact management is a key feature of CRM for financial services. It enables financial institutions to centralise and manage customer information, including personal details, contact information, and financial data, in a single, easily accessible platform. This allows financial advisors and customer service representatives to quickly access customer information, understand their financial needs, and provide personalised advice and services.
Lead management is another important feature of CRM for financial services. It helps financial institutions capture, qualify, and track leads, and manage the sales pipeline. With a CRM system, financial advisors can easily capture lead information, such as contact details, interests, and financial goals, and track their progress through the sales funnel. This enables them to prioritise high-quality leads, allocate resources effectively, and optimise their sales efforts.
Task management is also a valuable feature of CRM for financial services. It allows financial institutions to assign, track, and manage tasks and activities related to client management, customer interactions, sales, and service. Financial advisors can use the task management feature to create to-do lists, set priorities, and track their progress in completing tasks. This helps them stay organised, manage their time effectively, and ensure that no important tasks fall through the cracks.
Reporting and analytics is another essential feature of CRM for financial services. It enables financial institutions to generate reports and analyse data related to customer interactions, sales, and service performance. Financial advisors can use these reports to gain insights into customer behaviour, identify trends, and measure the effectiveness of their sales and service strategies. This information can help them make data-driven decisions, improve their performance, and achieve better business outcomes.
Finally, integration with other financial software is a key feature of CRM for financial services. It allows CRM systems to connect and exchange data with other financial software applications, such as accounting systems, portfolio management systems, and trading platforms. This integration enables financial institutions and organisations to streamline their operations, reduce manual data entry, and improve the accuracy and efficiency of their financial processes.
Best financial CRM software
When it comes to choosing the best financial CRM software for your business, there are many factors to consider. Some of the top financial CRM software solutions on the market for Financial Services. Each of these solutions offers a unique set of features and benefits, so it’s important to evaluate your specific needs and requirements before making a decision on one platform.
LIKE.TG Financial Services Cloud is a comprehensive CRM solution that offers a wide range of features specifically designed for the financial services industry. These features include contact management, lead management, opportunity management, forecasting, and reporting. LIKE.TG Financial Services Cloud also integrates with other LIKE.TG products, such as Sales Cloud and Service Cloud, to provide a complete customer experience management solution.
Oracle Financial Services Cloud is another CRM solution for the financial services industry. Oracle Financial Services Cloud offers a comprehensive set of features, including contact management, lead management, opportunity management, forecasting, and reporting. Oracle Financial Services Cloud also integrates with other Oracle products, such as Oracle E-Business Suite and Oracle Siebel CRM, to provide a complete customer experience management solution.
Microsoft Dynamics 365 for Financial Services is a cloud-based CRM solution that offers a range of features designed for the financial services industry. These features include contact management, lead management, opportunity management, forecasting, and reporting. Microsoft Dynamics 365 for Financial Services also integrates with other Microsoft products, such as Office 365 and Dynamics 365 Sales, to provide a complete customer experience management solution.
SAP Hybris Cloud for Financial Services is a cloud-based CRM solution that offers a comprehensive set of features specifically designed for the financial services industry. These features include contact management, lead management, opportunity management, forecasting, and reporting.
Pegasystems CRM for Financial Services is a cloud-based CRM solution that offers a range of features specifically designed for the financial services industry. These features include contact management, lead management, opportunity management, forecasting, and reporting. Pegasystems CRM for Financial Services also integrates with other Pegasystems products, such as Pegasystems BPM and Pegasystems Customer Service, to provide a complete customer experience management solution.

3 Easy Steps to Pick the Best AI Chatbot for Your Business
Choosing the best AI chatbot for your business is an important part of getting the most out of artificial intelligence (AI). There are many chatbots on the market, and each is trained to excel at different things — Siri as your hand-held personal assistant, Einstein Copilot as your do-it-all digital employee, or ChatGPT as your human-like conversational partner.
An AI chatbot is a computer program designed to simulate human-like conversation through text or voice. In contrast to older rules-based chatbots, which have been around for decades, AI chatbots are created by training large language models (LLMs) on vast datasets of human conversations and knowledge, which helps the chatbot to understand natural language and generate relevant, contextual responses.
Here, you’ll discover how to clarify your needs, cut through the chatbot clutter, and find the best AI chatbot for the job.
What you’ll learn:
The benefits of using AI chatbots
How to pick the best chatbot
The AI chatbot revolution
The benefits of using AI chatbots
Thanks to AI chatbots, gone are the days of canned robotic responses, endless hold times, or making customers wait days for an email reply. Having always-on digital assistants available to deliver fast and personalised service is now the norm.
But chatbots don’t just benefit customers — they’re transformative for businesses, too. Every customer interaction is an opportunity for bots to uncover insights quickly and systematically, and potentially pinpoint ones you may have missed otherwise. In an age where data is king, chatbots can collect and analyse conversational data from direct interactions with your customers. What are their biggest pain points? What questions keep coming up? With that knowledge, you can fine-tune your products and deliver even better service and support for your customers. (Back to top)
How to pick the best AI chatbot
With new chatbot options constantly hitting the market and offering varying specialties, it’s important to sort through the clutter and determine what is the best AI chatbot for your business. Understanding your specific needs, comparing costs, exploring customisation options, utilising your company’s existing data, and weighing functionality are all important factors to consider when deciding which AI chatbot to use.
To get started, consider these questions to pick the best AI chatbot for your business:
1. What are your goals?
There are specialised chatbots for everything from customer service to sales to marketing. So, the first step is to identify how a chatbot will help you accomplish your business goals. “What is the business outcome you want? For example, reduce cost, increase sales, or minimise customer service volume?” said Aron Kale, director of product management on the LIKE.TG AI team. “Getting really clear on what you want out of your chatbot is an important first step.”
For example, if providing better, more efficient customer service is your goal, prioritise chatbots that are adept at resolving common customer queries. Or, if automating repetitive internal tasks is the priority, look for chatbots that will integrate with existing systems and can handle multi-step workflows. If you’re focused on finding a solution to help increase sales and drive more conversions, find an AI assistant that can do things like make personalised customer recommendations and manage transactions.
A broader view is also beneficial to consider the ways an AI chatbot could make a bigger long-term impact. Are there downstream key performance indicators that your AI chatbot could affect?
For example, an HR chatbot could improve employee productivity and engagement by providing around-the-clock employee support, answering questions about company benefits, or sending automated reminders for project deadlines. A sales chatbot might boost conversion rates and lower customer acquisition costs by helping sales teams zero in on the highest-quality prospects. Or a customer service chatbot can improve satisfaction, loyalty, and retention metrics by providing personalised customer assistance.
Whatever your goals are, it’s important to understand the varied abilities of the different AI chatbot solutions on the market. Chatbots run the gamut, from those that are purely question-and-answer solutions to tools that can resolve issues independently from start to finish. Map out the ideal level of conversational complexity, task automation, language support, and human-AI interaction for your business.
2. Where does your data live?
One of the most important factors to consider when picking the best AI chatbot for your business is access to your data. How easily the tool can tap into your company’s own data can mean the difference between a richly personalised output and one that’s generic and unhelpful.
“Think about it in terms of what your human workers need in order to do their jobs well. Those are the same things your chatbot needs,” Kale explained. “So if you want your chatbot to focus on customer service issues, your chatbot needs to know your policies and look up an order status. Which you can pull in through tools like Data Cloud and MuleSoft.”
Think about the wealth of information contained in your company’s customer records, product information, service histories, internal policies, prospective customer data, and more. This data is a goldmine of insights, but it’s often spread across siloed databases, CRM software, conversation histories, and document repositories. Instead of giving generic responses, an AI chatbot that can access, understand, and seamlessly use this data can provide actionable answers that are specific to your business.
Let’s say that you run an online sports apparel store and a new customer asks the chatbot, “When will my order arrive?” A basic chatbot might reply with a standard line like “Orders typically ship in 3-5 business days.” In contrast, a chatbot with access to your order management system would be able to respond in a much more personalised way: “Your order #913 for a Sacramento Kings jersey shipped yesterday via UPS. It’s expected to arrive this Thursday.” This kind of interaction goes a long way toward building brand loyalty, and the customer data needed to enable it likely already resides within your existing systems.
A data-connected chatbot can also work wonders for your HR team. A chatbot that can tell employees exactly how many vacation days they have left or explain retirement plans based on their personal file makes for a great employee experience. And in sales, it can recommend products that complement a customer’s past purchases. In short, an AI chatbot that connects seamlessly to your company data is a true expert in your business, not just a generic assistant.
When comparing chatbots, understand how different solutions connect to various data sources. Can it integrate with common systems like LIKE.TG, SAP, or Microsoft Dynamics? Does it support APIs to link to custom databases? How does it handle data security? The best chatbot for your business is the one that not only understands natural language, but also transforms your data into an important asset that can build lasting brand loyalty.
3. What resources do you need to get going?
As you evaluate different chatbot solutions, it’s important to have realistic expectations around technical expertise, budget, time to invest in learning, and what the AI can and can’t do right out of the box. How easy it is to integrate into your existing system should be a consideration.
For example, is it a low- or no-code option that needs minimal training to get up and running? What level of technical skill is required for customisations or updates? Are there additional costs for scaling or advanced functionality down the road? Getting a strong handle on the real human effort and total cost of ownership needed to get the most out of your chatbot is key.
Finally, consider what internal data your chatbot needs to be trained on to be effective across your marketing, service, sales, and IT teams. Uniting all your structured and unstructured data under one roof is the best way to get the most out of it, and making that data available through a conversational AI like Einstein Copilot can be a game changer for every facet of your business.
And if this big, exciting new world of AI is all a bit overwhelming, it’s important to know that you can start small and build up. “It’s really possible to start small and really simple, with things like QA and knowledge articles,” Kale said. “It doesn’t have to integrate to 50 things right away — you can add use cases and expand it to meet different use cases over time.” (Back to top)
The AI chatbot revolution
As natural language processing capabilities continue to advance, we can expect these virtual assistants to become even more humanlike and intuitive. The pace of AI innovation will continue to pick up speed, making now the best time to learn these tools, Kale said.
“The space is rapidly evolving. We’re seeing so much innovation, and businesses are seeing real value like never before,” he said. “It’s going to keep getting faster and faster, better and better. Start learning and getting hands-on now so that you’re well prepared as the technology evolves.”(Back to top)
Cassidy Myers-Sims contributed to this blog article.
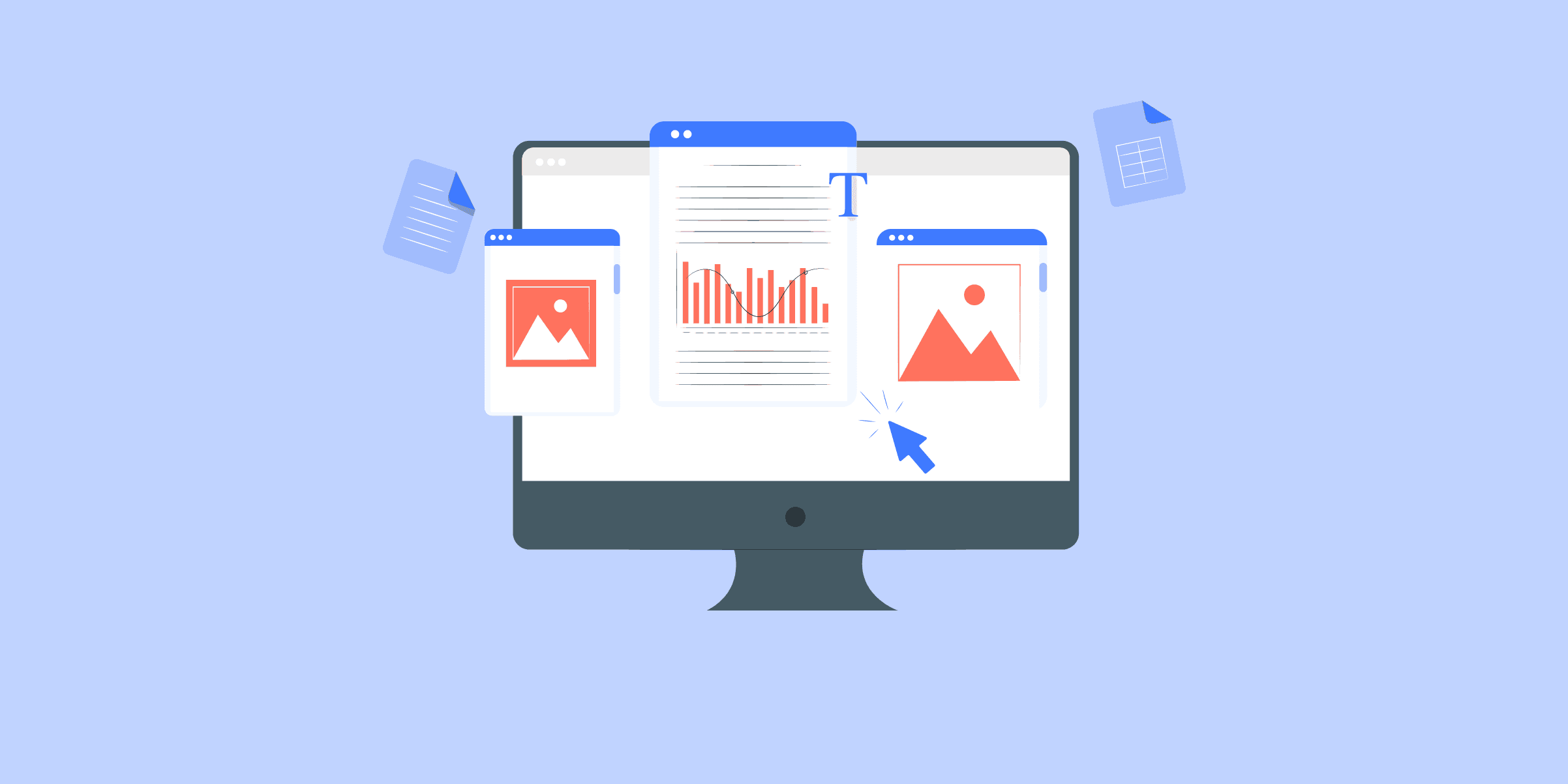
Retail Supply Chain Management Guide: The Importance & Strategies
Retail is an industry that is constantly changing and growing, so managing the supply chain effectively is essential to its success. Improving collaboration with suppliers and utilising technology to streamline all aspects of the supply chain can significantly enhance overall supply chain performance. Join us as we take a closer look at the complexities of retail supply chain management, exploring the interplay of processes, people, and technology that bring products from suppliers to customers. Along the way, we’ll uncover challenges that retailers face, including demand uncertainty, supply chain disruptions, and inventory management. We’ll also examine the practical strategies to overcome these hurdles and discover the best practices and emerging trends that are reshaping the future of retail supply chain management. From the transformative power of AI and machine learning to the growing emphasis on sustainability, this guide will equip you with the knowledge and insights needed to excel within the retail industry.
What Is Retail Supply Chain Management?
Within retail, the supply chain encompasses a vast network that orchestrates the movement of products and services from the hands of suppliers to the doorsteps of customers. This intricate system involves a multitude of players, from raw material providers and manufacturers to distributors, retailers, and end consumers. Retail Supply Chain Management (RSCM) emerges as the art of meticulously planning, executing, controlling, and monitoring all activities within this network. Its primary objective? To generate net value, establish a competitive infrastructure, and seamlessly align the supply chain strategy with ever-changing demand.
RSCM encompasses every step of the product journey, from the initial sourcing of raw materials to the final delivery to the customer. It involves the coordination of various processes, including manufacturing, warehousing, transportation, and retailing. Each step demands careful management to ensure the smooth flow of goods, minimise costs, and maintain optimal inventory levels.
Effective RSCM is not simply a logistical endeavour; it’s a strategic imperative that can significantly impact a retailer’s success. By optimising the supply chain, retailers can enhance customer satisfaction, reduce operational costs, and gain a competitive edge in the dynamic retail business.
Retail Supply Chain Management Explained
Retail Supply Chain Management (Retail Supply Chain Management, or RSCM for short) is a critical aspect of the retail industry, encompassing all the processes involved in bringing products from suppliers to end customers. It’s a complex network that requires careful planning, coordination, and execution to ensure efficient and cost-effective operations.
The key components of RSCM include:
Sourcing and procurement: This involves identifying and selecting suppliers, negotiating contracts, and managing the flow of raw materials and components into the production process.
Manufacturing: This stage involves converting raw materials into finished products. Retailers may own and operate their manufacturing facilities or outsource production to third-party manufacturers.
Warehousing and distribution: This involves storing finished products in warehouses and managing their distribution to retail stores or directly to customers.
Retailing: This is the final stage of the supply chain, where products are sold to end consumers through various channels, including physical stores, online platforms, and mobile apps.
Throughout the supply chain, retailers face numerous challenges, including demand uncertainty, supply chain disruptions, inventory management, and the need to maintain high levels of customer service. Effective RSCM involves developing strategies to mitigate these challenges and ensure that products reach customers in a timely, cost-effective, and satisfactory manner.
Challenges in retail supply chain management
Retailers face a myriad of challenges in managing their supply chains. One of the most daunting is the inherent uncertainty of demand. Consumer preferences can be highly volatile, influenced by factors such as fashion trends, economic conditions, and unforeseen events. This makes it challenging for retailers to accurately forecast demand and align their supply chains accordingly. Overestimating demand can lead to excess inventory and associated costs while underestimating demand can result in stockouts and lost sales.
Another significant challenge in retail supply chain management is the vulnerability to disruptions. The COVID-19 pandemic has underscored the fragility of global supply chains, exposing retailers to risks such as factory closures, port congestion, and transportation delays. Geopolitical tensions, natural disasters, and trade disputes can further exacerbate these disruptions. Retailers must develop contingency plans and build resilience into their supply chains to mitigate the impact of such disruptions and ensure business continuity.
Inventory management is another challenge in retail supply chain management. Retailers and supplier management must strike a delicate balance between maintaining adequate stock levels to meet customer demand and avoiding excessive inventory that ties up capital and incurs storage costs. Factors such as product seasonality, lead times, and inventory turnover rates need to be carefully considered to optimise inventory levels and minimise the risk of stockouts or overstocking.
Building and maintaining strong relationships with suppliers is essential for effective retail supply chain management. Retailers rely on suppliers for a steady flow of quality products at competitive prices. However, for many retail businesses, managing supplier relationships can be complex, involving negotiations, contract management, and ensuring compliance with ethical and sustainability standards. Retailers must foster collaborative partnerships with suppliers to ensure mutual benefit and supply chain efficiency.
In addition to these challenges, retailers must also contend with the rapid pace of technological advancements. The rise of e-commerce, the proliferation of mobile devices, and the increasing adoption of automation and artificial intelligence are transforming the retail industry. Retailers must embrace these technologies and adapt their supply chains accordingly to remain competitive and meet the evolving needs of their customers.
Strategies for effective retail supply-chain management
Effective Retail Supply Chain Management (RSCM) is necessary within retail. To achieve supply chain excellence, retailers must adopt innovative strategies that optimise inventory management, leverage technology, foster supplier collaboration, and ensure continuous monitoring and improvement.
One key strategy for managing inventory well is the utilisation of data analytics to optimise inventory levels. By leveraging historical sales data, demand forecasting, and real-time inventory tracking, retailers can strike a balance between minimising stockouts and avoiding excess inventory. This data-driven approach reduces carrying costs, improves cash flow, and enhances customer satisfaction by ensuring product availability.
Embracing automation and technology is also essential for streamlining supply chain processes. Implementing automated systems for tasks like order fulfilment, warehouse management, and transportation scheduling can significantly enhance efficiency and accuracy. Technologies such as radio frequency identification (RFID) and blockchain can provide real-time visibility and traceability throughout the supply chain, facilitating better coordination and reducing the risk of errors.
Collaboration with suppliers is another basis for effective RSCM. Establishing strong relationships built on trust and transparency fosters open communication, enabling retailers to gain insights into suppliers’ production schedules, inventory levels, and potential disruptions. This collaboration facilitates proactive planning, reduces lead times through inventory optimisation, and minimises the impact of unforeseen challenges.
Developing robust contingency plans is paramount in mitigating risks and disruptions. Given the changing nature of the retail industry, retailers must be prepared to handle disruptions such as natural disasters, supplier bankruptcies, or market fluctuations. Creating alternative sourcing strategies, maintaining safe stock levels, and diversifying transportation routes can help retailers navigate disruptions effectively and minimise their impact on customer service.
Continuous monitoring and evaluation of supply chain performance are vital for identifying areas of improvement. Establishing key performance indicators (KPIs) and regularly analysing data related to inventory turnover, order fulfilment rates, and transportation costs enables retailers to pinpoint inefficiencies and make data-driven decisions for ongoing optimisation.
By implementing these strategies, retailers can achieve a resilient, efficient, and agile supply chain that supports their business objectives and delivers exceptional customer experiences.
Best practices for successful retail supply-chain management
Retailers can achieve significant improvements in their supply chain management practices by adopting a range of proven strategies. One crucial element is implementing real-time inventory tracking systems. These systems provide accurate and up-to-date information on inventory levels, enabling retailers to make informed decisions about replenishment, production, and distribution. This reduces the risk of stockouts, optimises inventory levels, and minimises the need for costly expedited shipping.
Another best practice involves optimising warehouse operations. Retailers should strive to streamline warehouse processes, such as receiving, put-away, picking, and packing. This can be achieved through efficient warehouse layout design, the use of automation and material handling equipment, and the implementation of inventory management systems. By optimising warehouse operations, retailers can reduce labour costs, improve order fulfilment accuracy, reduce shipping costs and enhance overall supply chain efficiency.
Building strong relationships with suppliers is also essential for successful retail supply chain management. Retailers should work closely with their suppliers to establish clear communication channels, agree on performance expectations, and collaborate on inventory planning and forecasting. This fosters trust and cooperation, leading to improved product quality, reliable supply chain partners, and reduced lead times. Additionally, retailers can benefit from supplier expertise and gain access to innovative products and technologies.
Leveraging technology to automate tasks is another key best practice. Technology solutions, such as inventory management software, transportation management systems, and warehouse management systems, can automate repetitive and time-consuming tasks, freeing up resources to focus on strategic initiatives. Automation also enhances accuracy, reduces errors, and improves overall supply chain visibility.
Finally, retailers should continuously monitor and analyse their supply chain performance. This involves collecting and analysing data on key performance indicators (KPIs), such as inventory turnover, order fulfilment rates, and transportation costs. By regularly reviewing this data, retailers can identify areas for improvement, make data-driven decisions, and proactively address potential issues. This ongoing monitoring and analysis enable retailers to maintain a competitive edge and adapt to changing market dynamics.
By implementing these best practices, retailers can achieve successful Retail Supply Chain Management, resulting in improved operational efficiency, reduced costs, enhanced customer satisfaction, and increased profitability for retail businesses.
The Future of the Retail Supply Chain
The retail supply chain is undergoing a period of rapid transformation driven by technological advancements and changing consumer behaviours. Retail Supply Chain Management is embracing innovative technologies such as artificial intelligence (AI) and machine learning (ML) to optimise various aspects of the supply chain. AI and ML algorithms are being used for accurate demand forecasting, enabling retailers to maintain optimal inventory levels and reduce the risk of stockouts. These technologies also enhance logistics planning by suggesting efficient routes and modes of transportation, resulting in reduced costs and improved delivery times.
The growth of omnichannel retailing has further emphasised the need for seamless integration between online and offline channels. Customers expect a consistent and convenient shopping experience regardless of the channel they choose. To meet this demand, retailers are investing in Retail Supply Chain Management systems that can synchronise inventory levels, order fulfilment, and customer data across all channels. This integration ensures that customers can easily purchase products online and pick them up in-store or have them delivered to their doorstep.
E-commerce has significantly impacted traditional brick-and-mortar stores, leading to increased pressure on supply chains to become more flexible and agile. Retailers are adopting new strategies such as drop shipping and micro-fulfillment at distribution centres, to reduce inventory holding costs and speed up delivery times. These approaches require real-time visibility and coordination throughout the supply chain to ensure that products reach customers quickly and efficiently.
Sustainability has become a critical consideration in Retail Supply Chain Management. Consumers are increasingly demanding products that are produced ethically and with minimal environmental impact. Retailers are responding by partnering with suppliers and chain partners who share their commitment to sustainability and implementing eco-friendly practices throughout their supply chains. This includes reducing packaging waste, optimising transportation routes, and using renewable energy sources.
Blockchain technology is also gaining attention as a means to improve supply chain transparency and traceability. By providing a secure and immutable record of transactions, blockchain can help retailers track the movement of goods from their origin to the end consumer. This enhances product authenticity, reduces the risk of counterfeiting, and enables faster product recalls in case of quality or safety issues.
Essentially, the future of Retail Supply Chain Management is characterised by the adoption of innovative technologies in warehouse automation, omnichannel integration, e-commerce adaptation, sustainability practices, and the exploration of blockchain technology. Retailers who embrace these trends and invest in efficient and resilient supply chains will be well-positioned to meet the evolving demands of consumers and stay ahead of the competition.
LIKE.TG and Retail Supply Chain Management
As the retail industry grows and changes, technology plays a main part in optimising supply chain management. Retail Supply Chain Management involves a complex network of processes, people, and technology that bring products from suppliers to customers. LIKE.TG, a leading customer relationship management (CRM) platform, offers a comprehensive suite of tools that can significantly enhance retail supply chain management.
LIKE.TG’s supply chain management solutions provide retailers with real-time visibility into their inventory levels, order fulfilment status, and transportation activities. This enhanced visibility enables retailers to make informed decisions, optimise inventory levels, and inventory costs, and reduce the risk of stockouts. LIKE.TG also streamlines order management processes by automating order capture, processing, and fulfilment, resulting in faster and more accurate order fulfilment.
LIKE.TG offers robust transportation management capabilities that help retailers optimise their logistics operations. Retailers can utilise LIKE.TG to mine inventory data, plan and execute shipments, track shipments in real time, and manage carrier relationships. These features enable retailers to reduce transportation costs, improve delivery times, and provide a superior customer experience.
One of the key advantages of using LIKE.TG for Retail Supply Chain Management is its ability to integrate with other supply chain management systems. Retailers can seamlessly integrate LIKE.TG with their existing enterprise resource planning (ERP) systems, warehouse management systems (WMS), and transportation management systems (TMS). This integration ensures a unified view of the entire supply chain, eliminates data silos and facilitates smoother information flow.
Another advantage of using LIKE.TG is its cloud-based platform. LIKE.TG’s cloud-based solutions offer retailers flexibility, scalability, and accessibility. Retailers can access their supply chain management data and insights from anywhere, anytime, using any device with an internet connection. This flexibility allows retailers to make real-time decisions and respond swiftly to changing market dynamics.
By leveraging LIKE.TG’s supply chain management solutions, retailers can gain a serious competitive advantage and edge in the market. Improved visibility, reduced costs, enhanced customer service, and the ability to integrate with other systems empower retailers to streamline their supply chain operations and deliver exceptional customer experiences.
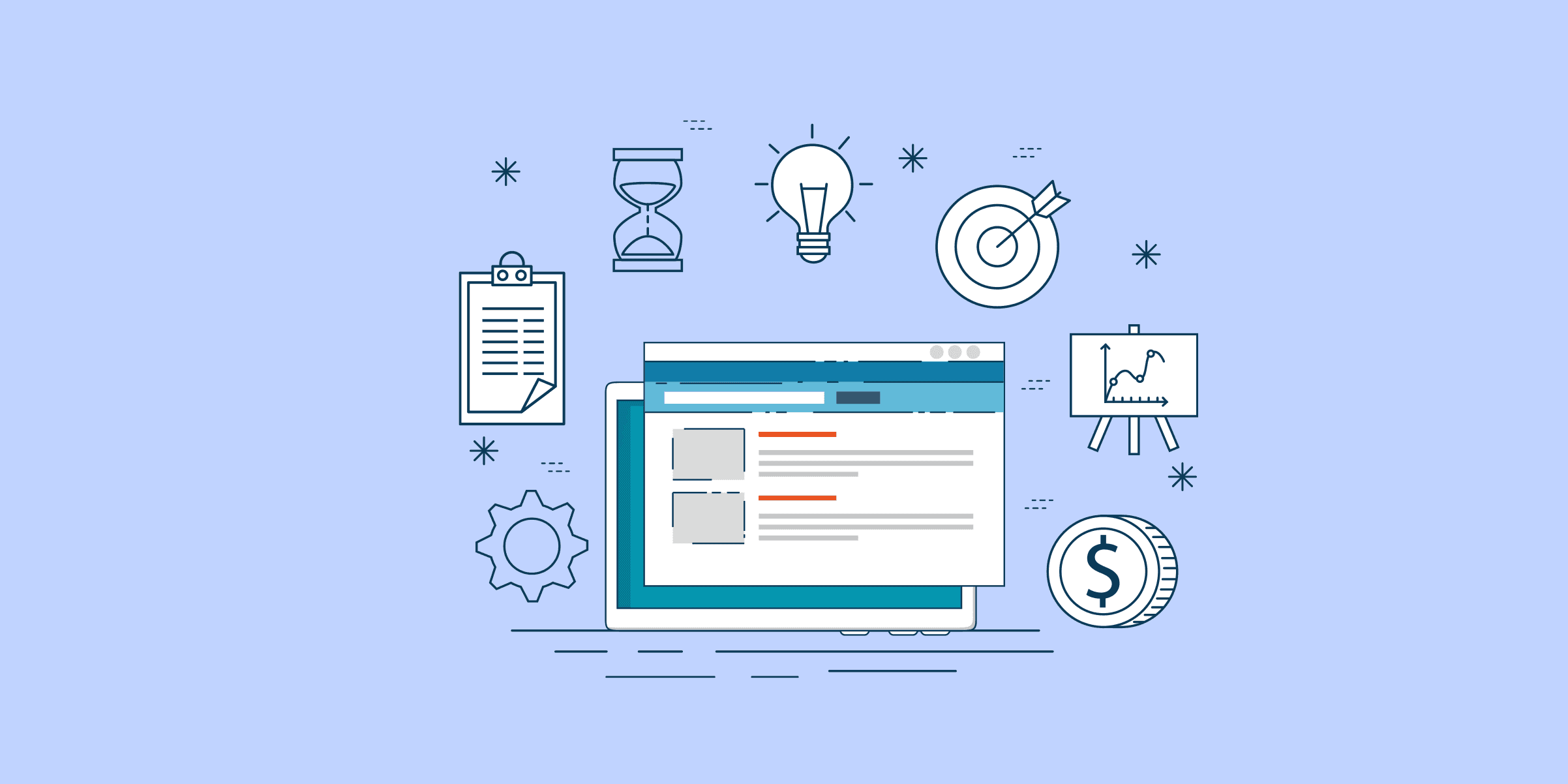
What is Leadership?
