数据 API
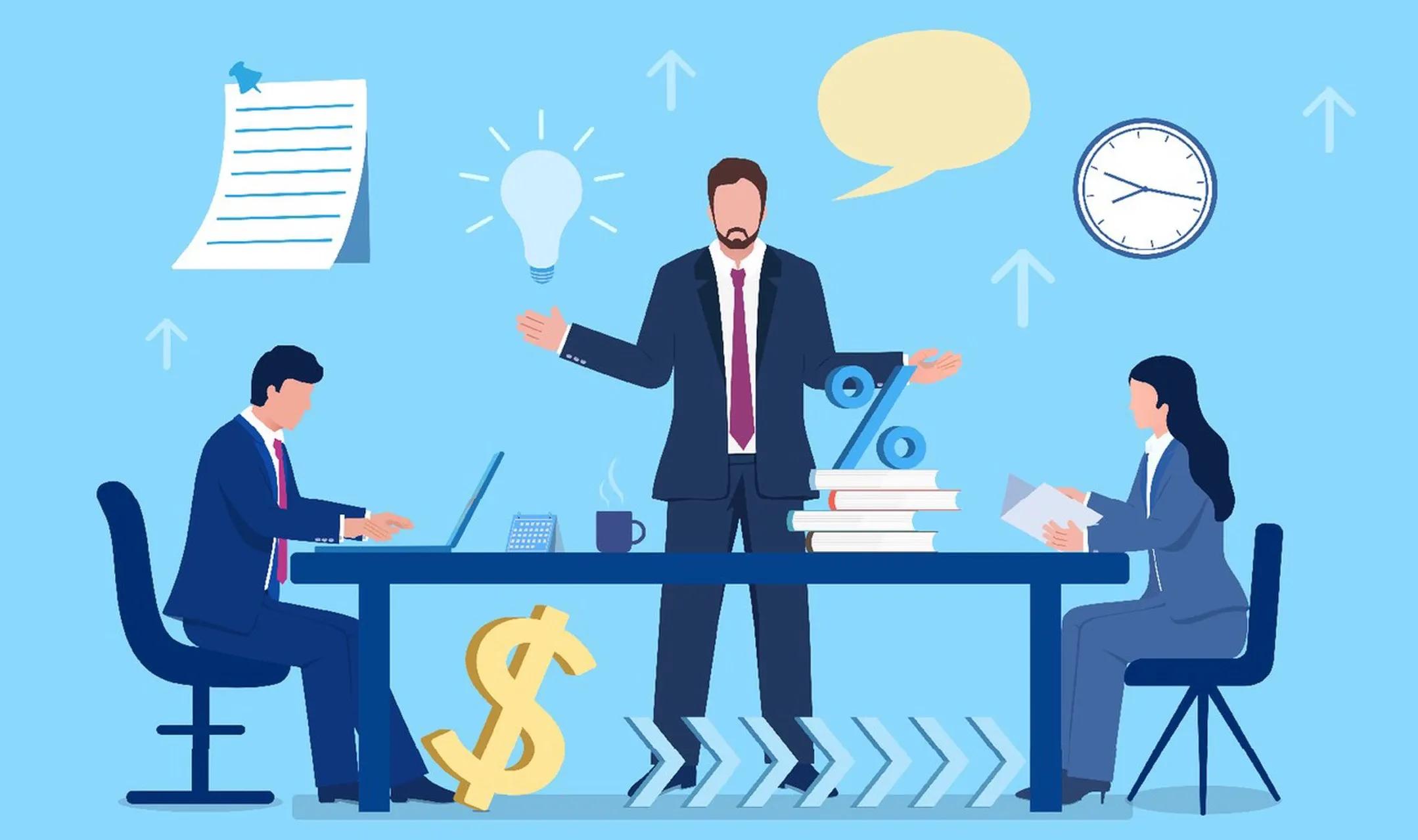
PDF Parsing: Automate Data Extraction from PDF Files & Forms
PDFs have rapidly become a preferred format for sharing and distributing information, favored for their readability. However, the lack of a standardized data structure can present challenges for data extraction. An effective solution to this challenge is PDF parsing, which automates the extraction process, significantly eliminating the need for manual effort and streamlining data extraction. What is PDF Parsing? PDF parsing, also known as PDF scraping or PDF data extraction, is the process of extracting unstructured data from PDF files and transforming the information into a format that can be easily processed and analyzed. Designed for fixed-layout documents, PDFs can store various data types and are found in different types such as searchable PDFs, scanned PDFs and fillable PDFs. Parsing these files is essential for unlocking the hidden insights within the documents. PDF parsing is an indispensable technique for automating data extraction since it enables businesses to process large volumes of business documents efficiently — without requiring manual intervention. By automating PDF extraction processes, companies can streamline document processing, saving significant time and resources and enabling faster reporting and analytics. PDF Parsing Use Cases Here are some common use cases for PDF parsing: Insurance Claims Processing In the insurance sector, claim forms are submitted by customers, often in PDF format. These forms contain vital information such as customer details, address, claim amount, policy type, and policy number. Manually transcribing this information, especially with a high volume of forms, is time-consuming and prone to errors. Processing these claims swiftly is essential for customer satisfaction and operational efficiency. PDF parsing accomplishes this by automating the entire process, ensuring accuracy and efficiency. Patient Records PDF parsing facilitates the extraction of patient details, diagnoses, and treatment information. This data can be analyzed for research purposes, integrated with other systems, or utilized to streamline medical workflows. Employee Onboarding PDF parsing captures and extracts data from onboarding documents, making the employee onboarding process more efficient. This automation ensures accurate and streamlined data entry, allowing HR teams to focus on providing a smooth onboarding experience for new hires. Invoice Data Extraction Businesses receive a high volume of invoices on a daily basis, often in the form of PDFs. Extracting data from these invoices poses a significant challenge due to their unstructured format. Invoice data capture is crucial for businesses to analyze spending patterns, identify cost-saving opportunities, and generate accurate financial reports. Additionally, businesses can integrate this extracted data into accounting systems or leverage it for advanced analytics. Common PDF Parsing Challenges While PDF parsing is immensely beneficial, it comes with its own set of challenges. Many organizations face difficulties in ingesting data from PDF files, often resorting to manual data entry as the default solution, which can be inefficient and resource-intensive. Also, managing the substantial volume of PDF files processed daily demands a sizable team dedicated to continuous data re-entry. An alternative approach is developing in-house software and coding solutions. While this approach has potential, it introduces its own set of challenges such as capturing data from scanned PDFs, accommodating diverse formats, and transforming the data into a structure compatible with the storage system. Additionally, the variability in the structure of PDFs, such as different layouts and fonts, poses a challenge for creating a one-size-fits-all parsing solution. Encryption and password protection further complicate the process, requiring decryption before parsing and necessitating secure handling of passwords. Addressing these challenges is crucial for developing effective and efficient PDF parsing solutions in enterprise settings. The Need for Automation in PDF Data Extraction Instead of manually inputting data or creating a tool from the ground up, we recommend opting for an enterprise-level PDF parsing solution to automate the process. Research shows that organizations employing Intelligent Automation achieve cost savings ranging from 40 to 75 percent. Therefore, investing in automated PDF parsing tools is wise, as it can offer businesses a competitive advantage over depending on manual procedures. Benefits of Using an Automated PDF Parsing Solution Time and Effort Reduction: Eliminating manual intervention streamlines extraction workflows, ensuring that tasks are performed efficiently and accurately. This also saves valuable time for employees. Accuracy and Consistency: Employing sophisticated algorithms and machine learning minimizes the risk of human error, resulting in a more dependable dataset for analysis and decision-making. Employee Productivity and Satisfaction: Automation technology frees employees from the burden of tedious manual tasks involved in copying and pasting data from PDFs. This pivots focus to more strategic and value-added responsibilities. Scalability: Whether dealing with a few hundred or several thousand documents, automation technology can efficiently handle varying volumes of PDFs. This scalability is particularly advantageous for organizations dealing with large amounts of unstructured data, such as financial institutions, healthcare providers, and government agencies. How to Choose the Right PDF Parser? When choosing a PDF parser, it is crucial to consider the following aspects: Accuracy and Reliability Pick a solution with high accuracy for extracting data from PDFs. The parser should handle diverse PDF layouts, fonts, and structures to ensure reliable extraction results. Template-based PDF extraction ensures 100% accuracy when configured correctly, while template-less extraction tools can be inaccurate if models are not trained correctly. Flexibility and Customization Evaluate the parser’s ability to adapt to specific data extraction needs through customization and configuration. Look for features that enable the definition of extraction rules, patterns, or templates for consistent data extraction. Versatility in handling different content, is also essential. Automation and Scalability Assess the level of automation provided by the parser, ensuring it supports batch processing for extracting data from multiple PDF files simultaneously and in real-time (as soon as new PDFs are introduced in the system). Integration with other systems or automation capabilities including workflow orchestration and scheduling should be considered for streamlining the data extraction process. Integration and Output Formats Check whether the parser supports exporting extracted data in various formats like CSV, Excel, JSON, or databases for further processing and integration. Consider cloud applications being used by the organization via their APIs for seamless data integration. Support and Updates Ensure the parser offers reliable technical support and regular updates to address any issues promptly. Regular updates keep the parser compatible with the latest PDF standards and technologies. User-Friendly Interface Look for a parser with a user-friendly interface to simplify configuration, monitoring, and management of PDF extraction tasks. A well-designed interface can significantly enhance the overall user experience. Find the Perfect PDF Parser with LIKE.TG ReportMiner Discover why LIKE.TG ReportMiner is the ultimate PDF parser for your needs. Book a session with our experts to learn how LIKE.TG ReportMiner can transform your data extraction process and become your go-to solution for PDF parsing. Schedule a Demo LIKE.TG ReportMiner for PDF Parsing LIKE.TG ReportMiner is an advanced PDF parsing solution utilizing artificial intelligence for automated data extraction from PDF files. Specifically designed for PDF documents with diverse layouts, the solution streamlines the extraction process and efficiently loads data into databases or Excel files. LIKE.TG’s user-friendly and no-code interface simplifies PDF data extraction, minimizing manual efforts and accelerating the overall extraction process. Key Features of LIKE.TG ReportMiner: Intelligent Data Extraction: LIKE.TG’s AI-powered engine efficiently extracts data from various templates by identifying the desired fields. It adeptly manages variations across different templates, ensuring quick and accurate extraction. Data Transformation: LIKE.TG transforms extracted data into the desired format, facilitating filtering, validating, cleansing, or reformatting according to specific requirements. Batch Processing: With support for batch processing, the tool enables simultaneous extraction of data from multiple PDF documents for efficient and scheduled processing. Real-Time Processing: LIKE.TG’s File Drop feature in Scheduler processes extracts information from a file as soon as it appears in a folder, enabling real-time processing. Integration with External Systems: LIKE.TG ReportMiner seamlessly integrates with external systems or databases, facilitating direct loading of extracted data into preferred destinations. Error Handling and Logging: Powered by robust error handling mechanisms, ReportMiner manages exceptions during the extraction process. The tool also provides logging capabilities to capture and track any errors or issues encountered, ensuring a smooth extraction experience. Experience Seamless PDF Data Extraction with LIKE.TG ReportMiner Unlock the potential of AI-driven PDF data extraction with LIKE.TG ReportMiner. Simplify your processes, ensure data accuracy, and save valuable time. Try LIKE.TG ReportMiner free for 14 days and witness the transformation in your data workflows. Start Your Free 14-Day Trial Enhance your PDF data extraction experience with LIKE.TG. Explore our solution with a free trial or view a product demo to experience the potential of AI-driven PDF data extraction today!

Automated Credit Risk Assessment: How ETL Is Unlocking Better Investments
What if you could automate your credit risk assessment workflows and make them faster, easier, and more accurate?What if you could save up to 91% on coding and data preparation time, 80% on data pipeline building time, and 65% on tool costs? Automated credit risk assessment workflows allow you to do just that. Let’s explore how! End-to-End Credit Risk Assessment Process The credit risk assessment is a lengthy process where banks receives hundreds of loan applications daily from various channels, such as online forms, email, phone, and walk-in customers. You must evaluate the creditworthiness of each applicant and decide whether to approve or reject the loan request. To assess credit risk accurately, you must collect and analyze data from multiple sources, such as its internal databases, external credit bureaus, and third-party data providers. However, collecting this data can lead to several several challenges, such as: The data is in different formats, such as CSV, JSON, XML, etc. The data is stored in different locations, such as local files, cloud storage, databases, etc. The data is updated at different frequencies, such as daily, weekly, monthly, etc. The data quality is inconsistent, such as missing values, errors, duplicates, etc. Data transformation and analysis require complex coding and scripting skills, such as SQL, Python, and R. The data pipeline is prone to errors and failures, such as network issues, server downtime, data corruption, etc. How LIKE.TG Helps Simplify Credit Assessment Data Extraction Some of the native connectors in LIKE.TG Banks use internal databases (like customer relationship and loan management systems) and external data providers (like credit bureaus) as the data sources. LIKE.TG offers Data Connectorsto set up secure connections to these data sources and ensure they have the necessary data access permissions. LIKE.TG has built-in connectors for various data sources, such as databases, files, web services, etc. You can leverage these connectors to select the data sources and select the fields they want to extract, such as customer demographics, credit history, transaction details, and loan details. LIKE.TG allows you to preview the data and apply filters and joins as needed using drag-and-drop operations without writing SQL queries or Python scripts. The data is retrieved and stored in a temporary location for further processing. LIKE.TG handles the data extraction process in the background and notifies the users when the data is ready. Data Transformation LIKE.TG also offers Data QualityandData Transformation features to inspect, clean, and transform the data. You can leverage a graphical interface to drag and drop various operations on the data without writing any code. You can clean the data by removing duplicates, filling in missing values, correcting errors, and standardizing entries. For instance, LIKE.TG’s Address Verification feature can help you standardize all addresses to a standard format. You can also transform the data by converting data types, encoding categorical variables like gender or loan type, normalizing numerical variables like income, and creating new features like debt-to-income ratio. For instance, you can also use LIKE.TG’s Expression Builderto create custom calculations and expressions on the data. Likewise, once your team has cleansed data for credit risk assessment, you can validate the transformed data by checking it against predefined rules and sample outputs. Here are some data validation rules that can make credit risk assessment more reliable: Probability of Default (PD): This is a key parameter in credit risk models. It measures the likelihood that a borrower will default on a loan.The validation of this parameter involves checking the discriminatory power of PD models. Loss Given Default (LGD): This measures the potential loss to the lender or investor in the event of default by a borrower.The validation process should include a review of the discriminatory power of LGD models. Expected Loss Best Estimate (ELBE): This is an estimate of the expected loss on an exposure.The validation process should ensure that the ELBE is calculated accurately. Credit Conversion Factor (CCF): This is used in the calculation of potential future exposure.The validation process should check the accuracy of the CCF. Data Quality: Credit institutions should have internally established quality and reliability standards on data (historical, current, and forward-looking information) used as model inputs. Model Design and Outputs: The validation should include a review of model design and model outputs/performance. Data Loading Once you’ve have ensured data quality, you must configure a secure connection to the bank’s data warehouse using LIKE.TG’s Data Connectors. LIKE.TG has native connectors for various data warehouses, such as Amazon Redshift, Google BigQuery, or Snowflake, and can also load data into other destinations, such as files, databases, etc. LIKE.TG’s Data Destinations can be critical in setting up the credit risk assessment pipelines. You must select the data warehouse and specify the destination table to store the cleaned and transformed data. LIKE.TG allows them to set the table name, schema, and keys with a few clicks without writing SQL commands. LIKE.TG also provides options to append, replace, or update the existing data with simple configurations. Once you decide on how to model your data, you can configure data loading using LIKE.TG’s Data Flows. LIKE.TG enables you to create and execute data flows that connect the data sources and destinations and apply the transformations and validations along the way. LIKE.TG also supports Change Data CaptureandSlowly Changing Dimensionsto load incremental and historical data. You can validate the loaded data by running sample queries and checking the results using LIKE.TG’s Data Preview. LIKE.TG allows them to view and query the data in the data warehouse and compare it with the source data. Data Aggregation Your team can define aggregation rules using LIKE.TG’s Aggregate transformation to group/aggregate the data by customer and month. You can group the data according to these rules. Lastly, you can validate the aggregated data by checking it against predefined rules and sample outputs using LIKE.TG’s Data QualityandData Preview features. LIKE.TG allows you to define and apply data quality rules and validations on the aggregated data and view and query the data in the data warehouse or any other data source or destination. Risk Analysis Credit risk assessment experts can define risk indicators using LIKE.TG’s data transformations. For instance, they can leverage LIKE.TG’s Expression Builder to create custom calculations and expressions on the data, such as debt-to-income ratio. You can also leverage LIKE.TG’s Data Quality Rulesto define and apply business rules and validations on the data, such as high credit risk. Automation Once deployed, your team can automate the credit risk assessment flows using LIKE.TG’s AutomationandOrchestration features. LIKE.TG allows you to create and execute workflows that automate the data extraction, transformation, loading, aggregation, and analysis processes and orchestrate them with other tasks, such as sending emails, calling web services, running commands, etc. You can also test the automation in a controlled environment using LIKE.TG’s Test ModeandDebug Mode features. LIKE.TG allows you to run the workflows in a test mode that simulates the data flow without affecting the actual data and debug the workflows in a debug mode that shows the data flow step by step and identifies any errors or issues. You can deploy the automation in the production environment using LIKE.TG’s DeploymentandScheduling features. LIKE.TG allows you to deploy the workflows to a server deployed on-premises or on the cloud and schedule them to run at regular intervals, such as every night at midnight, or trigger them on demand or by an event, such as a file drop or a web request. Monitoring and Updating LIKE.TG allows you to monitor your data warehouse and alert your team if the system fails or produces unexpected results. You can configure these mechanisms in LIKE.TG’s workflow canvas, notifying your team of of errors or failures by email or web service. You can regularly check the system to ensure it is working as expected and to identify any issues as soon as they arise by analyzing your workflow logs. LIKE.TG maintains a log of the workflow execution history and the data lineage and an audit trail of the workflow changes and the user actions. With these insights, your team can continually improve the system based on feedback from the data and risk management teams and new data using LIKE.TG’s Version ControlandMetadata Management features. LIKE.TG supports version control of the workflows, data sources and destinations, and metadata management of the data schemas and mappings. The Impact of LIKE.TG on Automating Credit Risk Assessment Save Time and Costs One of the benefits of using LIKE.TG to automate credit risk assessment workflows is that it can save time and costs for Banks. By automating the credit processes and digitizing the critical steps in the credit value chain, LIKE.TG can help Banks reduce the manual effort and complexity involved in data integration and processing. Likewise, LIKE.TG enables users to design, execute, and manage data integration workflows without writing any code, using a graphical interface, and using drag-and-drop operations. LIKE.TG also automates the data extraction, transformation, loading, aggregation, and analysis processes and orchestrates them with other tasks, such as sending emails, calling web services, running commands, etc. This means that users can save time and effort and focus on the business logic and outcomes rather than the technical details and complexities. LIKE.TG also makes it easy to collaborate and share workflows with other users and maintain and update workflows as the data and business requirements change. Read The Full Case Study According to McKinsey, this can yield up to 50 percent cost savings for Banks. The article also states that digitization can reduce credit losses by 10 to 20 percent by improving the accuracy and speed of credit decisions. Similarly, a survey by SP Global found that 71% of banks indicated that digitization provides better risk control and management to protect organizational profitability. Therefore, automating and digitizing the credit risk assessment workflows could help Banks save time and costs. Improve Data Quality and Accuracy One of the benefits of using LIKE.TG to automate credit risk assessment workflows is that it can improve data quality and accuracy for banks. These two metrics are crucial for making sound credit decisions, managing risk exposures, complying with regulations, and controlling pricing. Using