The concept of leadership has undergone significant transformations in recent years, moving away from the traditional command-and-control approach towards a more inclusive and empowering style. Defining leadership requires establishing a shared understanding of the connection between leadership vision, language, and behaviours to critical business needs, focusing on outcomes such as direction, alignment, and commitment in a social process. Today’s leaders face unique challenges in fostering engagement and collaboration in hybrid workplaces, where remote work and technology play a prominent role. This article delves into the evolving nature of leadership, exploring the limitations of conventional management styles, the impact of new approaches on teams, and the distinctive dynamics of leadership in hybrid environments. Additionally, we will examine the role of CEOs in navigating this changing landscape and the significance of leadership development through mentorship, sponsorship, and apprenticeship. Join us as we explore the intricacies of effective leadership in the modern workplace.
How is leadership evolving?
Today’s modern workplace varies greatly from that of the past. Leadership has undergone a profound transformation. The traditional command-and-control approach, where leaders held all the power and made all the decisions, has given way to a more empowering and inclusive style of leadership known as servant leadership. This shift has revolutionised the way teams operate, giving employees greater autonomy and a sense of ownership over their work and decision-making.
The rise of diverse and inclusive workplaces has redefined the very essence of leadership. Leaders today must be able to foster an environment where everyone feels valued and respected, regardless of their background, beliefs, or experiences. This requires that great leaders make a conscious effort to create an inclusive culture that celebrates diversity and leverages the unique strengths of each individual.
The increasing complexity of global business has further amplified the demands placed on leaders. In a world where borders are becoming increasingly blurred and competition is intensifying, leaders must possess strong cross-cultural competence and adaptability. They need to be able to navigate varying cultural norms, understand different business practices, and build bridges across different regions and countries. Flexible leaders are essential in navigating these diverse cultural norms and adapting to different business practices. Only then can they effectively lead their teams and organisations to success in the global arena.
Last but not least, the rapid pace of technological advancement has demanded a new breed of leaders who embrace innovation and are comfortable with ambiguity. In an era of constant disruption, leaders must be able to think outside the box, challenge the status quo, and adapt quickly to changing circumstances. They must be willing to experiment, take calculated risks, and learn from both successes and failures. Only by embracing innovation and ambiguity can leaders stay ahead of
What are the limits of traditional management styles?
Traditional management styles, often rooted in strict hierarchies and command-and-control approaches, face several limitations in the modern workplace. These styles assume that employees are primarily motivated by financial incentives and that a top-down approach to decision-making is the most effective way to achieve organisational goals. However, these assumptions are outdated and ineffective in motivating today’s employees who seek purpose, shared values, and opportunities for growth and development.
Traditional management styles often stifle innovation and adaptability, which are essential for success in today’s rapidly changing business environment. These same leadership styles tend to discourage employee input and creativity, leading to a lack of fresh ideas and solutions. Traditional management structures can also be inflexible and slow to respond to market changes, making it difficult for organisations to stay competitive.
Traditional management styles can create barriers to diversity and inclusion, hindering organisations from fully leveraging the talents and perspectives of their workforce. These styles often perpetuate biases and favouritism, creating an environment where employees from underrepresented groups may feel marginalised or undervalued. This not only undermines employee morale and productivity but also limits the organisation’s ability to attract and retain top talent.
Essentially, traditional management styles are limited in their ability to motivate employees, foster innovation and adaptability, and create inclusive work environments. To thrive in the modern workplace, organisations need to embrace new leadership approaches that empower employees, promote collaboration, and value diversity and inclusion.
How does a new approach to leadership impact teams?
When organisations adopt a new approach to leadership, the impact on teams can be transformative. By shifting from traditional, top-down management styles to more collaborative and empowering approaches, leaders can unlock the full potential of their teams and drive exceptional results.
It is so necessary to develop leadership skills that can adapt to any situation or need. Various leadership models such as servant leadership, affiliative and democratic styles, and commanding or visionary models contribute to the development of strong leadership skills.
One of the most significant impacts of a new leadership approach is the increased employee engagement and collaboration it fosters. When leaders focus on empowering employees, valuing their input, developing leaders and creating a sense of shared purpose, team members become more invested in their work and more willing to contribute their ideas and expertise. This collaborative environment encourages open communication, knowledge sharing, and mutual support, leading to improved problem-solving and decision-making.
A new leadership approach empowers employees to take ownership of their work and make decisions. When leaders trust their team members and delegate responsibilities, it instils a sense of autonomy and accountability, motivating employees to take initiative and drive projects forward. This empowerment fosters a culture of continuous learning and improvement, as team members are encouraged to experiment, innovate, and take calculated risks.
A new approach to leadership also creates a more positive and productive work environment. By prioritising employee wellbeing, fostering open communication, and promoting a healthy work-life balance, leaders cultivate a supportive and nurturing culture. This positive environment enhances employee morale, reduces stress, and increases job satisfaction, ultimately leading to improved team performance and retention of top talent.
In conclusion, a new approach to leadership has a profound impact on teams. By embracing collaboration, empowerment, and a positive work environment, leaders can unlock the collective potential of their teams, driving innovation, achieving results, and fostering a culture of continuous growth and success. This transformative shift in leadership is essential for organisations that seek to thrive in today’s dynamic and competitive business landscape.
How does leadership differ in hybrid workplaces?
In hybrid workplaces, leadership takes on a unique set of challenges and requires a different approach to effectively manage and motivate employees. One key difference lies in providing consistent support for remote workers. Leaders must ensure that remote employees have the resources and support they need to succeed, including access to necessary technology, clear communication channels, and opportunities for collaboration. Establishing clear expectations and guidelines for remote work is also essential to maintain productivity and accountability.
Fostering a culture of trust and accountability is crucial in hybrid work environments. Since remote employees may not be physically present in the office, building trust and ensuring accountability require conscious effort from leaders. Regular check-ins, open communication, and setting clear performance metrics can help maintain a high level of accountability. Additionally, leveraging technology to facilitate communication and collaboration among remote and in-office teams is vital. Video conferencing, project management tools, and instant messaging platforms can help bridge the gap and create a cohesive work environment.
Inspirational leaders provide a rich picture of what the future will look like when their visions have been realised. They tell inspiring stories and explain their visions in ways that everyone can relate to.
By understanding these key differences and adapting their leadership style accordingly, leaders can be more effective leaders in hybrid work environments. They can create an inclusive and supportive culture that fosters engagement, collaboration, and productivity, regardless of employees’ physical location. In this evolving landscape of leadership, leaders who embrace these changes and adapt their approach will be well-positioned to lead their teams to success in hybrid workplaces. Leadership, in its essence, is about inspiring and guiding individuals to achieve shared goals, and in hybrid workplaces, this takes on a new dimension that requires leaders to embrace technology, build trust, and foster a sense of community among their leadership teams.
How should CEOs lead in this new world?
CEOs are all-important in shaping the culture and success of their organisations, especially in the modern workplace. To effectively lead in this ever-changing landscape, CEOs must adopt new leadership strategies and embrace a leadership style that prioritises growth, wellbeing, and innovation. Leadership lies in its ability to get things done, allowing for clear communication of vision, uniting teams, fostering creativity and innovation, and ultimately improving the company’s bottom line.
First and foremost, CEOs should cultivate a growth mindset, fostering a culture of continuous learning and development. This involves encouraging employees to embrace new challenges, experiment with innovative ideas, and seek out opportunities for professional growth. By providing access to training, mentorship, and resources, CEOs can empower the best leaders on their teams to reach their full potential and drive the organisation forward.
Prioritising employee wellbeing is another key aspect of effective leadership in the modern workplace. CEOs should recognise that happy and healthy employees are more productive and engaged. This involves creating a positive and supportive work environment, offering flexible work arrangements, and implementing policies that promote work-life balance. By prioritising employee wellbeing, CEOs can boost morale, reduce turnover, and create a more productive and innovative workforce.
Fostering open communication is crucial for building trust and maintaining a healthy organisational culture. CEOs should encourage open dialogue, listen actively to employee feedback, and be transparent in their communication. This creates an environment where employees feel valued, respected, and empowered to share their ideas and concerns. By fostering open communication, CEOs can make informed decisions, address issues promptly, and maintain a positive work atmosphere.
Leading by example is a powerful way for CEOs to inspire and motivate their teams. They say successful leaders should embody the values and behaviours they expect from their employees, demonstrating integrity, authenticity, and a commitment to excellence. When CEOs lead by example, they set a high standard of performance and create a culture where employees are inspired to follow suit. This fosters a positive work environment, increases employee engagement, and drives organisational success.
Finally, CEOs should continuously invest in learning and development to stay ahead of the curve and adapt to the evolving business landscape. This involves keeping up with industry trends, exploring new technologies and management skills, and seeking out opportunities for personal growth. By continuously learning and developing, CEOs can remain relevant, make informed decisions, and effectively guide their organisations through the challenges and opportunities of the modern workplace.
CEOs who embrace a growth mindset, prioritise employee wellbeing, foster open communication, lead by example, and invest in continuous learning can effectively navigate the modern workplace and drive their organisations towards success. By adopting these strategies, CEOs can create a positive and productive work environment that fosters engagement, collaboration, and innovation, ultimately achieving exceptional results and maintaining a competitive edge in today’s dynamic business landscape.
What is leadership development?
Leadership development is a crucial investment that organisations make to enhance their leadership capabilities. It involves a combination of formal training, practical experiences, various leadership programs and the invaluable guidance of mentors, sponsors, and apprenticeship opportunities. These elements work together to propel individuals toward successful leadership roles within the organisation.
Mentorship involves a one-on-one relationship where a more experienced individual (the mentor) shares their knowledge, skills, and insights with a less experienced individual (the mentee). The mentor provides guidance, advice, and support to help the mentee develop their leadership capabilities and navigate the challenges they face. Mentorship is often informal and can occur within or outside the organisation.
Sponsorship, on the other hand, is a more structured and influential relationship. Sponsors are typically senior leaders within the organisation who use their influence and resources to support and advocate for the career advancement of their protégés. Sponsors provide visibility, opportunities, and access to networks that can accelerate the protégé’s career growth. Sponsorship is crucial for individuals who aspire to reach higher leadership and management positions.
Apprenticeship, in contrast, involves learning a trade or skill under the supervision of a skilled craftsperson or professional. Apprentices work closely with their masters to acquire practical knowledge and expertise in a particular field. This hands-on approach allows apprentices to develop their skills and gain valuable experience that can lead to successful careers.
These three approaches to leadership development offer distinct benefits and contribute to the growth of future leaders. Mentorship provides personalised guidance and support, sponsorship accelerates career advancement, and leadership skills while apprenticeship cultivates practical skills and expertise. By embracing these approaches, organisations can build a strong pipeline of talent and develop leaders who are equipped to meet the challenges within business.
How do mentorship, sponsorship, and apprenticeship differ?
Mentorship, sponsorship, and apprenticeship are three distinct forms of professional development and career growth. While often used interchangeably, each offers unique roles, responsibilities, and benefits. Understanding these differences is crucial for individuals seeking guidance and organisations aiming to foster a culture of learning and growth.
Mentorship involves a relationship between a more experienced individual (the mentor) and a less experienced one (the mentee). The mentor provides guidance, advice, and support to help the mentee navigate their career, develop skills, and achieve their goals. Mentorship is often informal and based on shared interests or expertise.
Sponsorship, on the other hand, is a more active and influential relationship. Sponsors use their power and influence within an organisation to advocate for and support the career advancement of their protégés. They provide opportunities, visibility, and access to networks that the protégé might not otherwise have. Sponsorship is often formal and involves a commitment from both parties.
Apprenticeship is a structured learning experience where an apprentice works closely with a skilled craftsperson or professional to learn a specific trade or skill. Apprenticeships typically involve hands-on training, mentorship, and a formal agreement outlining the terms of the relationship. They provide a pathway to skill development and mastery in various fields.
In summary, mentorship focuses on personal and professional development, sponsorship accelerates career advancement, and apprenticeship imparts specialised skills and knowledge. By recognising these distinctions and cultivating these relationships, individuals can unlock their potential, organisations can develop their talent pipeline, and the concept of leadership can be further enhanced.
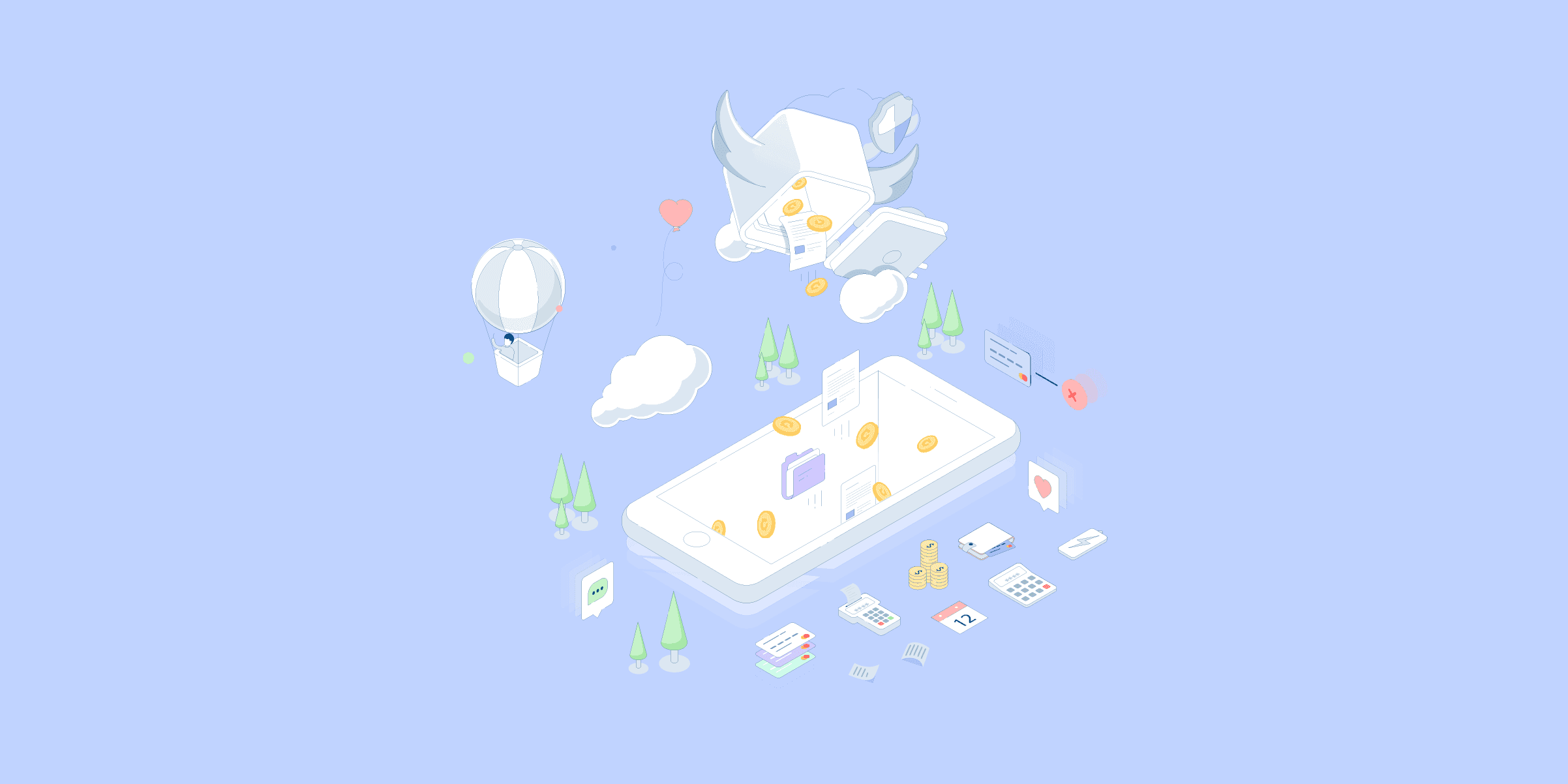
What is public administration?
Public administration and government administration play a major role in shaping our communities and influencing our daily lives. From managing public resources and implementing policies to ensuring efficient governance and societal progress, public administration stands as a vital field that touches every aspect of our society. Throughout our guide, we’ll look at the intricacies of public administration, exploring its definition, significance, historical evolution, and the career opportunities it offers.
Public administration: defining the term
At its core, public administration is the management of public resources and services. It encompasses a wide range of activities, from the provision of essential services like education, healthcare, and infrastructure to the regulation of industries and the implementation of public policies.
A function of public administration is the implementation of public policy. Public policy refers to the decisions and actions taken by governments to address societal issues and achieve desired outcomes. Public administrators are responsible for executing and managing government programs that aim to address societal issues and achieve desired outcomes. Public administrators are essential for translating these policies into concrete actions and programs. They develop and manage budgets, allocate resources, and oversee the implementation process to ensure that policies are effectively carried out.
Public administration is also a field of study that focuses on the organisation and management of government agencies. Public administration also encompasses public management, which involves the application of private-sector management techniques to improve the efficiency and effectiveness of government operations. Public administrators analyse and design organisational structures, develop management systems, and implement best practices to improve the efficiency and effectiveness of government operations. They work to ensure that government agencies are responsive to the needs of the public and accountable for their actions.
Public administration is essential for the effective functioning of government. Without it, governments would be unable to provide essential services, implement policies, or manage their resources effectively. Public administrators play a vital role in ensuring that governments are transparent, accountable, and responsive to the needs of the public.
Public administration is a challenging and rewarding career. It offers opportunities to make a positive impact on society and to work with a diverse group of people. Public administrators have the chance to work on a wide range of issues and projects, from environmental protection to social justice to economic development. They have the opportunity to make a real difference in the world and to leave a lasting legacy.
Importance of Public Administration in the Public Sector
Public administration is the backbone of modern society, playing a pivotal role in ensuring societal progress and wellbeing. Its significance lies in the wide range of functions it performs, the services it provides, and the tangible impact it has on our daily lives.
One of the primary reasons for public administration’s importance is its role as a service provider. It oversees the provision of essential infrastructure, such as roads, bridges, and public transportation, facilitating mobility and economic activity. Public administration also ensures access to quality education and healthcare, empowering citizens to reach their full potential and live fulfilling lives.
Beyond service delivery, public administration acts as a catalyst for policy-making and implementation. Through its expertise in public policy analysis and program development, it ensures that policies are well-informed, effective, and responsive to society’s needs. Public administration also plays a crucial role in foreign affairs, managing international assistance and diplomatic efforts. Public administration translates policies into actionable plans and supervises their implementation, bridging the gap between theory and practice.
Public administration is also a staunch advocate for transparency, accountability, and ethical governance. It establishes mechanisms for public participation in decision-making processes, ensuring that citizens have a say in matters that affect them. By promoting transparency and integrity, public administration fosters public trust and confidence in the government, strengthening the foundations of democracy and good governance.
Public administration is also a key player in economic development and social progress. Public administration often collaborates with international organisations to address global challenges and promote sustainable development. It fosters an environment conducive to business growth and individual aspiration. By developing regulations that protect consumers and promote fair competition, public administration creates a level playing field for economic prosperity. Additionally, it invests in initiatives that address social challenges, such as poverty alleviation, environmental protection, and social welfare programs, promoting a more equitable and compassionate society.
To sum it up, public administration is an indispensable field that plays a multifaceted and crucial role in society. Its importance lies in its ability to provide essential services, drive policy-making, foster good governance, contribute to economic development and social progress, and adapt to changing circumstances. Public administration is the basis of a well-functioning society and offers rewarding career opportunities to individuals passionate about making a positive impact on the world.
History of Public Administration
Public administration has a rich and varied history, with its roots reaching back to the earliest forms of organised governance in ancient civilisations. Throughout history, there have been numerous examples of public administration practices, such as the construction of public works, the collection of taxes, and the provision of essential services. However, it wasn’t until the 19th century that public administration emerged as a distinct field of study.
The development of public administration as a discipline was influenced by several key factors, including industrialisation, urbanisation, and the expansion of the welfare state. Industrialisation and urbanisation led to an increase in the complexity of society and a growing demand for public services. The establishment of a higher civil service with rigorous entry requirements and professional training became a hallmark of modern public administration. This, in turn, necessitated the development of more efficient and effective methods of public administration. Additionally, the expansion of the welfare state in the late 19th and early 20th centuries further increased the role of government in society and created a need for professionals who could manage and deliver public programs.
In the United States, the Progressive Era played a particularly significant role in shaping the field of public administration. During this period, there was a widespread belief that the government should be more efficient, effective, and responsive to the needs of the people. This led to the development of new approaches to public administration, such as the use of scientific management techniques and the creation of professional associations. One prominent figure in the development of public administration during this time was Woodrow Wilson, who advocated for treating public administration as a separate discipline from politics. Wilson’s ideas helped to establish public administration as a distinct field of study and laid the foundation for its continued growth and development.
Today, public administration is a well-established field with a rich history and a wide range of career opportunities. Public administrators play a prominent part in managing public resources, implementing public policies, and ensuring the effective functioning of government. The field continues to evolve and adapt to meet the changing needs of society, and it remains an essential component of modern governance.
Types of Public Administration in Government Agencies
Public administration encompasses an array of functions and responsibilities, leading to the emergence of various types based on their scope, functions, and levels of government. Each category plays a unique role in managing public affairs and delivering essential services to the community.
One primary distinction lies in the functional areas of public administration. Public administration programs provide specialised training in areas such as policy analysis, financial management, and human resources management. These areas include general administration, financial management, human resources management, policy analysis, and program evaluation. Each functional area requires specialised expertise and knowledge, to ensure the effective and efficient operation of public organisations.
Another classification of public administration is based on the level of government. Public administration can be categorised as federal, state, or local. Federal public administration pertains to the management of public affairs at the national level. State public administration focuses on the administration of public programs and services within individual states. Local public administration, on the other hand, deals with the management of public resources and services at the city or county level.
Public administration can also be categorised based on its scope and focus. For instance, there is public administration in the areas of education, healthcare, transportation, environmental protection, and public safety. Each of these specialised fields requires a deep understanding of the specific sector’s policies, regulations, and challenges.
Understanding these different types of public administration is crucial for grasping the complexities and diversity of the field. Each category contributes to the effective functioning of government and the provision of essential services that impact the lives of citizens daily.
What Can I Do With a Public Administration Programs Degree?
With a public administration degree, you’ll have the opportunity to embark on a diverse and fulfilling career path that allows you to make a meaningful impact on society. The skills and knowledge you acquire in public administration, such as policy analysis, budgeting, and project management, are highly sought after in various sectors beyond traditional government agencies. Let’s explore some exciting career options that await you with a public administration degree.
In the public sector, you can work at the local, state, or federal level, contributing to policy development and implementation. You might find yourself managing community development programs, overseeing public works projects, or analysing data to inform policy decisions. If you’re passionate about advocacy and community service, non-profit organisations offer opportunities to work on causes close to your heart, such as environmental protection, social welfare, or education.
Beyond the public and non-profit sectors, the private sector also values the skills of public administration graduates. Many companies seek individuals with a deep understanding of public policy and regulations to navigate complex business environments. You could work in human resources, public relations, or consulting, providing valuable insights and strategies to organisations looking to engage with the public sector or manage their social responsibilities.
If you’re drawn to academia, a public administration degree can prepare you for a rewarding career as a professor or researcher, contributing to the advancement of knowledge in the field. Additionally, international development organisations offer opportunities to work on projects that address global challenges and promote sustainable development. A background in international relations can be particularly valuable for those looking to work on global public administration projects and diplomatic initiatives. With your public administration expertise, you can make a difference on a global scale, supporting communities in need and fostering positive change.
Remember, these are just a few examples of the many career paths available to public administration graduates. With your passion, dedication, and the versatile skills you gain, you can shape your career to create positive change in any sector that interests you. Embrace the opportunities that await you and embark on a journey of impactful public service or leadership in the private sector.
Public Administrators Career Outlook
The field of public administration offers a compelling career outlook for professionals seeking meaningful and impactful work. The demand for skilled public administrators is growing steadily due to the increasing complexity of public policies, the need for effective resource management, and the emphasis on improving public services. This demand creates a favourable job market with ample opportunities for career advancement and specialisation.
Public administration professionals are rewarded with competitive salaries that reflect the importance of their roles in society. The compensation packages often include benefits such as health insurance, retirement plans, and professional development opportunities. The financial rewards of a public administration career, combined with the intrinsic satisfaction of making a positive difference, make it an attractive choice for individuals seeking both personal and professional fulfilment.
The diverse work settings in public administration offer a wide range of opportunities to match individual interests and skills. Public administrators may work in federal, state, or local government agencies, as well as in nonprofit organisations and international institutions. They may specialise in areas such as public policy, budgeting, human resources, urban planning, or environmental management. This variety of work environments ensures that public administration professionals can find roles that align with their passions and expertise.
One of the most rewarding aspects of a public administration career is the chance to make a positive impact on society. Public administrators play a crucial role in shaping public policies, implementing programs, and managing resources that directly affect the lives of citizens. They have the opportunity to address pressing social challenges, promote equity and justice, and contribute to the overall wellbeing of their communities. The sense of fulfilment that comes from making a meaningful difference in the world is a key motivator for many public administration professionals.
Public administration also offers excellent opportunities for career growth and advancement. With experience and proven competence, public administrators can move up the ranks within their organisations or pursue leadership positions in other sectors. The transferable skills and knowledge gained in public administration, such as policy analysis, project management, and communication, are highly valued across various fields. This opens up possibilities for career diversification and further professional development.
The public administration career outlook is bright, with growing demand, competitive salaries, diverse work settings, opportunities for societal impact, and potential for advancement. It is a rewarding field that attracts professionals who are passionate about making a positive difference and shaping a better future for their communities. For those seeking a meaningful and impactful career, public administration offers a compelling path to professional success and personal fulfilment.
Frequently Asked Questions about Public Administration
To deepen our understanding of public administration, let’s delve into some frequently asked questions about this multifaceted field.
One common query is the distinction between public administration and political science. While both disciplines are concerned with governance, public administration focuses on the practical aspects of managing public resources and implementing policies. Political science, on the other hand, examines the theoretical foundations of government and political behaviour. Public administrators are the ones who put policies into action, while political scientists analyse and critique the systems that produce those policies.
Another question that arises is the nature of challenges faced by public administration in today’s world. In an era of globalisation, technological advancements, and rapidly evolving societal expectations, public administration must adapt to keep pace with these changes. Balancing the demand for efficiency with the need for inclusivity and social justice poses a significant challenge. Additionally, the increasing complexity of public policy issues, such as climate change and healthcare, requires public administrators to possess a broad range of expertise.
Many individuals are curious about the diverse career paths available in public administration. The field offers a wide array of opportunities, from policymaking and program implementation to budgeting and project management. Public administrators can work in various settings, including government agencies, non-profit organisations, and private sector entities involved in public service delivery. Some specialised roles within public administration include urban planning, public finance, human resources management, and international development.
To be successful in public administration, certain essential skills are required. Effective leadership is crucial for managing teams and achieving organisational goals. Analytical thinking and problem-solving abilities are necessary for navigating complex policy issues. Strong communication skills are vital for interacting with stakeholders, building consensus, and conveying ideas effectively. Additionally, an understanding of budgeting, financial management, and research methods is often advantageous.
Finally, it is worth considering the future of public administration and how it may evolve in the coming years. Emerging trends, such as the rise of digital governance, open data, and citizen participation, are reshaping the way public services are delivered. Technological innovations, including artificial intelligence and blockchain, have the potential to transform public administration processes. As society continues to grapple with complex challenges, public administration will play an increasingly critical role in shaping a more responsive, inclusive, and effective government.
By exploring these frequently asked questions, we gain a deeper appreciation for the multifaceted nature of public administration and its vital role in shaping our communities and society at large.
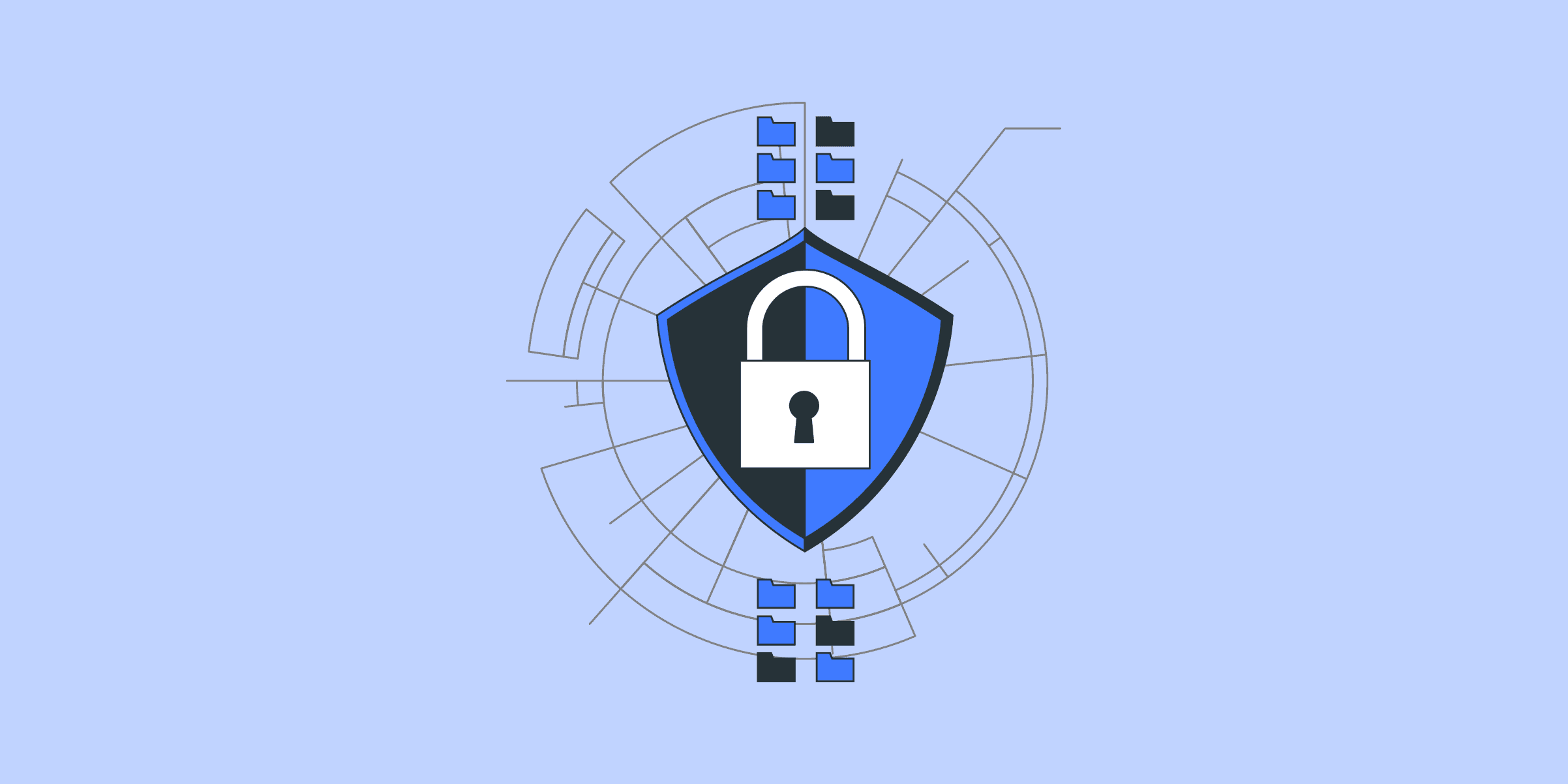
50 Sales Statistics that Reveal How Great Teams Sell
Read on to discover the most important sales statistics that reveal how the greatest teams are pushing forward. The data shows there are many bright spots to be found, but a nuanced picture emerges when we look more closely. Yes, revenue is up, but reps are struggling to meet quota. AI is yielding benefits, but data concerns remain. Employee retention is high, but fragile and dependent on high pay.Sales statistics about revenue growthIn the previous State of Sales report, conducted during the pandemic, 82% of sales professionals said they had to quickly adapt to new ways of selling in the face of economic headwinds. Today, that hard work has paid off. Sales are growing this year, partly thanks to recurring revenue and partner sales.Growth is on the rise1. 79% of sales leaders and managers say revenue increased over the past year.2. 78% of sales leaders and managers say new customer acquisition increased over the past year.3. 82% of sales leaders and managers feel confident about their company’s 12-month growth strategy4. The top 3 tactics for growth among sales leaders: Improving sales enablement and training, targeting new markets, and improving use of tools and technologies.One-off sales take a back seat to recurring revenue5. Over 90% of sales teams use more than one revenue source.6. 42% of sales leaders and managers say recurring sales is their top revenue source.7. Sales leaders and managers say one-off sales is their third top revenue source, after recurring sales, upsells, and cross-sells.Within 12 months, nearly every company expects to use partner sales8. 83% of sales pros say partner selling has a bigger impact on revenue than a year ago.9. 89% of sales teams currently use partner sales.10. Of those sales teams who don’t use partner sales, 58% expect to within a year.Sales statistics about challengesChanging customer expectations is the number one challenge today. Increasing marketplace competition is adding to the squeeze. Meanwhile, buyers are demanding more personalization than before. But even as sellers are asked to deliver more value, they get trapped in non-selling tasks.Sellers feel the pressure11. 67% of sales reps don’t expect to meet their quota this year, and 84% missed it last year.12. 53% of sales pros say it’s harder to sell than a year ago.Changing customer expectations and marketplace competition are coming in hot13. Sales leaders and managers say their top challenge is changing customer needs and expectations (for example, demanding lower costs, deeper understanding, and greater value).14. 57% of sales leaders and managers say competition has increased since last year.15. Similarly, macroeconomic conditions and inflation still pose a major challenge but is decreasing, with 27% of sales leaders and managers saying it’s a challenge compared to 39% in 2022.16. 78% of business buyers say their company is more careful about spending money than before.*17. 76% of business buyers say their company extracts maximum value from every purchase.**This data comes from the LIKE.TG State of the Connected Customer report.Buyers demand personalization*18. 86% of business buyers are more likely to buy if companies understand their goals.19. But 59% say most sales reps don’t take the time to understand them.20. 84% of business buyers expect sales reps to act as trusted advisors.21. But 73% say most sales interactions feel transactional.*The data in this section comes from from the LIKE.TG State of the Connected Customer report.Sales reps still have productivity woes22. Reps spend 70% of their time on nonselling tasks — a figure that is virtually unchanged from the 2022 State of Sales report, when reps spent 72% of their time on nonselling tasks.23. The tasks that take the most time to complete are meeting customer budget needs, personalizing communication, and building a strong personal relationships.Sales statistics about data and AIFour in five sales teams use AI today. As adoption becomes widespread, sales teams are experiencing powerful benefits like accelerated growth and productivity gains. But data concerns like trust and training gaps are preventing teams from making the most out of AI, and many sales pros fear they’re missing out.Sales teams are benefiting from AI, but obstacles remain29. 81% of sales teams are investing in AI. Of those, about half are experimenting with AI and the other half have fully implemented it.30. 83% of sales teams with AI saw revenue growth in the past year — versus 66% of teams without AI.31. The top 3 areas that AI is improving are sales data quality and accuracy, understanding customer needs, and personalization for customers.32. AI-using sales teams are 1.4x more likely to say headcount increased over the past year33. 33% of sales ops pros using AI say they have insufficient budget, headcount, and training on how to use it.34. Among teams currently using AI, sales ops pros say the top tactic to prepare is to consolidate tools and tech stacks.The focus turns to building a foundation of trustworthy data35. 98% of sales leaders say trustworthy data is more important in times of change.*36. 94% of sales leaders say their organization should be getting more value from their data.*37. 78% of sales leaders say they’re concerned about missing out on generative AI.*38. Only 35% of sales professionals completely trust the accuracy of their organization’s data.39. 73% of sales pros say generative AI introduces new security risks.**40. 49% of sales pros say they’re not sure how to safely use generative AI at work.**41. 39% of sales pros say accurate forecasting is hindered by poor data quality.**42. Among sales pros who don’t trust their organization’s data, 38% say the reason is incompleteness, 37% say it’s because it’s stored in multiple formats, and 37% say it’s because it’s not regularly updated.***This data comes from the LIKE.TG Trends in Data and Analytics for Sales report.**This data comes from the LIKE.TG Trends in Generative AI for Sales report.Sales statistics about enablement and trainingSales teams are improving their training programs for direct sellers and partners alike, a key strategy for delivering more value to customers. Most reps say their company’s enablement programs prepare them to meet quota, rating these activities as the most impactful: support materials, product-specific training, and one-on-one coaching.Enablement is the top tactic for growth24. The number one growth tactic for sales leaders and managers is improving sales enablement and training.25. The top enablement activity is sales strategy reviews, which 53% of sales teams use. The least popular activity is one-on-one meetings with managers, which only 32% have regularly.Enablement helps reps meet quota, but isn’t perfect26. 76% of reps say their enablement prepares them to make quota.27. But only 29% of reps say they are completely satisfied with enablement materials.Enablement gets a lift from AI28. Among sales teams who use AI for enablement, 70% offer real-time selling guidance, 67% offer customized enablement for individual reps, and 47% offer call coaching.Sales statistics about the employee experienceWhile employee retention has grown overall, it remains fragile. Sales pros say the top reason they’d consider changing jobs is a lack of career advancement opportunities, and the second top reason is uncompetitive pay or benefits.43. 76% of reps say leadership makes employee satisfaction a priority at their company.44. Sales teams report an 18% average turnover over the last 12 months — down from 25% in 2022.45. 81% of sales professionals say their pay has increased over the past year.46. 82% of sales reps saying they understand which metrics affect their pay.47. 71% of sales leaders and managers say overall budget is up.48. 63% of sales leaders and managers say employee headcount is up.49. 64% of sales professionals say they would leave if offered a similar job elsewhere with better pay.50. The top reasons why sales reps are considering leaving their current job are lack of career advancement, lack of clarity from leadership, and uncompetitive pay.
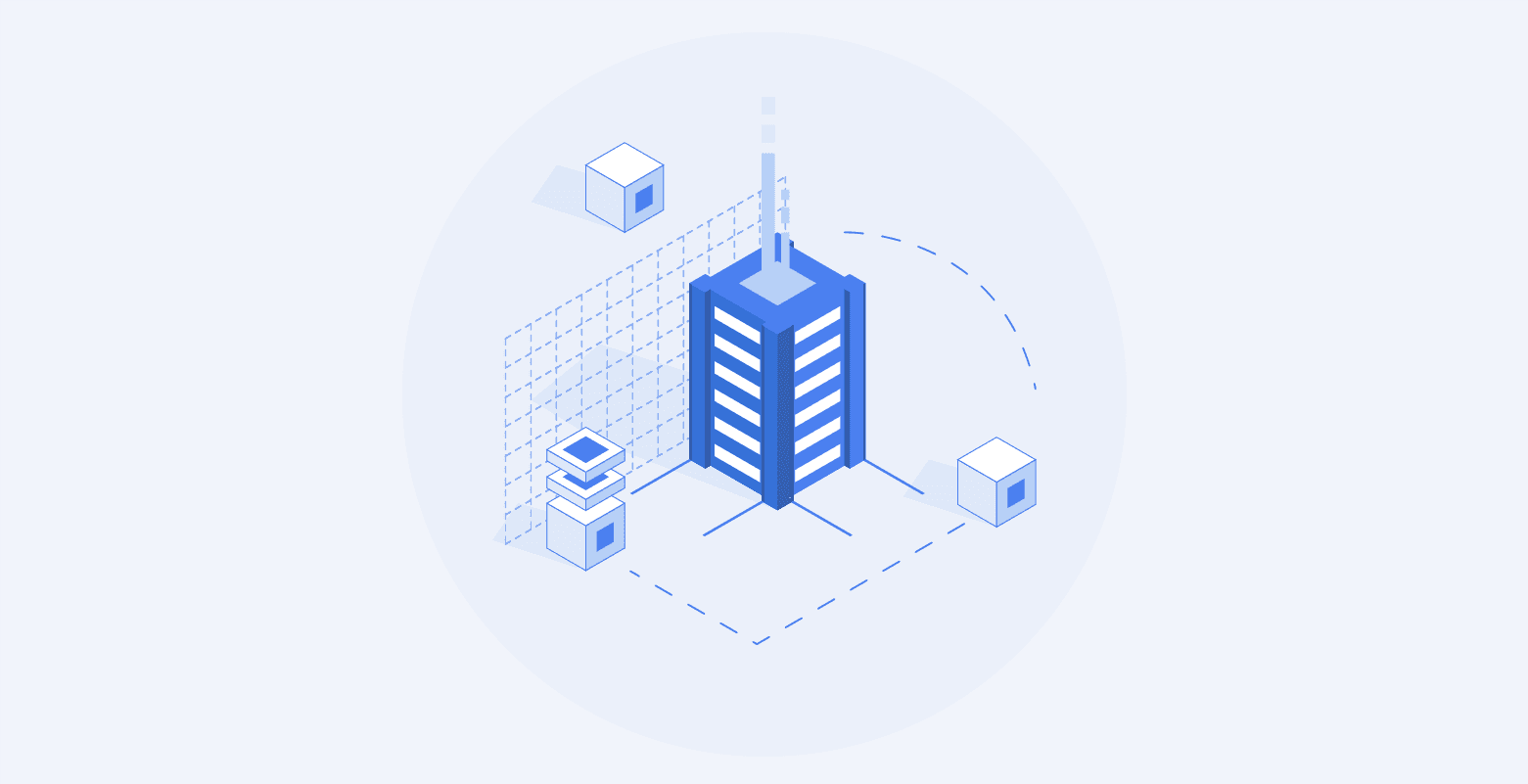
ERP vs CRM: What’s the Difference?
Leveraging technology to streamline operations and enhance customer experiences has become increasingly important as the business space becomes progressively more saturated and competitive. Customer relationship management (CRM) and enterprise resource planning (ERP) emerge as indispensable software solutions that empower businesses to achieve these goals. While CRM focuses on nurturing customer interactions and relationships, ERP streamlines internal processes, encompassing varied aspects such as supply chain management, inventory control, and financial management. Understanding the unique strengths and potential synergies of CRM and ERP is necessary for businesses seeking to thrive in the digital age. We’ll take a look into the intricacies of CRM and ERP integration, highlighting their distinct features, benefits and the transformative power of their integration.
What is Customer Relationship Management?
Within the business space, as it is today, it’s no longer enough to provide an exceptional product or service simply. Now, you must also showcase and maintain unmatched customer service to gain an edge over competitors. Customer Relationship Management (CRM) software has quickly become a powerful tool that helps businesses manage and nurture these relationships effectively. CRM systems serve as centralised platforms that capture, organise, and analyse customer interactions and data, providing businesses with valuable insights into their customers’ needs, preferences, and behaviours.
CRM systems facilitate the efficient management of customer information, encompassing contact details, purchase history, support requests, and communication history. This comprehensive data repository empowers businesses to deliver personalised and targeted customer experiences, fostering loyalty and driving business growth.
Additionally, CRM systems automate various tasks associated with managing customer data and interactions, such as sending marketing emails, tracking customer support tickets, and generating reports. These automation capabilities streamline operations, freeing up valuable time and resources that can be redirected towards enhancing customer engagement and satisfaction.
By leveraging CRM systems, businesses gain a competitive edge by improving customer service, boosting sales, and optimising marketing efforts. These systems provide a holistic view of customer interactions, enabling businesses to make informed decisions, build stronger relationships, and ultimately drive business success.
What is ERP?
In business management, the concept of ERP, or Enterprise Resource Planning, emerges as a transformative force, revolutionising how organisations manage their operations. Unlike customer relationship management (CRM) systems, which focus on interactions with external clients, ERP systems serve as comprehensive hubs, integrating various departments and functions within a company. This centralised approach allows for seamless coordination and resource management, streamlining business processes and fostering overall operational excellence.
ERP systems encompass a diverse range of modules, catering to critical business areas such as accounting and finance, supply chain management, manufacturing, human resources, project management, and more. These modules act as interconnected components, seamlessly communicating and sharing data in real-time. This interconnectedness empowers businesses with the ability to gain instant insights into their operations, enabling informed decision-making and strategic planning.
One of the key benefits of ERP systems lies in their ability to automate repetitive tasks, significantly reducing the burden of manual labour and enhancing operational efficiency. By eliminating data silos and ensuring a consistent flow of information across the organisation, ERP systems foster collaboration and communication among different departments. This interconnectedness breaks down barriers, allowing for a streamlined exchange of ideas and resources, ultimately driving organisational success.
The implementation of ERP systems provides businesses with a multitude of advantages, including optimised processes, reduced costs, and a distinct competitive edge. By offering a holistic view of the organisation’s performance, ERP systems empower data-driven decision-making and strategic planning, ensuring that businesses remain agile and responsive to market dynamics. In today’s fast-paced business landscape, ERP systems stand as indispensable tools, enabling organisations to thrive in an increasingly competitive environment.
CRM Benefits
CRM software offers numerous benefits that can significantly enhance a business’s operations, customer satisfaction, and overall success. Here are some of the key benefits of using CRM solutions and systems:
Improved customer service: CRM systems provide businesses with a centralised platform to manage customer interactions, ensuring that customers receive prompt and personalised assistance. By accessing customer information and interaction history, support teams can quickly address customer queries and concerns, leading to higher customer satisfaction and loyalty.
Increased customer retention: CRM systems enable businesses to gain a deeper understanding of their customers’ needs and preferences. This knowledge empowers businesses to tailor their products, services, and marketing strategies to meet customer expectations, resulting in increased customer retention and repeat business.
Automated sales and marketing processes: CRM systems automate various sales and marketing tasks, such as lead generation, contact management, email campaigns, and sales forecasting. This automation streamlines workflows, reduces manual labour, and allows sales and marketing teams to focus on more strategic activities that drive revenue growth.
Valuable insights into customer behaviour: CRM systems collect and analyse vast amounts of customer data, providing businesses with valuable insights into customer behaviour, preferences, and buying patterns. This information enables businesses to make data-driven decisions, optimise their marketing campaigns, and develop targeted strategies to attract and retain customers.
Enhanced collaboration and communication: CRM systems foster collaboration and communication between different departments within an organisation, ensuring customer-related information is seamlessly shared. This alignment improves the overall customer experience and allows businesses to respond to customer needs more effectively.
Increased profitability: By leveraging CRM systems, businesses can optimise their sales and marketing efforts, and sales cycle, leading to increased revenue generation. Additionally, the automation of tasks and improved customer retention contribute to cost savings, resulting in enhanced profitability for the business.
Overall, CRM systems provide a comprehensive suite of tools and functionalities that empower businesses to manage customer relationships effectively, deliver exceptional customer service, and drive business growth.
ERP Benefits
Enterprise resource planning (ERP) software can help businesses improve efficiency, productivity, and profitability in a number of ways. By streamlining business processes across multiple departments, ERP systems can help businesses reduce costs, improve customer service, and make better decisions.
One of the key benefits of ERP systems is their ability to automate repetitive tasks, which can significantly reduce the burden of manual labour and free up employees to focus on more strategic tasks. For example, ERP systems can automate tasks such as order processing, inventory management, and financial reporting. This can save businesses time and money, and it can also help to improve accuracy and consistency.
Another benefit of ERP systems is their ability to improve decision-making. By providing businesses with a single source of truth for all of their data, ERP systems can help them to make better decisions about how to allocate resources, manage inventory, and serve customers. For example, ERP systems can help businesses to identify trends in customer demand, which can help them to make better decisions about what products to produce or sell.
ERP systems can also help businesses to improve customer service. By providing customer service representatives with access to all of the information they need about a customer, ERP systems can help them to resolve customer issues quickly and efficiently. This can lead to increased customer satisfaction and loyalty.
Finally, ERP systems can help businesses to reduce costs. By automating repetitive tasks and improving decision-making, ERP systems can help businesses to save time and money. Additionally, ERP systems can help businesses to identify and eliminate waste, which can further reduce costs.
What is the Difference Between CRM and ERP?
CRM and ERP systems are both essential business software solutions, but they serve different purposes and cater to different aspects of business operations.
CRM systems are primarily focused on managing customer interactions and relationships. They provide businesses with a central platform to store and analyse customer data, track customer interactions, and manage sales and marketing campaigns. These CRM tools and systems help businesses understand their customers, personalise their experiences, and provide exceptional customer service.
On the other hand, ERP systems are more comprehensive and encompass a wider range of business functions. They integrate various modules such as accounting and finance, supply chain management, manufacturing, financial and operational systems, human resources, and project management. ERP systems provide businesses with a holistic view of their operations, streamline processes, and facilitate collaboration between different departments.
The key difference between CRM and ERP systems lies in their scope and focus. CRM systems are customer-centric, while ERP systems are business-wide. CRM systems help businesses manage customer relationships, while ERP systems help businesses manage all aspects of their operations.
In terms of users, CRM systems are primarily used by sales, marketing, and customer service teams. ERP systems, on the other hand, are used by a wider range of users across different departments, including finance, accounting, operations, and human resources.
Both CRM and ERP systems can provide significant benefits to businesses. CRM systems can improve customer satisfaction, increase sales, and optimise marketing efforts. ERP systems can streamline operations, reduce costs, and improve decision-making.
For businesses looking to improve their customer relationships and sales performance, a CRM system is a valuable investment. For businesses looking to optimise their operations and gain a holistic view of their business, an ERP system is the better choice.
CRM and ERP Similarities
Although CRM and ERP are distinct software applications, they share several similarities. Both systems help businesses manage and organise data, track customer interactions and sales, generate reports and analytics, and integrate with other business applications. Ultimately, both CRM and ERP can be used to improve customer service and satisfaction.
One of the key similarities between CRM and ERP is their focus on data management. Both systems collect and store data about customers, sales, and other business operations. This data can then be used to generate reports and analytics, which can help businesses make informed decisions about how to improve their operations.
Another similarity between CRM and ERP is their ability to track customer interactions and sales. CRM systems track customer interactions, such as phone calls, emails, and website visits. ERP systems track sales, such as orders, invoices, and payments. This information can be used to improve customer service and sales performance.
Finally, both CRM and ERP systems can be integrated with other business applications. This allows businesses to share data between different systems and improve their overall efficiency. For example, a CRM system can be integrated with an ERP system to share customer data, or an ERP system can be integrated with a financial management system to share financial data.
By understanding the similarities between CRM and ERP, businesses can make informed decisions about which system is right for them. CRM systems are best suited for businesses that need to manage customer interactions and sales. ERP systems are best suited for businesses that need to manage all aspects of their operations.
Do I need CRM or ERP or both?
Whether you need CRM, ERP, or both depends on several factors, including the size of your business, the nature of your industry, and your specific business goals.
If you are a small business with a relatively simple operation, you may be able to get by with just a CRM system. CRM systems can help you track customer interactions, manage sales opportunities, and provide customer service. However, if your business is larger or more complex, you may need an ERP system to manage all aspects of your operations, including accounting, finance, inventory, and manufacturing.
Here are some factors to consider when deciding whether you need an CRM or ERP system, or both:
The size of your business: CRM systems are typically more suitable for small businesses with a relatively simple operation. ERP systems are better suited for larger businesses with more complex operations.
The nature of your industry: CRM systems are particularly beneficial for businesses in the sales, marketing, and customer service industries. ERP systems are better suited for businesses in the manufacturing, distribution, and retail industries.
Your specific business goals: CRM systems can help you improve customer service, increase sales, and grow your business. ERP systems can help you improve efficiency, reduce costs, and make better decisions.
If you are unsure whether you need CRM, ERP, or both, it is a good idea to consult with a business software expert. They can help you assess your needs and recommend the best software solution for your business.
Ultimately, the best way to decide whether you need CRM, ERP, or both is to evaluate your business needs and goals. If you are still not sure which software solution is right for you, it is a good idea to consult with a business software expert. They can help you assess your needs and recommend the best software solution for your business.
Key Features of ERP vs. CRM
CRM and ERP systems offer a range of features that cater to the specific needs of their respective users. CRM systems prioritise customer-facing functions, such as contact management, sales tracking, and customer service. These features enable businesses to centralise customer data, streamline communication, and deliver personalised experiences.
ERP systems, on the other hand, focus on managing internal operations. They encompass modules for financial management, supply chain management, inventory control, and project management. These features help businesses optimise their processes, reduce costs, and make informed decisions based on real-time data.
The key features of CRM systems include:
Contact management: Centralises customer information, including contact details, interactions, and preferences, providing a comprehensive view of customer relationships.
Sales tracking: Allows sales teams to track leads, opportunities, and deals, enabling them to monitor progress and identify potential roadblocks.
Customer service: Offers tools for managing outstanding customer service tickets, inquiries, complaints, and feedback, ensuring prompt and effective resolution.
Marketing automation: Streamlines marketing campaigns, automates tasks, and personalised customer communications.
Analytics and reporting: Provides insights into customer behaviour, sales performance, and overall business metrics, aiding decision-making.
The key features of ERP systems include:
Financial management: Manages financial transactions, accounts payable and receivable, budgeting, risk management and forecasting, providing a clear financial picture of the business.
Supply chain management: Optimises the flow of goods and services, from procurement to delivery, ensuring efficient inventory management and timely fulfilment.
Inventory control: Tracks inventory levels, reorder points, and warehouse locations, minimising stockouts and optimising storage space.
Project management: Facilitates project planning, resource allocation, scheduling, and progress tracking, ensuring successful project execution.
Business intelligence: Offers advanced analytics and reporting capabilities, allowing businesses to analyse data, identify trends, and make data-driven decisions.
These features in both CRM and ERP systems cater to diverse user groups within an organisation. CRM systems are primarily used by sales, marketing, and customer service teams, while ERP systems serve a broader range of users, including finance, accounting, operations, and human resources departments.
Integration of ERP and CRM Systems
Integrating ERP and CRM systems can provide businesses with a unified view of their data and improve their ability to deliver exceptional customer service. This section will discuss the benefits of integrating ERP and CRM systems, including improved data management and consistency, enhanced customer service, lower customer acquisition costs, streamlined order management, and accurate inventory management.
By integrating ERP and CRM systems, businesses can ensure that customer information is consistent across all departments. This means that sales reps, marketing, and customer service teams will have access to the same up-to-date customer data, which can lead to improved customer service and increased sales. For example, if a customer calls in with a question about their order, the customer service representative will be able to access the customer’s order history and provide them with the information they need.
In addition to improving customer service, integrating ERP and CRM software systems can also help businesses streamline their order management process. When sales orders are created in the CRM system, they can be automatically transferred to the ERP system, which can then generate invoices and ship orders. This can save businesses time and money by eliminating the need for manual data entry.
Finally, integrating ERP and CRM systems can help businesses improve their inventory management. By having a single view of inventory levels, businesses can avoid stockouts and overstocking. This can lead to increased sales and reduced costs.
In conclusion, integrating ERP and CRM systems can provide businesses with a number of benefits, including improved data management and consistency across operational systems, enhanced customer service, streamlined order management, and accurate inventory management. By integrating these two systems, businesses can improve their operational efficiency and profitability.
LIKE.TG ERP integration and CRM
Businesses seeking to enhance customer service and operational efficiency can benefit greatly from integrating LIKE.TG’s CRM and ERP solutions. LIKE.TG CRM excels in managing customer interactions and fostering relationships, while LIKE.TG ERP seamlessly handles various business operations. Integrating these systems provides a comprehensive view of customers, enhancing customer service solutions and the ability to deliver personalised and exceptional service.
One significant advantage of this integration lies in automating customer service processes. When customers reach out with inquiries, representatives can swiftly access account and order information within LIKE.TG CRM, ensuring prompt and accurate responses. Moreover, LIKE.TG ERP’s inventory tracking and shipping data empower sales representatives to furnish customers with up-to-date order information, fostering trust and satisfaction.
This integration also elevates sales processes by identifying high-potential customers through data analysis of customer interactions and sales history. Armed with this knowledge, businesses can target personalised marketing campaigns, increasing the likelihood of conversions and revenue growth. Additionally, LIKE.TG ERP’s sales order management and inventory tracking capabilities ensure that businesses maintain adequate stock levels, preventing missed sales opportunities.
The synergy of LIKE.TG CRM and ERP delivers numerous benefits, including superior customer service, increased sales, and reduced operational costs. By presenting a unified customer data view, these systems empower businesses to make informed decisions, optimise processes, and provide a customer experience that stands out in today’s competitive landscape. Embracing this integration is a strategic move towards sustained growth and customer loyalty.
Businesses seeking to thrive in the modern marketplace should consider integrating LIKE.TG CRM and ERP solutions. The advantages they offer, such as streamlined customer service, data-driven sales strategies, and operational efficiency, contribute to long-term success and customer satisfaction.
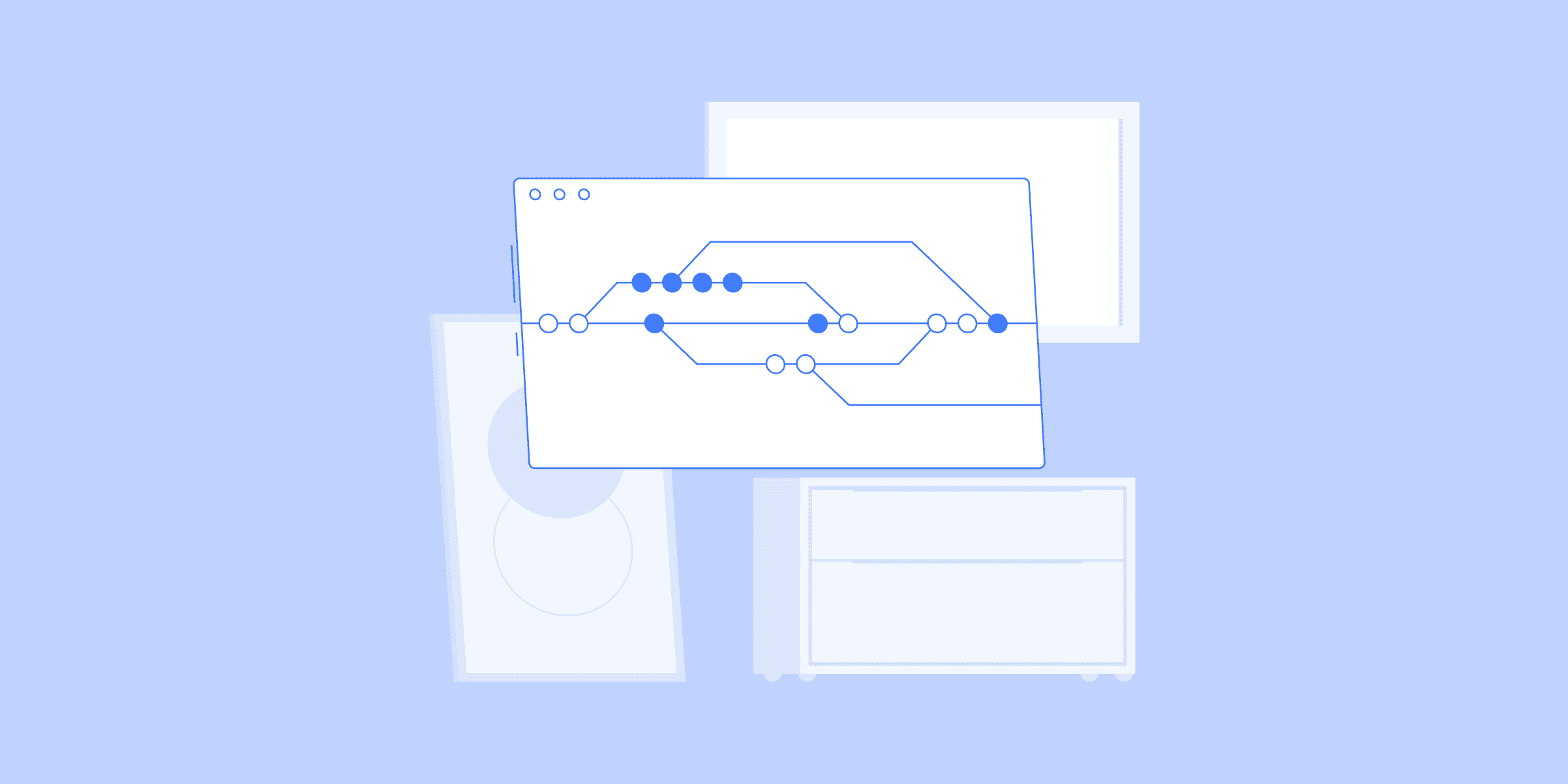
Rule the Market: 9 Retail Pricing Strategies 2024
Pricing is everything in retail. Understanding price elasticity, or how price changes affect demand, is so important for setting effective retail pricing strategies. Get it right, and you can drive sales, profit margin and customer loyalty; get it wrong, and you can quickly lose out to your competitors. Adopting a competitive pricing strategy, which involves setting prices based on market conditions to offer the lowest possible prices and monitoring competitors’ prices, can be effective but may also affect profits and customer perceptions. But with so many different pricing strategies to choose from, how do you know which one is right for your business?