LIKE.TG’s data quality features, finance firms can eliminate errors and inconsistencies and ensure the data is accurate, consistent, and reliable. LIKE.TG provides a range of data quality and data transformation functions, such as cleansing, validating, enriching, converting, encoding, normalizing, and creating custom transformations. LIKE.TG also provides real-time data health features, such as data profiling, data quality dashboard, and data quality rules, that allow users to inspect, monitor, and resolve data quality issues, such as missing, inconsistent, duplicate, or invalid values. LIKE.TG also supports data lineage and metadata management, allowing users to track and document data sources, destinations, schemas, mappings, and transformations. Lastly, Moody’s Analytics discovered that integrating risk data elements improves the origination workflow by providing higher accuracy in decisions, better assessment of total risk exposures, improved compliance, and greater control over pricing. LIKE.TG allows users to tap into multiple diverse data sources, which are typically difficult to access. Scale Data Operations and Performance LIKE.TG’s cloud-based and distributed computing capabilities can help scale data operations and performance. Cloud-based data sources such as Amazon S3, Google Cloud Storage, Azure Blob Storage, etc., allow users to store and access large volumes of data in the cloud and benefit from the cloud services’ scalability, availability, and cost-effectiveness. According to Accenture, cloud-based data integration solutions can reduce the total cost of ownership by 30% to 40%. Likewise, LIKE.TG supports cloud-based data warehouses, such as Amazon Redshift, Google BigQuery, or Snowflake. Lastly, parallel processing, load balancing, and fault tolerance helps users process large volumes of data faster and more reliably. As a result, they can benefit from the distributed systems’ efficiency, scalability, and resilience. Increase Data Security and Compliance Another benefit of using LIKE.TG is increased data security and compliance. Using LIKE.TG’s robust features, banks can encrypt the data in transit and at rest and adhere to industry standards and regulations. LIKE.TG provides data encryption features, such as SSL/TLS, AES, and PGP, that allow users to encrypt the data when it is transferred or stored and protect it from unauthorized access or tampering. One study revealed that 40% of teams continually review compliance controls with automation, which can increase data security and compliance. Therefore, LIKE.TG can help Banks improve data security and compliance by using its data security and compliance features. Save Millions in Investments by Simplifying Risk Assessment Automate your Risk Assessment using LIKE.TG Centerprise – a simple no-code ETL tool with a drag-and-drop interface that helps you simplify your ETL process with significant time and money savings. Book a FREE Demo Today! Transform Credit Risk Assessment with LIKE.TG LIKE.TG Centerprise is a powerful and user-friendly ETL tool that can help finance organizations automate credit risk assessment. It allows you to integrate data from various sources and systems, such as databases, data warehouses, file formats, cloud-based data providers, file systems, and web services. LIKE.TG Centerprise can help automate and orchestrate data flows and perform complex data transformations, validations, and mappings, providing your team with timely insights into credit risk assessment. With LIKE.TG, your team has a holistic view of each applicants data, giving them all the instruments they need to assess their credit worthiness effectively. If you want to experience the power of LIKE.TG for yourself, sign up for a free 14-day trial or schedule a custom demo with our experts.
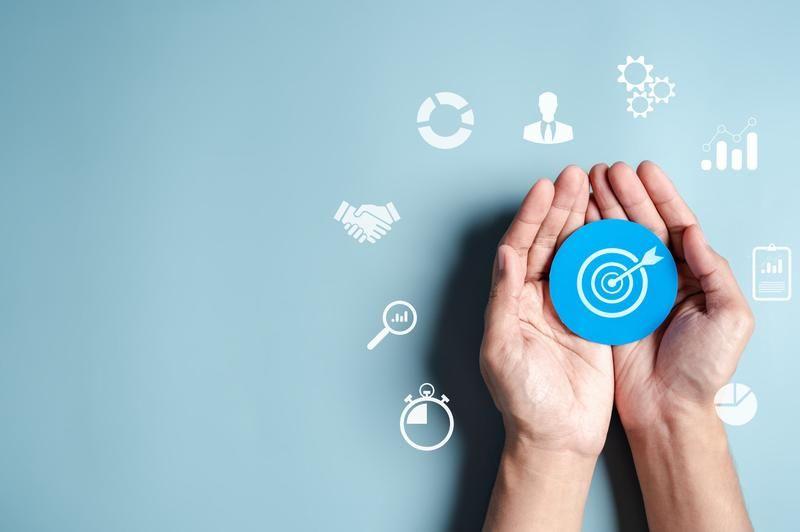
Salesforce Data Migration: What Is It & How to Set It Up?
What is Data Migration in Salesforce? Salesforce data migration is the process of transferring data from external sources, legacy systems, typically databases, into the Salesforce platform, a cloud-based customer relationship management (CRM) platform. This migration is often undertaken when an organization adopts Salesforce for managing its customer data, sales, marketing, and other business processes. Why Migrate Data from Legacy Systems to Salesforce? Salesforce data migration will likely be an inevitable process if you decide to adopt Salesforce as your customer relationship management (CRM) solution or need to integrate it with other applications. Migrating to Salesforce from legacy platforms, in particular, offers numerous advantages that can significantly enhance your company’s efficiency, scalability, and overall business operations. Here are a few key reasons why you might want to migrate to Salesforce: Scalability: With Salesforce, your system can effortlessly handle increasing data volumes and user loads as your business grows, ensuring optimal performance and responsiveness. Cloud Accessibility: Access your data and applications anytime, anywhere, with the convenience of a cloud-based platform, fostering collaboration and enabling remote work. Advanced Automation: Leverage robust automation tools in Salesforce to streamline and automate your sales and service processes, reducing manual effort and enhancing operational efficiency. Flexible Customization: Tailor Salesforce to match your unique business processes, ensuring a perfect fit for your organization’s specific needs and evolving requirements. Security and Compliance: Benefit from Salesforce’s top-tier security measures, including data encryption and user access controls, ensuring the confidentiality and compliance of your sensitive business data. Types Of Data Migration in Salesforce Salesforce data migration can be divided into four different types: Database Migration: Migrate data from external databases, such as SQL, NoSQL, or other CRM systems, to Salesforce. Ensure a smooth transition by considering schema differences. Application Migration: Transition both data and replicate application functionality from your existing CRM or business application to Salesforce. This involves extensive configuration or customization. Storage Migration: Manage the migration of attachments and files within Salesforce by moving them to external storage systems. Alternatively, utilize Salesforce’s external file storage options to efficiently handle data within specified storage limits. Cloud Migration: Execute the transfer of data from on-premises systems or other cloud platforms to Salesforce. Leverage Salesforce’s cloud-based infrastructure, including IaaS, PaaS, or SaaS, for improved accessibility and scalability. There’s another use case of data migration from Salesforce Classic to Lightning. If you’re currently using the legacy version and considering a move from Salesforce Classic to Lightning, you’ll be stepping into the revamped edition of the system. This transition offers you the opportunity to access exclusive features and enjoy an improved UI/UX. The Salesforce Data Migration Checklist While your actual Salesforce data migration journey will likely be different—owing to your unique requirements—the following are the typical Salesforce data migration steps that you can expect: Planning: First and foremost, you need a Salesforce data migration plan that defines the scope, objectives, and success criteria for your Salesforce data migration. Identify the source systems, data entities, and stakeholders involved. Your Salesforce data migration plan should also be clear about the timelines, resources, and responsibilities. Data Assessment: Conduct a thorough analysis of your existing data. Identify data sources quality and potential issues such as duplicates or incomplete records. Assess data structure, formats, and relationships to create a clear picture of what needs to be migrated. Data Mapping: Create a mapping between source and target data fields in Salesforce. Specify how data will be transformed and mapped during the migration process. Ensure alignment with Salesforce data models and consider any necessary data cleansing or enrichment. Data Extraction: Extract data from the source systems according to the mapping plan. Ensure that data extraction processes maintain data integrity and consider the use of extraction tools or scripts to automate the process and minimize errors. Data Transformation: Apply necessary transformations to the extracted data to align it with Salesforce requirements. This includes data cleaning, formatting adjustments, or any other modifications needed to ensure compatibility with Salesforce data structures. For example, you may need to standardize address information if it’s in a different format. Data Validation: Perform thorough validation checks on the data to ensure accuracy and completeness. Apply custom validation rules to validate data against predefined criteria and reconcile any discrepancies to maintain data quality. Data Loading: Load the transformed data into Salesforce. Depending on the volume of data, consider using enterprise-grade data migration solutions for moving your data into Salesforce. Remember, this Salesforce data migration checklist is not exhaustive. However, it should give you an idea of the overall process. Salesforce Data Migration Tools Salesforce offers native data migration tools, such as Salesforce Data Loader, but they can be complex for non-technical users. Users without a strong technical background find it challenging to navigate and use these tools effectively. Moreover, they face limitations when dealing with large volumes of data. For enterprises with extensive datasets, the performance of these tools is not as efficient. In addition, they struggle with complex data transformations and are unable to handle intricate data structures. To mitigate these issues, you must consider third-party solutions. When it comes to migrating data to Salesforce, Extract, Transform, Load (ETL) tools are key players. There’s a variety to pick from, suitable for different technical skill levels. Despite similarities, each tool has its own technical strengths and weaknesses. When deciding, consider your company goals and ensure the chosen tool has the technical capabilities needed for a smooth transition to Salesforce. There’s a notable shift towards the adoption of no-code solutions for Salesforce data migration. These tools streamline the process, requiring no coding expertise and ensuring accessibility for a diverse user base. Importantly, the focus extends beyond singular data migrations; these tools excel at automating ongoing ETL processes. This commitment to automation contributes to sustained operational efficiency, offering a solution for long-term data management strategies. We recommend using LIKE.TG—a unified, no-code data management solution to handle complex Salesforce data migration as it can handle large-volume migration and ETL processes with ease. How to Migrate from Legacy Platforms to Salesforce Using LIKE.TG, you can seamlessly migrate your data from legacy platforms to Salesforce in a matter of minutes. Here’s a step-by-step guide: Step 1: Connect to your legacy destination. This initial step establishes a connection to the source system where your data is currently residing. LIKE.TG supports a wide range of legacy and new systems. For example, let’s select the legacy IBM Db2 system. After ingesting the data, you can use the data preview feature to examine the data fields. In this instance, we examine the data within the customer table. Step 2: Apply transformations to cleanse and filter data Cleaning the data by removing records without specified regions is essential for ensuring data integrity and accuracy in the new Salesforce system. This step enhances the quality of the information being migrated, preventing issues related to incomplete or inconsistent data. The above data preview shows missing data in the region column. So, let’s add a data quality object rule to remove the records that have no region specified. Now, the data preview shows the fields with data in the Region column. What about the rows with missing data? We can use the Log function to get a record-level log of the data. It highlights all the fields with empty values in the Region column. Step 3: Connect to the Salesforce Database. Establish a secure connection to the Salesforce database. Select Specify Database Information, choose Salesforce REST V2 as a Data Provider, enter the credentials, and you’re all set. After the connection is established, map the fields to the Salesforce Database. Step 4: Execute the workflow. The final step is to execute the dataflow to complete the transition from the outdated IBM Db2 system to Salesforce. And that’s it! The data is migrated to Salesforce. Salesforce Data Migration Best Practices Here are some best practices to keep in mind when migrating data from legacy system to Salesforce: Thorough Data Assessment: Before initiating Salesforce data migration, carefully assess your existing data to identify inconsistencies, redundancies, and incomplete records. A comprehensive understanding of your data will guide your migration strategy and minimize disruptions. Define Clear Objectives: Clearly outline your data migration goals and set specific objectives. Define what success looks like in terms of data accuracy, completeness, and timeliness. Having a well-defined plan will keep your migration on track and ensure that you meet your business requirements. Establish a Robust Data Mapping Strategy: Create a comprehensive data mapping document that clearly outlines how data from the source aligns with Salesforce objects and fields. Use a data mapping tool to quickly map source and target data fields. Ensure data integrity by mapping fields accurately, considering data types, and addressing any transformations needed during migration. Thoroughly Test and Validate: Rigorously test your Salesforce data migration plan in a sandbox environment before executing it in a live Salesforce instance. Verify data accuracy, completeness, and adherence to validation rules. Prioritize Ease of Use: Choose a user-friendly Salesforce data migration solution that facilitate a smooth migration process without extensive coding requirements. Embrace no-code platforms to simplify tasks and empower non-technical users to actively participate in the migration process, promoting efficiency and reducing the risk of errors. Cost of Salesforce Data Migration Determining the cost of migrating to Salesforce involves assessing variables such as your company size, migration complexity, and the specific Salesforce product selection. Due to the nuanced nature of these factors, providing a number, even a ballpark figure, is difficult. For a customized cost estimate tailored to your use case, reach out to our sales team. We’ll discuss your requirements and provide a cost estimate for data migration into Salesforce. Conclusion Migrating your data to Salesforce is a smart choice because it centralizes and streamlines your customer information. But keep in mind that it’s just the first step in the right direction. You must design a data architecture to ensure your Salesforce system has accurate and complete data available for timely reporting and analytics. Therefore, consider investing in a tool like LIKE.TG that allows you to seamlessly migrate data into Salesforce and create automated data pipelines — without writing code. In addition to smooth data migration, it serves as a scalable, long-term solution to handle complex ETL and data integration processes. Would you like to learn more about how LIKE.TG can help you migrate data into Salesforce? With years of experience in data migration, our team stands ready to offer valuable guidance and advice to support you throughout the entire process. Contact us now or request a free 14-day trial! Is Salesforce Data Migration Holding You Back? Let's Fix It. Simplify your Salesforce migration process with LIKE.TG's no-code solution. Migrate data seamlessly from outdated platforms and unlock the full potential of Salesforce. Sign Up for FREE trial
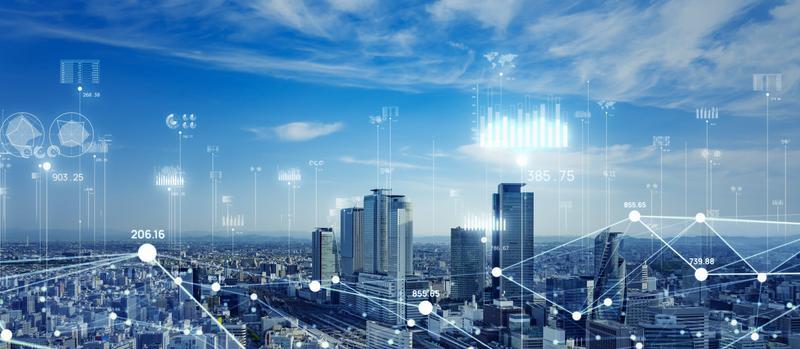
Data Quality Framework: What It Is and How to Implement It
What is a data quality framework? A data quality framework is a set of guidelines that enable you to measure, improve, and maintain the quality of data in your organization. The goal is to ensure that organizational data meets specific standards, i.e., it is accurate, complete, consistent, relevant, and reliable at all times—from acquisition and storage to subsequent analysis and interpretation. eBook: A Guide to Data Quality Management Download eBook With a well-defined framework, you can establish roles, responsibilities, and accountability mechanisms for data quality and stewardship. So, when everyone in your organization understands their role in maintaining data quality, everyone will take ownership of the data they interact with, and, as a result, everyone will have the same high-quality information to work with. As important as it is to know what a data quality framework is, it’s equally important to understand what it isn’t: It’s not a standalone concept—the framework integrates with data governance, security, and integration practices to create a holistic data ecosystem. It’s not a single tool or a piece of software—it’s a comprehensive strategy that combines various tools, processes, and best practices to achieve data quality goals. It’s not a magic bullet—data quality is an ongoing process, and the framework is what provides it a structure. It’s not just about fixing errors—the framework goes beyond cleaning data as it emphasizes preventing data quality issues throughout the data lifecycle. A data quality management framework is an important pillar of the overall data strategy and should be treated as such for effective data management. Why do you need a data quality framework? Most organizations are overwhelmed with vast amounts of data from various sources, such as internal systems, external partners, and customer interactions. Without a clear understanding of the quality of this data, they risk making decisions based on information that might be flawed and incomplete, leading to suboptimal outcomes and missed opportunities. Consider this: as the chief data officer (CDO), you are responsible for cultivating a data-driven culture across the organization to harness the full potential of its data. One of the key activities in the process is laying the groundwork for delivering the data needed by everyone in the organization. However, simply providing access to this data is not enough—its quality must be impeccable. And this is why you need to implement a framework for data quality management. From the business perspective, the framework is a strategic asset that directly impacts your organization’s success. While the timely delivery of data is crucial, it’s the quality of that data that truly drives meaningful insights and decision-making. A well-established data quality management framework leads to healthy data that is necessary for: Improved diagnoses and better patient outcomes Timely fraud detection and better risk management Development of better products and enhanced customer experiences Efficient resource allocation and optimized supply chain management So, instead of viewing it as a short-term expense, understand that building and implementing a data quality framework is an investment in the sustained growth of your organization. Ensure Only Healthy Data Reaches Your Data Warehouse Learn More What are the components of a data quality framework? These are important elements or building blocks that come together to create a system that ensures your data is trustworthy and useful. Just like a building needs a solid foundation and supporting structures to stand tall, a data quality framework requires specific components to function effectively. These components encompass various aspects of data management, governance, processes, and technologies to uphold data quality standards. Some set the ground rules and expectations, while others actively assess and improve the data itself. There are also components that ensure you’re continuously measuring and tracking progress. While there isn’t a single, universally agreed-upon list of components for a data quality framework, some common elements appear in most frameworks: Data quality tools and technologies This includes using data quality tools and technologies to support data quality management. These tools enable you to automate several tasks that go into improving data quality. The actual processes these tools automate vary depending on the specific needs and objectives of your organization. However, common tasks that can be automated include data standardization, profiling, cleansing, and validation. Data quality standards These are the guidelines that define what constitutes high-quality data in your organization. For example, a data quality standard may specify that customer information should include email addresses and phone numbers as part of