Within this guide, we’ll explore 9 common pricing strategies that small businesses can use to succeed in 2024. From value-based and competition-based pricing to penetration and psychological pricing, we’ll cover it all. We’ll also provide real-world examples and expert insights to help you find the perfect pricing strategy for your target market and business goals. So, whether you’re just starting out or you’re looking to take your business to the next level, read on to learn how to rule the market with your pricing strategy.
9 Common Pricing Strategies for Small Businesses
Selecting the right pricing strategy is a game-changer within retail. To assist you in this decision, we present 9 effective discount pricing strategies that can propel your small business to success in 2024. From value-driven approaches to psychological tactics, we’ve got you covered.
Value-Based Pricing: This strategy revolves around setting prices based on the perceived value of your product or service to the customer. By understanding your target market’s preferences and willingness to pay, you can determine a price that aligns with their perceived benefits. For instance, if you offer a unique and high-quality product that solves a specific problem, you can command a premium price.
Competition-Based Pricing: Keeping an eye on your competitors’ pricing is essential. By setting your prices in line with or slightly below theirs, you can attract customers looking for a competitive deal. However, avoid engaging in wholesale price wars, as this can erode your profits. Instead, focus on differentiating your offerings through superior quality, customer service, or unique features.
Cost-Plus Pricing: This straightforward method involves adding a markup to the cost of producing your product or service to determine the selling price. While it ensures you cover your expenses and make a profit, cost-plus pricing may not always reflect the market value of your offerings. To strike a balance, consider market demand and customer willingness to pay before finalising your prices.
Penetration Pricing: If you’re looking to quickly capture market share, penetration pricing can be a powerful tool. By setting your prices significantly lower than the competition, you can entice customers to try your product or service. Once you’ve established a customer base, you can gradually increase your prices to more profitable levels.
Loss Leader Pricing: Loss leader pricing involves setting a low price on a popular item to attract customers, with the expectation that they will purchase additional items at regular prices. This strategy can drive traffic and increase overall sales.
Psychological Pricing: This strategy taps into the psychology of consumers to influence their perceptions of higher price or value. Techniques such as setting prices just below a round number (e.g., $9.99 instead of $10) or using odd-numbered prices can create the illusion of a better deal. Additionally, offering discounts, limited-time offers, or free shipping can further enhance the perceived value of your products or services.
Bundle Pricing: Bundle pricing, also known as multiple pricing, involves selling a group of products for a single price, such as a three-pack of socks or a five-pack of underwear. Retailers use bundle pricing to streamline marketing campaigns and attract customers. However, it can impact the sale of individual items and may lead to cognitive dissonance among consumers.
Keystone Pricing: Keystone pricing is a product pricing strategy in which the retail price is doubled based on the wholesale cost paid for a product. It is a simple approach to pricing, often used for products considered to be necessity items. However, it may not work for all items and could potentially lead to overpricing or underselling.
Price Skimming: Price skimming involves setting a high initial price for a new or innovative product and gradually lowering it over time. This strategy helps maximise profits from early adopters before targeting more price-sensitive customers.
How to choose a pricing strategy
Selecting the right Retail Pricing Strategy is necessary for the success of your business. Several factors come into play when determining the optimal pricing strategy. Here are key considerations to guide your decision-making process:
Understand Your Target Market:
Begin by thoroughly understanding your target market’s preferences, needs, and willingness to pay. Conduct market research to gain insights into their price sensitivity, purchasing behaviour, and the value they place on your products or services. Assessing price sensitivity, or how responsive consumers are to changes in price, can provide valuable insights into setting optimal prices. This knowledge empowers you to set prices that resonate with your customers and align with their perceived value.
Align with Business Goals:
Your pricing strategy should directly support your business goals. Whether you prioritise maximising profits, increasing market share, or enhancing customer loyalty, your pricing decisions should reflect these objectives. For instance, if customer acquisition is a primary goal, consider implementing a penetration pricing strategy to attract new customers.
Conduct a Cost Analysis:
Accurately calculate the costs associated with producing and delivering your products or services. This includes direct costs such as raw materials, labour, and shipping, as well as indirect costs like rent, utilities, and marketing expenses. A complete cost analysis ensures that your pricing covers all expenses and contributes to your business’s profitability.
Research Competitors’ Strategies:
Analyse the pricing strategies of your competitors to gain valuable insights into market trends and customer preferences. While it’s not advisable to base your pricing solely on competitors’ prices, understanding their approach can help you position your products or services effectively and identify opportunities for differentiation. Consider using the manufacturer-suggested retail price (MSRP) as a baseline for creating your pricing strategies and standardising prices across retailers.
Test Different Pricing Approaches:
Don’t hesitate to experiment with different pricing strategies to determine what works best for your business. Implement A/B testing or conduct market surveys to assess customer response to various price points. This data-driven approach allows you to make conscious decisions about your pricing strategy and optimise it for maximum success.
By considering these factors and adapting your pricing strategy accordingly, you can effectively position your business in the market, drive sales, and achieve your business goals. Remember, the right pricing strategy is one that strikes a balance between customer value, cost coverage, and business profitability.
Competitive pricing strategy examples
To gain a deeper understanding of these pricing strategies, let’s explore real-world examples of their implementation.
1. Cost-Plus Pricing:
Example: A boutique adds a 50% markup to its clothing items, which includes the cost of materials, labour, and overhead expenses.
2. Value-Based Pricing:
Example: An electronics store charges a premium price for a high-end smartphone due to its advanced features and brand recognition.
3. Competition-Based Pricing:
Example: A grocery store matches the prices of its competitors to remain competitive in the local market.
4. Penetration Pricing:
Example: A new coffee shop offers discounted prices for a limited time to attract customers and establish a loyal customer base.
5. Psychological Pricing:
Example: A clothing store prices its items at Retail Pricing Strategies just below a whole number (e.g., $9.99 instead of $10.00) to create the perception of a better deal. Additionally, displaying a discounted price alongside the original price can enhance this perception by highlighting the savings.
6. Dynamic Pricing:
Example: An airline adjusts ticket prices based on demand, time of booking, and competition to maximise revenue.
7. Premium Pricing:
Example: A luxury car brand sets high prices for its vehicles to reflect their superior quality and exclusivity, attracting affluent customers.
These examples demonstrate how businesses across various industries apply different Retail Pricing Strategies to achieve their specific goals. By carefully considering customer perceptions, market dynamics, and business objectives, retailers can leverage these strategies to optimise their pricing approach and drive business success.
Finding the best pricing strategy for you
So, you’ve familiarised yourself with the various retail and wholesale pricing strategies available. Now, it’s time to embark on a journey to discover the pricing strategy that resonates most effectively with your retail business. This involves a holistic approach that encompasses several crucial considerations.
First and foremost, it’s essential to gain a strong understanding of your target market and their willingness to pay. Conduct thorough market research to decipher their preferences, purchasing patterns, and the value they associate with your products or services. This knowledge acts as a fundamental principle for crafting a pricing strategy that aligns seamlessly with their expectations.
Next, take a deeper look into your business’s cost structure, meticulously evaluating all expenses incurred during production, distribution, and marketing. This analysis will provide a clear picture of your break-even point, ensuring that your pricing strategy safeguards profitability while remaining competitive.
It’s all-important to conduct a meticulous competitor analysis, examining the competitive pricing strategies employed by your industry peers. Identify their strengths and weaknesses, and leverage this knowledge to position your pricing effectively within the competitive landscape. Consider using price anchoring, where a higher-priced item is placed next to a lower-priced item to make the latter appear more attractive, to influence customer perception and drive sales.
Lastly, don’t shy away from experimentation. Test different pricing strategies on a small scale before committing to a long-term approach. This hands-on approach will provide valuable insights into customer response, allowing you to refine your pricing strategy until you discover the perfect formula for success.
Remember, the retail landscape is ever-changing, and customer preferences are constantly evolving. So it’s imperative to regularly review and adjust your pricing strategy based on market conditions and customer feedback. This proactive approach will ensure that your own discount pricing strategy always remains relevant, competitive, and aligned with your business goals, ultimately leading to sustained success in the ever-evolving retail industry.
Finding the best Retail Pricing Strategy for your business is a process of exploration, analysis, and adaptation. By meticulously considering your target market, costs, competition, and customer feedback, you can craft a dynamic pricing strategy that propels your retail business to new heights of profitability and customer satisfaction.
Pricing strategy FAQ
As you navigate the dynamic landscape of retail pricing, it’s natural to encounter questions that may influence your approach. Let’s address some frequently asked questions to clarify key aspects of pricing strategies:
How do I assess the effectiveness of my current pricing strategy?
Evaluating your current pricing strategy is necessary for identifying areas of improvement. Here are a few metrics to consider:
Sales Volume: Monitor changes in sales volume over time. A consistent increase or decrease can indicate the effectiveness or ineffectiveness of your pricing.
Customer Feedback: Gather input from customers through surveys, reviews, or direct conversations. Understand their perceptions of your pricing compared to competitors and the value they associate with your products.
Profit Margins: Analyse your profit margins to determine the profitability of your pricing strategy. Ensure that your prices cover production costs and allow for sustainable growth.
How can I implement pricing changes without losing customers?
Implementing pricing changes requires a delicate balance to minimise customer churn. Here are some strategies to consider:
Communicate Clearly: Openly communicate the reasons behind the price change to your customers. Explain the value they will continue to receive or any improvements being made.
Offer Incentives: Provide incentives such as discounts, loyalty programs, or added value to offset the price increase and retain customer loyalty.
Implement Gradually: Consider implementing price changes gradually over a period of time, allowing customers to adjust and understand the new pricing structure.
What are some common pricing pitfalls to avoid?
Avoid these common pricing pitfalls to maintain a competitive retail price edge:
Price Wars: Engaging in aggressive price wars can lead to a race to the bottom, eroding profits and diminishing brand value.
Ignoring Customer Value: Focusing solely on cost-based pricing without considering customer-perceived value can lead to missed opportunities for higher profits.
Ignoring Competition: Setting prices without considering competitor pricing can result in being overpriced or underpriced, impacting sales and market share.
How can I test different pricing approaches?
Testing different premium pricing approaches allows you to gauge customer response and optimise your strategy. Here are a few methods to consider:
A/B Testing: Conduct A/B tests by offering different prices to different customer segments or on different platforms. Analyse the results to determine the most effective pricing approach.
Surveys and Feedback: Collect customer feedback through surveys or focus groups to understand their willingness to pay and preferences for different pricing options.
Experimental Pricing: Implement temporary price changes for a limited period to gather data and insights into customer behaviour and demand.
What is price discrimination and how can it be used effectively?
Price discrimination involves charging different prices to different customer segments based on their willingness to pay. This strategy can maximise revenue by capturing consumer surplus, but it must be implemented carefully to avoid customer dissatisfaction.
By addressing these frequently asked questions and implementing the recommended strategies, you can fine-tune your retail pricing strategies for success in 2024. Embrace the power of effective pricing to drive sales, enhance customer loyalty, and optimise your business performance.
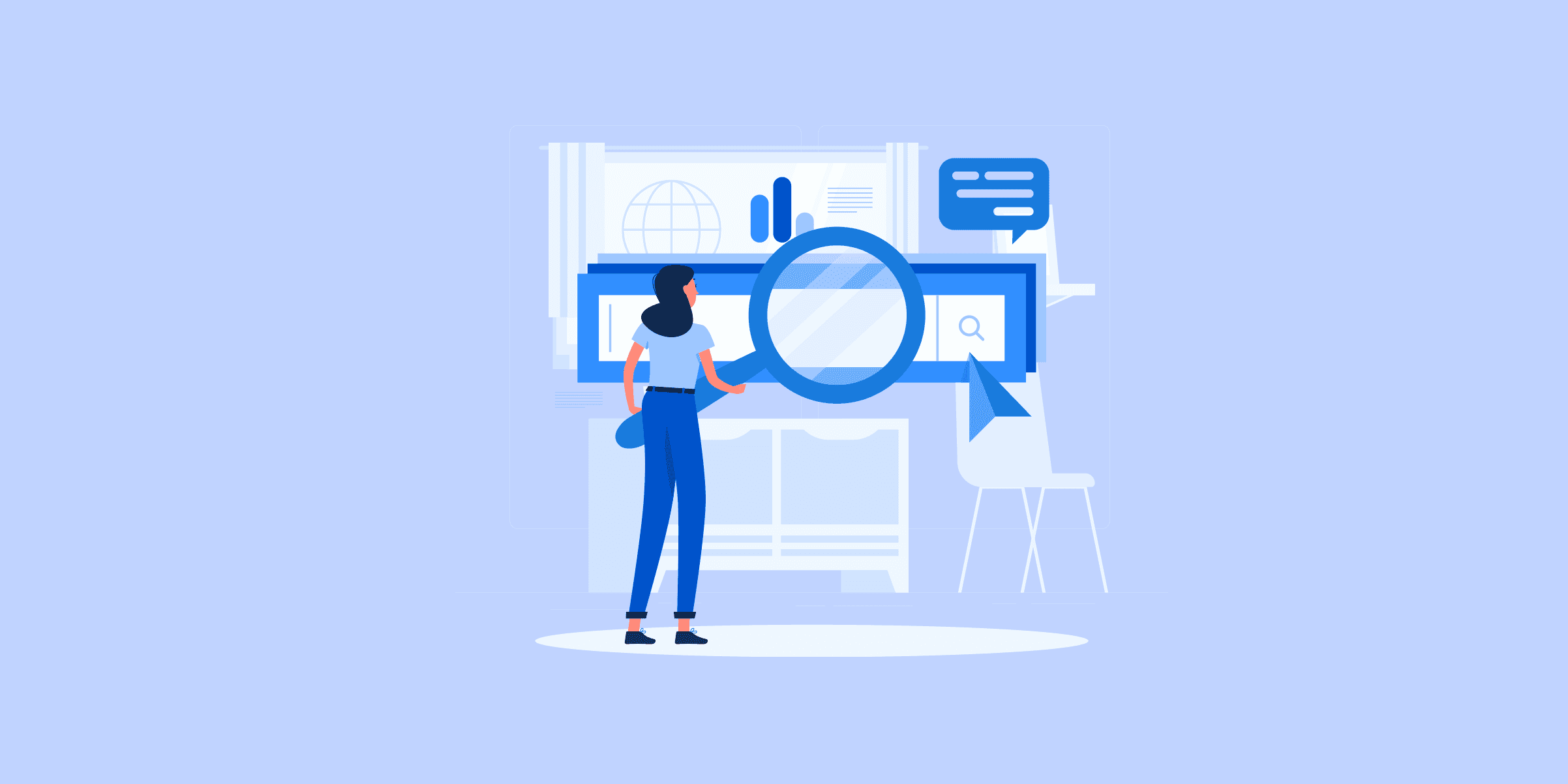
How to Perform a SWOT Analysis for Your Small Business
SWOT (not to be confused with SWAT) can be a game changer for any team, business, or organisation that wants to be successful. Oh, you say you want that too? Well then, you just may need a SWOT analysis.
A SWOT analysis for a small or medium-sized business (SMB) is a powerful but simple process. It gives businesses a clear view of their current position and helps them understand how to be more successful. We’ll cover what it is, the benefits, and how you can get started with your small business, today.
Here’s your guide to SWOT:
What is a SWOT analysis?
Why is a SWOT analysis important?
Four key elements of SWOT analysis for small business
How to conduct a SWOT analysis for small business
SWOT analysis example: a small business case study
What’s next for small businesses?
What is a SWOT analysis?
The term ‘SWOT’ stands for Strengths, Weaknesses, Opportunities, and Threats. By identifying how they stack up within these four categories, businesses can discover their strengths and potential weaknesses, and identify their long-term competitive differentiation and potential threads. SWOT analysis is a strategic planning and management technique that’s sometimes called situational assessment or situational analysis.
Why is a SWOT analysis important?
A SWOT analysis includes both internal and external factors. Internal factors (strengths and weaknesses) are those that businesses can control or change. External factors (opportunities and threats in the wider economy) are those that lie outside of a business’s control.
These four key factors provide the foundations that businesses can use to plan for the future. They can do this by using their internal strengths to counter external threats. (Back to top.)
Four key elements of SWOT for small business
A small business will look at the following four categories when conducting a SWOT analysis:
1. Strengths (internal)
A business’s strengths are a sign of its main advantages in the marketplace. Strengths can include a one-of-a-kind product, or excellent service and aftercare. Ideally, strengths are unique, are not easily replicated by the competition, and help maintain customer loyalty.
For example, your company may have a unique, patented product, or a highly loyal customer base. These things would be difficult for your competition to replicate.
2. Weaknesses (internal)
These are the elements of a business that aren’t operating as efficiently as they could and might hold you back from competing effectively. Your business might lack experience in design, or you might be using outdated systems that don’t talk to each other. A business’s weaknesses are a sign of what it needs to do better to operate at peak efficiency.
For example, a company might be failing to generate repeat purchases due to poor after-sales communication and a sub-optimal customer journey. You could improve this by increasing staff training, or by automating certain processes.
3. Opportunities (external)
Opportunities are areas where your business may gain a competitive advantage. They can present themselves at any time, and even sometimes out of the blue. Small businesses can ensure they are ready to take advantage of them whenever they arise. Having identified your strengths and weaknesses through SWOT analysis, you can understand how you are positioned to capitalise on potential opportunities – and where you might need to improve in order to take advantage of them.
An opportunity can be anything from a competitor’s misstep to changed legislation, to weather that impacts your competition’s supply change.
4. Threats (external)
We live in an unpredictable world, and threats can come at any time. From changing regulations, rising materials costs, and shifts in customer priorities. Threats are external factors, as they are things that businesses can’t influence. But you can try to future-proof yourself in key areas and mitigate your weaknesses.
Automating processes can make you more efficient, so you can focus more on keeping customers happy. And, making contingency plans using digital solutions can help operations to run smoothly in times of crisis. (Back to top.)
How to conduct a SWOT analysis for small business
A SWOT analysis is a highly flexible tool that can be tailored to fit the needs of any business that’s using it. Here are some key points to consider when conducting a SWOT analysis.
Put together a broad team
For a SWOT analysis to be effective, it needs to gather a range of viewpoints from around the business. Talking only to customer services or business analytics teams will give a skewed perspective. Ensure that each major department is represented, from those handling day-to-day operations on the ground to those planning for the future.
Listen to ideas
The team you assemble will be unique, with a particular mix of perspectives and skills. A good first step is to encourage everyone to share their initial thoughts, perspectives, and ideas. Do this either in person, as a group, or virtual, the most important thing is to allow people to share their views in an open and non-judgmental setting.
Create your timeline
When everyone has shared their ideas, it’s time to make some decisions. Those leading the SWOT analysis will want to identify key focus areas, choose a methodology, and decide on a timeline.
Present the findings: A simple grid, with one quadrant for Strengths, Weaknesses, Opportunities, and Threats, is a great way to represent the findings of a SWOT analysis visually. In this form, the insights can be easily shared across the business. (Back to top.)
SWOT analysis example: SMB case study
Here’s a SWOT analysis example that small businesses can use to identify their strengths, weaknesses, opportunities, and threats. We will use Clara’s Cake Kitchen, a fictitious bakery.
Strengths
Location: Suburban location near a train station that draws in foot traffic during rush hour.
Product: The owner produces high-quality artisanal cakes that customers come back for, again and again.
Marketing: The owner successfully uses social media channels to generate buzz about the business and has a large following.
Weaknesses
Unpredictable ROI: Although the bakery is very busy at several points during the day, and on weekends, there are lots of quiet times during the day, and during the summer.
Online reach: The owner has not invested in click-and-collect or online services, as she doesn’t know if it will be worth it in the long run.
Equipment: Some of the kitchen equipment is second-hand, and is prone to break, requiring expensive repairs and causing order delays.
Opportunities
Loans and funding: Organisations like the Small Business Administration (SBA) offer loans and funding programs that could help Clara’s Cake Kitchen expand, upgrade equipment, and capitalise on new opportunities — potentially creating jobs in the process.
External events: There are some new food festivals and markets starting up in the nearby city. Having a presence at these events could help to expand the brand’s reach.
Threats
Cost of materials: The cost of raw materials that the owner uses to bake the cakes may likely increase. It’s becoming harder to find key ingredients without a long lead time.
Competition: More local bakeries are offering custom cakes from home kitchens, with lower overhead costs and fewer equipment issues.
Summary
Clara’s Cake Kitchen will need to use its strengths to counter its weaknesses while taking advantage of opportunities and preparing to tackle the threats. Adding online ordering and easy pickup for commuters might be an avenue for the bakery to guard against new competitors and higher costs. Her team could host an event for their customers, offering discounts or freebies. And, together, they can build and grow with a low-cost collaboration tool, like Starter Suite. (Back to top.)
What’s next for small businesses?
We’re in a challenging moment where competition is fierce, the landscape is in flux, and seizing new opportunities is crucial. Conducting a SWOT analysis, and enlisting the right tools for the job, can help today’s small businesses address weaknesses, double down on what they do well, and position themselves to succeed in any economy.
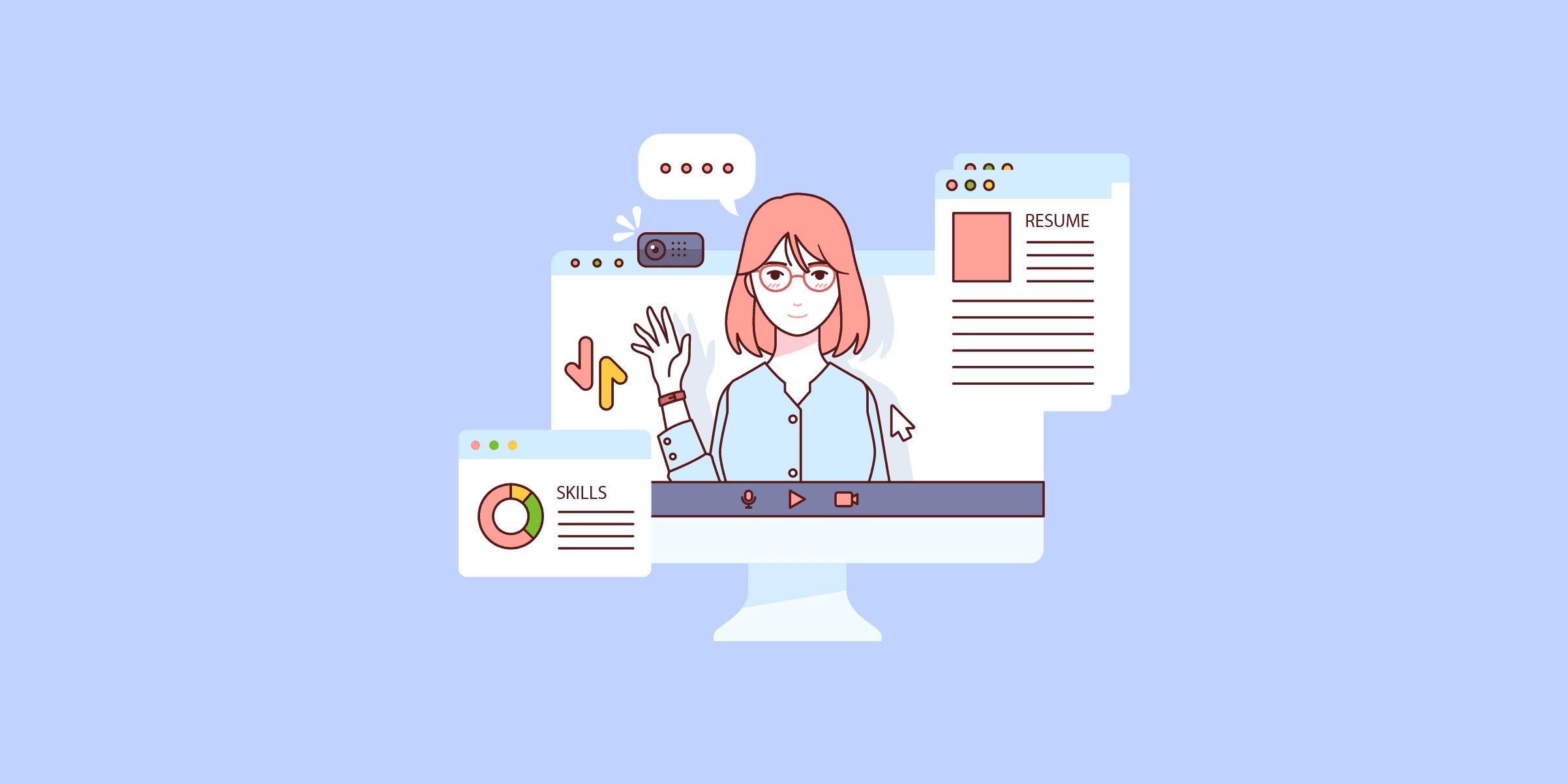
Decoding the Marketing Mix: Mastering the 4 P’s for Business Success
Wondering how to steer your business towards success? The marketing mix might just be your answer. It’s a proven blend of four essential elements—Product, Price, Place, and Promotion—that, when combined effectively, can elevate your marketing strategy and deliver results. This concept, a basis of marketing strategy, equips businesses to align their offerings with customer demands and stand out in a competitive landscape. Throughout this article, we will explore each ‘P’ in detail, showing you how to harness the marketing mix for business success.
Key Takeaways
The marketing mix, composed of the four Ps (Product, Price, Place, Promotion), provides a framework for businesses to create a successful marketing strategy that satisfies customers’ needs, effectively communicates value, and stands out in a competitive market.
A comprehensive marketing strategy requires understanding and fulfilling customer needs, differentiating the product, and optimising pricing strategies to reflect the perceived value and maintain competitiveness.
Expanding the traditional marketing mix to include People, Processes, and Physical Evidence enables companies to create a more holistic and customer-centric strategy, ensuring efficiency in service delivery and a memorable brand experience through physical aspects of interaction.
Demystifying the Marketing Mix: A Comprehensive Guide
The ‘marketing mix’ is essentially the bedrock upon which modern marketing strategies are constructed. It’s a term that was coined by E. Jerome McCarthy in 1960, a professor who reshaped traditional approaches to marketing with his innovative concept. Today, understanding the marketing mix is crucial for developing an effective marketing strategy, as it enables companies to provide customers exactly what they want—offering their products or services at the right place and price point, and effectively promoting them.
McCarthy laid out this foundational formula for success through what became known as the four Ps of Marketing:
Product
Price
Place
Promotion
Each component plays its own distinctive role within an all-encompassing whole—much like individual instruments contribute to an orchestral performance—to create harmonious results capable of commanding market success when performed skillfully.
The Essence of the Marketing Mix
The marketing mix provides a strategic framework that assists companies in navigating market complexities. It encompasses four key elements, often referred to as the “four Ps,” which act as navigational beacons for new entrepreneurs and established executives.
Product: the offering designed to fulfil customer needs
Price: the cost at which value is exchanged
Place: optimal locations where products are accessible to customers
Promotion: communicative efforts that connect services with consumers.
This method of management transcends a basic enumeration. It represents an evolving synergy of strategic decisions. By integrating these essential components into their strategies, businesses can successfully tailor their marketing efforts to engage effectively with their target audience and distinguish themselves in a competitive marketplace.
Key Elements of a Robust Marketing Strategy
Crafting an effective marketing strategy demands mastery in merging the four essential pillars of marketing to amplify their cumulative effect. Each element must function synergistically with its counterparts, creating a fine-tuned balance that drives increased sales and advances the company toward achieving its goals. The critical components known as the four Ps include:
Product: Ensuring that what is offered meets consumer needs.
Price: Setting it at a level consumers are prepared to pay.
Place: Carefully selecting distribution locations for optimum access.
Promotion: Communicating persuasive messages that captivate and connect with audiences.
When these factors harmonise, they form the foundation of impactful marketing initiatives, from basic strategies through to complex campaigns. A product’s features aligned with well-considered pricing structures, strategic distribution channel choices, and cohesive promotional activities together orchestrate success—building brand loyalty, increasing market visibility and securing a dominant spot within today’s competitive marketplace for businesses seeking distinction.
Crafting Your Offering: Product Strategies
Embarking on a successful marketing strategy hinges upon the product—a concrete exemplification of what a business brings to its clientele. Such products may encompass various forms from:
Physical goods
Services lacking physical form
Experiences provided
Digital offerings
The true test involves not merely crafting an item, but grasping its attributes, promotional narratives, and, most importantly, how it addresses customer needs.
To resonate with its target audience aptly, a product must navigate the evolving landscape of consumer behaviour and trends. This necessitates deep insights into what customers seek, extensive experimentation, and continuous enhancement of the value proposition offered by the product. Companies that agilely adjust to changing tastes in consumer preferences are typically those that attain market success with their products.
Understanding Customer Needs
Marketing strategy is fundamentally anchored in the profound grasp of what customers seek. Decoding their needs—akin to deciphering an esoteric language—not only paves the way for tailored products and marketing initiatives but also enables personalised customer experiences. By delving beyond apparent desires into core motivations, companies can craft offerings that connect profoundly with their target market.
Deep knowledge of both product intricacies and consumer preferences must precede a product’s market introduction. An insightful exploration into potential customers’ mindsets guides every aspect of marketing—from crafting content to orchestrating sales promotions—ensuring each communication resonates accurately and that every service or item perfectly aligns with customer expectations within the specified target audience.
Product Differentiation and Positioning
Within the commerce industry, it is just as vital to stand out from the competition as it is to resonate with customers. Carving a distinct place in the minds of consumers through product differentiation and strategic positioning sets a brand apart from its rivals. By integrating distinctive features and designing appealing packaging, companies can draw in and maintain clientele, setting a foundation for enduring customer loyalty.
Take, for instance, brands like Dollar Tailoring their offerings by focusing on lower-income groups and budget-conscious buyers—through competitive pricing strategies and ongoing promotional deals—how they have effectively captured their desired market segment. Having an acute awareness of cultural distinctions and local customs is necessary for businesses striving to create international appeal. This ensures that products are not only visible but also embraced across various cultures.
Pricing Mastery: Developing Your Product Pricing Strategy
Pricing extends beyond simply attaching a number to a product. It communicates the perceived value, quality, and position of the brand. To craft an effective pricing strategy, companies must possess comprehensive insights into their production expenses, competitors’ price points, and most importantly, how consumers perceive value and quality. This requires careful consideration, as organisations need to measure their own costs against what customers are prepared to pay while ensuring that their chosen pricing models complement the overarching marketing strategy.
Executing a robust pricing strategy is needed for driving revenue growth and sustaining profitability. The process involves:
Comprehending the fundamental cost associated with creating goods along with determining suitable markups that sustain financial objectives.