contact details to be considered complete. Data quality rules Data quality rules take a granular approach to maintaining data quality. These rules validate data as they define specific criteria or conditions that it must meet to be considered high quality. For instance, if you collect customer data, your business rules might state that all dates should be in a particular format (e.g., mm/dd/yyyy). Any date that does not conform to this rule will be considered invalid. Data profiling This is your framework’s diagnostic tool that can provide insights into your data’s health. Data profiling is analyzing and summarizing data to learn about its current state, i.e., its structure and content. Specifically, it uncovers problems such as missing values and invalid formats. Data profiling is one of the most effective ways to ensure that your decisions are based on healthy data, as it helps identify data quality issues before you load data into the data warehouse. Data quality assessment Data quality assessment is a complete evaluation of your data’s quality. It’s a systematic approach to measuring and analyzing the quality of your data and identifying areas for improvement, and, therefore, an effective way to confirm whether it meets the organization’s needs. As it provides a comprehensive view of the data’s health, you can use it to inform decisions on data governance and compliance efforts. Data cleaning The data you collect from various sources is not always clean. In fact, it’s commonplace for it to contain errors, duplicates, or missing values. Data cleaning, or cleansing, enables you to detect and fix these inconsistencies in your data sets, making it fit for purpose. Data quality monitoring Data quality monitoring is the ongoing process of measuring and evaluating the quality of your data across various dimensions. Your data teams must define and keep track of a tailored set of KPIs to monitor the health of data in your organization. It’s one of the most important components of a data quality framework as it guides the decisions pertinent to improving the framework itself. Take the First Step Towards Enhancing Data Quality. Try LIKE.TG for Free. Ready to maximize the health of your data? Try LIKE.TG's leading platform and witness firsthand how it improves data quality, elevating your insights and decision-making. Download Trial What are the different data quality frameworks in use today? As previously stated, there is no one-size-fits-all solution when it comes to data quality frameworks. Every organization has unique requirements driven by: Its business objectives Data sources and technology infrastructure The industry it operates in and the regulatory environment This is why there are a number of different data quality frameworks that organizations either implement with modifications or use as references to create their own framework. Let’s go through the different frameworks and approaches: Leveraging the data governance frameworks Because data governance and data quality are interconnected and mutually reinforcing, many organizations develop their data quality frameworks as part of broader data governance initiatives. Integrating data quality into data governance frameworks facilitates the alignment of data management processes with strategic business objectives as you adopt a comprehensive approach that addresses not only data quality but also data privacy, security, compliance, and stewardship. On the flip side, implementing data governance frameworks alongside data quality initiatives can be complex as it requires restructuring and realigning organizational roles and reporting relationships for effective coordination and collaboration. You will also need to create additional policies specifically focused on data quality standards and metrics. Additionally, you will need to account for compatibility with additional solutions, such as data quality tools or data profiling software. Data Quality Assessment Framework (DQAF) IMF’s DQAF is a structured approach to evaluating how well your data meets your organization’s specific needs. It helps you define what “good quality data” means in your context and then assess how close your current data comes to that definition. The DQAF proves to be valuable in several situations, For example, when initiating a data quality improvement project, it provides a baseline understanding of your current data quality standing, allowing you to prioritize improvement efforts accordingly. While DQAF defines clear data quality expectations, ensuring everyone is on the same page about what constitutes good data, it has its fair share of shortcomings. Notably, it emphasizes statistical data, which may not be the best choice if your data types are highly varied. Additionally, the framework does not lay a strong emphasis on data governance. Data Quality Maturity Models (DQMMs) Data Quality Maturity Models (DQMMs) take on a different approach to ensuring data quality in an organization. DQMMs, such as the Data Management Maturity (DMM) model or the Capability Maturity Model Integration (CMMI), provide your organization with a structured framework for assessing its maturity in managing data quality. More specifically, they offer a roadmap that your organization can follow to understand its current state of data quality management, identify areas for improvement, and establish a path toward achieving higher levels of maturity. An important point to keep in mind is that assessing maturity levels in data quality management involves subjective judgments and interpretations, which introduces variability in assessments. Moreover, DQMMs involve multiple dimensions, levels, and criteria for assessing maturity, which can be overwhelming for organizations, particularly if they have limited experience or expertise in data quality management. Data Quality Scorecard (DQS) The Data Quality Scorecard (DQS) is a data quality framework designed to give you a comprehensive picture of your data’s health over time. It goes beyond simply identifying issues and delves into tracking progress toward data quality goals. DQS assigns a single, high-level score (e.g., percentage or grade), calculated by combining the individual metric values. These values are typically weighted based on their relative importance to your organization. A high score indicates good overall data quality. That being said, setting up a DQS involves selecting the metrics relevant to your organization, assigning them weights, and defining a scoring methodology, all of which are time-consuming—especially if your organization has a complex data landscape. This is mostly due to the inherent subjectivity in the process of deciding on the “most relevant” metrics and assigning them weights. Furthermore, while DQS does track progress made toward achieving data quality goals, it doesn’t offer any guidelines to actually improve data quality. Total Data Quality Management (TDQM) TDQM, developed at MIT by Richard Y. Wang, is a holistic data quality framework—it establishes standards, policies, and procedures for managing data quality throughout the entire data lifecycle, from collection to analysis. Along with processes for monitoring, preventing, and fixing data quality issues, TDQM also emphasizes ongoing improvement. Unlike some frameworks with predefined data quality dimensions, TDQM allows you to define your own set of dimensions. While the idea of defining custom dimensions sounds excellent, it’s easier said than done. Defining and selecting the most relevant dimensions requires reaching a consensus, which is often a tedious process—stakeholders usually have varying priorities. But that’s not all; you also need to establish data quality measurement processes and integrate data quality tools with existing workflows—warranting a dedicated team with expertise in data quality management. Take the First Step Towards Enhancing Data Quality. Try LIKE.TG for Free. Ready to maximize the health of your data? Try LIKE.TG's leading platform and witness firsthand how it improves data quality, elevating your insights and decision-making. Download Trial Creating and implementing a data quality framework It goes without saying that you need to understand your business needs down to the finest detail before venturing into creating and implementing a data quality framework. To start off, pinpoint the data elements driving core business decisions. Is it customer information for marketing campaigns, product data for sales, or financial records for accounting? Define data quality goals and dimensions Your data quality goals should vary based on departmental needs to ensure alignment with business needs. Define what “good data” means for your organization using relevant data quality dimensions. Having said that, defining data quality goals and dimensions can be a challenge due to multiple reasons. First, “good data” can mean different things for different parts of your organization. The marketing team might prioritize customer contact information accuracy, while the finance department might care more about the completeness and timeliness of financial data. Second, there’s usually a trade-off between different data quality dimensions. For instance, achieving 100% accuracy might require extensive manual data entry, slowing down the process (timeliness). Third, external data sources might have inherent quality issues beyond your control. A simple example would be that of customer addresses from a purchased list having a higher error rate than internally collected information. Let’s not forget that the goals you set today will need to be updated to reflect future priorities as your business needs and data usage change over time. Set data quality standards and metrics Before you can establish standards and metrics, you must evaluate the current state of data quality in your organization to identify inconsistencies, inaccuracies, and gaps in the data across various systems and departments. These issues usually stem from disparate data sources, a lack of standardized data entry procedures, and insufficient data governance measures. Use specialized tools to accelerate the process. Once there’s clarity on the current state of your data, set quality standards and metrics for each data quality dimension. Define acceptable thresholds for data quality to ensure consistency and reliability. Develop data quality policies and procedures Next, along with creating policies and procedures for data quality management, define clear ownership for data quality. Who creates data quality standards? Who monitors and enforces them? This also calls for setting up rules to ensure incoming data adheres to your standards. This could involve defining data formats, acceptable value ranges, or mandatory fields. Leverage data quality tools, such as data profiling tools, data cleansing software, and data quality monitoring platforms, to automate data validation and quality checks as part of your data ingestion and processing pipelines. The goal is to identify issues early and prevent them from cascading downstream. Monitor and control data quality Based on the dimensions that you’ve already defined earlier in the process, establish KPIs to measure data quality. You can implement automated alerts for detecting data quality issues in real-time to simplify the process. To ensure continuous progress, have your data governance committee regularly review these metrics and KPIs. The data quality framework does not end here—regularly reviewing your data quality processes based on insights from monitoring and adapting them to address evolving needs is a critical part of the framework. Tips and best practices Clearly communicate data quality goals, standards, and best practices across your organization. Focus on improving data quality for the data sets with the most significant business impact, for example, customer information, sales data, or financial records. Integrate data quality initiatives with broader data management processes, such as data integration, data migration, and master data management, to ensure consistency and alignment across the organization. Ensure data related to areas like healthcare or finance meets industry standards and regulatory requirements. Utilize modern data management tools with build in data governance features, such as LIKE.TG, for automating the data profiling, validation, and cleansing tasks. Conduct regular reviews and audits of the data quality framework to assess its effectiveness and identify areas for improvement. Bringing it all together Data quality is not a one-time fix; it’s an ongoing effort. What streamlines it for your organization is a tailored data quality framework—one that directly addresses your unique data quality challenges. However, given the exponential rise in data volume, and the associated data quality issues, what your organization needs is a data quality framework reinforced by a modern data management platform with advanced data quality and governance features, such as LIKE.TG Data Stack. LIKE.TG Data Stack is an AI-powered, end-to-end data management platform with powerful data quality and governance capabilities built into it. Its 100% no-code UI makes data profiling, validation, and cleansing effortless—even for business users. To get started with LIKE.TG, sign up for a free demoor get in touch with one of our data solutions experts if you want to discuss your use case. See It in Action: Sign Up for a Demo Curious about how LIKE.TG's platform improves data quality? Sign up for a demo and explore all the features you can leverage to get analysis-ready data without writing a single line of code. View Demo
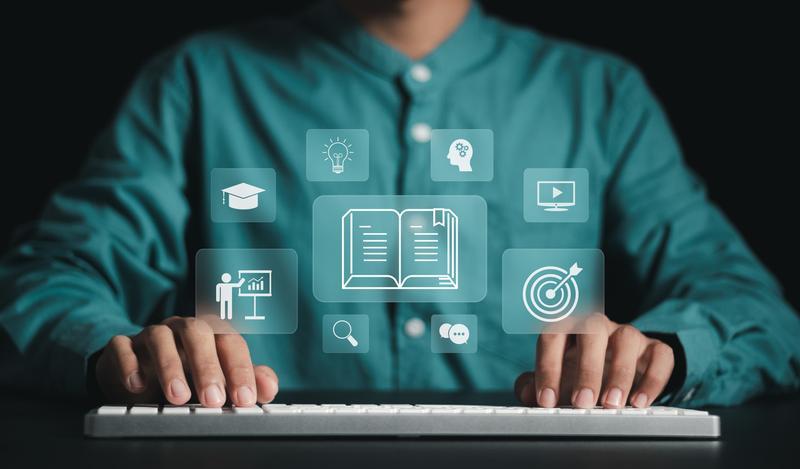
Finance 360: How ETL Drives Strategic Insights In Minutes
Did you know gaining customer behavioral insights can increase sales by 85 percent and gross margin by over 25 percent? Customer data is strategic, yet most finance organizations use only a fraction of their data. Sprawling legacy systems, siloed databases, and sporadicautomationare common obstacles. Finance 360 is a comprehensive approach to data management that bypasses these challenges, giving you a complete and accurate picture of your financial performance and health. Data pipelines enable Finance 360, allowing you to collect data from diverse sources, formats, and systems while ensuring high quality, security, and accuracy. ETL: The Key to Finance 360 ETL is the building block of Finance 360. It facilitates the seamless collection, consolidation, and transformation of data from diverse sources and systems into a unified and standardized format.The advantages of this integration extend beyond mere organization; it significantly improves data quality and accuracy. It eradicates data silos, eliminates duplicates, rectifies errors, and addresses inconsistencies so that your businesses can cultivate a foundation of reliable and trustworthy financial data. How No-code ETL Helps Achieve Finance 360 No-code ETL simplifies and automates the data integration process, allowing to build Finance 360 faster, easier, and more efficiently. Data pipelines help you save time and resources, improve data quality and accuracy, and enable data-driven decision-making. As a result, the tool can help your team: Design, build, and execute data pipelines that perform data extraction, transformation, and loading (ETL) tasks using a graphical user interface (GUI) and drag-and-drop functionality. You don’t need to write code or script to create and run your data pipelines. You can also schedule, monitor, and manage your data pipelines from a centralized dashboard, ensuring that Finance 360 pipelines are always up-to-date and reliable. You can access and ingest data from any source and system, regardless of the data’s location, format, or structure. You can also convert data into a standard data model and format, such as CSV, JSON, XML, or Parquet, to facilitate data analysis and reporting. As a result, your Finance 360 solution can collect customer data from a diverse set of sources. Perform various data transformation and enrichment functions, such as filtering, sorting, joining, merging, splitting, aggregating, calculating, validating, cleansing, and more. You can apply various functions and operations to your data to transform and enrich it according to your business rules and logic. You can also add metadata, such as data type, data lineage, data quality, and data governance, to your data to enhance its quality and value. Finance 360 Benefits Cost optimization: You can reduce your data management processes’ complexity, redundancy, and inefficiency by applying cost optimization techniques to data integration. This can lower the total cost of ownership, improve the return on investment, and free up resources for more strategic initiatives. According to a report by Gartner, cost optimization is crucial for modern data management programs, including data integration. Business value: You can enhance the quality, accuracy, and timeliness of your financial data by enabling greater visibility, standardization, and oversight in data management practices. This can lead to better insights, actions, and outcomes for your business. According to a study by McKinsey, by recovering and redeploying as much as 35 percent of their current data spend, companies can increase their data value by 10 to 20 percent. Financial perspective: You can go beyond merely listing subscribed SaaS products by providing a 360° financial perspective. You can delve into the intricate user dynamics behind these subscriptions, such as usage, adoption, and satisfaction. This can help you identify opportunities for optimizing, rationalizing, and consolidating your SaaS portfolio. According to a survey by Blissfully, a 360° financial perspective can help you save up to 30 percent on your SaaS spending. Pricing negotiation: You can negotiate better pricing and reduce the overall cost of your SaaS subscriptions by standardizing on a select set of tools. You can also leverage Finance 360 capabilities to benchmark your spending against industry standards and best practices and to demonstrate your value proposition to vendors and partners. The benefits of ETL extend to enhancing both accessibility and usability. Standardizing and harmonizing financial data streamlines accessing, analyzing, and sharing information within the organization and beyond, fostering a more collaborative and informed decision-making environment. The ultimate goal of this integration is to empower data-driven decision-making and action. Providing a comprehensive and holistic view of financial data enables businesses to uncover valuable insights, identify trends, recognize patterns, and seize opportunities that can substantially improve overall performance, efficiency, and profitability. Use Cases for Finance 360 Insurance Finance 360 allows institutions to comprehensively manage insurance contracts, claims, premiums, and losses by accessing and integrating data from various sources, such as reinsurers, brokers, and regulators. For instance, finance 360 can help insurance providers manage First Notice of Loss (FNOL) more efficiently. FNOL is the initial report made to an insurance provider following a loss, accident, or theft. This process is challenging for insurers as they need to balance multiple objectives simultaneously: providing a user-friendly experience, managing real-time expectations, creating an emotional connection with customers, collecting accurate information, and keeping costs in line. According to McKinsey, one leading North American insurer improved its FNOL process by leveraging three additional capabilities by leveraging digitization and advanced analytics. Digitization: The insurer improved response times by using digital technologies to access third-party data sources and connect with mobile devices.This allowed the insurer to track claimant locations, automatically dispatch emergency services, and enable customers to upload pictures of damages and file and track claims online. Advanced analytics: The digitization of the FNOL journey provided the insurer with more and better data faster. This allowed the insurer to apply the latest modeling capabilities to better data, improving decision making in the FNOL journey.For example, intelligent triage is used to close simple claims more quickly, and smart segmentation identifies claims likely to be total losses and those liable to require the special investigative unit (SIU) far earlier than before. Now, let’s see how Finance 360 could help in this use case: Finance 360 enables and empowers digitization and advanced analytics in the following ways: It helps the insurer measure the impact of their transformation efforts and benchmark their performance against their peers. By using Finance 360, the insurer can gain cost transparency, performance comparison, improvement tracking, and industry insights.This helps them identify areas for improvement, understand the root causes of performance gaps, track the success of improvement efforts, and learn from the best practices and strategies of top performers. It supports the insurer’s digitization of the FNOL journey by providing a clear view of the cost structure along the value chain. Finance 360 can help the insurer understand where they are incurring the most costs during the FNOL process and identify areas for optimization.For example, the insurer can use Finance 360 to compare the costs of different channels, such as online, mobile, or agent, and determine the optimal mix of channels for their customers. It enhances the insurer’s use of advanced analytics by providing access to more and better data faster. Finance 360 allows the insurer to collect and analyze data from multiple sources, such as ERP, CRM, and BI systems, and reconcile it with third-party data sources. This enables the insurer to apply the latest modeling capabilities to better data, improving decision making in the FNOL journey.For example, the insurer can use Finance 360 to segment claims based on various criteria, such as complexity, severity, or fraud risk, and assign them to the appropriate resources. This data can help institutions streamline their operations and optimize their contracts by reducing their exposure and liability and increasing their coverage and capacity. Additionally, by using big data and analytics, institutions can efficiently estimate premiums, assess claims, and resolve disputes using historical, current, and predictive data. Customer Segmentation Finance 360 allows businesses to access and analyze customer data, such as demographics, transactions, behaviors, and preferences. This data can help companies to segment their customers into different groups based on their characteristics, needs, and value. For example, a business can identify its most profitable, loyal, or at-risk customers and tailor its products, services, and promotions accordingly. This can help increase customer satisfaction, retention, and revenue and reduce costs and churn. McKinsey reports that implementing customer segmentation strategies may increase revenue by 10 to 15 percent while concurrently reducing costs by 10 to 20 percent. Personalized Banking Finance 360 enables banks to provide their customers with seamless and customized banking experiences by accessing and leveraging their data, such as identities, accounts, transactions, and feedback. This data can help banks deliver personalized services, such as online and mobile banking, chatbots, and voice assistants, that cater to the customers’ preferences, needs, and goals. Furthermore, using artificial intelligence and natural language processing, banks can enhance customer interactions by understanding their queries, requests, and feedback and providing relevant recommendations and suggestions. According to Accenture’s survey, initiatives in personalized banking have the potential to elevate customer satisfaction by 20 percent and improve retention rates by 30 percent. Fraud Detection and Prevention Finance 360 allows institutions to consolidate and monitor transaction data from various sources, such as banks, payment processors, credit bureaus, and government agencies. This data can help institutions detect and prevent fraudulent activities, such as identity theft and money laundering, using advanced analytics and machine learning to identify patterns, anomalies, and outliers. By detecting suspicious activities in real-time, institutions can trigger alerts and notifications and take appropriate actions to mitigate the risks and losses. IBM’s research suggests that implementing effective fraud detection and prevention measures could save the banking industry up to $4 billion annually. Asset Management Finance 360 allows institutions to streamline and optimize asset management processes by accessing and aggregating data from various sources, such as market trends, portfolio performance, and risk assessments. This data can help institutions manage their assets, such as stocks, bonds, and funds, using data science and optimization techniques to devise and execute effective allocation and portfolio strategies. These strategies can help improve the assets’ performance, efficiency, and profitability and reduce costs and risks. Deloitte’s reports indicate that such methods can boost performance by 15 to 20 percent while concurrently reducing costs by 10 to 15 percent. Credit Scoring Finance 360 allows institutions to accurately assess their customers’ creditworthiness and risk profiles by accessing and analyzing diverse credit data, such as credit history, income, expenses, and behavior. This data can help institutions assign precise credit scores and ratings to their customers, reflecting their ability and likelihood of repaying their debts. Moreover, by using machine learning and deep learning algorithms, institutions can refine their credit decisions by incorporating more variables, features, and models and ensuring fairness and accuracy. PwC’s analysis suggests that effective credit scoring mechanisms can reduce default rates by 25 percent and increase approval rates by 15 percent. How LIKE.TG Enables End-to-End Data Pipelines for Finance 360 LIKE.TG is a leading ETL solution enabling end-to-end data pipelines for Finance 360. Through LIKE.TG Centerprise, businesses can establish a single source of truth for their data assets. This unified view allows for the extraction of valuable insights and facilitates informed, data-driven decision-making. By leveraging LIKE.TG Centerprise’s capabilities, businesses can effectively navigate the complexities of data integration and harness the power of their data to drive success in their finance operations. Some of the key features and capabilities of LIKE.TG Centerprise are: No-code data pipeline builder and ETL solution: LIKE.TG Centerprise allows businesses to build end-to-end data pipelines without writing code, using a drag-and-drop interface and a parallel-processing engine. Robust, user-friendly, and scalable features: LIKE.TG Centerprise can handle complex and varied datasets, effortlessly performing ETL/ELT operations to harmonize data from transactions, customer profiles, market trends, and risk assessments. It can also load data into any destination, adding flexibility and adaptability to the process. Extensive library of connectors, transformations, and data quality features: LIKE.TG Centerprise offers a wide range of connectors, transformations, and data quality features, ensuring seamless integration and reliable data delivery. It can access, cleanse, transform, validate, and load data from any source to any destination, all within a unified platform. Single source of truth for data assets: LIKE.TG Centerprise enables businesses to establish a single source of truth for their data assets by consolidating and transforming data from various sources into a unified and consistent format. This unified view allows for the extraction of valuable insights and facilitates informed, data-driven decision-making. LIKE.TG helps you effectively navigate the complexities of data integration and harness the power of your data to drive success in their finance operations. LIKE.TG Centerprise can also help businesses achieve significant benefits, such as: Reducing data integration time: LIKE.TG Centerprise’s data integration platform is designed for superior usability, productivity, and performance. It has powerful transformations, data validation capabilities, and automation features to schedule tasks, which minimize the time required to download files and set up manual integration processes, increasing productivity. As a result, your team can reduce integration time by 80%. Parallel Processing: LIKE.TG is backed by a parallel processing ETL engine that can execute massive integration jobs in milliseconds, making handling large volumes of data easier. Improving data quality and accuracy: LIKE.TG Centerprise offers comprehensive data quality features to ensure data accuracy, reliability, and completeness. It can help build an end-to-end pipeline to clean, validate, and standardize data as it arrives from different sources across the enterprise, fixing data quality issues. With the help of these features, LIKE.TG helps you improve data quality by up to 90%. If you want to learn more about how LIKE.TG Centerprise can enable Finance 360 for your business,schedule a custom demo now! Discover LIKE.TG Centerprise’s Benefits for Finance 360 LIKE.TG's user-friendly ETL automates data pipelines for Finance 360. Boost efficiency gain a single source of truth. Learn More
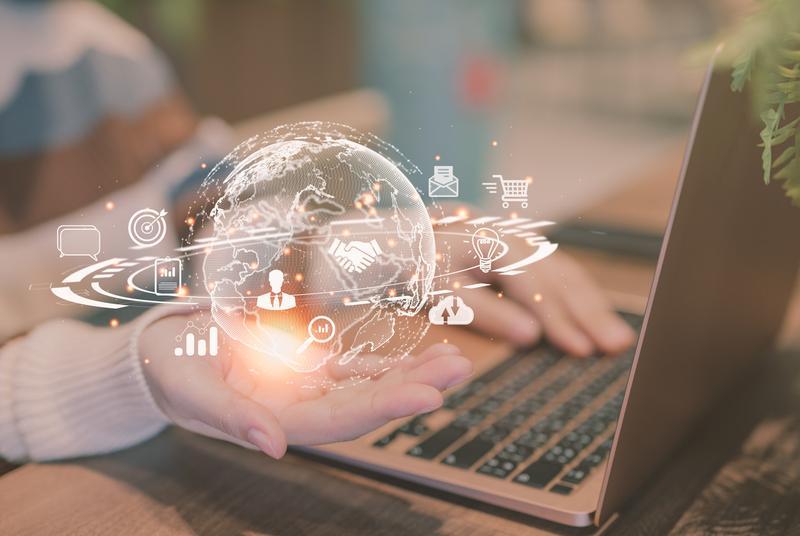
Beyond OCR Form Processing: The Comprehensive Guide to Intelligent Form Data Extraction
As businesses continue to deal with an ever-increasing volume of forms, invoices, and documents, the need for accuracy, speed, and adaptability in data extraction has never been more pronounced. Traditional Optical Character Recognition (OCR) technology has long been a staple in form processing, but as technology advances, so do the expectations for smarter, more intelligent solutions What is OCR Form Processing? OCR, or Optical Character Recognition, is a technology that converts different types of documents, such as scanned paper documents and pdfs into editable and searchable data. OCR form processing specifically refers to the application of OCR technology to extract data from forms. These forms can include contracts, surveys, applications, and medical records. Key Steps in OCR Form Processing OCR converts text images into machine-readable text by following these steps: Step 1: Scan and digitize the image. OCR scans and changes the text image into a digital format, like a bitmap or vector file. This makes a grid of pixels or points that show the shapes and colors of the image. Step 2: Enhance the image quality. OCR improves image quality and readability by using techniques such as noise reduction, binarization, skew correction, segmentation, and scaling. These techniques remove any things that are not needed, like dust, shadows, or background, and change the image’s direction, size, and quality. Step 3: Recognize the characters and symbols. OCR identifies the individual characters and symbols in the image by using methods such as template matching, neural networks, or machine learning. These methods match the shapes and patterns of the pixels or points with a list of known characters and symbols and pick the best one. Step 4: Correct and improve text accuracy. OCR corrects and improves text accuracy by using techniques like context analysis and natural language processing. These techniques check and fix any mistakes or differences in the text and use clues like the position, size, font, and meaning of the text to do this. Limitations of OCR Form Processing While OCR is a valuable tool, it is not perfect and can encounter some challenges while extracting data. The accuracy of OCR forms varies based on different kinds of forms: structured/fixed layout forms and semi-structured. Structured forms have a fixed and predefined layout and format, such as tax forms, surveys, or application forms. OCR works well with structured forms; however, its accuracy is never 100% while some OCR tools are capped at 95% accuracy. Therefore, you roughly lose 3% to 5% of your data if you use OCR for data extraction. On the other hand, semi-structured forms have a variable and dynamic layout and format, such as invoices, receipts, or contracts. Up to 80% of all forms are semi-structured. OCR form processing performs even worse when dealing with semi-structured forms. Therefore, OCR form processing compromises accuracy further. Some of the common mistakes that OCR can make are: Missing or reading wrong letters or numbers, especially symbols Mixing up letters or numbers that look alike, like O and 0, or l and 1 OCR depends on the quality of the input images, which can be affected by factors such as resolution, lighting, contrast, and noise. Poor quality images can lead to errors and misrecognition of text. OCR works best with standard fonts and the Latin alphabet. It may have difficulty recognizing unique fonts and non-Latin languages. OCR may struggle with documents that have complex or specific layouts and structures, such as multiple columns, tables, or graphs. These elements can interfere with the text recognition and segmentation process. OCR may have limited ability to recognize special characters and symbols, such as mathematical notations, currency signs, or emoticons. These characters and symbols may not be part of the standard alphanumeric set that OCR systems are designed for. These challenges can also affect the accuracy and reliability of the OCR results. Intelligent Form Data Extraction Intelligent form data extraction is a better alternative to form processing as it overcomes the limitations of OCR. Intelligent form data extraction uses artificial intelligence and machine learning to extract relevant data from various sources and formats, such as documents, images, or forms. It can also validate, verify, structure, and enrich the extracted data, making it ready for further use or integration. Data Validation and Verification Intelligent form extraction can check extracted data against predefined rules, databases, or external sources to ensure its accuracy and completeness. It can also flag any missing, incorrect, or inconsistent data for further review or correction. For instance, intelligent form extraction can verify the identity of a customer by comparing the data extracted from their ID document with their online profile or a third-party service. This can help prevent fraud, identity theft, or money laundering. Intelligent form extraction also offers higher accuracy than traditional OCR methods. OCR models don’t offer 100% accuracy; their accuracy ranges, whereas AI-powered template-based extraction can provide up to 100% accuracy. Therefore, a better approach would be to use AI-powered solutions simultaneously with OCR technology. Data Structuring and Enrichment Intelligent form extraction can organize the extracted data into a structured format, such as JSON, XML, or CSV, that can be easily integrated with other systems or applications. For example, intelligent form extraction can structure the data extracted from a contract into a table that shows the parties, terms, dates, and amounts involved. It can also add metadata, such as the source, format, and location of the contract. Intelligent form data extraction employs AI to enhance data quality. AI can apply techniques such as machine learning, natural language processing, computer vision, and deep learning to analyze, interpret, and validate the data from forms. Benefits of Intelligent Form Extraction The business benefits of leveraging intelligent form extraction are manifold, such as: Increased productivity and efficiency: Intelligent form extraction can reduce the time and effort required to process forms manually, allowing staff to focus on more value-added tasks. It can also increase the scalability of form processing, enabling faster and more reliable service delivery. A Forbes study revealed AI-based extraction techniques save around 30% to 40% of the hours spent on extraction tasks. Improved data quality and accuracy: Intelligent form extraction eliminates human errors, such as typos, misreading’s, or omissions, that can compromise the quality and accuracy of data. It can also ensure the consistency and standardization of data across different forms and sources. Enhanced data insights and analytics: Intelligent form extraction can unlock the value of data hidden in forms, by transforming it into a structured and usable format. It can also enable deeper and richer data analysis, by providing additional information, such as metadata, timestamps, or confidence scores. This can help businesses gain insights, make decisions, and drive innovation. Applications of Intelligent Form Extraction Intelligent form extraction can play a vital role in various industries, such as healthcare, insurance, banking, and supply chain, to streamline tasks like document review, contract analysis, invoice processing, and more. Examples of how intelligent form extraction can be applied in different domains include: Healthcare Intelligent form extraction helps healthcare providers and insurers automate the processing of medical forms, such as claims, prescriptions, lab reports, and patient records. This reduces manual errors, speeds up reimbursements, improves patient care, and ensures compliance with regulations. For example, intelligent form extraction can extract the diagnosis, treatment, and billing information from a claim form and match it with the patient’s medical history and insurance policy. It can also flag any discrepancies, such as overbilling, underbilling, or duplicate claims. One of the leading medical device manufacturers, Boston Scientific, has automated form processing, processing invoices, transmission summaries, preregistration processes, and inventory processing to improve customer service. Apart from monetary savings (US$240,000), it has resulted in error-free handling of medical data. Insurance Intelligent form extraction can help insurance companies streamline the processing of forms, such as policies, applications, claims, and renewals. This can enhance customer service, reduce operational costs, enhance fraud detection, and optimize risk management. For instance, intelligent form extraction can extract the personal, vehicle, and accident information from a car insurance claim form and compare it with the policy details and the damage assessment. It can also flag any suspicious or fraudulent claims, such as staged accidents, inflated damages, or false injuries. Banking Intelligent form extraction can help banks and financial institutions automate the processing of forms, such as loan applications, credit reports, identity documents, and statements. This can improve customer experience, reduce processing time, increase security, and comply with regulations. For instance, intelligent form extraction can extract the income, expense, and debt information from a loan application form and calculate the credit score and the loan eligibility of the applicant. It can also verify the identity and the financial history of the applicant by cross-checking the data extracted from their ID document and their bank statement. Intelligent form extraction can also save a significant amount of time and money for the finance team, by reducing the rework caused by human error. According to a report by Gartner, intelligent data extraction can save 25,000 hours of rework for the finance team caused by human error costing $878,000 annually for an enterprise with 40 members of an accounting team. Supply Chain Intelligent form extraction helps supply chain and logistics companies automate the processing of forms, such as invoices, purchase orders, delivery notes, and bills of lading. This improves efficiency, accuracy, visibility, and collaboration across the supply chain. Intelligent form extraction can extract the product, quantity, price, and delivery information from an invoice and match it with the corresponding purchase order and delivery note. It can also flag any discrepancies, such as missing, incorrect, or delayed deliveries. Harnessing the Power of Intelligent Form Extraction with LIKE.TG ReportMiner LIKE.TG ReportMiner is a powerful tool that enables you to capture, transform, and convert data from any form or document format. It combines the best of AI-driven automation and OCR capabilities to streamline your data extraction and integration workflows. Data Extraction from Various Formats: LIKE.TG ReportMiner can handle a wide range of document formats, including PDFs, PDF forms, PRN, TXT, RTF, DOC, DOCX, XLS, and XLSX. Whether it’s invoices, reports, or other structured or unstructured data, ReportMiner can extract relevant information efficiently. Advanced AI: ReportMiner leverages AI algorithms to automatically identify data fields within documents. It can intelligently parse names, addresses, and other critical data points. This ensures accurate extraction even from complex documents. Reusable Extraction Templates: With ReportMiner, you can create reusable extraction templates. These templates allow you to handle multiple files with similar formats consistently. By defining extraction rules once, you can achieve up to 100% accuracy in data extraction across various documents. OCR Integration: When dealing with scanned documents or non-extractable PDFs (which contain images), ReportMiner offers an OCR option. OCR allows it to process scanned copies and convert it into machine-readable text, which can be parsed via extraction templates. Data Validation and Quality Assurance: ReportMiner not only extracts data but also validates its quality. You can clean and correct any misread items or erroneous readings using the Edit Mode. This ensures that the extracted data is accurate and reliable. Don’t miss out on harnessing the potential of your unstructured data—try LIKE.TG ReportMiner today! You can download the free 14-day trial here or request a personalized demo here.