Assessing consumer evaluations regarding both quality and worth.
Confirming prices mirror how much consumers believe the product deserves.
Psychological Pricing Tactics
Understanding the mind is like becoming an expert in psychological pricing. Adopting strategies that set product prices at, say, $9.99 as opposed to a round $10 exploits consumer perception, fostering an impression of greater value and cost savings. This clever yet impactful approach significantly enhances purchase probabilities by appealing to consumers’ innate appetite for deals, thereby augmenting the effectiveness of sales promotions.
For marketers, it’s imperative to delve into the psychological foundations that underlie pricing techniques. The essence lies not merely within digits adorning tags, but in how these figures are perceived and the emotions they incite. Within the promotional mix landscape, price wields considerable influence over consumer choices and satisfaction levels post-purchase. Hence it serves as an influential tool for shaping purchasing behaviours.
Competitor Price Analysis
In sectors where there is a high degree of similarity in products and services offered, the price often becomes the critical element that sways customers toward one brand over another. Conducting competitor price analysis enables companies to fine-tune their pricing strategies with careful consideration of what competitors are charging. This insight empowers them to competitively place themselves within the market by either matching value or setting themselves apart through unique selling points.
The necessity for strategic placement amplifies in environments dense with competition, as carving out a distinct space can prove difficult. With insights gained from examining the prices set by their industry counterparts, businesses have the opportunity to:
Revisit and refine their own pricing models
Enhance profit margins
Ensure that their product’s cost accurately mirrors both how they want the brand perceived and meets customer expectations.
Placement Decisions: Optimising Distribution Channels
The component of ‘Place’ within the marketing mix emphasises ensuring product availability when and where customers desire it. This entails identifying optimal selling points, discerning the preferred shopping venues of the target audience, and adeptly handling stock levels and delivery logistics to streamline and enhance the customer’s purchasing experience.
Determining the appropriate retail platforms and determining whether to engage in B2B or B2C commerce are vital determinants affecting a product’s market performance. Ensuring that products are readily available at places frequented by potential buyers is essential—this strategic placement has a direct impact on satisfying consumer needs and providing accessible services.
Digital Presence and E-commerce
Maintaining a solid online presence and the ability to engage in e-commerce, now more than ever, are essential. As 93% of business-to-business purchasers show a preference for using online avenues when making buying choices, possessing an active digital footprint is now pivotal within effective distribution methodologies. This approach not only extends market access but also enhances the efficiency of transactions and offers instantaneous insights that empower companies to make adjustments to their marketing undertakings.
By integrating diverse tools associated with digital marketing into systems such as Marketing Hub, particularly those focused on search, often referred to as “search engine marketing,” enterprises can significantly enhance their operational prowess. The array of instruments at one’s disposal includes:
Content creation through blogging
Search Engine Optimisation (SEO)
Managing social media platforms
Strategic email campaigns
Monitoring advertisement performance
Leveraging these resources allows organisations not just broader exposure but also provides them with opportunities for more profound engagement with audiences. Fostering conversions into sales while strengthening bonds with consumers, thereby maximising their overall outreach impact in the framework of modern-day commerce.
Delivery Logistics and Physical Location
Just as needed as digital approaches are the concrete aspects of positioning, which include the physical placement and the management of delivery logistics. Choosing a strategic physical location can significantly boost product sales and elevate the overall customer experience. The design and visual appeal of a place, be it for retail or providing services, is essential in both drawing customers in and keeping them coming back.
How products are transported to customers—via shipping methods, transit systems, or options like picking up in-store—is essential to shaping their purchasing journey. Swift and competent handling of delivery logistics ensure that items reach clients quickly and undamaged, greatly affecting their impression of your brand along with their inclination to become repeat buyers.
Amplifying Visibility: Crafting a Promotion Strategy
Promotion is essentially the platform businesses use to introduce their offerings to the world. A well-crafted promotion strategy employs a variety of tactics, including:
Advertising
Public relations
Social media marketing
Content marketing
These tactics work together to create compelling marketing messages that showcase the importance of marketing skills. These messages must resonate with the target audience and reinforce brand awareness, ultimately leading to increased lead generation and sales.
Identifying the perfect timing and utilising the most efficient marketing channels for compelling advertising is key to engaging the targeted audience. An effective marketing strategy is not just about broadcasting messages; it’s about engaging in a dialogue with potential customers, understanding their needs, and providing them with reasons to choose your brand over others.
Integrated Marketing Communications
Integrated Marketing Communications (IMC) functions like a conductor leading an orchestra, ensuring all communication methods convey a unified brand message. IMC transcends the alignment of advertising strategies. It’s about crafting a cohesive experience for consumers across various platforms, such as:
Email marketing
Print media
Social networking sites
Television commercials
Public relations initiatives
Direct mailing campaigns
This integration bolsters customer satisfaction and fosters loyalty through harmonised messages.
Synchronising promotional activities not only extends reach but can also trim costs and amplify returns on investment. Digital marketing shines in this ensemble by providing targeted outreach and detailed analytics regarding campaign effectiveness. This empowers businesses to refine their engagements with clientele, elevating direct marketing efforts and other aspects of their overall strategy.
Leveraging Social Media Marketing
Social media has become an integral element of a brand’s marketing strategy. This medium allows brands to build a community by directly engaging with their customer base. Social media marketing stands as a vital pillar within the broader scope of digital for its real-time interaction capabilities. It provides platforms for customers to provide immediate feedback and allows brands to adjust their services or products according to customer needs.
Social media affords marketers critical insights gathered from data analysis that can greatly enhance how they engage with customers and refine overall marketing efforts. Marketers are empowered through these insights to craft campaigns tailored specifically toward their audience, which helps drive deeper engagement and cultivate enduring loyalty towards the brand.
Extending Beyond Basics: The Extended Marketing Mix or 7 P’s of the Modern Marketing Mix
The traditional four Ps of marketing is enriched by adding three essential elements to form an extended mix.
People: concentrating on the business’s human factor
Process: emphasising efficient service provision
Physical Evidence: acknowledging the concrete items that customers come into contact with
These components broaden the scope of the traditional marketing mix and are vital in forging a holistic, consumer-focused marketing strategy that connects more profoundly with customers.
When businesses incorporate people, processes, and physical evidence into their marketing approach, they don’t just satisfy customer expectations—they surpass them. These additional facets allow companies to set themselves apart from competitors, enhance customer delight, and cultivate a robust and enduring brand identity.
People at the Heart of Your Business
People form the lifeblood of any organisation, influencing the customer experience and fostering loyalty. A customer-centric organisational culture enhances product and service delivery and attracts and retains top talent. When employees are motivated and aligned with the company’s values, they’re more likely to go above and beyond in their roles, directly contributing to customer satisfaction.
Businesses prioritising their people and cultivating a supportive company culture find that it pays dividends. Happy employees lead to happy customers, and when customers feel valued and understood, they’re more likely to become loyal brand advocates. This human-focused approach is crucial to any successful marketing strategy, as it ensures that every interaction reflects the company’s dedication to excellence.
Process Optimisation for Customer Satisfaction
The procedure functions as a guiding framework for providing products and services, with its fine-tuning being crucial for achieving consumer satisfaction. Successful methodologies provide ease, swift delivery, and outstanding service—each element shaping how customers view a brand. Companies can deliver individualised and impactful services by centring employees on key client-oriented processes.
Marketing Hub exemplifies the simplification technology brings to marketing automation. It enables marketers to handle data and instruments more effectively while elevating customer satisfaction. Procedures ought to be customised according to product types and anticipated by the target audience to align in relevancy and productivity.
The Role of Physical Evidence in Marketing
In marketing, the concept of physical evidence goes beyond the product itself and includes all visible elements that a customer might encounter when engaging with a brand. This encompasses aspects such as branding, packaging, and even how a company’s physical location is designed—all crucial factors that can sway consumer perception and enhance the impact of an organisation’s marketing strategy.
These concrete components act like mute promoters for the brand, transmitting messages about its values and quality without saying anything. The atmosphere provided by retail space, aesthetic choices in product packaging design, and consistent staff uniforms play key roles in forging memorable customer experiences. When businesses pay attention to these details and intentionally shape them, they can forge an attractive brand identity that connects deeply with their target audience and gives them an edge over the competition in today’s marketplaces.
Summary
The journey to business success is multifaceted. Mastering the 4 Ps—Product, Price, Place, Promotion—and incorporating People, Process, and Physical Evidence into the mix can create a powerful marketing strategy that resonates with consumers and drives business growth. Each element plays a crucial role, and when harmonised, they form a symphony of strategic decisions that captivate the target audience and cement a brand’s market presence.
Let this be the catalyst for innovation and inspiration in your marketing endeavours. With the insights and strategies discussed, you’re now equipped to craft marketing campaigns that meet customer expectations and exceed them, fostering loyalty and carving out a distinctive place for your brand in the marketplace.
Frequently Asked Questions
What exactly is the marketing mix?
The 4 Ps—Product, Price, Place, and Promotion—constitute the core framework of the marketing mix. This critical model steers businesses in formulating successful marketing tactics to satisfy customer needs and accomplish business objectives.
How do psychological pricing tactics influence consumer behaviour?
Employing psychological pricing strategies, like placing price points at $9.99 rather than an even $10, crafts the illusion of a better deal, which persuades customers to believe they are receiving greater value for their expenditure.
Such methods can have a considerable effect on consumer purchasing choices.
Why is an integrated marketing communications strategy important?
A strategy for integrated marketing communications is crucial as it guarantees uniformity in the brand’s messaging across every marketing channel. This coherence results in a fluid customer experience that boosts overall contentment and fosters loyalty.
Can social media marketing improve customer engagement?
Marketing through social online platforms enhances the capacity for immediate communication and collection of instantaneous responses from clients, thereby providing an opportunity to elevate customer engagement substantially.
What role do people play in the extended marketing mix?
People play a central role in the extended marketing mix. They create the customer experience and contribute to loyalty and business success.
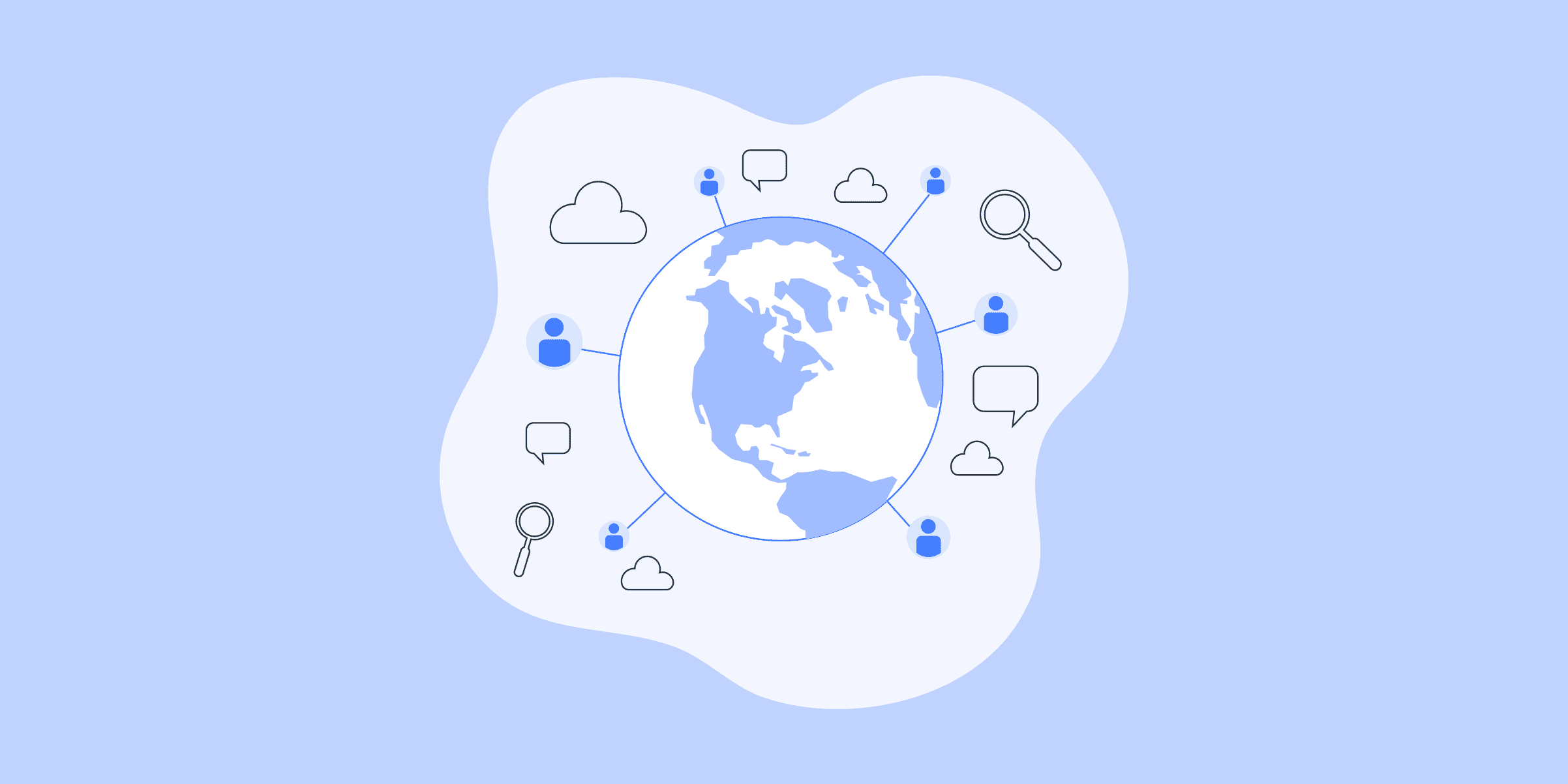
Data Science: A Complete Guide 2024
This guide encompasses the critical techniques of data science, which employs programming and statistical methods to glean insights from information, a process essential for better decision-making in business.
Key Takeaways
Data science involves using a variety of techniques such as classification, regression, and clustering to analyse and derive insights from raw data, playing a critical component in business decision-making and strategy.
While related, data science and business analytics have distinct focuses: data science utilises interdisciplinary methods and machine learning for predictive modelling, whereas business analytics examines historical data to optimise business operations.
Ethical considerations in data science, such as privacy, bias, and the societal impact of data use, are crucial, highlighting the importance of transparency and fairness in data collection and analysis.
What is Data Science?
The field of data science emerges at the intersection of programming, mathematics, and statistics. This trio forms the critical framework underlying contemporary analytical methods. Data science’s strength resides in its capacity to meticulously parse through an immense expanse of quantitative data to unearth patterns and connections that are pivotal for making informed business choices. Given the torrential outpouring of information stemming from every digital interaction—each click, swipe, or engagement—it is a discipline with an unquenchable thirst for data.
The significance of data understanding has become even more pronounced in our modern era drenched with data. Every phase of the journey — from gathering raw figures to distilling insights and distributing them — relies on a sophisticated synergy between technologies and techniques aimed at deciphering big data’s complexity. The necessity for insight into extensive datasets propels this once specialised skill into a fundamental pillar as organisations across sectors generate unprecedented amounts of publicising profound consequences. Both industries and societies alike must adapt rapidly within transforming environments where crucially maintaining competitive advantage lies.
Understanding Data Science
At the heart of data science lies the dual-natured discipline focused on deriving valuable information from unprocessed data. It harnesses a diverse array of techniques within data science, ranging from simple to highly sophisticated methods, to transform large and often disorganised datasets into clear and useful insights. What sets data here apart from related fields is its comprehensive set of tools that span simplistic approaches like crafting data visualisations to implementing complex machine learning algorithms—all to parse through data to discover invaluable points.
The Evolution of Data Science
The lineage of data science can be traced back to the nascent days of computer science and statistics when these two fields began their dance in the 1960s and 70s. It was a time when the term ‘data science’ was first whispered as an alternative to statistics, hinting at a broader scope that would come to include techniques and technologies beyond traditional statistical methods. As databases and data warehousing became prevalent, the ability to store and work with structured data grew, laying the groundwork for the data science we know today.
This evolution witnessed the formal recognition of the profession, with Hal Varian defining what it means to be a data scientist. The field has grown from simple statistical analysis to encompass predictive models and machine learning, marking a transformation that has redefined the possibilities of data-driven decision-making. As society moves forward, the history of data science continues to be written, with each chapter unveiling new technologies and methodologies that push the boundaries of what can be achieved with data.
Key Data Science Techniques
Data science encompasses various statistical, computational, and machine-learning methodologies aimed at understanding and forecasting data. The toolkit for data science projects consists of various specialised techniques, such as classification, regression, and clustering, to address unique challenges presented by different data sets. By implementing these methods in real-world scenarios, practitioners can derive significant knowledge.
Complex quantitative algorithms underpin these methods in data science projects, enabling data scientists to unravel intricacies within massive datasets. Thus, obscure patterns are made evident, and intricate information is translated into comprehendible forms through these sophisticated behind-the-scenes mechanisms in machine learning-driven analysis.
Classification
Classification is the cornerstone of numerous applications in machine learning, such as identifying spam or providing medical diagnoses. It involves employing decision-making algorithms to organise data within specific predefined groups and is a vital part of science and machine learning.
A variety of methods are deployed for classification purposes.
Decision trees utilise branching logic to split the data.
Support vector machines establish divisions between categories by creating boundaries with maximum margins.
Neural networks apply deep learning techniques to process complex and extensive datasets.
The brilliance of classification stems from its capacity to be educated using existing datasets and then extend this accumulated knowledge towards analysing new, unfamiliar data. Whether it’s engaging Naive Bayes classifiers that leverage probability theory or employing logistic regression for fitting information along a prognostic curve, classification involves making educated predictions about which group an incoming datum should fall under.
Regression
Regression methods serve as data scientists’ predictive oracle, forecasting numerical results by analysing variable interconnections. This kind of data analysis involves delving into historical trends to predict future events. The simplest form is linear regression, which aims to discover the optimal straight line that fits the dataset. In contrast, lasso regression improves prediction accuracy by focusing on a select group of influential elements.
When working with datasets rich in variables, multivariate regression broadens these insights across several dimensions, helping data scientists decipher intricate patterns of interconnectedness among factors. For business analysts, regression acts as their navigational aid through vast oceans of information, steering them towards predictions that shape strategies and guide key decisions.
Clustering
Clustering involves identifying inherent groupings within data by analysing patterns and outliers, thus gathering similar data points. Methods such as k-means clustering utilise central points around which data is grouped, whereas hierarchical clustering forms a dendrogram that links the data based on resemblance.
Sophisticated techniques like Gaussian mixture models and mean-shift clustering provide subtle approaches for delineating between concentrated and dispersed areas in a dataset. Excelling when there are no preset categories, this technique equips data scientists with the capability to decipher unstructured data—unearthing revelations that have the potential to spur significant breakthroughs.
Data Science Tools
The arsenal of tools available to data scientists is as diverse as the challenges they confront, encompassing everything from high-capacity big data processing systems to sophisticated data visualisation platforms and advanced machine learning technologies. These instruments serve as the bedrock for the entire data science process, allowing experts in the field to sift through, make sense of, and illustrate massive datasets in manners previously deemed unattainable. Technologies such as Apache Spark, Hadoop, and NoSQL databases equip these professionals with the ability to rapidly manage information on a large scale—matching strides with our continuously expanding digital footprint.
On the one hand, tools like Tableau, D3.js, and Grafana convert undigested numbers into impactful narratives told through visuals that clarify abstract concepts or simplify intricate details. On another front, machine learning frameworks include TensorFlow and PyTorch. These lay down essential frameworks for devising complex algorithms capable of evolving by discerning patterns within data over time. An appropriate tool does not simply facilitate a task—it allows those immersed in different spheres of data to achieve groundbreaking advancements within their domain using an array of specially tailored mechanisms, undoubtedly transforms it into something more meaningful – enabling specialists to transcend previously often established boundaries enabled by utilising distinct data science methodologies.
Data Science in Business
Data science for business serves as a critical competitive differentiation within the commercial landscape. Companies can unlock the immense value of their data by using it to unearth transformative patterns that were previously unknown, fostering product innovation and enhancing operational efficiency, which ultimately steers them toward expansion and triumph.
Utilising tools such as predictive analytics, machine learning algorithms, and deep customer insights fall under data-driving techniques that propel businesses into an era where decisions are informed by robust data analysis. This approach is reshaping traditional business methodologies with improved precision in efficiency and pioneering innovations.
Discovering Transformative Patterns
Data science operates like an expert detective, sifting through data to reveal insights and patterns that can revolutionise a company. These discoveries can reinvent how products are strategised, optimise operational efficiencies, and unlock new avenues in the marketplace. Employing data mining methodologies within vast datasets allows businesses to identify both emerging trends and irregularities—thus equipping them with the foresight to evade potential pitfalls while seizing advantageous prospects.
The strength of data science stems from its capability to:
Illuminate successful elements of a business as well as areas needing improvement.
Direct companies toward refining their methods and embracing more effective strategies.
Integrate techniques from various fields into deciphering the narrative told by data, rooted in the basic principle behind it, which is synonymous with the core principle behind data science itself.
Propel an organisation towards growth beyond expectations.
Engaging in this analytical journey holds profound implications for enhancing a business’s prosperity.
Innovating Products and Solutions
Data science is critical to the lifeblood of business innovation, acting as a catalyst that uncovers voids and chances for groundbreaking products and solutions. Through rigorous examination of consumer insights and industry tendencies, data scientists can steer product evolution to align more closely with user desires and tastes while simultaneously pinpointing enhancements in current workflows.
The perpetual loop of inventive progression guarantees companies stay agile and attuned to their customer base’s demands. Data science does not merely accelerate product creation. It cultivates a setting where imagination is underpinned by solid factual analysis, resulting in novel yet impactful offerings.
Real-Time Optimisation
In the current rapid market environment, business agility is crucial, and data science serves as the driving force behind immediate optimisation. Data Science allows companies to foresee shifts and alter their tactics on the fly, keeping them flexible and ahead of the curve. The use of real-time data encompasses a range of applications, from enhancing marketing campaigns to streamlining inventory management, which ensures that a business maintains its competitive edge.
As an essential feature of data science, predictive analytics empowers businesses by allowing them to:
Predict what customers will want
Tailor operations to align with customer needs
Gain critical insights continuously flowing in from data
Improve process efficiency
Enhance operational efficacy instantaneously
The synergy between big data and IoT has opened avenues for organisations to tap into these competencies, propelling their growth trajectory toward greater prosperity.
Differences Between Data Science and Related Fields
It is essential to understand the distinctive features of data science and how it stands apart from allied domains such as data analytics, business analytics, and machine learning. Although these fields are interrelated, and each plays a role in using data for informed decision-making, they differ in their emphases, methodologies, and specific contributions.
Data Science vs. Data Analytics
The difference between data analysts and data scientists can be characterised by the breadth and depth of their work. Data science dives into a more comprehensive array of tasks, including predictive modelling and developing sophisticated algorithms, while data analytics concentrates on digesting and portraying information to discern patterns. Typically, those in data analytics harness tools like SQL and Tableau for cleansing the data and presentation purposes, while those in the realm of science employ more complex technologies such as Python or R to execute machine learning processes and anticipatory analyses.
Understanding this distinction is vital for companies when recruiting personnel suited to their operational requirements. Analysts examine current datasets while scientists look forward. Forecasting trends involves seeing what’s around the corner based upon scientific approaches within machine learning domains—all intended to help plan effective strategies informed by present insights from analysts alongside future projections posited by scientists adept at handling vast quantities of intricate datasheets.
Data Science vs. Business Analytics
Data science and business, along with business analytics, both pursue the objective of leveraging data’s potential. There’s a notable difference in their approaches: data science relies on exploiting unstructured data through interdisciplinary strategies to gain knowledge while analytics in science and business emphasises reviewing historical information to enhance decision-making processes within businesses. Business analysts use statistical techniques and tools such as ERP systems and business intelligence software to derive valuable insights from past events.
This contrast between these domains underscores how vital it is that certain skills are matched appropriately with respective corporate goals. Data scientists bring an extensive set of technical capabilities alongside machine learning proficiency, which makes them ideal for developing new data-driven products or creating sophisticated predictive models.
On the other side of this spectrum are business analysts who channel their analytical acumen into interpreting complex datasets. They deliver concrete advice aimed at improving operational practices, and social aid companies map out a course for future endeavours requiring strategic foresight.
Data Science vs. Machine Learning
Within the expansive domain of data science, machine learning is a distinct subset centred around algorithms designed to learn from and make predictions based on data. The emphasis on adaptive learning and forecasting distinguishes machine-learning initiatives from the broader scope of data science, which includes various tasks such as data analysis and data processing.
There is an unmistakable interplay between data science and machine learning. With data science, laying down the foundational infrastructure and providing the necessary datasets upon which models for machine learning are devised and honed. Together, they form a formidable force driving industry evolution by facilitating automated complex decision-making systems and engendering deep insights at scales previously unseen.
Ethical Considerations in Data Science
Navigating the digital landscape comes with its own moral compasses, and in the field of data science, ethical considerations are at the forefront. Privacy, bias, and social impact are paramount, as data misuse can lead to violations of individual rights and the perpetuation of societal inequalities. Data ethics, therefore, becomes a guiding principle, ensuring that the collection and use of data are governed by standards that respect privacy, consent, and fairness.
Transparency in data collection and use policies is essential in building trust between data providers and users. As machine learning models become more prevalent, addressing and mitigating biases in training data is critical to ensure fair and unbiased outcomes. Ethical data science is about more than just compliance with regulations; it’s about fostering an environment where the benefits of data are balanced with the responsibility to use it wisely and humanely.
Career Paths in Data Science
In the present era, where data reigns supreme, there is a surging demand for professionals in the field of data science across various industries, opening up a realm brimming with career possibilities. The new trailblazers of the digital era are those adept at dissecting complex data to draw out significant implications. Many entities ranging from behemoth tech companies to sprouting startups are on the lookout for proficient data scientists, machine learning experts, and data engineers—roles that come with enticing remuneration and influential positions in shaping both technological advancements and corporate landscapes.
Possessing a distinct combination of competencies, including command over programming languages, statistical acumen, savvy in machine learning techniques as well as prowess in decoding intricate datasets into practicable tactics, defines what it means to be a successful data scientist today. Conversely, roles like data analysts primarily entail sifting through vast amounts of information to uncover trends that can steer tactical business resolutions. This necessitates mastery of analytical tools such as SASR and Python.
In the diverse spectrum of data science careers, data engineers take charge of building and overseeing infrastructure facilitating smooth data transfers. At the same time, machine learning specialists work to enhance predictive algorithms—each career offers its own unique complexities and fulfilling opportunities.
The Future of Data Science
Peering into the future, we can see a vibrant and continuously evolving landscape for data science. The rise of cloud computing is levelling the playing field by granting access to high-powered computational tools, thus empowering data scientists to handle vast datasets with unparalleled ease. In this age where the significance of data storage cannot be overstated, blockchain technology stands as a bastion for security and transparency in managing our digital information—assuring both integrity and verifiability when dealing with transactions involving data.
As innovations forge ahead, such as augmented analytics paving the way towards automated processing and data explorations, organisations are given more freedom to concentrate on gleaning interpretations from their insights rather than entangling themselves within complex facets of handling raw material—the intricate aspects tied up with processing it. Burgeoning marketplaces devoted exclusively to data are redefining their value proposition. Transforming it not just into an operational necessity but also into a negotiable currency ripe for a trade-off or potential financial gain. This revolutionary step broadens horizons for both entities conducting businesses and enfranchising individuals.
For companies seeking relevance and professionals aspiring toward continued significance within this emerging terrain driven by datum dynamics, it’s crucial they keep pace and adapt progressively. They must harness every technological tide shift efficiently while skillfully leveraging what already lies at their fingertips: existing troves of valuable data ready at hand.
Summary
Throughout this journey, we have unveiled the layers of data science, a domain where mathematics, statistics, and programming converge to create a symphony of insights. We’ve explored the evolution of this field, from its early intersection with computer science to its current state as an indispensable tool for modern business. The key classification, regression, and clustering techniques have been dissected, revealing their power to predict, analyse, and interpret the wealth of data surrounding us.
As we’ve seen, data science is not only about the tools and techniques but also about the ethical implications and the impact on society. It’s a field with a dynamic range of career opportunities, each offering the chance to significantly contribute to the world of data. With the future beckoning with advancements like machine learning and cloud computing, the potential for data science to continue reshaping industries is boundless. May this guide inspire you to delve deeper into data science, whether as a professional, a student, or an enthusiast eager to understand the forces shaping our data-driven world.
Frequently Asked Questions
What is the difference between data science and data analytics?
Machine learning and predictive modelling are integral components of data science, while identifying trends and informed decision-making is at the core of data analytics through its emphasis on processing and visualising data. Both disciplines are essential in deriving significant insights from vast amounts of information.
Can data science be used to innovate products and solutions?
Certainly, data science can spur innovation in products and solutions by uncovering process inefficiencies and fostering innovations rooted in data.
What are some of the ethical considerations in data science?
Ethical considerations in data science are fundamental for maintaining responsible practices and entail safeguarding privacy, avoiding bias, and contemplating the broader societal implications to direct appropriate data gathering and utilisation. Data ethics play a pivotal role in ensuring these responsible behaviours.
What skills are required to become a data scientist?
In pursuit of a career as a data scientist, one must acquire proficiency in programming languages such as SAS, R, and Python, possess strong statistical knowledge, be able to visualise data effectively, and be well-versed in frameworks for machine learning and data processing.
What does the future of data science look like?
The future of data science looks promising, with advancements in technologies such as cloud computing, blockchain, augmented analytics, and data marketplaces revolutionising data processing and analysis across industries.

Insurers: Beyond Transactions, What’s Your People Policy?
The insurance industry is filled with potential, but companies remain stuck in old ways, leaving customers unhappy and disconnected. One in three customers switched providers last year, due to unsatisfactory insurance customer experiences. At LIKE.TG, we ask, “What do people truly want when they interact with their insurance company?”
Extensive research shows that customers, both new and old, want more than just coverage and affordability. They seek understanding, simplicity, and empathy. Only 43% of customers say their insurer anticipates their needs, a disappointing statistic considering evolving expectations.
Let’s look at this through the lens of a typical customer. Meet Natalie, a physical therapist and mother, who’s budget-conscious, comfortable with technology, and who values human connection when engaging with businesses, no matter the size. We’ll trace her path to understand where she feels supported and, more importantly, where she feels lost while navigating her insurance journey.
Our goal is to examine how we might reimagine the insurance customer experience in a way that speaks to her core needs and desires, inspiring transformation along the way. Let’s begin.