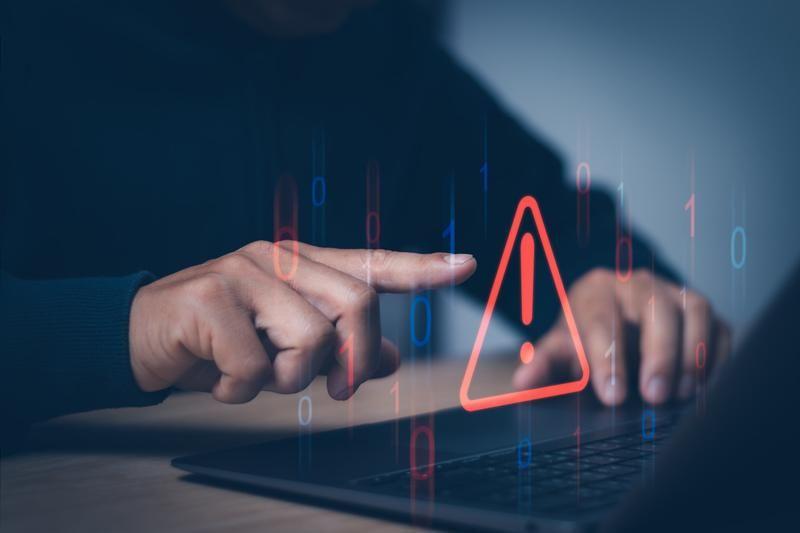
Transcript Processing with AI-Powered Extraction Tools: A Guide
The class of 2027 saw a massive influx of applications at top universities across the United States. Harvard received close to 57,000 applications for the class of 2027, while MIT received almost 27,000. UC Berkeley and UCLA, meanwhile, received 125,874 and 145,882 respectively. Manual transcript processing is an uphill battle for educational institutions at every level. With students’ academic futures at stake, admissions staff must quickly process every transcript, document, and form — ensuring accuracy and adherence to tight deadlines. When the sheer volume of applications is combined with short turnaround times, it can result in a concerning rise in mistakes, inefficiency, and delays. However, modern automated transcript processing solutions, with AI-driven data extraction at their core, are a powerful solution to this problem. Benefits of Automated Transcript Processing Faster Processing Manual handling, input, and processing of transcripts take considerably longer to complete, creating otherwise avoidable delays. Automated solutions can perform similar tasks in less time, improving efficiency. Fewer Errors Manual data processes are vulnerable to human errors, such as incorrect entries and wrong calculations to transposition mistakes. Automated transcript processing decreases errors and ensures more accurate transcript data. Better Scalability Manual transcript processing offers limited scalability. In contrast, educational institutions can readily scale automated transcript processing solutions as needed. This eliminates bottlenecks and enables smooth functioning. Resource Optimization AI-powered data extraction tools automate repetitive tasks, such as data entry and validation. This enables personnel to focus on more complex areas where human involvement is necessary—such as student counseling, curriculum development, and academic research. Compliance Regulations such as the General Data Protection Regulation (GDPR) and the Family Educational Rights and Privacy Act (FERPA) are applicable to academic institutions. AI-powered data tools help ensure compliance and keep data safe through measures such as anonymization and encryption. A Closer Look at AI-Powered Data Extraction AI-powered data extraction tools are ideal for automating transcription processing. They are less resource-intensive and require little to no human intervention. Automated data extraction and processing includes the following steps: 1. Data Identification and Access The process begins by identifying the sources of information, which range from documents and databases to web services and emails. Once identified, these sources are accessed through direct database connections, Application Programming Interfaces (APIs), or other methods for obtaining the data. 2. Data Extraction Different kinds of data extraction techniques are used depending on the purpose. Some of the most used extraction techniques used in education include: Pattern Matching: Pattern matching entails identifying specific patterns or sequences in data. In education, pattern matching enables data extraction from data sources like course syllabi, student records, or test scores, followed by trend identification in student performance and anomaly detection in assessment data. Natural Language Processing: NLP techniques allow the analysis and understanding of human language. In education, NLP helps with sentiment analysis of student feedback, educational content summarization, and automatic grading of written work. Named Entity Recognition: As a subset of NLP, NER entails identifying and categorizing named entities (such as people or locations) within text data. In education, NER can be used to extract author names from academic papers, names of institutions from a research paper, or student and faculty names from an administrative document. Machine Learning Models: Machine learning models include supervised, semi-supervised, and unsupervised learning algorithms. In the education sector, these models can be trained for predictive modeling, creating recommendation systems, performing clustering and segmentation, and topic modeling. 3. Processing and Validation Following extraction, data is parsed into a structured format for further processing or analysis, an example of which is filtering data based on some criteria. For instance, users can filter data to only see the details of students registered in 2023. Data quality checks are implemented to validate the data and ensure it’s aligned with what’s required. 4. Data Enrichment Data enrichment steps are optionally performed to enhance the extracted data. For example, text data is annotated, or extracted records are linked to external databases. 5. Output Generation In the final step, extracted and processed data is shared as a structured database, spreadsheet, or custom report. Customizable output formats ensure that the data remains usable for end users’ needs or downstream applications. What to Look for in an Automated Transcript Processing Tool 1. Accuracy Accuracy is the most important factor when working with academic transcripts. The right tool should be highly accurate in recognizing and processing transcripts. It should correctly capture information such as course titles, credits, grades, and other details to ensure reliability. 2. Robust Data Extraction Capabilities Features such as optical character recognition (OCR), template-based extraction, natural language processing (NLP) and data parsing algorithms signify that a tool has reliable data extraction processes. 3. Customization Options Customization options can configure a tool’s transcript processing workflow to individual requirements. Useful customization features include options to create custom data fields, modifying extraction parameters, and reconfiguring validation rules as needed. 4. Data Security and Compliance Adherence to rigorous data security standards and compliance regulations is a must-have for any automation tool. These tools process massive amounts of sensitive student information, and need to have encryption, access control and other security procedures in place to keep this information safe. 5. Ease of Use and User Interface Complicated tools are difficult to understand and use. For a transcript processing tool to have maximum usability, it should have features like an intuitive, user-friendly interface, drag-and-drop functionality and highly customizable workflows for simpler transcript processing and increased productivity. Take Your Transcript Processing Up a Notch Faster, error-free, scalable, and optimized. LIKE.TG's AI-powered data extraction capabilities don't just automate your transcript processing — they transform it! Learn more today. I Want to Start My FREE Trial Streamlining Transcript Processing Using LIKE.TG LIKE.TG is a no-code, automated solution simplifies data extraction, processing, validation, and transfer to various destinations. It can handle different kinds of documents—including transcripts. It uses a template-based extraction model to extract pertinent data from unstructured sources/documents. To do so, all it needs is a user-defined customized data extraction template, also called a Report Model. LIKE.TG’s no-code interface ensures that even the non-technical administrative staff in an academic institution can operate it easily. The outcome is a more streamlined and efficient transcript processing system. A Step By Step Guide to Extracting Data from Transcripts The Extraction Template The first step in processing transcripts using LIKE.TG is the creation of an extraction template. This template ensures that all pertinent information is accurately captured. For this use case, let’s start with the sample transcript (in PDF format) below: Loaded into LIKE.TG, the transcript above will look like this: After loading a document, template creation is a simple process where a user can indicate data regions (area captured within the source document) and fields on the document. This template instructs LIKE.TG about how it should process a document. The extraction template for our transcript will look like this: Data Preview LIKE.TG’s ‘Preview Data’ feature allows users to preview the output of the extraction template and verify that it’s functioning correctly. The data preview for the sample transcript will be as follows: Exporting The Transcript The Report Source object is using the transcript and the extraction template we designed to Once the extraction template is built and verified, we can run it and export the extracted data into a specified destination. In this use case, our destination is an Excel file. We are now working in a dataflow where we can use the external data in our data pipelines.We can process it further and load it into our desired destination. For the sake of simplicity, we are writing our extracted data to Excel via the Excel Workbook Destination object. Now the destination is configured to append transcript records to the same Excel file. To process multiple transcripts and write them to our consolidated destination automatically, we have designed our workflow with the File System Item Source Object (to access all the files inside our transcripts folder) linked to the Run Dataflow object in a loop, processing each transcript through our designed flow and writing them to the Excel file. Multiple transcripts processed and exported to Excel will appear as follows. Note that Excel flattens hierarchical data so if, for example, a student has four course records, the exported data in Excel will show four separate entries for each course, and each entry will have the student’s name. Processing The Data Data can be processed in different ways to generate new insights. Here, we are processing the consolidated transcripts data generated in the last step to view students’ average grades: Previewing such a data pipeline will show us the average grades and make additional information — such as the highest-scoring student — easily visible. Conclusion AI is here to stay, and automated transcript processing is quickly becoming essential for every academic institution. Educational institutions at every level can benefit tremendously from implementing AI-powered transcript processing into their workflows. Move to automated form processing with LIKE.TG and discover its benefits today. Request a quote or start your free trial now.