Stage 1: Searching for security
In this opening stage, Natalie feels anxious but hopeful as she begins evaluating her options for how to best protect her home and automobiles. Her discovery can either empower her with clarity and conviction or leave her feeling overwhelmed and uncertain. As a discerning consumer, Natalie has the grit to push past the noise to find the right solution, but insurance companies vying for her business must challenge themselves to deliver a helpful, seamless experience.
Today’s customer experience, with its numerous touchpoints and channels, can present several challenges for individuals like Natalie as they start to explore their options and evaluate potential solutions. A majority of insurers use three or more systems for client engagement, frustrating customers as they encounter repetitive tasks across different channels. This often leads to key data and information getting duplicated or lost in the shuffle. For the insurer, this puts an unnecessary administrative burden on their employees, who should be focused on building relationships and delivering value.
Tomorrow’s insurance customer experience:
At LIKE.TG, we’re helping design an entirely new insurance customer experience with intelligent systems and automation. As Natalie evaluates her options, her information and activity are discreetly monitored and used for context. This advantages both the end customer and the agent. It ensures a thoughtful experience by recalling Natalie’s journey and providing tailored guidance and reduced friction, while also helping agents know when to prioritise her for personal follow-up. Overall, the streamlined approach empowers agents to excel in relationship-building while also boosting productivity.
Stage 2: Getting to know the insurer
After choosing an insurer, Natalie enters the onboarding phase, where initial interactions set the tone of her relationship. Carefully designed onboarding can instill trust and reassurance. At this stage, insurance companies should lead with thoughtfulness around the reasons for which the policy was purchased, going beyond transaction talk. Onboarding is not a checkbox to be marked complete; it is an opportunity to demonstrate the company values while creating moments to understand what customers like Natalie expect from the insurer.
Insurance onboarding frequently amounts to a series of decontextualised emails, overwhelming new customers with policy minutiae, bundling promotions, add-on features, loyalty programs, and app downloads. The communications can feel impersonal and poorly timed – a missed opportunity and a significant shortcoming of today’s customer experience since the onboarding window is when Natalie will be most attentive and engaged.
Tomorrow’s insurance customer experience:
The future of onboarding will vastly improve. Insurers will simplify the process for customers like Natalie and provide only essential information. Onboarding that keeps customer needs at the core can help obtain meaningful consent and establish trust and transparency around data practices. This, coupled with the harmonisation of engagement, behavioural signals, and third-party data will help insurers anticipate Natalie’s needs, resulting in her feeling connected and supported in her interactions.
With a renewed understanding that onboarding is an ongoing practice, insurers will only introduce new offers and programs when the moment is right. If six months in, Natalie adds a new young driver to her policy, an insurer might seize the opportunity to promote a young driver discount program.
Activating a data-driven approach can create a trusted environment where customers like Natalie share more data because they feel heard, understood, and helped. By understanding this ‘trust’-oriented purpose behind the data model, insurers can make informed decisions about what data to collect and how to use it, ultimately leading to better outcomes for both the customer and the organisation.
Personalise your customer journey
Learn how to manage, track, and automate customer interactions with smarter technology. This Trail is a helpful learning module that can help you get started on the free online learning platform by LIKE.TG.
Take the Trail
+2900 points
Trail
Deliver Personalised Insurance Service with Financial Services Cloud
Learn the ways of this trail.
Stage 3: Filing a claim
When the unexpected strikes, Natalie may need to submit a claim. How efficiently and compassionately her insurer handles this process can impact her overall satisfaction and trust in the company. A claim signals uncharted territory where Natalie feels vulnerable and relies on guidance. Now is the time for companies to demonstrate their values in action.
When an incident occurs today, Natalie will submit a claim amid stress and uncertainty – only to face disjointed, manual processes that exacerbate her challenges. While some insurance companies have modernised their claims experience, much of the industry lags, resulting in today’s fragmented experiences marked by delays, opacity, and eroded trust.
Get articles selected just for you, in your inbox
Sign up now
Purpose-driven insurers view claims as an opportunity to provide comfort and care when customers feel most vulnerable. They recognise that efficient claims fuel trust and loyalty more than marketing ever could. By leveraging existing data and new technologies, insurers can transform a traditionally tedious and anxiety-inducing process into a streamlined, personalised journey.
Tomorrow’s insurance customer experience:
Say Natalie previously enrolled in a usage-based auto insurance program to help manage costs and reward conscientious driving habits. The same telematics providing safe driving discounts can help expedite her claim. When an accident occurs, her location, speed, and impact data can be instantly shared, enabling insurers to proactively reach out, respond to the situation, and accelerate claim filing. For the policyholder, this means less stress during a difficult time and greater trust. The claims process, though rarely enjoyable, can at least be hassle-free.
This future experience, which is already here for our customers at LIKE.TG, is a powerful convergence of AI, data, and trust, underpinned by a foundation of customer-centricity – all in one tool.
Stage 4: Ongoing assurance
Regular interactions with the insurance company can either deepen Natalie’s engagement and loyalty or leave her feeling detached and uninformed.
In today’s customer experience, it is not uncommon for policyholders to only hear from their insurers during significant milestones such as policy issuance, billing, or claims. These interactions are often transactional in nature, focusing on the functional aspects of the policy rather than building a relationship with the customer.
Tomorrow’s insurance customer experience:
Natalie’s future experience is proactive and emotionally intelligent. Say she lives in a climate hazard zone – subject to hurricanes, floods, or fires. Because her insurer has a firm grasp on the risk she faces, she consistently receives prevention guidance and personalised offers to enhance protection. When disaster strikes, outreach is immediate, empathetic, and supportive. Natalie knows her insurer has her back and that she can trust them as an advisor who delivers tailored recommendations, anticipatory guidance, and compassionate care.
The ingredients are all there: rich data, smart technology, and most importantly, human-centered strategy. Now insurers must combine them to design powerful insurance customer experiences that put people first.
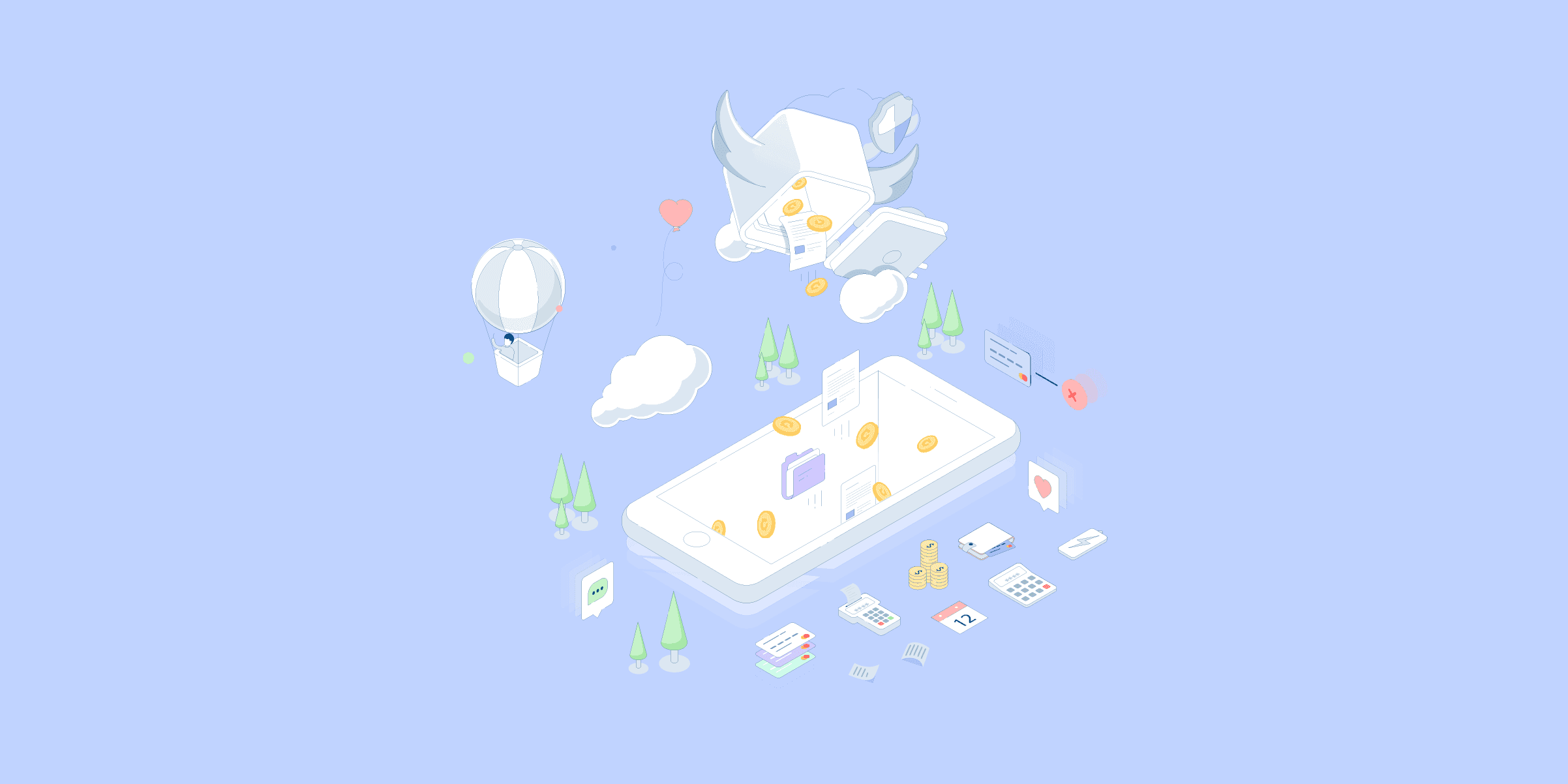
Amazon Redshift Vs Athena: Compare On 7 Key Factors
In the Data Warehousing and Business Analysis environment, growing businesses have a rising need to deal with huge volumes of data. In cases like this, key stakeholders often debate on whether to go with Redshift or with Athena – two of the big names that help seamlessly handle large chunks of data. This blog aims to ease this dilemma by providing a detailed comparison of Redshift Vs Athena.Although both the services are designed for Analytics, both the services provide different features and optimize for different use cases. This blog covers the following:
Amazon Redshift Vs Athena – Brief Overview
Amazon Redshift Overview
Amazon Redshift is a fully managed, petabyte data warehouse service over the cloud. Redshift data warehouse tables can be connected using JDBC/ODBC clients or through the Redshift query editor.
Redshift comprises Leader Nodes interacting with Compute nodes and clients. Clients can only interact with a Leader node. Compute nodes can have multiple slices. Slices are nothing but virtual CPUs
Athena Overview
Amazon Athena is a serverless Analytics service to perform interactive queries over AWS S3. Since Athena is a serverless service, the user or Analyst does not have to worry about managing any infrastructure. Athena query DDLs are supported by Hive and query executions are internally supported by Presto Engine. Athena only supports S3 as a source for query executions. Athena supports almost all the S3 file formats to execute the query. Athena is well integrated with AWS Glue Crawler to devise the table DDLs
Redshift Vs Athena Comparison
Feature Comparison
Amazon Redshift Features
Redshift is purely an MPP data warehouse application service used by the Analyst or Data warehouse engineer who can query the tables. The tables are in columnar storage format for fast retrieval of data. You can watch a short intro on Redshift here:
Data is stored in the nodes and when the Redshift users hit the query in the client/query editor, it internally communicates with Leader Node. The leader node internally communicates with the Compute node to retrieve the query results. In Redshift, both compute and storage layers are coupled, however in Redshift Spectrum, compute and storage layers are decoupled.
Athena Features
Athena is a serverless analytics service where an Analyst can directly perform the query execution over AWS S3. This service is very popular since this service is serverless and the user does not have to manage the infrastructure. Athena supports various S3 file-formats including CSV, JSON, parquet, orc, and Avro. Along with this Athena also supports the Partitioning of data. Partitioning is quite handy while working in a Big Data environment
Redshift Vs Athena – Feature Comparison Table
Scope of Scaling
Both Redshift and Athena have an internal scaling mechanism.
Get the best content from the world of data science in your inbox once a month.Thank you for Subscribing to our Newsletter!
Amazon Redshift Scaling
Since data is stored inside the node, you need to be very careful in terms of storage inside the node. While managing the cluster, you need to define the number of nodes initially. Once the cluster is ready with a specific number of nodes, you can reduce or increase the nodes.
Redshift provides 2 kinds of node resizing features:
Elastic resize
Classic resize
Elastic Resize
Elastic resize is the fasted way to resize the cluster. In the elastic resize, the cluster will be unavailable briefly. This often happens only for a few minutes. Redshift will place the query in a paused state temporarily. However, this resizing feature has a drawback as it supports a resizing in multiples of 2 (for dc2.large or ds2.xlarge cluster) ie. 2 node clusters changed to 4 or a 4 node cluster can be reduced to 2, etc. Also, you cannot modify a dense compute node cluster to dense storage or vice versa.
This resize method only supports VPC platform clusters.
Classic Resize
Classic resize is a slower way of resizing a cluster. Your cluster will be in a read-only state during the resizing period. This operation may take a few hours to days depending upon the actual data storage size. For classic resize you should take a snapshot of your data before the resizing operation.
Workaround for faster resize -> If you want to increase 4 node cluster to 10 node cluster, perform classic resize to 5 node cluster and then use elastic resize to increase 10 node cluster for faster resizing.
Athena Scaling
Being a serverless service, you do not have to worry about scaling in Athena. AWS manages the scaling of your Athena infrastructure. However, there is a limit on the number of queries, databases defined by AWS ie. number of concurrent queries, the number of databases per account/role, etc.
Ease of Data Replication
Amazon Redshift – Ease of Data Replication
In Redshift, there is a concept of the Copy command. Using the Copy command, data can be loaded into Redshift from S3, Dynamodb, or EC2 instances. Although the Copy command is for fast loading it will work at its best when all the slices of nodes equally participate in the copy command
Download the Guide to Select the Right Data Warehouse
Learn the key factors you should consider while selecting the right data warehouse for your business.
Below is an example:
copy table from 's3://<your-bucket-name>/load/key_prefix'
credentials 'aws_access_key_id=<Your-Access-Key-ID>;aws_secret_access_key=<Your-Secret-Access-Key>' Options;
You can load multiple files in parallel so that all the slices can participate. For the COPY command to work efficiently, it is recommended to have your files divided into equal sizes of 1 MB – 1 GB after compression.
For example, if you are trying to load a file of 2 GB into DS1.xlarge cluster, you can divide the file into 2 parts of 1 GB each after compression so that all the 2 slices of DS1.xlarge can participate in parallel.
Please refer to AWS documentation to get the slice information for each type of Redshift node.
Using Redshift Spectrum, you can further leverage the performance by keeping cold data in S3 and hot data in the Redshift cluster. This way you can further improve your performance.
In case you are looking for a much easier and seamless means to load data to Redshift, you can consider fully managed Data Integration Platforms such as LIKE.TG . LIKE.TG helps load data from any data source to Redshift in real-time without having to write any code.
Athena – Ease of Data Replication
Since Athena is an Analytical query service, you do not have to move the data into Data Warehouse. You can directly query your data over S3 and this way you do not have to worry about node management, loading the data, etc.
Data Storage Formats Supported by Redshift and Athena
Redshift data warehouse only supports structured data at the node level. However, Redshift Spectrum tables do also support other storage formats ie. parquet, orc, etc.
On the other hand, Athena supports a large number of storage formats ie. parquet, orc, Avro, JSON, etc. It also has a feature called Glue classifier. Athena is well integrated with AWS Glue. Athena table DDLs can be generated automatically using Glue crawlers too. Glue has saved a lot of significant manual tasks of writing manual DDL or defining the table structure manually. In Glue, there is a feature called a classifier.
Using the Glue classifier, you can make Athena support a custom file type. This is a much better feature that made Athena quite handy dealing in with almost all the types of file formats.
Data Warehouse Performance
Redshift Data Warehouse Performance
The performance of the data warehouse application is solely dependent on the way your cluster is defined. In Redshift, there is a concept of Distribution key and Sort key. The distribution key defines the way how your data is distributed inside the node. The distribution key drives your query performance during the joins. Sort key defines the way data is stored in the blocks. The more the data is in sorted order the faster the performance of your query will be.
Sort key can be termed as a replacement for an index in other MPP data warehouses. Sort keys are primarily taken into effect during the filter operations. There are 2 types of sort keys (Compound sort keys and Interleaved sort keys). In compound sort keys, the sort keys columns get the weight in the order the sort keys columns are defined. On the other hand in the compound sort key, all the columns get equal weightage. Interleaved sort keys are typically used when multiple users are using the same query but are unsure of the filter condition
Another important performance feature in Redshift is the VACUUM. Bear in mind VACUUM is an I/O intensive operation and should be used during the off-business hours. However, off-late AWS has introduced the feature of auto-vacuuming however it is still advised to vacuum your tables during regular intervals. The vacuum will keep your tables sorted and reclaim the deleted blocks (For delete operations performed earlier in the cluster). You can read about Redshift VACUUM here.
Athena Performance
Athena Performance primarily depends on the way you hit your query. If you are querying a huge file without filter conditions and selecting all the columns, in that case, your performance might degrade. You need to be very cautious in selecting only the needful columns. You are advisable to partition your data and store your data in columnar/compressed format (ie. parquet or orc). In case you want to preview the data, better perform the limit operation else your query will take more time to execute.
Example:-
Select * from employee; -- High run time
Select * from employee limit 10 -- better run time
Amazon Redshift Vs Athena – Pricing
AWS Redshift Pricing
The performance of Redshift depends on the node type and snapshot storage utilized. In the case of Spectrum, the query cost and storage cost will also be added
Here is the node level pricing for Redshift for the N.Virginia region (Pricing might vary based on region)
AWS Athena Pricing
The good part is that in Athena, you are charged only for the amount of data for which the query is scanned. Your query needs to be designed such that it does not perform unnecessary scans. As a best practice, you should compress and partition the data to save the cost significantly
The usage cost of N.Virginia is $5 per TB of data scanned (The pricing might vary based on region)
Along with the query scan charge, you are also charged for the data stored in S3
Architecture
Athena – Architecture
Athena is a serverless platform with a decoupled storage and compute architecture that allows users to query data directly in S3 without having to ingest or copy it. It is multi-tenant and uses shared resources. Users have no control over the compute resources that Athena allocates from the shared resource pool per query.
Amazon Redshift Architecture
The oldest architecture in the group is Redshift, which was the first Cloud DW. Its architecture was not built to separate storage and computation. While it now has RA3 nodes, which allow you to scale compute and only cache the data you need locally, it still runs as a single process. Because different workloads cannot be separated and isolated over the same data, it lags behind other decoupled storage/computing architectures. Redshift is deployed in your VPC as an isolated tenant per customer, unlike other cloud data warehouses.
Scalability
Athena – Scalability
Athena is a multi-tenant shared resource, so there are no guarantees about the amount or availability of resources allocated to your queries. It can scale to large data volumes in terms of data volume, but large data volumes can result in very long run times and frequent time outs. The maximum number of concurrent queries is 20. Athena is probably not the best choice if scalability is a top priority.
Redshift – Scalability
Even with RA3, Redshift’s scale is limited because it can’t distribute different workloads across clusters. While it can automatically scale up to 10 clusters to support query concurrency, it can only handle 50 queued queries across all clusters by default.
Use Cases
Athena – Use Cases
For Ad-Hoc analytics, Athena is a great option. Because Athena is serverless and handles everything behind the scenes, you can keep the data where it is and start querying without worrying about hardware or much else. When you need consistent and fast query performance, as well as high concurrency, it isn’t a good fit. As a result, it is rarely the best option for operational or customer-facing applications. It can also be used for batch processing, which is frequently used in machine learning applications.
Redshift – -Use Cases
Redshift was created to help analysts with traditional internal BI reporting and dashboard use cases. As a result, it’s commonly used as a multi-purpose Enterprise data warehouse. It can also use the AWS ML service because of its deep integrations into the AWS ecosystem, making it useful for ML projects. It is less suited for operational use cases and customer-facing use cases like Data Apps, due to the coupling of storage and compute and the difficulty in delivering low-latency analytics at scale. It’s difficult to use for Ad-Hoc analytics because of the tight coupling of storage and compute, as well as the requirement to pre-define sort and dist keys for optimal performance.
Data Security
Amazon Redshift – Data Security
Redshift has various layers of security
Cluster credential level security
IAM level security
Security group-level security to control the inbound rules at the port level
VPC to protect your cluster by launching your cluster in a virtual networking environment
Cluster encryption -> Tables and snapshots can be encrypted
SSL connects can be encrypted to enforce the connection from the JDBC/ODBC SQL client to the cluster for security in transit
Has facility the load and unload of the data into/from the cluster in an encrypted manner using various encryption methods
It has a feature of CloudHSM. With the help of CloudHSM, you can use certificates to configure a trusted connection between Redshift and your HSM environment
Athena: Data Security
You can query your tables either using console or CLI
Being a serverless service, AWS is responsible for protecting your infrastructure. Third-party auditors validate the security of the AWS cloud environment too.
At the service level, Athena access can be controlled using IAM.
Below is the encryption at rest methodologies for Athena:
Service side encryption (SSE-S3)
KMS encryption (SSE-KMS)
Client-side encryption with keys managed by the client (CSE-KMS)
Security in Transit
AWS Athena uses TLS level encryption for transit between S3 and Athena as Athena is tightly integrated with S3.
Query results from Athena to JDBC/ODBC clients are also encrypted using TLS.
Athena also supports AWS KMS to encrypted datasets in S3 and Athena query results. Athena uses CMK (Customer Master Key) to encrypt S3 objects.
Conclusion
Both Redshift and Athena are wonderful services as Data Warehouse applications. If used in conjunction, it can provide great benefits. One should use Amazon Redshift when high computation is required and query large datasets and use Athena for simple queries.
Share your experience of learning about Redshift vs Athena in the comments section below!
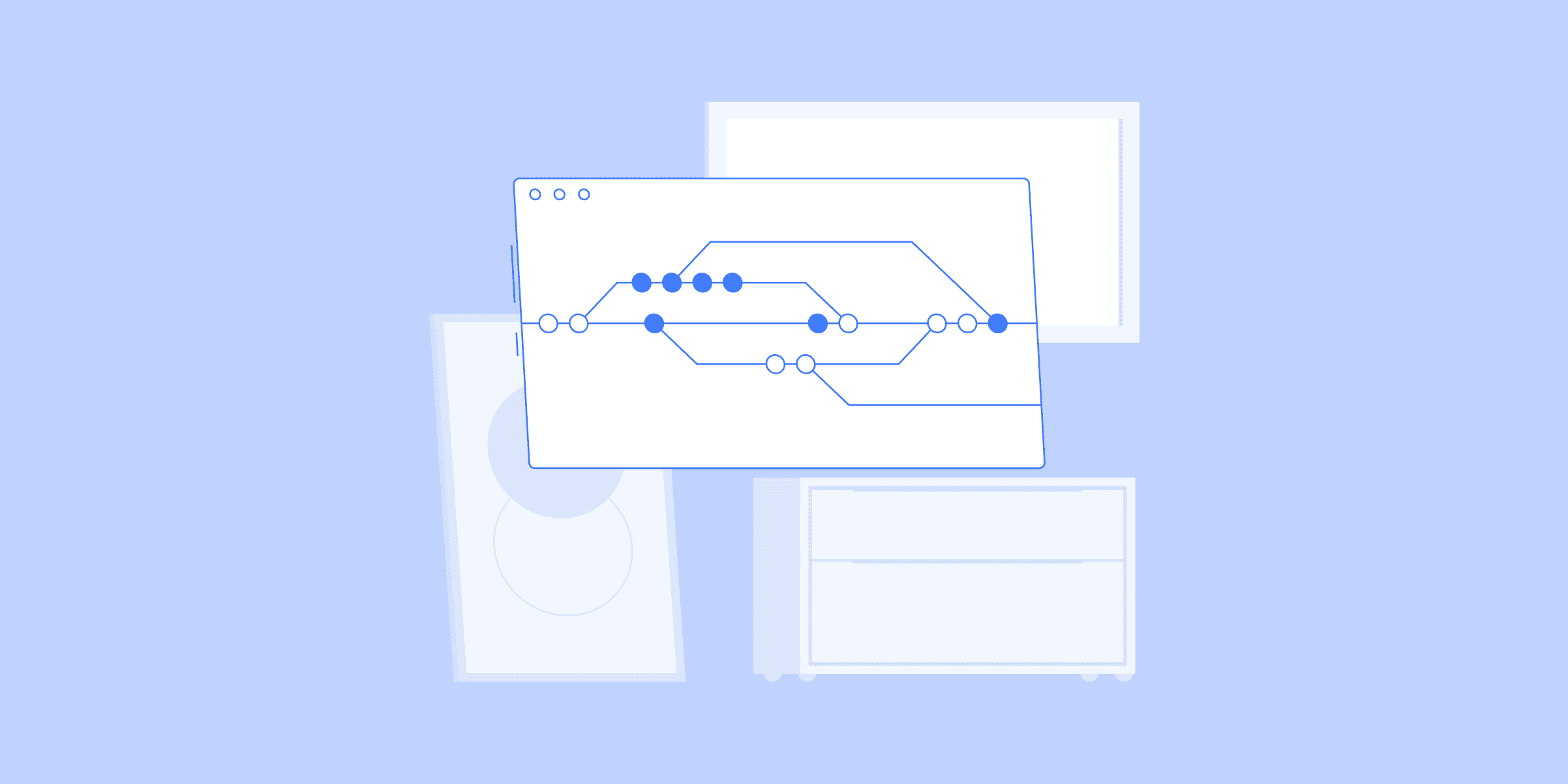
LIKE.TG vs DMS AWS – 7 Comprehensive Parameters
Migrating data from different sources into Data Warehouses can be hard. Hours of engineering time need to be spent in hand-coding complex scripts to bring data into the Data Warehouse. Moreover, Data Streaming often fails due to unforeseen errors for eg. the destination is down or an error in a piece of code. With the increase in such overheads, opting for a Data Migration product becomes impertinent for smooth Data Migration.LIKE.TG Data and DMS AWS are two very effective ETL tools available in the market and users are often confused while deciding one of them. The LIKE.TG vs DMS AWS is a constant dilemma amongst the users who are looking for a hassle-free way to automate their ETL process.
This post on LIKE.TG vs DMS AWS has attempted to highlight the differences between LIKE.TG and AWS Database Migration Service on a few critical parameters to help you make the right choice. Read along with the comparisons of LIKE.TG VS DMS AWS and decide which one suits you the best.
Introduction to LIKE.TG Data
LIKE.TG is a Unified Data Integration platform that lets you bring data into your Data Warehouse in real-time. With a beautiful interface and flawless user experience, any user can transform, enrich and clean the data and build data pipelines in minutes. Additionally, LIKE.TG also enables users to build joins and aggregates to create materialized views on the data warehouse for faster query computations.
LIKE.TG also helps you to start moving data from 100+ sources to your data warehouse in real-time with no code for the price of $249/month!
To learn more about LIKE.TG Data, visit here.
Introduction to AWS DMS
AWS DMS is a fully managed Database Migration service provided by Amazon. Users can connect various JDBC-based data sources and move the data from within the AWS console.
AWS Database Migration Service allows you to migrate data from various Databases to AWS quickly and securely. The original Database remains fully functional during the migration, thereby minimizing downtime for applications that depend on the Database.
To learn more about DMS AWS, visit here.
Simplify your ETL Process with LIKE.TG Data
LIKE.TG Datais a simple to use Data Pipeline Platform that helps you load data from100+ sourcesto any destination like Databases, Data Warehouses, BI Tools, or any other destination of your choice in real-time without having to write a single line of code. LIKE.TG provides you a hassle-free data transfer experience. Here are some more reasons why LIKE.TG is the right choice for you:
Minimal Setup Time: LIKE.TG has a point-and-click visual interface that lets you connect your data source and destination in a jiffy. No ETL scripts, cron jobs, or technical knowledge is needed to get started. Your data will be moved to the destination in minutes, in real-time.Automatic Schema Mapping:Once you have connected your data source, LIKE.TG automatically detects the schema of the incoming data and maps it to the destination tables. With its AI-powered algorithm, it automatically takes care of data type mapping and adjustments – even when the schema changes at a later point.Mature Data Transformation Capability:LIKE.TG allows you to enrich, transform and clean the data on the fly using an easy Python interface. What’s more – LIKE.TG also comes with an environment where you can test the transformation on a sample data set before loading to the destination.Secure and Reliable Data Integration:LIKE.TG has a fault-tolerant architecture that ensures that the data is moved from the data source to destination in a secure, consistent and dependable manner with zero data loss.Unlimited Integrations: LIKE.TG has a large integration list for Databases, Data Warehouses, SDKs Streaming, Cloud Storage, Cloud Applications, Analytics, Marketing, and BI tools. This, in turn, makes LIKE.TG the right partner for the ETL needs of your growing organization.
Try out LIKE.TG by signing up for a14-day free trial here.
Comparing LIKE.TG vs DMS AWS
1) Variety of Data Source Connectors: LIKE.TG vs DMS AWS
The starting point of the LIKE.TG vs DMS AWS discussion is the number of data sources these two can connect. With LIKE.TG you can migrate data from not only JDBC sources, but also from various cloud storage (Google Drive, Box, S3) SaaS (Salesforce, Zendesk, Freshdesk, Asana, etc.), Marketing systems (Google Analytics, Clevertap, Hubspot, Mixpanel, etc.) and SDKs (iOS, Android, Rest, etc.). LIKE.TG supports the migration of both structured and unstructured data. A complete list of sources supported by LIKE.TG can be found here.
LIKE.TG supports all the sources supported by DMS and more.
DMS, on the other hand, provides support to only JDBC databases like MySQL, PostgreSQL, MariaDB, Oracle, etc. A complete list of sources supported by DMS can be found here.
However, if you need to move data from other sources like Google Analytics, Salesforce, Webhooks, etc. you would have to build and maintain complex scripts for migration to bring it into S3. From S3, DMS can be used to migrate the data to the destination DB. This would make migration a tedious two-step process.
DMS does not provide support to move unstructured NoSQL data.
Other noteworthy differences on the source side:
LIKE.TG promises a secure SSH connection when moving data whereas DMS does not.
LIKE.TG also allows users to write custom SQL to move partial data or perform table joins and aggregates on the fly while DMS does not.
With LIKE.TG users can enjoy granular control on Table jobs. LIKE.TG lets you control data migration at table level allowing you to pause the data migration for certain tables in your database at will. DMS does not support such a setup.
LIKE.TG allows you to move data incrementally through SQL queries and BinLog. With DMS, incremental loading of data is possible only through BinLog.