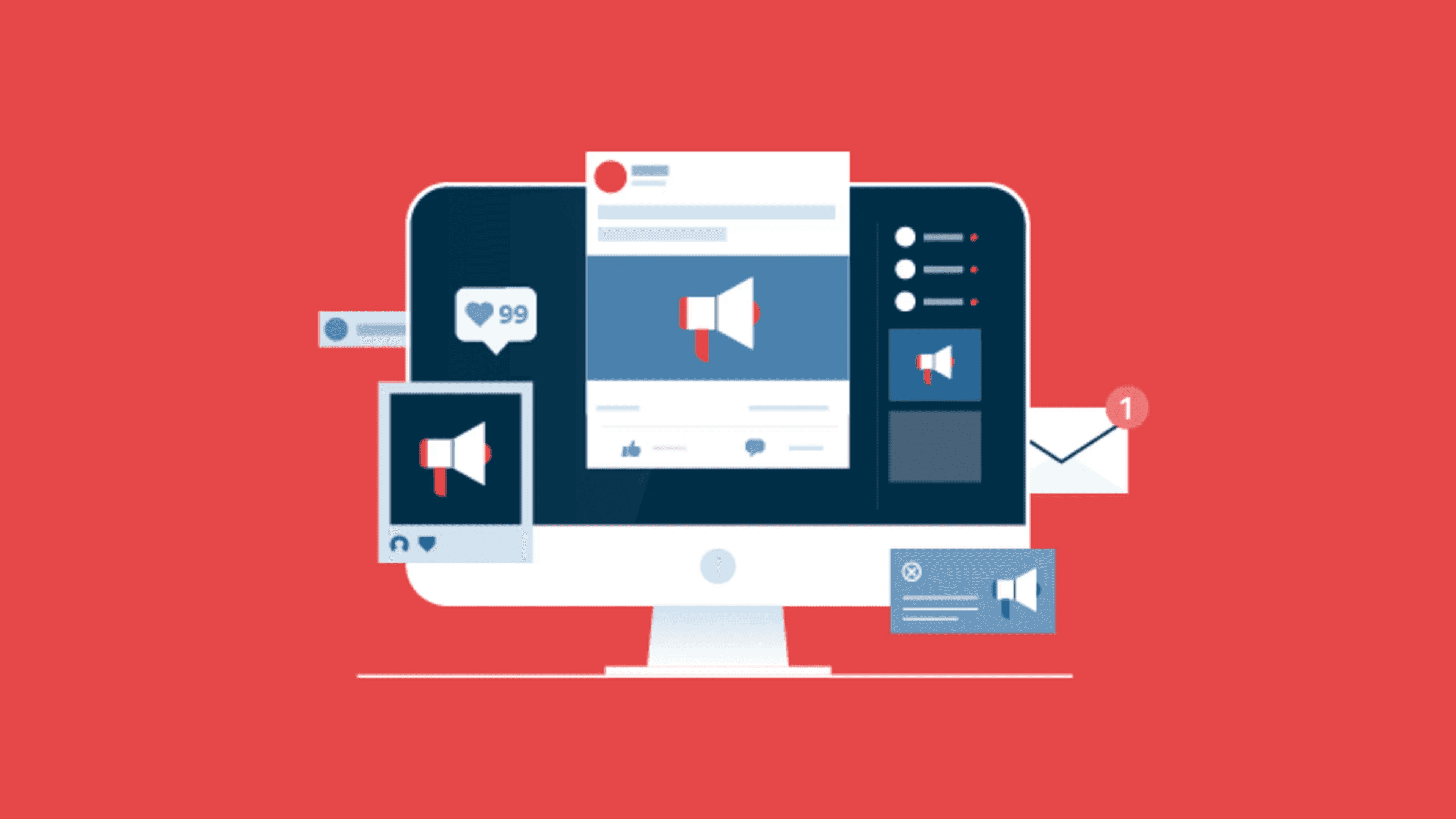
Simplifying Medical Claims Processing with LIKE.TG
Efficient medical claims processing is crucial for timely patient care and financial stability in healthcare. Processing claims swiftly and accurately ensures that healthcare providers receive prompt reimbursements, enabling them to maintain services, invest in staff training, and acquire advanced medical technologies. LIKE.TG is an advanced data extraction solution that is specifically designed to handle complex data extraction tasks, such as medical claims processing. It’s engineered to convert unstructured data, often found in medical claim forms, into structured, actionable information. Furthermore, LIKE.TG has an intuitive and user-friendly interface that allows users to ingest data from various sources, define extraction rules, and specify output formats without writing code. This makes it an ideal tool for healthcare providers and insurance companies who need to extract data from medical claims for analysis but do not have a technical background. Therefore, our solution expedites the claim settlement cycle, enhances operational efficiency, and benefits patients by facilitating quicker access to necessary healthcare services. Example Use Case: Medical Claims Processing An insurance organization processes hundreds of medical claims daily. Various healthcare providers submit these claims, each presenting crucial data such as patient details, diagnosis codes, and treatment procedures in unique formats. Traditionally, the administrative staff manually extracts this data, a process that is time-consuming and susceptible to human errors. LIKE.TG offers an automated and efficient solution to these challenges. With its rich set of features tailored for handling the complexities of medical claims data, it simplifies and automates the data extraction process. The solution enables the configuration of specific extraction parameters, allowing the system to intelligently identify and pull relevant information from various document types, including: PDFs. Excel sheets. Or even scanned documents. By choosing LIKE.TG, insurance organizations can significantly reduce the risk of errors and improve the claims processing time, thereby improving its overall efficiency and service quality. A Step-By-Step Guide to Streamlining Claims Processing As a large-scale insurer processing a substantial volume of medical claims daily, you encounter challenges with claims submitted by different healthcare providers containing critical data in diverse formats. You’re looking for a solution to efficiently extract, process, and analyze this data to expedite claims processing. Here’s how you can use LIKE.TG’s data stack to streamline your medical claims processing: 1. Receiving Claims The process starts when you receive healthcare claims on a regular basis from various healthcare providers. Each claim is a unique document, rich with vital information that needs to be accurately processed. These claims are promptly stored in a dedicated folder upon receipt. Healthcare Insurance Claim Form in PDF Format 2. Data Extraction Next, you can use LIKE.TG to automate the extraction of relevant data from these claims. LIKE.TG’s AI recommended layout feature allows you to automatically generate models for multiple source files at once. This can be achieved by specifying the data layout that needs to be extracted from the document, which can be done manually, through an existing LIKE.TG artifact, or in JSON format. This feature, coupled with its ability to handle different formats and complex hierarchical data structures, ensures accurate and efficient data extraction. Automating data extraction using LIKE.TG 3. Data Verification and Validation After the data is extracted, it is ready for validation. By employing the report model as a source within dataflows, you can effortlessly utilize LIKE.TG to ensure the accuracy, consistency, and integrity of the data through rigorous validation and verification processes tailored to meet its specific requirements. Verifying details from the insurer’s database Key steps in this phase include cross-verifying insurer details in the claim form with the company’s database, validating the requested claim amount against policy limits, and generating a unique claim ID for each new record. Data transformations in LIKE.TG 4. Workflow Automation The final step involves automating the entire workflow. Upon detection of a new claims file in the directory where all our unstructured claims appear, the dataflow, configured to use the source file path information, initiates real-time data extraction and transformation. Once processed, the file is moved to a designated folder, ensuring an organized workflow. To maintain efficiency, a scheduler is deployed to monitor the directory for unprocessed claims. LIKE.TG’s file drop automation feature ensures that the arrival of a new file automatically triggers the workflow, eliminating manual intervention and enhancing the overall efficiency of the claims processing operation. Scheduling claims file drop automation in LIKE.TG Utilizing LIKE.TG’s unstructured data extraction solution, each claim is processed in real time, ensuring all claim records are swiftly and accurately added to the repository. Medical claims data is consolidated in an Excel File On structured, consolidated claims records, you can leverage LIKE.TG’s advanced data preparation capabilities to prepare it for analysis. This involves cleansing the data to remove any inaccuracies, filling in missing values, and eliminating duplicates. LIKE.TG provides instant data preview, allowing you to modify and monitor the data in real-time. Moreover, its profile browser, displayed as a side window, allows you to assess the data’s health, ensuring that it is clean, accurate, and ready for analysis. Data profiling and preparation using LIKE.TG Benefits of LIKE.TG in Medical Claims Processing LIKE.TG offers many benefits that significantly simplify the process of medical claims processing: Automation: LIKE.TG plays a pivotal role in automating the data extraction process within medical claims processing, reducing the reliance on manual efforts. By streamlining the handling of diverse medical data formats, the tool introduces efficiency into a traditionally time-consuming task, allowing healthcare organizations to process claims with greater speed and accuracy. Enhanced Accuracy: The intelligent extraction capabilities of LIKE.TG significantly enhance the accuracy of medical claims processing. The tool is adept at precisely identifying and retrieving relevant information from various document types, minimizing the risk of errors that could impact the integrity of critical data points, including patient details, treatment histories, and billing codes. Efficiency Gains: LIKE.TG contributes to efficiency gains by accelerating the overall claims processing timeline. This not only expedites the reimbursement cycle but also frees up valuable resources that would otherwise be dedicated to manual data entry. The result is a more streamlined and resource-efficient workflow for healthcare providers. Time Savings: The rapid and consistent data extraction facilitated by LIKE.TG translates into significant time savings for healthcare organizations. The automation of labor-intensive processes enables staff to allocate their time more strategically, focusing on tasks that require human expertise and contributing to a more effective and agile healthcare environment. Adaptability: LIKE.TG’s adaptability to diverse data formats commonly encountered in the healthcare sector, including PDFs, Excel sheets, and scanned documents, underscores its versatility. By ensuring seamless integration with existing systems, LIKE.TG proves to be a robust solution that aligns with the dynamic nature of medical claims data and supports the interoperability of various data sources. Final Word LIKE.TG is a powerful tool that empowers healthcare providers to streamline their medical claims processing. Its suite of features, including automated data extraction, advanced pattern recognition, and AI-recommended layouts, are designed to enhance productivity, ensure accuracy, and simplify workflows. By reducing manual effort, minimizing errors, and accelerating claims processing times, LIKE.TG allows healthcare providers to focus on what truly matters — delivering quality patient care. Take the first step towards a more efficient and effective medical claims processing system with LIKE.TG by downloading its free 14-day trial today. Experience Hassle-Free Medical Claims Processing with LIKE.TG ReportMiner Unlock the power of automated data extraction with LIKE.TG ReportMiner. Simplify your medical claims processing, reduce errors, and save valuable time. Try LIKE.TG ReportMiner free for 14 days and see the difference it can make. Start Your Free 14-Day Trial
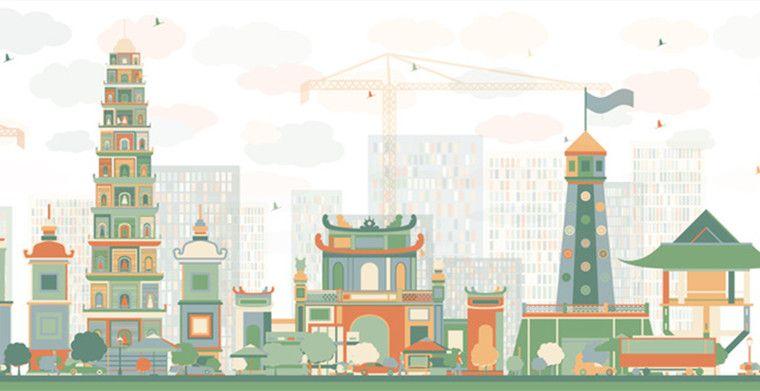
Snowflake ETL Tools: Top 7 Options to Consider in 2024
Snowflake has restructured the data warehousing scenario with its cloud-based architecture. Businesses can easily scale their data storage and processing capabilities with this innovative approach. It eliminates the need for complex infrastructure management, resulting in streamlined operations. According to a recent Gartner survey, 85% of enterprises now use cloud-based data warehouses like Snowflake for their analytics needs. Unsurprisingly, businesses are already adopting Snowflake ETL tools to streamline their data management processes. In this blog, we will explore the top Snowflake ETL tools that help businesses extract, transform, and load their data efficiently into the Snowflake Data Platform and derive actionable insights. What is Snowflake ETL? According to a study conducted by Forrester Consulting in 2023, organizations that adopted Snowflake ETL experienced a significant improvement in their analytics capabilities. Specifically, the study found that these organizations achieved a 45% reduction in data integration time and a 60% increase in the speed of generating insights compared to those using traditional ETL processes. Let’s discuss how the Snowflake ETL process works: 1. Data Extraction with Snowflake The first step, extraction, involves collecting data from multiple sources. These sources can range from traditional databases to SaaS platforms and even unstructured data. Snowflake allows direct data ingestion from these sources into its staging area. This is possible through Snowflake’s support for various data formats like JSON, Avro, XML, Parquet, and more. The platform’s ability to handle semi-structured data simplifies the extraction process, as it eliminates the need for pre-processing or converting data into a specific format. 2. Data Transformation with Snowflake Once data is in Snowflake, the next step is Transformation. This step is about converting the extracted data into a format or structure that is more suitable for analysis. Snowflake facilitates this process through its SQL interface, enabling users to perform complex transformations using familiar SQL queries. Snowflake’s unique architecture, which separates compute and storage, allows these transformations to occur in a highly efficient manner. Users can scale up or down the computational resources (warehouses) as needed, ensuring that transformations are both fast and cost-effective. 3. Data Loading with Snowflake The final step is Loading the transformed data into Snowflake’s storage layers for analysis and reporting. Snowflake’s architecture ensures that once data is loaded, it’s immediately available for querying. This is facilitated by the automatic handling of indexing and optimization, which removes the traditional administrative overhead associated with managing a data warehouse. What are Snowflake ETL Tools? Snowflake ETL tools are not a specific category of ETL tools. Instead, the term Snowflake ETL tools refers to using specialized tools, software solutions, and processes in conjunction with the Snowflake data platform for data extraction, transformation, and loading. 1. Extract The first step in the Snowflake ETL process involves extracting data from multiple sources such as databases, cloud storage platforms, APIs, and streaming services. ETL tools for Snowflake provide built-in connectors and APIs that allow organizations to extract data from these sources efficiently. Various tools use different techniques for extracting data, such as incremental extraction that fetches only new or updated data since the previous extraction, and full extraction, which retrieves all the available data from the source. Some tools also offer delta extraction, which allows the extraction of only the changed data since the last extraction, thus optimizing the process and reducing resource usage. 2. Transform Once the data is extracted, it undergoes transformation to meet the specific requirements of Snowflake data platform and the analytical use cases. Snowflake ETL tools offer a wide range of transformation capabilities, allowing organizations to tailor their data to suit their analytical needs. Transformations mostly include cleaning, filtering, aggregating, joining, and enriching the data to ensure its accuracy, consistency, and relevance. Snowflake ETL tools also assist in data mapping by providing functions to map source data fields to corresponding fields in the Snowflake data platform. This guarantees that data is accurately transferred and aligned with the designated schema within Snowflake, allowing for smooth integration and analysis. By offering intuitive mapping interfaces and features, these tools simplify the mapping process, enabling users to efficiently configure data mappings and maintain data integrity throughout the ETL workflow. 3. Load Finally, the data is loaded into Snowflake database tables, where it can be stored, managed, and analyzed. Snowflake ETL tools provide mechanisms for loading data into Snowflake tables using different techniques, such as bulk and parallel loading. Why Incorporate a Third-Party Snowflake ETL Tool? While Snowflake supports ETL processes natively, incorporating a third-party ETL tool for Snowflake offers several advantages: Automation: Many Snowflake ETL tools provide advanced automation capabilities, simplifying the scheduling and management of ETL pipelines. This reduces manual effort and increases efficiency. Pre-built Connectors: Third-party ETL tools for Snowflake often come with a wide range of pre-built connectors for various data sources and destinations, streamlining the integration process. Transformation Capabilities: Some tools offer powerful transformation capabilities, including visual data mapping and transformation logic, which can be more intuitive than coding SQL transformations manually. Monitoring and Error Handling: Snowflake ETL tools typically provide monitoring and error handling features, making it easier to manage the ETL process and ensure data quality. Security and Compliance: They often include built-in security features and compliance standards that help protect sensitive data and meet regulatory requirements. Benefits of Using Snowflake ETL Tools Snowflake ETL tools provide several benefits that help organizations effectively manage their data integration procedures, which in turn enables them to extract valuable insights from their data. Seamless Data Integration Snowflake readily accepts incoming data from cloud storage solutions, enabling organizations to integrate data from diverse sources seamlessly. For example, a retail company can effortlessly import sales data stored in cloud storage directly into Snowflake for analysis and reporting without the need for complex processes during data movement. Flexible ETL Initiation Automation features such as Cloud Messaging and REST API endpoints in Snowflake enable continuous data loading and flexible ETL initiation, enhancing efficiency and coordination. For instance, a healthcare provider can automate the extraction of patient data from an external system using Snowflake’s Cloud Messaging feature, checking that the data is continuously loaded into Snowflake for real-time analytics and reporting. Simplified Integration Integration with existing applications and analytics tools is simplified through Snowflake’s REST API endpoints and intuitive web interface. For example, a marketing team can seamlessly connect Snowflake to their preferred analytics tool, using Snowflake’s REST API endpoints, enabling them to visualize and analyze data directly within their familiar analytics environment without requiring manual data exports or imports. Transparent Billing Model Snowflake’s transparent billing model separates compute and storage costs, enabling businesses to control spending based on their specific needs and usage patterns. For instance, a financial services firm can monitor and adjust their compute and storage resources in Snowflake to optimize costs based on fluctuating data processing requirements, making sure that they only pay for the resources they consume. Advanced Security Features Advanced security features such as encryption and authentication in Snowflake ensure data protection and compliance with industry regulations. For example, a government agency can encrypt sensitive citizen data stored in Snowflake to safeguard it from unauthorized access, while also implementing multi-factor authentication to ensure that only authorized users can access the data, thereby maintaining compliance with regulatory requirements. Regular Innovation Snowflake’s commitment to innovation and regularly adding advanced features provide users with access to cutting-edge capabilities for enhanced data analytics and insights. For instance, Snowflake regularly releases updates and enhancements to its platform, such as new data processing algorithms and integrations with emerging technologies, empowering organizations to stay ahead of the curve and leverage the latest advancements in data analytics. 7 Best Snowflake ETL Tools The following ETL tools for Snowflake are popular for meeting the data requirements of businesses, particularly those utilizing the Snowflake data warehouse. 1. LIKE.TG LIKE.TG is a comprehensive data management platform designed to simplify and streamline enterprise data operations, with a strong focus on compatibility and integration with multiple databases, data warehouses, and cloud platforms, including Snowflake. With its unified approach to data management and extensive features, LIKE.TG empowers businesses to utilize Snowflake for efficient data processing and analytics. Key Features of LIKE.TG Data Stack You can connect natively to a wide range of data sources and destinations, both on-premises and in the cloud, ensuring flexibility in your data integration efforts. Transform and shape your data according to your business needs using pre-built transformations and functions without writing any code. Automate data quality management to ensure only high-quality data reaches your data warehouses, cleansing, de-duplicating, and validating data based on your business rules. Seamlessly automate and orchestrate your data integration workflows, reducing manual intervention and streamlining operations. Use the visual drag-and-drop interface to easily and quickly build ETL and ELT pipelines, enabling you to set up data pipelines within minutes. Leverage AI-powered data extraction capabilities to pull data from unstructured sources like PDFs, text files, and spreadsheets, enhancing your data collection process. Access built-in connectors for a variety of file formats, databases, cloud storage options, data warehouses including Snowflake, and data lakes, as well as support for various applications through native and custom connectors, broadening your integration capabilities. Benefit from advanced transformations, an AI-powered auto mapper for efficient field mapping, and powerful data quality features to cleanse and ensure the integrity of your data, all through simple drag-and-drop operations in the data flow designer. 2. Matillion Matillion is an ETL tool, offering seamless integration with platforms like Amazon Redshift, Google BigQuery, and Snowflake. It provides data transformation capabilities and orchestration for data loading. Pros With Matillion ETL, users can perform complex data transformations using custom SQL or by creating transformation components via an intuitive GUI. Matillion supports multiple data sources including databases, CRM platforms, ERPs, and more. Customer support is available through online ticketing and phone assistance, ensuring prompt assistance whenever you need it. Cons Setting up some connections can be complex, and upgrades often necessitate creating a backup instance, complicating the process further. The lack of direct documentation for new features forces you to search through FAQs or discover them independently, hindering your ease of use. It lacks pre-made templates, so users must start building everything from scratch, which can be time-consuming. 3. Stitch Stitch is a cloud based ETL platform that aims to simplify data ingestion from various SaaS applications and databases into data warehouses and data lakes. Stitch also offers solutions for non-technical teams to quickly set up data pipelines. Pros Users can define transformations required for compatibility with Snowflake destinations, such as denesting data and translating data types, using Python, Java, SQL, or a graphical user interface. The platform allows users to schedule data loading at predefined times, maintaining timely updates of tables within Snowflake. Users can add new data sources to the system autonomously. Cons It offers limited data transformation capabilities and requires a Singer tap for on-premises SQL Server support, restricting flexibility. It supports a restricted number of destinations, capped at one, three, or five, depending on the subscription tier, limiting scalability. Replication of data from a single source to multiple destinations is difficult with Stich. 