2) Data Transformations: LIKE.TG vs DMS AWS
With LIKE.TG , users can Clean, Filter, Transform and Enrich both structured and unstructured data on the fly through a simple Python interface. You can even split an incoming event into multiple arbitrary events making it easy for you to normalize nested NoSQL data. All the standard Python Libraries are made available to ensure users have a hassle-free data transformation experience. The below image shows the data transformation process at LIKE.TG .
DMS allows users to create basic data transformations such as Adding a prefix, Changing letters to uppercase, Skip a column, etc. However, advanced transformations like Mapping IP to location, Skipping rows based on conditions, and many others that can be easily done on LIKE.TG are not supported by DMS.
The above image shows the Data transformation process of DMS AWsS. To be sure that the transformation is error-free, DMS users will have to hand-code sample event pulls and experiment on them or worse, wait for data to reach the destination to check. LIKE.TG lets users test the transformation on a sample data set and preview the result before deployment.
3) Schema handling: LIKE.TG vs DMS AWS
Schemas are important for the ETL process and therefore can act as a good parameter in the LIKE.TG vs DMS discussion. LIKE.TG allows you to map the source schema to the destination schema on abeautiful visual interface. DMS does not have an interface for schema mapping. The data starts moving as soon as the job is configured. If the mapping is incorrect the task fails and someone from engineering will have to manually fix the errors.
Additionally, LIKE.TG automatically detects the changing schema and notifies the user of the change so that he can take necessary action.
4) Moving Data into Redshift: LIKE.TG vs DMS AWS
Amazon Redshift is a popular Data Warehouse and can act as a judging parameter in this LIKE.TG vs DMS AWS discussion. Moving Data into Redshift is a cakewalk with LIKE.TG . Users would just need to connect the sources to Redshift, write relevant transformations, and voila, data starts streaming.
Moving data into Redshift through DMS comes with a lot of overheads. Users are expected to manage the S3 bucket (creating directories, managing permissions, etc.) themselves. Moreover, DMS compulsorily requires the user’s Redshift cluster region, the DMS region to be the same. While this is not a major drawback, this becomes a problem when users want to change the region of the Redshift cluster but not for S3.
5) Notifications: LIKE.TG vs DMS AWS
LIKE.TG notifies all exceptions to users on both Slack and Email. The details of the exceptions are also included in the notification to enable users to take quick action.
DMS notifies all the anomalies over AWS Cloudwatch only. The user will have to configure Cloudwatch to receive notifications on email.
6) Statistics and Audit log: LIKE.TG vs DMS AWS
LIKE.TG provides a detailed audit log to the user to get visibility into activities that happened in the past at the user level. DMS provides logs at the task level.
LIKE.TG provides a simple dashboard that provides a one-stop view of all the tasks you have created. DMS provides data migration statistics on Cloudwatch.
7) Data Modelling: LIKE.TG vs DMS AWS
Data Modeling is another essential aspect of this LIKE.TG vs DMS AWS dilemma. LIKE.TG ’s Modelling and Workflows features allow you to join and aggregate the data to store results as materialized views on your destination. With these views, users experience faster query response times making any report pulls possible in a few seconds.
DMS restricts its functions to data migration services only. Data Models on LIKE.TG
Conclusion
The article explained briefly about LIKE.TG Data and DMS AWS. It then provided a detailed discussion on the LIKE.TG vs DMS AWS choice dilemma. The article considered 7 parameters to analyze both of these ETL tools. Moreover, it provided you enough information on each criterion used in the LIKE.TG vs DMS AWS discussion.
LIKE.TG Data, understand the complex processes involved in migrating your data from a source to a destination and LIKE.TG has been built just to simplify this for you. With a superior array of features as opposed to DMS, LIKE.TG ensures a hassle-free data migration experience with zero data loss.
LIKE.TG Data, with its strong integration with100+ sources BI tools, allows you to export, load, transform enrich your data make it analysis-ready in a jiffy.
Want to take LIKE.TG for a spin. Try LIKE.TG Data’s14 days free trialand experience the benefits!
Share your views on the LIKE.TG vs DMS discussion in the comments section!
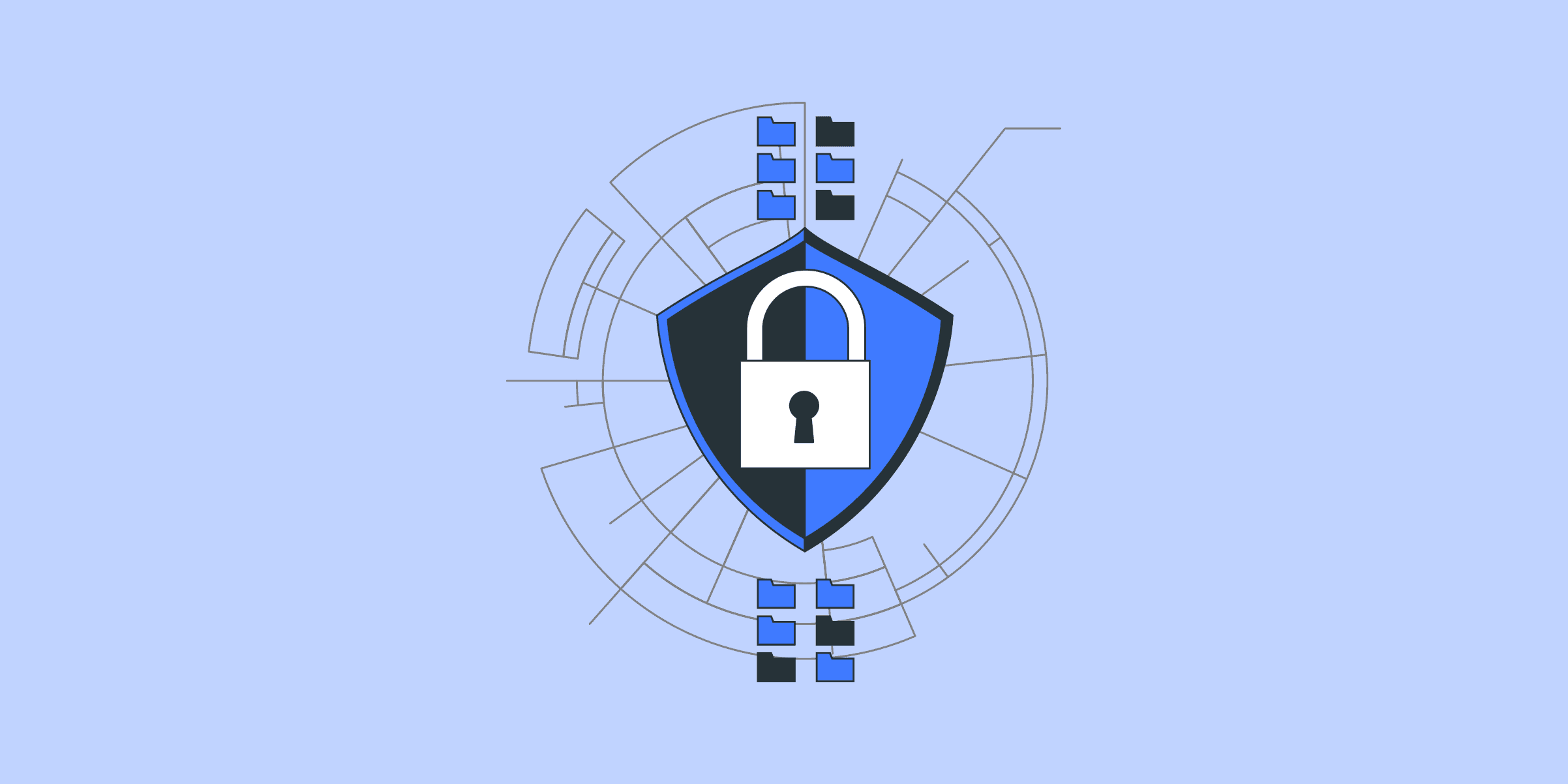
Webhook to BigQuery: Real-time Data Streaming
Nowadays, streaming data is a crucial data source for any business that wants to perform real-time analytics. The first step to analyze data in real-time is to load the streaming data – often from Webhooks, in real-time to the warehouse. A common use case for a BigQuery webhook is automatically sending a notification to a service like Slack or email whenever a dataset is updated. In this article, you will learn two methods of how to load real-time streaming data from Webhook to BigQuery.Note: When architecting a Webhooks Google BigQuery integration, it’s essential to address security concerns to ensure your data remains protected. Also, when connecting BigQuery webhook, defining your webhook endpoint is essential – the address or URL that will receive the incoming data is essential.
Connect Webhook to BigQuery efficiently
Utilize LIKE.TG ’s pre-built webhook integration to capture incoming data streams. Configure LIKE.TG to automatically transform and load the webhook data into BigQuery tables, with no coding required.
Method 1: Webhook to BigQuery using LIKE.TG Data
Get Started with LIKE.TG for Free
Method 2: Webhook to BigQuery ETL Using Custom Code
Develop a custom application to receive and process webhook payloads. Write code to transform the data and use BigQuery’s API or client libraries to load it into the appropriate tables.
Method 1: Webhook to BigQuery using LIKE.TG Data
LIKE.TG is the only real-time ELT No-code Data Pipeline platform that cost-effectively automates data pipelines that are flexible to your needs. With integration with 150+ Data Sources (40+ free sources), we help you not only export data from sources load data to the destinations but also transform enrich your data, make it analysis-ready.
LIKE.TG Data lets you load real-time streaming data from Webhook to BigQuery in two simple steps:
Step 1: Configure your source
Connect LIKE.TG Data with your source, in this case, Webhooks. You also need to specify some details, such as the Event Name Path and Fields Path.
Step 2: Select your Destination
Load data from Webhooks to BigQuery by selecting your destination. You can also choose the options for auto-mapping and JSON fields replication here.
Now you have successfully established the connection between Webhooks and BigQuery for streaming real-time data.
Click here to learn more on how to Set Up Webhook as a Source.
Click here to learn more on how to Set Up BigQuery as a Destination.
Integrate Webhooks to BigQueryGet a DemoTry itIntegrate Webhooks to RedshiftGet a DemoTry itIntegrate Webhooks to SnowflakeGet a DemoTry it
Method 2: Webhook to BigQuery ETL Using Custom Code
The steps involved in migrating data from WebHook to BigQuery are as follows:
Getting data out of your application using Webhook
Preparing Data received from Webhook
Loading data into Google BigQuery
Step 1: Getting data out of your application using Webhook
Setup a webhook for your application and define the endpoint URL on which you will deliver the data. This is the same URL from which the target application will read the data.
Step 2: Preparing Data received from Webhook
Webhooks post data to your specified endpoints in JSON format. It is up to you to parse the JSON objects and determine how to load them into your BigQuery data warehouse.
You need to ensure the target BigQuery table is well aligned with the source data layout, specifically column sequence and data type of columns.
Step 3: Loading data into Google BigQuery
We can load data into BigQuery directly using API call or can create CSV file and then load into BigQuery table.
Create a Python script to read data from the Webhook URL endpoint and load it into the BigQuery table.
from google.cloud import bigquery
import requests
client = bigquery.Client()
dataset_id = 'dataset_name'
#replace with your dataset ID
table_id = 'table_name'
#replace with your table ID
table_ref = client.dataset(dataset_id).table(table_id)
table = client.get_table(table_ref) # API request
receive data from WebHook
Convert received data into rows to insert into BigQuery
errors = client.insert_rows(table, rows_to_insert)# API request
assert errors == []
You can store streaming data into a file by a specific interval and use the bq command-line tool to upload the files to your datasets, adding schema and data type information. In the GCP documentation of the GSUTIL tool, you can find the syntax of the bq command line. Iterate through this process as many times as it takes to load all of your tables into BigQuery.
Once the data has been extracted from your application using Webhook, the next step is to upload it to the GCS. There are multiple techniques to upload data to GCS.
Upload file to GCS bucket
Using Gsutil: Using Gsutil utility we can upload a local file to GCS(Google Cloud Storage) bucket.
gsutil cp local_folder/file_name.csv gs://gcs_bucket_name/path/to/folder/
To copy a file to GCS:
Using Web console: An alternative way to upload the data from your local machine to GCS is using the web console. To use the web console option follow the below steps.
First of all, you need to login to your GCP account. You must have a working Google account of GCP. In the menu option, click on storage and navigate to the browser on the left tab.
If needed create a bucket to upload your data. Make sure that the name of the bucket you choose is globally unique.
Click on the bucket name that you have created in step #2, this will ask you to browse the file from your local machine.
Choose the file and click on the upload button. A progression bar will appear. Next, wait for the upload to complete. You can see the file is loaded in the bucket.
Create Table in BigQuery
Go to the BigQuery from the menu option.
On G-Cloud console, click on create a dataset option. Next, provide a dataset name and location.
Next, click on the name of the created dataset. On G-Cloud console, click on create table option and provide the dataset name, table name, project name, and table type.
Load the data into BigQuery Table
Once the table is created successfully, you will get a notification that will allow you to use the table as your new dataset.
Alternatively, the same can be done using the Command Line as well.
Start the command-line tool and click on the cloud shell icon shown here.
The syntax of the bq command line to load the file in the BigQuery table:
Note: The Autodetect flag identifies the table schema
bq --location=[LOCATION] load --source_format=[FORMAT]
[DATASET].[TABLE] [PATH_TO_SOURCE] [SCHEMA]
[LOCATION] is an optional parameter that represents Location name like “us-east”
[FORMAT] to load CSV file set it to CSV [DATASET] dataset name.
[TABLE] table name to load the data.
[PATH_TO_SOURCE] path to source file present on the GCS bucket.
[SCHEMA] Specify the schema
bq --location=US load --source_format=CSV your_dataset.your_table gs://your_bucket/your_data.csv ./your_schema.json
You can specify your schema using bq command line
Loading Schema Using the Web Console
BigQuery will display all the distinct columns that were found under the Schema tab.
Alternatively, to do the same in the command line, use the below command:
bq --location=US load --source_format=CSV your_dataset.your_table gs://your_bucket/your_data.csv ./your_schema.json
Your target table schema can also be autodetected:
bq --location=US load --autodetect --source_format=CSV your_dataset.your_table gs://mybucket/data.csv
BigQuery command-line interface allows us to 3 options to write to an existing table.
The Web Console has the Query Editor which can be used for interacting with existing tables using SQL commands.
Overwrite the table
bq --location = US load --autodetect --replace --source_file_format = CSV your_target_dataset_name.your_target_table_name gs://source_bucket_name/path/to/file/source_file_name.csv
Append data to the table
bq --location = US load --autodetect --noreplace --source_file_format = CSV your_target_dataset_name.your_table_table_name gs://source_bucket_name/path/to/file/source_file_name.csv ./schema_file.json
Adding new fields in the target table
bq --location = US load --noreplace --schema_update_option = ALLOW_FIELD_ADDITION --source_file_format = CSV your_target_dataset.your_target_table gs://bucket_name/source_data.csv ./target_schema.json
Update data into BigQuery Table
The data that was matched in the above-mentioned steps not done complete data updates on the target table. The data is stored in an intermediate data table. This is because GCS is a staging area for BigQuery upload. There are two ways of updating the target table as described here.
Update the rows in the target table. Next, insert new rows from the intermediate table
UPDATE target_table t
SET t.value = s.value
FROM intermediate_table s
WHERE t.id = s.id;
INSERT target_table (id, value)
SELECT id, value
FROM intermediate_table WHERE NOT id IN (SELECT id FROM target_table);
Delete all the rows from the target table which are in the intermediate table. Then, insert all the rows newly loaded in the intermediate table. Here the intermediate table will be in truncate and load mode.
DELETE FROM final_table f WHERE f.id IN (SELECT id from intermediate_table); INSERT data_setname.target_table(id, value) SELECT id, value FROM data_set_name.intermediate_table;
Sync your Webhook data to BigQuery
Start for Free Now
Limitations of writing custom Scripts to stream data from Webhook to BigQuery
The above code is built based on a certain defined schema from the Webhook source. There are possibilities that the scripts break if the source schema is modified.
If in future you identify some data transformations need to be applied on your incoming webhook events, you would require to invest additional time and resources on it.
Overload of incoming data, you might have to throttle the data moving to BQ.
Given you are dealing with real-time streaming data you would need to build very strong alerts and notification systems to avoid data loss due to an anomaly at the source or destination end. Since webhooks are triggered by certain events, this data loss can be very grave for your business.
Webhook to BigQuery: Use Cases
Inventory Management in E-commerce: E-commerce platforms can benefit from real-time inventory updates by streaming data from inventory management webhooks into BigQuery. This enables businesses to monitor stock levels, optimize supply chains, and prevent stockouts or overstocking, ensuring a seamless customer experience. Source
Patient Monitoring in Healthcare: Healthcare providers can leverage real-time data streaming for patient monitoring. By connecting medical device webhooks to BigQuery, clinicians can track patient health in real time, and receive alerts for abnormal readings, and provide timely interventions, ultimately leading to better patient outcomes.
Fraud Detection in Finance: Financial institutions can use webhooks to stream transaction data into BigQuery for fraud detection. Analyzing transaction patterns in real time helps to identify and prevent fraudulent activities, protect customer accounts, and ensure regulatory compliance.
Event-driven marketing: Businesses across various industries can stream event data, such as user sign-ups or product launches, into BigQuery. This allows for real-time analysis of marketing campaigns, enabling quick adjustments and targeted follow-ups to boost conversion rates.
Additonal Reads:
Python Webhook Integration: 3 Easy Steps
WhatsApp Webhook Integration: 6 Easy Steps
Best Webhooks Testing tools for 2024
Conclusion
In this blog, you learned two methods for streaming real-time data from Webhook to BigQuery: using an automated pipeline or writing custom ETL codes. Regarding moving data in real-time, a no-code data pipeline tool such as LIKE.TG Data can be the right choice for you.
Using LIKE.TG Data, you can connect to a source of your choice and load your data to a destination of your choice cost-effectively. LIKE.TG ensures your data is reliably and securely moved from any source to BigQuery in real time.
Want to take LIKE.TG for a spin?
Sign Up for a 14-day free trial and experience the feature-rich LIKE.TG suite firsthand.
Check out our LIKE.TG Pricing to choose the best plan for you. Do let us know if the methods were helpful and if you would recommend any other methods in the comments below.

Amazon Redshift vs Aurora: 9 Critical Differences
AuroraDB is a relational database engine that comes as one of the options in the AWS Relational Database as a service. Amazon Redshift, on the other hand, is another completely managed database service from Amazon that can scale up to petabytes of data. Even though the ultimate aim of both these services is to let customers store and query data without getting involved in the infrastructure aspect, these two services are different in a number of ways. In this post, we will explore Amazon Redshift Vs Aurora – how these two databases compare with each other in the case of various elements and which one would be the ideal choice in different kinds of use cases. In the end, you will be in the position to choose the best platform based on your business requirements. Let’s get started.
Introduction to Amazon Redshift
Redshift is a completely managed database service that follows a columnar data storage structure. Redshift offers ultra-fast querying performance over millions of rows and is tailor-made for complex queries over petabytes of data. Redshift’s querying language is similar to Postgres with a smaller set of datatype collection.
With Redshift, customers can choose from multiple types of instances that are optimized for performance and storage. Redshift can scale automatically in a matter of minutes in the case of the newer generation nodes. Automatic scaling is achieved by adding more nodes. A cluster can only be created using the same kind of nodes. All the administrative duties are automated with little intervention from the customer needed. You can read more on Redshift Architecture here.
Redshift uses a multi-node architecture with one of the nodes being designated as a leader node. The leader node handles client communication, assigning work to other nodes, query planning, and query optimization. Redshift’s pricing combines storage and computing with the customers and does not have the pure serverless capability. Redshift offers a unique feature called Redshift spectrum which basically allows the customers to use the computing power of the Redshift cluster on data stored in S3 by creating external tables.
To know more about Amazon Redshift, visit this link.
Introduction to Amazon Aurora
AuroraDB is a MySQL and Postgres compatible database engine; which means if you are an organization that uses either of these database engines, you can port your database to Aurora without changing a line of code. Aurora is enterprise-grade when it comes to performance and availability. All the traditional database administration tasks like hardware provisioning, backing up data, installing updates, and the likes are completely automated.
Aurora can scale up to a maximum of 64 TB. It offers replication across multiple availability zones through what Amazon calls multiAZ deployment. Customers can choose from multiple types of hardware specifications for their instances depending on the use cases. Aurora also offers a serverless feature that enables a completely on-demand experience where the database will scale down automatically in case of lower loads and vice-versa. In this mode, customers only need to pay for the time the database is active, but it comes at the cost of a slight delay in response to requests that comes during the time database is completely scaled down.
Amazon offers a replication feature through its multiAZ deployment strategy. This means your data is going to be replicated across multiple regions automatically and in case of a problem with your master instance, Amazon will switch to one among the replicas without affecting any loads.
Aurora architecture works on the basis of a cluster volume that manages the data for all the database instances in that particular cluster. A cluster volume spans across multiple availability zones and is effectively virtual database storage. The underlying storage volume is on top of multiple cluster nodes which are distributed across different availability zones. Separate from this, the Aurora database can also have read-replicas. Only one instance usually serves as the primary instance and it supports reads as well as writes. The rest of the instances serve as read-replicas and load balancing needs to be handled by the user. This is different from the multiAZ deployment, where instances are located across the availability zone and support automatic failover.
To know more about Amazon Aurora, visit this link.
Introduction to OLAP and OLTP
The term OLAP stands for Online Analytical Processing. OLAP analyses business data on a multidimensional level and allows for complicated computations, trend analysis, and advanced data modeling. Business Performance Management, Planning, Budgeting, Forecasting, Financial Reporting, Analysis, Simulation Models, Knowledge Discovery, and Data Warehouse Reporting are all built on top of it. End-users may utilize OLAP to do ad hoc analysis of data in many dimensions, giving them the knowledge and information they need to make better decisions.
Online Transaction Processing, or OLTP, is a form of data processing that involves completing several transactions concurrently, for example, online banking, shopping, order entry, or sending text messages. Traditionally, these transactions have been referred to as economic or financial transactions, and they are documented and secured so that an organization may access the information at any time for accounting or reporting reasons.
To know more about OLAP and OLTP, visit this link.
Simplify Data Analysis using LIKE.TG ’s No-code Data Pipeline
LIKE.TG Data helps you directly transfer data from 150+ data sources (including 30+ free sources) to Business Intelligence tools, Data Warehouses, or a destination of your choice in a completely hassle-free automated manner. LIKE.TG is fully managed and completely automates the process of not only loading data from your desired source but also enriching the data and transforming it into an analysis-ready form without having to write a single line of code. It helps transfer data from a source of your choice to a destination of your choice forfree. Its fault-tolerant architecture ensures that the data is handled in a secure, consistent manner with zero data loss.
LIKE.TG takes care of all your data preprocessing needs required to set up the integration and lets you focus on key business activities and draw a much powerful insight on how to generate more leads, retain customers, and take your business to new heights of profitability. It provides a consistent reliable solution to manage data in real-time and always have analysis-ready data in your desired destination.
Get Started with LIKE.TG for Free
Check out what makes LIKE.TG amazing:
Real-Time Data Transfer: LIKE.TG with its strong Integration with 150+ Sources (including 30+ Free Sources), allows you to transfer data quickly efficiently. This ensures efficient utilization of bandwidth on both ends.
Data Transformation: It provides a simple interface to perfect, modify, and enrich the data you want to transfer.
Secure: LIKE.TG has a fault-tolerant architecture that ensures that the data is handled in a secure, consistent manner with zero data loss.
Tremendous Connector Availability: LIKE.TG houses a large variety of connectors and lets you bring in data from numerous Marketing SaaS applications, databases, etc. such as HubSpot, Marketo, MongoDB, Oracle, Salesforce, Redshift, etc. in an integrated and analysis-ready form.
Simplicity: Using LIKE.TG is easy and intuitive, ensuring that your data is exported in just a few clicks.
Completely Managed Platform: LIKE.TG is fully managed. You need not invest time and effort to maintain or monitor the infrastructure involved in executing codes.
Live Support: The LIKE.TG team is available round the clock to extend exceptional support to its customers through chat, email, and support calls.
Sign up here for a 14-Day Free Trial!
Factors that Drive Redshift Vs Aurora Decision
Both Redshift and Aurora are popular database services in the market. There is no one-size-fits-all answer here, instead, you must choose based on your company’s needs, budget, and other factors to make a Redshift vs Aurora decision. The primary factors that influence the Redshift vs Aurora comparison are as follows:
Redshift vs Aurora: Scaling
Redshift offer scaling by adding more nodes or upgrading the nodes. Redshift scaling can be done automatically, but the downtime in the case of Redshift is more than that of Aurora. Redshift’s concurrency scaling feature deserves a mention here. This feature is priced separately and allows a virtually unlimited number of concurrent users with the same performance if the budget is not a problem.
Aurora enables scaling vertically or horizontally. Vertical scaling is through upgrading instance types and in the case of multiAZ deployment, there is minimal downtime associated with this. Otherwise, the scaling can be scheduled during the maintenance time window of the database. Aurora horizontal scaling is through read-replicas and an aurora database can have at most 15 read-replicas at the same time. Aurora compute scaling is different from storage scaling and what we mentioned above is only about compute scaling. Aurora storage scaling is done by changing the maximum allocated storage space or storage hardware type like SSD or HDD.
Download the Whitepaper on Database vs Data Warehouse
Learn how a Data Warehouse is different from a Database and which one should you prefer for your use case.
Redshift vs Aurora: Storage Capacity
Redshift can practically scale to petabytes of data and run complex queries out of them. Redshift can support up to 60 user-defined databases per cluster. Aurora, on the other hand, has a hard limit of 64 TB and the number of database instances is limited at 40.
Redshift vs Aurora: Data Loading
Redshift ETL also supports the COPY command for inserting data. It is recommended to insert data split into similar-sized chunks for better performance. In the case of data already existing in Redshift, you may need to use temporary tables since Redshift does not ensure unique key constraints. A detailed account of how to do ETL on Redshift can be found here.
Data loading in Aurora will depend on the type of instance type that is being used. In the case of MySQL compatible instances, you would need to use the mysqlimport command or LOAD DATA IN FILE command depending on whether the data is from a MySQL table or file. Aurora with Postgres can load data with the COPY command.
An alternative to this custom script-based ETL is to use a hassle-free Data Pipeline Platform like LIKE.TG which can offer a very smooth experience implementing ETL on Redshift or Aurora with support for real-time data sync, in-flight data transformations, and much more.
Redshift vs Aurora: Data Structure
Aurora follows row-oriented storage and supports the complete data types in both MySQL and Postgres instance types. Aurora is also an ACID complaint. Redshift uses a columnar storage structure and is optimized for column level processing than complete row level processing.
Redshift’s Postgres-like querying layer misses out on many data types which are supported by Aurora’s Postgres instance type. Redshift does not support consistency among the ACID properties and only exhibits eventual consistency. It does not ensure referential integrity and unique key constraints.
Redshift vs Aurora: Performance
Redshift offers fast read performance and over a larger amount of data when compared to Aurora. Redshift excels specifically in the case of complicated queries spanning millions of rows.
Aurora offers better performance than a traditional MySQL or Postgres instance. Aurora’s architecture disables the InnoDB change buffer for distributed storage leading to poor performance in the case of write-heavy operations. If your use case updates are heavy, it may be sensible to use traditional databases like MySQL or Postgres than Aurora.
Both the services offer performance optimizations using sharding and key distribution mechanisms. Redshift’s SORT KEY and DIST KEY need to be configured here for improvements in complex queries involving JOINs.
Aurora is optimized for OLTP workloads and Redshift is preferred in the case of OLAP workloads. Transactional workloads are not recommended in Redshift since it supports only eventual consistency.
Redshift vs Aurora: Security
When it comes to Security, there is nothing much to differentiate between the two services. With both being part of the AWS portfolio, they offer the complete set of security requirements and compliance. Data is ensured to be encrypted at rest and motion. There are provisions to establish virtual private clouds and restrict usage based on Amazon’s Identity and Access management. Other than these, customers can also use the specific security features that are part of Postgres and MySQL instance types with Aurora.
Redshift vs Aurora: Maintenance
Both Aurora and Redshift are completely managed services and required very little maintenance. Redshift because of its delete marker-based architecture needs the VACUUM command to be executed periodically to reclaim the space after entries are deleted. These can be scheduled periodically, but it is a recommended practice to execute this command in case of heavy updates and delete workload. Redshift also needs the ANALYZE command to be executed periodically to keep the metadata up to data for query planning.
Redshift vs Aurora: Pricing
Redshift pricing is including storage and compute power. Redshift starts at .25$ per hour for the dense compute instance types per node. Dense compute is the recommended instance type for up to 500 GB of data. For the higher-spec dense storage instance types, pricing starts at .85$. It is to be noted that these two services are designed for different use cases and pricing can not be compared independent of the customer use cases.
Aurora MySQL starts with .041$ per hour for its lowest spec instance type. Aurora Postgres starts at .082$ per hour for the same type of instance. The memory-optimized instance types with higher performance start for .29$ for both MySQL and Postgres instance types. Aurora’s serverless instances are charged based on ACU hours and start at .06$ per ACU hour. Storage and IO are charged separately for Aurora. It costs .1 $ per GB per month and .2$ per 1 million requests. Aurora storage pricing is based on the maximum storage ever used by the cluster and it is not possible to reclaim space after being deleted without re instantiating the database.
An obvious question after such a long comparison is about how to decide when to use Redshift and Aurora for your requirement. The following section summarizes the scenarios in which using one of them may be beneficial over the other.
Redshift vs Aurora: Use Cases
Use Cases of Amazon Redshift
The requirement is an Online analytical processing workload and not transactional.
You have a high analytical workload and running on your transactional database will hurt the performance.
Your data volume is in hundreds of TBs and you anticipate more data coming in.
You are willing to let go of the consistency compliance and will ensure the uniqueness of your keys on your own.
You are ready to put your head into designing SORT KEYS and DIST KEYS to extract the maximum performance.
Use Cases of Amazon Aurora
You want to relieve yourself of the administrative tasks of managing a database but want to stick with MySQL or Postgres compatible querying layer.
You want to stay with traditional databases like MySQL or Postgres but want better read performance at the cost of slightly lower write and update performance.
Your storage requirements are only in the TBs and do not anticipate 100s of TBs of data in the near future.
You have an online transactional processing use case and want quick results with a smaller amount of data.
Your OLTP workloads are not interrupted by analytical workloads
Your analytical workloads do not need to process millions of rows of data.
Conclusion
This article gave a comprehensive guide on difference between Aurora vs Redshift. You got a deeper understanding of Redshift and Aurora. Now, you are in the position to choose the best among the two based on your business goals and requirements. To conclude, the Redshift vs Aurora decision is entirely based on the company’s goals, resources, and also a matter of personal preference.
Visit our Website to Explore LIKE.TG
Businesses can use automated platforms like LIKE.TG Data to set the integration and handle the ETL process. It helps you directly transfer data from a source of your choice to a Data Warehouse, Business Intelligence tools, or any other desired destination in a fully automated and secure manner without having to write any code and will provide you a hassle-free experience. It helps transfer data from a source of your choice to a destination of your choice forfree.
Want to take LIKE.TG for a spin? Sign Up for a 14-day free trial and experience the feature-rich LIKE.TG suite first hand. You can also have a look at the unbeatable LIKE.TG Pricing that will help you choose the right plan for your business needs.
What use case are you evaluating these platforms for? Let us know in the comments. We would be happy to help solve your dilemma.
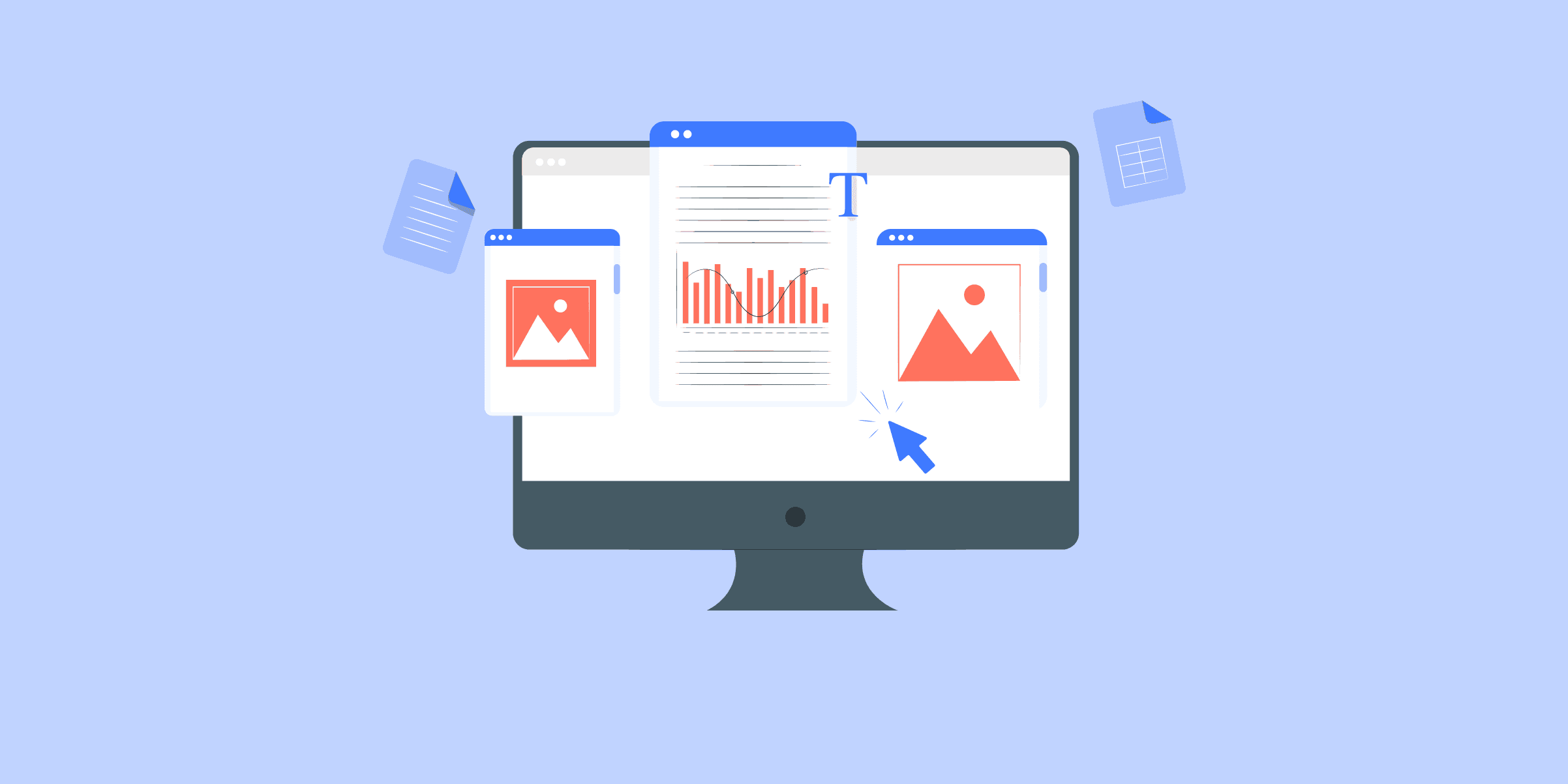
MS SQL Server to Redshift: 3 Easy Methods
With growing volumes of data, is your SQL Server getting slow for analytical queries? Are you simply migrating data from MS SQL Server to Redshift? Whatever your use case, we appreciate your smart move to transfer data from MS SQL Server to Redshift. This article, in detail, covers the various approaches you could use to load data from SQL Server to Redshift.
This article covers the steps involved in writing custom code to load data from SQL Server to Amazon Redshift. Towards the end, the blog also covers the limitations of this approach.
Note: For MS SQL to Redshift migrations, compatibility and performance optimization for the transferred SQL Server workloads must be ensured.
What is MS SQL Server?
Microsoft SQL Server is a relational database management system (RDBMS) developed by Microsoft. It is designed to store and retrieve data as requested by other software applications, which can run on the same computer or connect to the database server over a network.
Some key features of MS SQL Server:
It is primarily used for online transaction processing (OLTP) workloads, which involve frequent database updates and queries.
It supports a variety of programming languages, including T-SQL (Transact-SQL), .NET languages, Python, R, and more.
It provides features for data warehousing, business intelligence, analytics, and reporting through tools like SQL Server Analysis Services (SSAS), SQL Server Integration Services (SSIS), and SQL Server Reporting Services (SSRS).
It offers high availability and disaster recovery features like failover clustering, database mirroring, and log shipping.
It supports a wide range of data types, including XML, spatial data, and in-memory tables.
What is Amazon Redshift?
Amazon Redshift is a cloud-based data warehouse service offered by Amazon Web Services (AWS). It’s designed to handle massive amounts of data, allowing you to analyze and gain insights from it efficiently. Here’s a breakdown of its key features:
Scalability:Redshift can store petabytes of data and scale to meet your needs.
Performance:It uses a parallel processing architecture to analyze large datasets quickly.
Cost-effective:Redshift offers pay-as-you-go pricing, so you only pay for what you use.
Security:Built-in security features keep your data safe.
Ease of use:A fully managed service, Redshift requires minimal configuration.
Understanding the Methods to Connect SQL Server to Redshift
A good understanding of the different Methods to Migrate SQL Server To Redshift can help you make an informed decision on the suitable choice.
These are the three methods you can implement to set up a connection from SQL Server to Redshift in a seamless fashion:
Method 1: Using LIKE.TG Data to Connect SQL Server to Redshift
Method 2: Using Custom ETL Scripts to Connect SQL Server to Redshift
Method 3: Using AWS Database Migration Service (DMS) to Connect SQL Server to Redshift
Method 1: Using LIKE.TG Data to Connect SQL Server to Redshift
LIKE.TG helps you directly transfer data from SQL Server and various other sources to a Data Warehouse, such as Redshift, or a destination of your choice in a completely hassle-free automated manner. LIKE.TG is fully managed and completely automates the process of not only loading data from your desired source but also enriching the data and transforming it into an analysis-ready form without having to write a single line of code. Its fault-tolerant architecture ensures that the data is handled securely and consistently with zero data loss.
Sign up here for a 14-Day Free Trial!
LIKE.TG takes care of all your data preprocessing to set up SQL Server Redshift migration and lets you focus on key business activities and draw a much more powerful insight on how to generate more leads, retain customers, and take your business to new heights of profitability. It provides a consistent reliable solution to manage data in real-time and always have analysis-ready data in your desired destination.
Step 1: Configure MS SQL Server as your Source
ClickPIPELINESin theNavigation Bar.
Click+ CREATEin thePipelines List View.
In theSelect Source Typepage, select theSQLServer variant
In theConfigure yourSQL Server Sourcepage, specify the following:
Step 2: Select the Replication Mode
Select the replication mode: (a) Full Dump and Load (b) Incremental load for append-only data (c) Incremental load for mutable data.
Step 3: Integrate Data into Redshift
ClickDESTINATIONSin theNavigation Bar.
Click+ CREATEin theDestinations List View.
In theAdd Destinationpage, selectAmazonRedshift.
In theConfigure your AmazonRedshift Destinationpage, specify the following:
As can be seen, you are simply required to enter the corresponding credentials to implement this fully automated data pipeline without using any code.
Check out what makes LIKE.TG amazing:
Real-Time Data Transfer: LIKE.TG with its strong Integration with 100+ sources, allows you to transfer data quickly efficiently. This ensures efficient utilization of bandwidth on both ends.
Data Transformation: It provides a simple interface to perfect, modify, and enrich the data you want to transfer.
Secure: LIKE.TG has a fault-tolerant architecture that ensures that the data is handled securely and consistently with zero data loss.
Tremendous Connector Availability: LIKE.TG houses a large variety of connectors and lets you bring in data from numerous Marketing SaaS applications, databases, etc. such as Google Analytics 4, Google Firebase, Airflow, HubSpot, Marketo, MongoDB, Oracle, Salesforce, Redshift, etc. in an integrated and analysis-ready form.
Simplicity: Using LIKE.TG is easy and intuitive, ensuring that your data is exported in just a few clicks.
Completely Managed Platform: LIKE.TG is fully managed. You need not invest time and effort to maintain or monitor the infrastructure involved in executing codes.
Get Started with LIKE.TG for Free
Method 2: Using Custom ETL Scripts to Connect SQL Server to Redshift
As a pre-requisite to this process, you will need to have installed Microsoft BCP command-line utility. If you have not installed it, here is the link to download it.
For demonstration, let us assume that we need to move the ‘orders’ table from the ‘sales’ schema into Redshift. This table is populated with the customer orders that are placed daily.
There might be two cases you will consider while transferring data.
Move data for one time into Redshift.
Incrementally load data into Redshift. (when the data volume is high)
Let us look at both scenarios:
One Time Load
You will need to generate the .txt file of the required SQL server table using the BCP command as follows :
Open the command prompt and go to the below path to run the BCP command
C:Program Files <x86>Microsoft SQL ServerClient SDKODBC130ToolsBinn
Run BCP command to generate the output file of the SQL server table Sales
bcp "sales.orders" out D:outorders.txt -S "ServerName" -d Demo -U UserName -P Password -c
Note: There might be several transformations required before you load this data into Redshift. Achieving this using code will become extremely hard. A tool like LIKE.TG , which provides an easy environment to write transformations, might be the right thing for you. Here are the steps you can use in this step:
Step 1: Upload Generated Text File to S3 Bucket
Step 2: Create Table Schema
Step 3: Load the Data from S3 to Redshift Using the Copy Command
Step 1: Upload Generated Text File to S3 Bucket
We can upload files from local machines to AWS using several ways. One simple way is to upload it using the file upload utility of S3. This is a more intuitive alternative.You can also achieve this AWS CLI, which provides easy commands to upload it to the S3 bucket from the local machine.As a pre-requisite, you will need to install and configure AWS CLI if you have not already installed and configured it. You can refer to the user guide to know more about installing AWS CLI.Run the following command to upload the file into S3 from the local machine
aws s3 cp D:orders.txt s3://s3bucket011/orders.txt
Step 2: Create Table Schema
CREATE TABLE sales.orders (order_id INT,
customer_id INT,
order_status int,
order_date DATE,
required_date DATE,
shipped_date DATE,
store_id INT,
staff_id INT
)
After running the above query, a table structure will be created within Redshift with no records in it. To check this, run the following query:
Select * from sales.orders
Step 3: Load the Data from S3 to Redshift Using the Copy Command
COPY dev.sales.orders FROM 's3://s3bucket011/orders.txt'
iam_role 'Role_ARN' delimiter 't';
You will need to confirm if the data has loaded successfully. You can do that by running the query.
Select count(*) from sales.orders
This should return the total number of records inserted.
Limitations of using Custom ETL Scripts to Connect SQL Server to Redshift
In cases where data needs to be moved once or in batches only, the custom ETL script method works well. This approach becomes extremely tedious if you have to copy data from MS SQL to Redshift in real-time.
In case you are dealing with huge amounts of data, you will need to perform incremental load. Incremental load (change data capture) becomes hard as there are additional steps that you need to follow to achieve it.
Transforming data before you load it into Redshift will be extremely hard to achieve.
When you write code to extract a subset of data often those scripts break as the source schema keeps changing or evolving. This can result in data loss.
The process mentioned above is frail, erroneous, and often hard to implement and maintain. This will impact the consistency and availability of your data into Amazon Redshift.
Download the Cheatsheet on How to Set Up High-performance ETL to Redshift
Learn the best practices and considerations for setting up high-performance ETL to Redshift
Method 3: Using AWS Database Migration Service (DMS)
AWS Database Migration Service (DMS) offers a seamless pathway for transferring data between databases, making it an ideal choice for moving data from SQL Server to Redshift. This fully managed service is designed to minimize downtime and can handle large-scale migrations with ease.
For those looking to implement SQL Server CDC (Change Data Capture) for real-time data replication, we provide a comprehensive guide that delves into the specifics of setting up and managing CDC within the context of AWS DMS migrations.
Detailed Steps for Migration:
Setting Up a Replication Instance: The first step involves creating a replication instance within AWS DMS. This instance acts as the intermediary, facilitating the transfer of data by reading from SQL Server, transforming the data as needed, and loading it into Redshift.
Creating Source and Target Endpoints: After the replication instance is operational, you’ll need to define the source and target endpoints. These endpoints act as the connection points for your SQL Server source database and your Redshift target database.
Configuring Replication Settings: AWS DMS offers a variety of settings to customize the replication process. These settings are crucial for tailoring the migration to fit the unique needs of your databases and ensuring a smooth transition.
Initiating the Replication Process: With the replication instance and endpoints in place, and settings configured, you can begin the replication process. AWS DMS will start the data transfer, moving your information from SQL Server to Redshift.
Monitoring the Migration: It’s essential to keep an eye on the migration as it progresses. AWS DMS provides tools like CloudWatch logs and metrics to help you track the process and address any issues promptly.
Verifying Data Integrity: Once the migration concludes, it’s important to verify the integrity of the data. Conducting thorough testing ensures that all data has been transferred correctly and is functioning as expected within Redshift.
The duration of the migration is dependent on the size of the dataset but is generally completed within a few hours to days. The sql server to redshift migration process is often facilitated by AWS DMS, which simplifies the transfer of database objects and data
For a step-by-step guide, please refer to the official AWS documentation.
Limitations of Using DMS:
Not all SQL Server features are supported by DMS. Notably, features like SQL Server Agent jobs, CDC, FILESTREAM, and Full-Text Search are not available when using this service.
The initial setup and configuration of DMS can be complex, especially for migrations that involve multiple source and target endpoints.
Conclusion
That’s it! You are all set. LIKE.TG will take care of fetching your data incrementally and will upload that seamlessly from MS SQL Server to Redshift via a real-time data pipeline.
Extracting complex data from a diverse set of data sources can be a challenging task and this is where LIKE.TG saves the day!
Visit our Website to Explore LIKE.TG
LIKE.TG offers a faster way to move data from Databases or SaaS applications like SQL Server into your Data Warehouse like Redshift to be visualized in a BI tool. LIKE.TG is fully automated and hence does not require you to code.
Sign Up for a 14-day free trial to try LIKE.TG for free. You can also have a look at the unbeatable pricing that will help you choose the right plan for your business needs.
Tell us in the comments about data migration from SQL Server to Redshift!
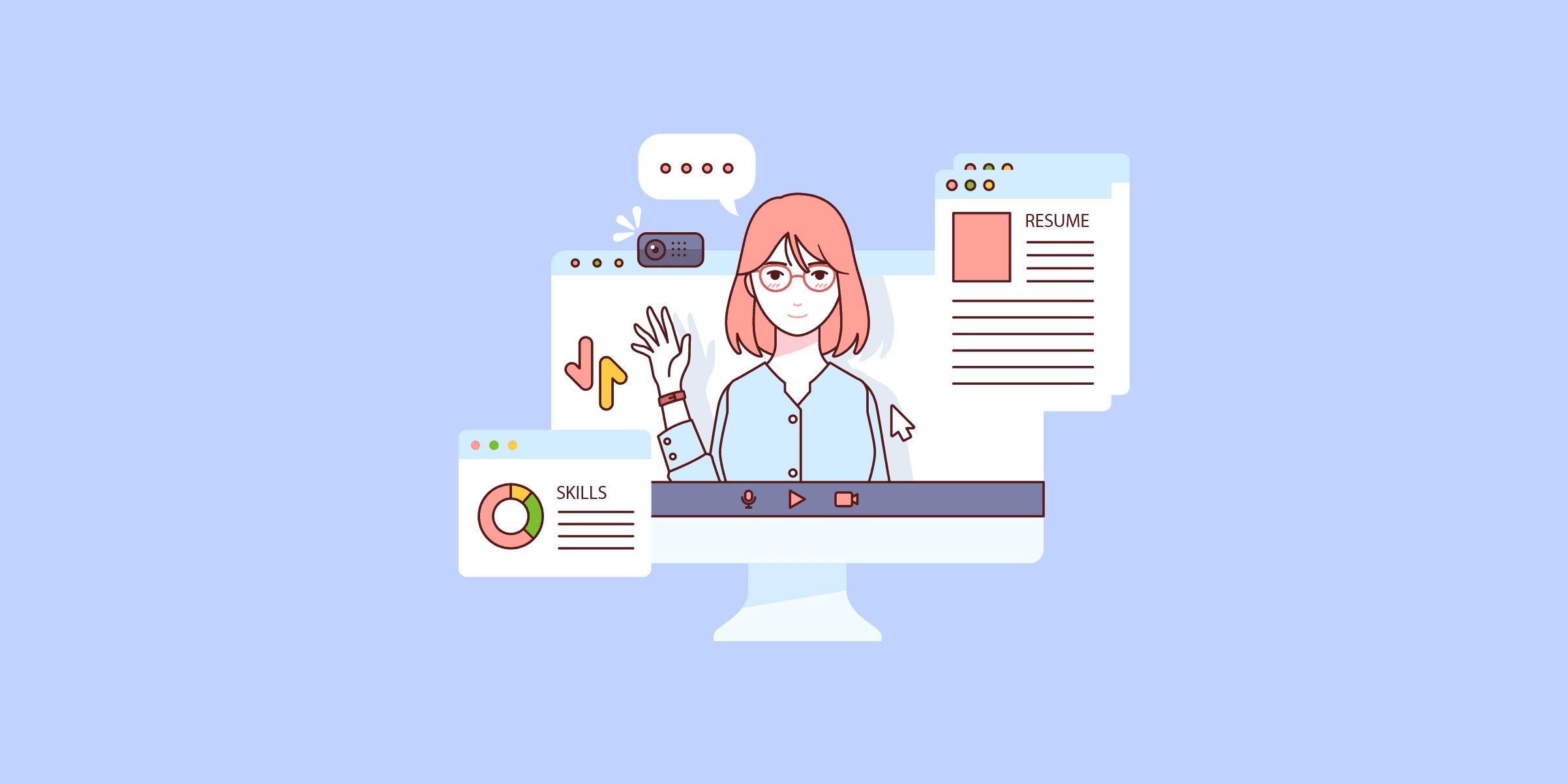
Snowflake Architecture & Concepts: A Comprehensive Guide
This article helps focuses on an in-depth understanding of Snowflake architecture, how it stores and manages data, and its micro-partitioning concepts. By the end of this blog, you will also be able to understand how Snowflake architecture is different from the rest of the cloud-based Massively Parallel Processing Databases.What is a Data Warehouse?
Businesses today are overflowing with data. The amount of data produced every day is truly staggering. With Data Explosion, it has become seemingly difficult to capture, process, and store big or complex datasets. Hence, it becomes a necessity for organizations to have a Central Repository where all the data is stored securely and can be further analyzed to make informed decisions. This is where Data Warehouses come into the picture.
A Data Warehouse also referred to as “Single Source of Truth”, is a Central Repository of information that supports Data Analytics and Business Intelligence (BI) activities. Data Warehouses store large amounts of data from multiple sources in a single place and are intended to execute queries and perform analysis for optimizing their business. Its analytical capabilities allow organizations to derive valuable business insights from their data to improve decision-making.
What is the Snowflake Data Warehouse?
Snowflake is a cloud-based Data Warehouse solution provided as a Saas (Software-as-a-Service) with full support for ANSI SQL. It also has a unique architecture that enables users to just create tables and start querying data with very less administration or DBA activities needed. Know about Snowflake pricing here.
Download the Cheatsheet on How to Set Up ETL to Snowflake
Learn the best practices and considerations for setting up high-performance ETL to Snowflake
Features of Snowflake Data Warehouse
Let’s discuss some major features of Snowflake data warehouse:
Security and Data Protection: Snowflake data warehouse offers enhanced authentication by providing Multi-Factor Authentication (MFA), federal authentication and Single Sign-on (SSO) and OAuth. All the communication between the client and server is protected y TLS.
Standard and Extended SQL Support: Snowflake data warehouse supports most DDL and DML commands of SQL. It also supports advanced DML, transactions, lateral views, stored procedures, etc.
Connectivity: Snowflake data warehouse supports an extensive set of client connectors and drivers such as Python connector, Spark connector, Node.js driver, .NET driver, etc.
Data Sharing: You can securely share your data with other Snowflake accounts.
Read more about the features of Snowflake data warehouse here. Let’s learn about Snowflake architecture in detail.
LIKE.TG Data: A Convenient Method to Explore your Snowflake Data
LIKE.TG is a No-code Data Pipeline. It supports pre-built data integration from100+ data sourcesat a reasonableprice. It can automate your entire data migration process in minutes. It offers a set of features and supports compatibility with several databases and data warehouses.
Get Started with LIKE.TG for Free
Let’s see some unbeatable features of LIKE.TG :
Simple:LIKE.TG has a simple and intuitive user interface.
Fault-Tolerant:LIKE.TG offers a fault-tolerant architecture. It can automatically detect anomalies and notifies you instantly. If there is any affected record, then it is set aside for correction.
Real-Time:LIKE.TG has a real-time streaming structure, which ensures that your data is always ready for analysis.
Schema Mapping:LIKE.TG will automatically detect schema from your incoming data and maps it to your destination schema.
Data Transformation:It provides a simple interface to perfect, modify, and enrich the data you want to transfer.
Live Support:LIKE.TG team is available round the clock to extend exceptional support to you through chat, email, and support call.
Sign up here for a 14-Day Free Trial!
Types of Data Warehouse Architecture
There are mainly 3 ways of developing a Data Warehouse:
Single-tier Architecture: This type of architecture aims to deduplicate data in order to minimize the amount of stored data.
Two-tier Architecture: This type of architecture aims to separate physical Data Sources from the Data Warehouse. This makes the Data Warehouse incapable of expanding and supporting multiple end-users.
Three-tier Architecture: This type of architecture has 3 tiers in it. The bottom tier consists of the Database of the Data Warehouse Servers, the middle tier is an Online Analytical Processing (OLAP) Server used to provide an abstracted view of the Database, and finally, the top tier is a Front-end Client Layer consisting of the tools and APIs used for extracting data.
Components of Data Warehouse Architecture
The 4 components of a Data Warehouse are as follows.
1. Data Warehouse Database
A Database forms an essential component ofa Data Warehouse. A Database stores and provides access to company data. Amazon Redshift and Azure SQL come under Cloud-based Database services.
2. Extraction, Transformation, and Loading Tools (ETL)
All the operations associated with the Extraction, Transformation, and Loading (ETL) of data into the warehouse come under this component. Traditional ETL tools are used to extract data from multiple sources, transform it into a digestible format, and finally load it into a Data Warehouse.
3. Metadata
Metadata provides a framework and descriptions of data, enabling the construction, storage, handling, and use of the data.
4. Data Warehouse Access Tools
Access Tools allow users to access actionable and business-ready information froma Data Warehouse. TheseWarehouse Toolsinclude Data Reporting tools, Data Querying Tools, Application Development tools, Data Mining tools, and OLAP tools.
Snowflake Architecture
Snowflake architecture comprises a hybrid of traditional shared-disk and shared-nothing architectures to offer the best of both. Let us walk through these architectures and see how Snowflake combines them into new hybrid architecture.
Overview of Shared-Disk Architecture
Overview of Shared-Nothing Architecture
Snowflake Architecture – A Hybrid Model
Storage Layer
Compute Layer
Cloud Services Layer
Overview of Shared-Disk Architecture
Used in traditional databases, shared-disk architecture has one storage layer accessible by all cluster nodes. Multiple cluster nodes having CPU and Memory with no disk storage for themselves communicate with central storage layer to get the data and process it.
Overview of Shared-Nothing Architecture
Contrary to Shared-Disk architecture, Shared-Nothing architecture has distributed cluster nodes along with disk storage, their own CPU, and Memory. The advantage here is that the data can be partitioned and stored across these cluster nodes as each cluster node has its own disk storage.
Snowflake Architecture – A Hybrid Model
Snowflake supports a high-level architecture as depicted in the diagram below. Snowflake has 3 different layers:
Storage Layer
Compute Layer
Cloud Services Layer
1. Storage Layer
Snowflake organizes the data into multiple micro partitions that are internally optimized and compressed. It uses a columnar format to store. Data is stored in the cloud storage and works as a shared-disk model thereby providing simplicity in data management. This makes sure users do not have to worry about data distribution across multiple nodes in the shared-nothing model.
Compute nodes connect with storage layer to fetch the data for query processing. As the storage layer is independent, we only pay for the average monthly storage used. Since Snowflake is provisioned on the Cloud, storage is elastic and is charged as per the usage per TB every month.
2. Compute Layer
Snowflake uses “Virtual Warehouse” (explained below) for running queries. Snowflake separates the query processing layer from the disk storage. Queries execute in this layer using the data from the storage layer.
Virtual Warehouses are MPP compute clusters consisting of multiple nodes with CPU and Memory provisioned on the cloud by Snowflake. Multiple Virtual Warehouses can be created in Snowflake for various requirements depending upon workloads. Each virtual warehouse can work with one storage layer. Generally, a virtual Warehouse has its own independent compute cluster and doesn’t interact with other virtual warehouses.
Advantages of Virtual Warehouse
Some of the advantages of virtual warehouse are listed below:
Virtual Warehouses can be started or stopped at any time and also can be scaled at any time without impacting queries that are running.
They also can be set to auto-suspend or auto-resume so that warehouses are suspended after a specific period of inactive time and then when a query is submitted are resumed.
They can also be set to auto-scale with minimum and maximum cluster size, so for e.g. we can set minimum 1 and maximum 3 so that depending on the load Snowflake can provision between 1 to 3 multi-cluster warehouses.
3. Cloud Services Layer
All the activities such as authentication, security, metadata management of the loaded data and query optimizer that coordinate across Snowflake happens in this layer.
Examples of services handled in this layer:
When a login request is placed it has to go through this layer,
Query submitted to Snowflake will be sent to the optimizer in this layer and then forwarded to Compute Layer for query processing.
Metadata required to optimize a query or to filter a data are stored in this layer.
These three layers scale independently and Snowflake charges for storage and virtual warehouse separately. Services layer is handled within compute nodes provisioned, and hence not charged.
The advantage of this Snowflake architecture is that we can scale any one layer independently of others. For e.g. you can scale storage layer elastically and will be charged for storage separately. Multiple virtual warehouses can be provisioned and scaled when additional resources are required for faster query processing and to optimize performance. Know more about Snowflake architecture from here.
Connecting to Snowflake
Now that you’re familiar with Snowflake’s architecture, it’s now time to discuss how you can connect to Snowflake. Let’s take a look at some of the best third-party tools and technologies that form the extended ecosystem for connecting to Snowflake.
Snowflake Ecosystem— This list will take you through Snowflake’s partners, clients, third-party tools, and emerging technologies in the Snowflake ecosystem.
Third-party partners and technologies are certified to provide native connectivity to Snowflake.
Data Integration or ETL tools are known to provide native connectivity to Snowflake.
Business intelligence (BI) tools simplify analyzing, discovering, and reporting on business data to help organizations make informed business decisions.
Machine Learning Data Science cover a broad category of vendors, tools, and technologies that extend Snowflake’s functionality to provide advanced capabilities for statistical and predictive modeling.
Security Governance tools ensure that your data is stored and maintained securely.
Snowflake also provides native SQL Development and Data Querying interfaces.
Snowflake supports developing applications using many popular programming languages and development platforms.
Snowflake Partner Connect— This list will take you through Snowflake partners who offer free trials for connecting to Snowflake.
General Configuration (All Clients)— This is a set of general configuration instructions that is applicable to all Snowflake-provided Clients (CLI, connectors, and drivers).
SnowSQL (CLI Client)— SnowSQL is a next-generation command-line utility for connecting to Snowflake. It allows you to execute SQL queries and perform all DDL and DML operations.
Connectors Drivers– Snowflake provides drivers and connectors for Python, JDBC, Spark, ODBC, and other clients for application development. You can go through each of them listed below to start learning and using them.
Snowflake Connector for Python
Snowflake Connector for Spark
Snowflake Connector for Kafka
Node.js Driver
Go Snowflake Driver
.NET Driver
JDBC Driver
ODBC Driver
PHP PDO Driver for Snowflake
You can always connect to Snowflake via the above-mentioned tools/technologies.
Conclusion
Ever since 2014, Snowflake has been simplifying how organizations store and interact with their data. In this blog, you have learned about Snowflake’s data warehouse, Snowflake architecture, and how it stores and manages data. You learned about various layers of the hybrid model in Snowflake architecture. Check out more articles about the Snowflake data warehouse to know about vital Snowflake data warehouse featuresand Snowflake best practices for ETL. You can have a good working knowledge of Snowflake by understandingSnowflake Create Table.
Visit our Website to Explore LIKE.TG
LIKE.TG , an official Snowflake ETL Partner, can help bring your data from various sources to Snowflake in real-time. You canreach out to us or take up a free trial if you need help in setting up your Snowflake Architecture or connecting your data sources to Snowflake.
Give LIKE.TG a try! Sign Up here for a 14-day free trial today.
If you still have any queries related to Snowflake Architecture, feel free to discuss them in the comment section below.