4. Apache Airflow Apache Airflow is a widely used open-source ETL tool for Snowflake, offering powerful capabilities for monitoring, scheduling, and managing workflows through a web application interface. Pros DAG visualizations and task trees offer you insights into the logical flow of your workflows, enabling you to monitor and manage your pipelines effectively. The open-source nature of Apache Airflow allows you to leverage a vast community and extensive documentation for setup, troubleshooting, and support. You can enhance your Airflow workflows with custom functionality using Python, enabling seamless integration with Snowflake and other systems. Cons Requires extensive user setup and technical expertise, making it challenging for a non-technical user. Mandates Python knowledge for creating data pipelines in Airflow, limiting accessibility if users lack programming skills. It’s difficult to modify pipelines once they’re created. 5. Integrate.io Integrate.io is a data integration tool designed to provide an integration layer for data warehouses, including Snowflake. Offering a no-code data pipeline platform, Integrate.io supports various data integration techniques such as ETL, ELT, CDC, and Reverse ETL. Pros Integrate.io offers the ELT data replication functionality, enabling real-time data synchronization with updates as recent as 60 seconds ago. The drag-and-drop, no-code interface simplifies the process to define transformations and connect data sources, making it accessible to users who have varying levels of technical expertise. Integrate.io provides a support team to assist users with any queries or issues they may encounter during implementation and operation. Cons Error messages during setup may be unclear for users, making it difficult to diagnose and fix issues without detailed guidance. The software requires development experience for users to use effectively, posing challenges especially if they are a beginner. As the user creates additional pipelines or increases their complexity, the interface becomes more complicated 6. Fivetran Fivetran allows users to replicate applications, databases, events, and files into high-performance cloud warehouses, including Snowflake. Pros Offers easy setup and automated data pipelines that require minimal maintenance, enabling users to focus on analytics. Supports SQL modeling with defined schemas and Entity-Relationship Diagrams (ERDs). Fivetran can be run in fully managed, hybrid, or self-hosted environments, accommodating different organizational needs and policies. Cons Lacks support for Kinesis stream as a data source and serverless Aurora, limiting its applicability for specific use cases. Does not support “before delete” triggers, restricting flexibility in data management and automation. It’s tricky to determine the final cost of the platform. 7. StreamSets StreamSets is a cloud-first, fully managed ETL tool that allows users to build enhanced data ingestion pipelines for continuous data delivery required for analytics, including Snowflake. Pros Provides an intuitive user experience with a drag-and-drop GUI for performing data transformations before loading into Snowflake. Allows to add new data sources and write custom data processors in JavaScript, Groovy, Scala, etc., providing users with flexibility and extensibility in data processing. Offers extensive product and operational documentation on its website, along with customer support through online ticketing and call-based assistance. Cons Logging may seem complicated and copying the same pipeline to a different server often leads to library dependency issues, complicating the deployment process. Establishing connectivity between a local Docker instance and external applications can be challenging, hindering seamless integration. When trying to diagnose issues, it can be challenging to sift through complex logging and error messages. Choosing the Right Snowflake ETL Tool When choosing a Snowflake ETL tool, several factors and features should be considered to ensure the tool meets the specific requirements: Paid or Open Source When deciding on a Snowflake ETL tool, the first thing to think about is whether to pay for it or use a free, open-source option. Paid tools usually come with more features and support. Open-source tools are free and can be a good choice for those just starting out with Snowflake ETL. Ease of use Ease of use is another important factor to consider. Some Snowflake ETL tools are made for tech experts, while others are designed for people with less technical know-how. Picking a tool that matches the team’s skill level can make setting up data warehouse quicker and easier. Ability to move Data When an organization uses a variety of data sources, it is important to select a Snowflake ETL tool that can handle data from different sources. Certain tools work well with specific types of data, while others can manage multiple sources. Selecting a tool that supports diverse data sources guarantees comprehensive data integration, which enables better data analysis. Option for Adding/Modifying Data Sources As the organization grows, it should look for a Snowflake ETL tool that enables easy addition and modification of data sources. This flexibility ensures that the data warehouse can adapt to the changing needs of the organization over time. Ability to transform the Data It is imperative to find the right tool that can handle specific transformation needs. The Snowflake ETL tools offer a wide range of options, making it easy to tailor the data to suit specific analytical needs. A tool with sturdy data transformation capabilities can deliver accurate insights and enable businesses to make informed decisions. Pricing When selecting an ETL tool, businesses must consider the cost of the tool. While some ETL tools are available for free, others come with a price tag. To manage financial resources effectively while still securing necessary ETL functionalities, businesses should choose a tool that fits their budget and provides the features they require. Product documentation When a user is trying to implement a new tool, one of the most important factors that can impact their success is the quality of the documentation available. Tools that have clear and helpful documentation make it easier for users to get started quickly, reducing the time needed to learn how to use them. Customer support When evaluating a Snowflake ETL tool, it’s important to consider the quality of customer support. Reliable support is key in resolving any issues while using the tool. Therefore, it’s essential to look for a tool with a reputable and responsive customer support team that can help resolve any problems promptly. This ensures that operations can continue without any interruption and data remains protected. Concluding Words The increasing reliance on cloud-based data warehouses like Snowflake highlights the importance of effective ETL tools. Among the leading solutions, LIKE.TG stands out for its user-friendly interface and advanced features. Businesses can explore LIKE.TG’s data integration capabilities firsthand with a complimentary 14-day trial. Experience how LIKE.TG streamlines data processes, enabling efficient insight extraction. Explore LIKE.TG today and schedule a demo to enhance your data management journey. Seamlessly Transform the Data Looking to simplify the complex process of Data Transformation? Try LIKE.TG for free for 14 days and optimize your ETL. Experience how LIKE.TG streamlines data processes, enabling efficient insight extraction. Start a Free Trial
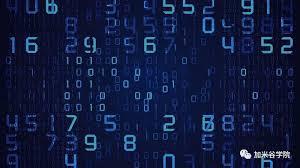
A Simple Guide to Medical Insurance Claims
Insurance companies and third-party administrators are increasingly turning to automated data extraction to expedite the processing of medical insurance claims. This approach serves as a better alternative to time-intensive manual claim management. Leveraging AI technology allows them to efficiently extract crucial data from documents, eliminating manual data entry errors and significantly reducing processing times. Moreover, automation streamlines workflows, enabling quicker reimbursements and enhancing overall efficiency. With the ability to handle large volumes of claims with accuracy and speed, automated data extraction is a consistent, reliable, and effective choice. If you want to harness the power of AI to drive success, keep on reading, as we’ll guide you through medical insurance claim processing and how AI can help simplify and optimize them. Understanding Unstructured Data in Medical Claims What is a Medical Insurance Claim? A medical insurance claim is a bill that healthcare providers submit to the patient’s healthcare insurance company after they receive treatment or care. These documents contain details about diagnosis, procedure, pharmaceuticals, medical supplies and devices, and medical transport. At its core, a medical insurance claim begins with a healthcare provider submitting a formal request for payment to the patient’s insurance company or TPA responsible for claims processing. Types of unstructured documents used in medical insurance claims include: Explanation of Benefits Explanation of Benefits (EOB) is a statement that outlines the details of the claim, including the amount billed by the healthcare provider, the allowed amount (as per the insurance plan), co-payments or coinsurance amounts, and the portion covered by the insurance company. This document helps policyholders understand their financial responsibilities. Despite its significance, the EOB lacks a uniform format, leading to considerable variability across providers and insurance companies. Statement of Physician A Statement of Physician is a detailed document, typically prepared by a physician or specialist, outlining the services provided during a patient’s visit or treatment.This document includes the nature of the services rendered, such as examinations, procedures, medications prescribed, diagnostic tests conducted, and other relevant medical interventions. These physician statements are typically manually written and lack standardization, which makes it difficult to extract reliable, relevant information. Medical Bills Medical bills are the invoices or statements healthcare providers issue after providing care. This document includes details of the charges for the services rendered. They have itemized lists of medical procedures, medications, treatments, and diagnostic tests the patient gets throughout the visit or treatment. Lab Reports Lab reports are essential for monitoring and diagnosing medical conditions. These documents include free-form text entries by medical professionals describing observations, interpretations, test results, and recommendations. They also contain important information like diagnostic interpretations and patient demographics. Lab report designs vary among service providers, lacking standardized formatting and organization. Why is Automating Medical Insurance Claims Processing Important? The adoption of automated medical insurance claims processing solutions emerges as a strategic imperative due to the following factors: Escalating Data Volumes The rapid increase in digital healthcare data has overwhelmed manual processing methods. Medical insurance claims involve various documents, like electronic health records and scanned invoices, some of which are semi-structured or unstructured, contributing to a surge in data volumes. Manual processing struggles to keep up with this deluge, hence resulting in processing delays and mistakes. Without automated systems to effectively handle this variety of data, the healthcare industry may encounter difficulties in ensuring insurance claims are processed promptly and accurately. Complexity of Unstructured Data Medical insurance claims often contain unstructured data, such as unorganized text fields and inconsistent formats. Further complexity arises from the diverse sources of information, including invoices, medical records, and correspondence, each having its own layout and structure. Human interpretation of such data is prone to errors and inconsistencies, leading to inefficiencies in claim processing and adjudication. Automated solutions leveraging machine learning is a need in managing unstructured data within medical insurance claims as it ensures accurate extraction of data for further processing and decision making. Demand for Expedited Processing As customers expect faster and more efficient responses, insurance companies must recognize the need to overhaul their systems. Traditional claim processing methods are reliant on human intervention and, hence, tend to be slow and time-consuming. These prolonged processing times result in customer dissatisfaction. Automated solutions offer a rapid turnaround by streamlining the extraction and validation of claim data, thereby enhancing operational efficiency and customer experience. Effective Techniques for Extracting Data from Medical Claims Companies have numerous choices for extracting data from medical insurance claims through AI. Each method offers unique value, improving accuracy and empowering companies to streamline their processes. Let’s take a look at some available techniques and how they work. Optical Character Recognition (OCR) OCR technology is important forconverting scanned documents, such as medical bills and invoices, into machine-readable text. It recognizes characters and symbols in images, making it easier to extract from scanned documents for data analysis and processing. Natural Language Processing (NLP) NLP techniques help automated systems understand human language, including specialized medical terminology and free-form text. By analyzing the context and semantics of textual data, NLP algorithms effectively extract important information from unstructured sources like medical notes and reports, improving accuracy and efficiency in data extraction. Machine Learning (ML) ML algorithms help automated systems understand data patterns and make predictions or decisions without explicit programming. In the context of medical insurance claims, ML algorithms can identify and extract crucial information such as patient demographics, diagnoses, procedures, and billing codes from diverse data sources. While ML itself does not guarantee high accuracy, template-based solutions like Report Miner ensure the most accurate results needed for medical claim processing. Robotic Process Automation (RPA) RPA technology automates repetitive and rule-based tasks by mimicking human actions within digital systems. In medical insurance claims, RPA bots handle tasks like data entry, validation, and reconciliation. This reduces manual workand speeds up claim processing workflows, hence improve operational efficiency. How Automated Solutions Streamline Medical Insurance Claims Handling? Automated data extraction solutions significantly improvemedical insurance claims processing by expediting and enhancing the entire workflow. These solutions offer several advantages, including: Improved Data Quality: Automated solutions ensure higher data quality by minimizing errors and inconsistencies associated with manual data entry. Advanced technologies such asnatural language processing and template-based extraction to enhance accuracy in extracting and interpreting data from various sources, resulting in more reliable information for claims processing. Enhanced Compliance: Automated solutions facilitate adherence to regulatory requirements and industry standards. By systematically applying rules and validations, these systems ensure that claims data meet compliance standards, reducing the risk of penalties and legal issues. Accelerated Claim Settlements: Advanced technologies have significantly reduced the standard claim processing time from 60-90+ days to just 30 days for most health insurance companies. This remarkable reduction ensures policyholders receive reimbursements swiftly, easing financial burdens and improving overall satisfaction with insurance services. Best Practices to Process Medical Insurance Claims When talking about validation and automation, it’s always good to talk about the context of our solution. For example, some of the best practices can be: Leverage AI Technology Employ AI-powered data extraction and automation tools designed specifically for medical insurance claims processing. Such tools not only streamline the workflow but also enhance accuracy by minimizing human errors. Moreover, consider choosing template-based solutions as they leverage AI techniques effectively and hence ensure 100% accuracy in medical claim processing. These solutions analyze patterns in documents and extract relevant information with great precision. Provide Effective Staff Training Develop comprehensive training programs for staff members, particularly those involved in claim processing. They should understand the intricacies of claim procedures, compliance requirements, and the utilization of AI solutions effectively. Other than that, a no-code data extraction solution can be implemented to train staff with non-technical backgrounds. This allows them to handle and process medical claims effectively. Implement Data Validation Rules Establish regular review processes for processed claims to identify inconsistencies and errors. Furthermore, implement quality control measures to address issues efficiently and maintain data integrity. It’s wise to invest in modern automation solutions as they are equipped with data quality rules. These rules are designed to minimize error rates by ensuring the accuracy and completeness of extracted data. Continuous Regulatory Monitoring Establish strategic mechanisms to monitor regulatory updates continuously and stay vigilant about any changes in medical claim processing regulations and compliance standards. Companies can implement agile processes to adapt quickly to regulatory changes, hence minimizing the risk of non-compliance issues or delays in claim processing. ReportMiner As a Leading Tech-Solution for Healthcare Providers When speaking of automated solutions, we’ve approaches like LIKE.TG’s ReportMiner that assist in extracting unstructured data from diverse formats. ReportMiner extracts data from different types of PDFs such as text files, spreadsheets, and fillable forms. Following is some of its key features: AI Powerered Data Extraction The AI – Powered data extraction feature within the Report Miner streamlines the creation of medical insurance claims efficiently. The end-to-end flow automates the extraction process, significantly reducing the time required to create extraction templates from scratch. With AI-recommended layouts, the software utilizes AI to generate extraction templates as per your specific data layout needs. This means you no longer have to spend hours manually crafting templates; instead, the tool identifies and creates templates based on your required data layout, ensuring seamless process. Versatile Data Extraction Methods Report Miner simplifies the extraction process by using various methods such as text-based, pattern matching, and table extraction. Text-based extraction efficiently extracts crucial details, like those found in medical reports, facilitating seamless integration into claims systems. Pattern matching identifies specific data formats, streamlining the collection of essential information for claim assessment. Moreover, table extraction simplifies the retrieval of data from tables within documents, ensuring accurate capture for claims processing. This, combined with the tool’s pattern-based template extraction feature, enables users to effortlessly extract data from unstructured documents by designing templates that guide the extraction process. Additionally, Report Miner now offers an AI-powered data extraction feature, automatically generating extraction templates by identifying patterns within documents using AI, further enhancing efficiency and accuracy. Multiformat Compatibility The software supports data extraction by supporting from a variety of file formats, including PDFs, text files, word documents etc.Report Miner uses advanced parsing and pattern matching algorithms that can extract relevant information from different data fields. This compatibility makes claim processing easier, more accurate and efficient. Conclusion Over the years, the medical insurance industry has undergone a revolution with modern technology solutions. Among the approaches we have LIKE.TG ReportMiner for handling unstructured data in claims processing. The ability to transform raw data into actionable insight makes it a fantastic tool for insurance companies. In the following part, we’ll discuss how RM works, and how you can leverage its potential to optimize a variety of documents. Don't Let the Potential of Your Unstructured Data to Remain Untapped Extract valuable insights from medical insurance claims effortlessly with LIKE.TG. Start Trial
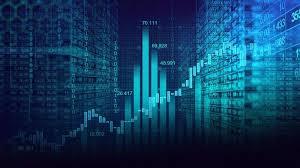
Optimizing Auto Insurance Claims Processing with LIKE.TG
The car insurance industry today is addressing the challenge of managing vast amounts of unstructured data through innovative solutions. A leading insurer in the Asia-Pacific anticipates significant improvements through Generative AI over the next 12 months to enhance auto insurance claims processing efficiency and accuracy. LIKE.TG is a powerful tool that uses AI to make processing claims easier and more accurate.
With LIKE.TG, insurance companies can quickly sort through and analyze important information from various sources, speeding up the entire claims process.
Simplifying Claims Processing
LIKE.TG simplifies the complex process of data extraction, specifically unstructured data, in claims processing. With its code-free interface, LIKE.TG makes data extraction accessible to business users.
The core functionality of LIKE.TG relies on a template-based extraction model. Users can create Report Models or Extraction Templates that guide the software in identifying and extracting necessary data from various unstructured sources, such as scanned documents, fillable PDF forms, and text documents related to car insurance claims. This approach streamlines the data handling process through automation.
By leveraging LIKE.TG, businesses can transform unstructured claims data into a structured format. This is critical for speeding up the claims resolution process, improving accuracy, and ultimately enhancing customer satisfaction.
Key Features of LIKE.TGfor Auto Insurance Claims Processing
LIKE.TG ReportMiner extracts information from complex claims documents, like PDFs of repair estimates or text documents carrying information about property damage. Businesses can use LIKE.TG ReportMiner to automate the tedious process of parsing various PDF documents, reducing the need for manual data entry operations.
AI-Powered Extraction
LIKE.TG ReportMiner uses AI to identify required fields within claim forms automatically, enhancing the extraction process. This AI-driven approach not only identifies but also intelligently evaluates the context and patterns in the data.
By automating data extraction from PDFs with AI, LIKE.TG ReportMiner eliminates the guesswork and manual effort traditionally involved in identifying and extracting key data points, streamlining the claims processing workflow.
Automated Workflows
LIKE.TG ReportMiner offers comprehensive automated workflow capabilities that cover the entire claims processing workflow from data extraction to decision-making. This includes automating the extraction pipeline to run on batches of PDF files, thereby making all desired information available promptly and efficiently.
Efficient Template Based Extraction
For text-based PDFs, users can create an extraction template using specific patterns present in the document, guiding LIKE.TG ReportMiner to accurately retrieve information. In the case of scanned PDFs, ReportMiner’s OCR capabilities transform these documents into text-based formats for building extraction templates.
Additionally, for form-based PDFs common in business operations, ReportMiner simplifies the extraction of business data for further reporting and analysis.
Once data is extracted, it can be transformed and exported to various destinations, including Excel spreadsheets, databases, and CSV files, facilitating a seamless integration into an organization’s existing data ecosystem.
Step-by-step Guide to Streamling Claims Processing
In this example, LIKE.TGstreamlines the process from initial data extraction to claim resolution, taking a batch of car insurance claims as the use case. Each claim in this batch comes with detailed damage information n PDF format.
Step 1: To begin the process, load the PDF form into LIKE.TG ReportMiner’s designer.
Step 2: Define extraction template by identifying and specifying the patterns that occur within the source report. These patterns will be identical for all files in our batch. which is why we will be able to use one extraction template on all files.
Step 3: In the field properties section, adjust the dimensions and positioning of data fields. For example, in the case of the Address Field, we defined it to follow string Address in the current line.
Step 4: After specifying the data fields and the region and their positions to capture in the report model, preview the extracted data output in the data preview window.
The claims information is now in structured format and can be stored in an excel sheet for further analysis.
Step 5: To further automate the process of the structured claims data, add this report model to dataflow, where we can build data pipelines.
Step 6:The Lookup object is looking up the insurance amount in the database against the Loss Category value in the extracted claim data. We are including this amount in a new field in the claims record
Also check if a claim is valid. For instance, in this case if a loss isn’t in the listed categories, the claim is marked invalid, and the system won’t assign any amount to it. In such cases, instruct the system to record an error.
Step 7: Once the Dataflow runs, we can see the process detects an error in one of the claims, resulting in the non-assignment of insurance amount and marking the validity as 0.
Step 8: Currently, this data processing is for a single file. To handle an entire batch, utilize the FileSystem to fetch every file in the folder and process each file through the data pipeline we designed.
Step 9: LIKE.TG ReportMiner also offers automated monitoring. By employing the SendMail tool, we can configure the system to automatically send an email once all files have been processed.
Once we run the workflow, the insurer has all the relevant information for each claim, simplifying the decision-making process and making it easier to determine which claims to proceed with.
Empowering Growth Satisfaction for Insurers
Improving Customer Satisfaction
When a customer files a claim, LIKE.TG ReportMiner quickly sifts through the submitted documents. The software uses its data extraction tool to identify and pull relevant information in real-time.
This accelerates the initial review process and ensures that all data used in the decision-making is accurate and comprehensive. As a result, customers receive updates and resolutions at an unprecedented pace.
LIKE.TG ReportMiner integrates with customer service platforms, like Salesforce, enabling insurers to provide claim status updates directly to customers, fostering a transparent and trust-filled relationship.
Supporting Scalability and Growth
LIKE.TG ReportMiner scales success by providing a framework that easily handles increasing volumes of claims. Its template-based extraction model allows for the rapid processing of similar documents without the need for constant reconfiguration. This means that as an insurance company grows and the number of claims rises, LIKE.TG ReportMiner’s efficient data handling keeps the workflow smooth and uninterrupted.
Because the automation reduces the manual workload, users can allocate resources more effectively and focus on strategic growth initiatives.
LIKE.TG ReportMiner can handle growing claim volumes without compromising quality or speed, thus supporting the company’s expansion goals while maintaining high customer satisfaction. Discover the benefits of integrating LIKE.TG into your workflow and see the impact on your operational efficiency and customer service.
To explore how LIKE.TG ReportMiner can revolutionize your claims processing, schedule a demo today.
Streamline Auto Claims Processing with LIKE.TG
Ready to Simplify Your Claims Process? Try LIKE.TG's Data Extraction for Free! Get started with a 14-Day Free Trial and see how easy and efficient your claims processing can be.
Start a Free Trial
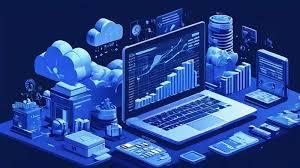
20 Data Warehouse Best Practices
52% of IT experts consider faster analytics essential to data warehouse success. However, scaling your data warehouse and optimizing performance becomes more difficult as data volume grows. Leveraging data warehouse best practices can help you design, build, and manage data warehouses more effectively. Let’s explore how these best practices allow you to process increased volume, variety, and velocity of data, optimize data warehouse functionality and performance, and harness the power of data-driven insights. What Is a Data Warehouse? A data warehouse is a centralized repository that stores and integrates data from multiple sources, such as operational systems, external databases, and web services. A data warehouse provides a consistent and consolidated view of data, regardless of where it originates from or how it is structured. In other words, a data warehouse is organized around specific topics or domains, such as customers, products, or sales; it integrates data from different sources and formats, and tracks changes in data over time. The best part about a data warehouse is that it does not overwrite or delete historical data, simplifying data management significantly. The key components of data warehouse architecture are: Source systems: Source systems are responsible for generating and storing the raw data. We’re talking transactional databases, enterprise applications, and web services. These systems can be part of the company’s internal workings or external players, each with its own unique data models and formats. Extract, Transform, and Load (ETL) process: ETL extracts data from source systems to transform it into a standardized and consistent format, and then delivers it to the data warehouse. This process ensures the data is clean, accurate, and in harmony with the data warehouse schema. Data warehouse: The data warehouse is where the transformed and integrated data is stored. The data warehouse schema sets the rules, defining the structure with tables, columns, keys, and relationships. It doesn’t just store data but also metadata like data definitions, sources, lineage, and quality insights. Data marts: Data marts (also called information marts) are tailored subsets of the data warehouse designed for specific business units, functions, or applications. They offer a more focused and customized view of the data, giving a performance boost to data analysis and reporting. Data access tools: Data access tools let you dive into the data warehouse and data marts. We’re talking about query and reporting tools, online analytical processing (OLAP) tools, data mining tools, and dashboards. Data access tools enable users to analyze and visualize data in their own unique way. Given the generally complex nature of the data warehouse architecture, there are certain data warehouse best practices that focus on performance optimization, data governance and security, scalability and future-proofing, and continuous monitoring and improvement. Best Practices for Data Warehouses Adopting data warehousing best practices tailored to your specific business requirements should be a key component of your overall data warehouse strategy. These strategies enable you to optimize query performance, fortify data security, establish robust data governance practices, and ensure scalability. Performance Optimization Boosting the speed and efficiency of data warehouse operations is the key to unleashing its full potential. Techniques like indexing, partitioning, caching, compression, and parallel processing play pivotal roles. Consider the following data warehouse best practices to enhance performance: 1. Strike the right balance with indexing to optimize query performance Indexes are data structures that store the values of a specific column or a combination of columns, along with pointers to the rows that contain them. This data warehouse best practice allows you to speed up data warehouse data retrieval by reducing the number of disk I/O operations. With indexing, your data warehouse no longer needs to scan the entire table, thus improving query performance. For instance, if you have a table with customer information, an index on the customer ID column will allow you to find a specific customer’s records quickly. 2. When partitioning, select the right column, align with query patterns, and avoid over-partitioning Partitioning is the process of dividing a large table or index into smaller, more manageable units called partitions. Partitioning improves performance by reducing the amount of data that needs to be scanned, loaded, or updated at a time. Partitioning can also enable other data warehouse best practices, such as parallel processing. For instance, if you have a table with sales data, you can partition it by date, region, or product category to optimize the queries that filter by these criteria. 3. Use caching to increase data access speeds Caching is a crucial data warehouse best practice as it allows you to store frequently accessed data or query results in a temporary memory location, such as RAM or SSD. Caching can improve performance by reducing the latency and increasing the throughput of data warehouse operations. For example, if you have a query that calculates the total revenue for each month, you can cache the result and reuse it for subsequent queries that need the same information. 4. Use data compression to enhance storage efficiency Compression allows you to reduce the size of data by applying some encoding or algorithm, such as dictionary encoding, run-length encoding, or delta encoding. Compression improves performance by saving disk space, reducing network bandwidth, and increasing the amount of data that can be processed in memory. For instance, if you have a table with product information, you can compress the product description column by using a dictionary-based algorithm that replaces repeated words or phrases with shorter codes. 5. Accelerate query execution with parallel processing Parallel processing helps execute multiple tasks or queries simultaneously by using multiple processors, cores, threads, or machines. Parallel processing improves data warehouse performance by distributing the workload and utilizing the available resources more effectively. For example, if you have a query that joins two large tables, you can use parallel processing to divide the tables into smaller chunks and join them in parallel. Data Governance and Documentation Establishing and enforcing rules, policies, and standards for your data warehouse is the backbone of effective data governance and documentation. Techniques like metadata management, data cataloging, data lineage tracing, and data quality management are some of the data warehouse best practices you can incorporate. 6. Maintain a metadata repository to facilitate data discovery Metadata Management allows you to define, collect, store, and maintain metadata, which is data about data. Metadata describes the structure, meaning, origin, and data usage. It is a valuable tool for managing and tracking changes and impacts. Keeping track of metadata can help you understand the data, facilitate data integration, enable data lineage tracing, and enhance data quality. This not only aids user comprehension of data but also facilitates seamless data discovery, access, and analysis. 7. Use data cataloging for improved data accessibility Data cataloging is a searchable and browsable inventory of the data assets in the data warehouse. It creates and maintains a metadata repository that describes the data sources, tables, columns, relationships, and business rules in the data warehouse. Cataloging helps users access a centralized and searchable source of truth for data discovery, exploration, and understanding. If you have a data warehouse with multiple schemas, tables, and views, a data catalog is essential keep a unified and user-friendly interface for exploring and querying the data. 8. Use data profiling to ensure your data is healthy Data profiling is the process of analyzing the data in the data warehouse to discover its characteristics, such as data type, format, range, distribution, frequency, uniqueness, completeness, accuracy, and relationships. It helps assess its structure, content, quality, and distribution, identify data anomalies and errors, and determine data cleansing and transformation requirements. Data profiling gives you an overview of the data health of a particular data asset. 9. Enhance data transparency with lineage tracing Data lineage is the process of tracking your data’s origin, transformation, and destination in the data warehouse. This provides a clear and auditable record of data movement, flow, and impact. Tracing data lineage helps understand the history and context of the data, verify the accuracy and reliability of the data, and troubleshoot data issues. For instance, if you have a table with sales data, you can use data lineage tracing to show the source systems, ETL processes, and intermediate tables that contributed to the data in the table. 10. Use relevant metrics to continuously monitor data quality Data quality monitoring is the process of measuring, reporting, and improving data quality in the data warehouse. It helps measure and report data health based on predefined data quality metrics, such as accuracy, completeness, timeliness, validity, or uniqueness over time. With data quality monitoring, your team can be alerted to data anomalies errors, or changes after the data warehouse has been deployed. Security Measures Guarding your data warehouse against unauthorized access, modification, or disclosure requires robust security measures. Encryption, data masking, authentication, authorization, and auditing are your arsenal. Here are some data warehouse best practices to ensure data security: 11. Authorize, control, and monitor data access with role-based access control Role-Based Access Control (RBAC) aligns access with user roles, ensuring individuals only access data and functions they need. Managing authorizations controls the level of access by defining what data or operations the users or applications can view, modify, or execute. As a result, RBAC simplifies data security management and minimizes the risks of data breaches and leaks. For example, if you have a data warehouse with multiple users, you can use RBAC to assign different roles and privileges to the users, such as admin, analyst, or viewer, and restrict their access to specific schemas, tables, columns, or queries. 12. Protect sensitive information with data encryption Encryption helps you transform the data or files into an unreadable form by using a secret key or algorithm. Encryption helps prevent data breaches, data theft, or data tampering by making the data inaccessible or meaningless to unauthorized parties. For instance, if you have a table with sensitive data, such as customer SSN, addresses, or credit card numbers, you can encrypt the data before storing it in the data warehouse or transferring it over the network. 13. Use dynamic masking to selectively hide data Data masking is the process of replacing the original data with fictitious or modified data that preserves the data format and functionality. It can protect the privacy and confidentiality of the data by hiding or obscuring the sensitive or identifying information. For instance, if you have a table with customer information, you can mask the data by replacing the customer names with random names, the addresses with random addresses, or the credit card numbers with asterisks. 14. Manage data access with user authentication Authentication is the process of verifying the identity of the users or applications that access the data warehouse. Authentication can prevent unauthorized access by ensuring that only legitimate and authorized parties can access the data warehouse. For example, if you have a data warehouse with multiple users, you can use authentication to require the users to provide their usernames and passwords, or other credentials, such as biometrics or tokens, before accessing the data warehouse. 15. Maintain accountability with regular audits Auditing helps record and review the activities and events that occur in the data warehouse. It helps you monitor the data warehouse performance, usage, and security by providing logs, reports, and alerts on the data or operations that are accessed, modified, or executed. For example, if you have a data warehouse with multiple users, you can use auditing to track who, when, what, and how the users accessed or changed the data in the data warehouse. Scalability and Future-Proofing Ensuring your data warehouse can evolve with the future growth of data, users, and business requirements is essential. Techniques like capacity planning, modular design, and embracing cloud computing are your go-to strategies. Incorporate the following data warehouse design best practices: 16. Leverage cloud computing to handle large data sets Cloud computing leverages remote servers and services to store, process, and analyze data. It offers scalability, flexibility, and cost-effectiveness by allowing the data warehouse to adjust the resources and services according to the demand dynamically and pay only for what is used. For instance, if you have a data warehouse that needs to handle large and variable volumes of data, you can use cloud computing to store the data in scalable and distributed storage systems, such as Amazon S3 or Google Cloud Storage, and process the data in scalable and elastic compute platforms, such as Amazon Redshift or Google BigQuery. 17. Optimize resource allocation based on the workload Capacity planning allows users to estimate and provision the resources and services needed to meet the current and future demands of the data warehouse. Capacity planning helps avoid performance degradation, resource wastage, or service interruption by ensuring the data warehouse has sufficient and optimal resources and services at all times. For instance, if you have a data warehouse that needs to support a growing number of users and queries, you can use capacity planning to monitor and forecast the resource and service utilization, such as CPU, memory, disk, network, and concurrency, and plan for the resource and service allocation, upgrade, or migration accordingly. This avoids resource shortages, bottlenecks, or over-provisioning and ensures data availability and performance. 18. Select the right data warehouse modeling technique Data warehouse modeling is the process of designing the logical and physical structure of the data warehouse, based on the business requirements and the data sources. Leveraging the appropriate schema, such as star or snowflake schema can help optimize your data warehouse for reporting. It does so by organizing the data into facts and dimensions. Data warehouse modeling also involves applying various techniques, such as normalization, denormalization, aggregation, and partitioning, to optimize the data warehouse for performance, storage, and usability. For instance, data warehouse modeling like star schema creates a central fact table that stores the measures of the business processes, and several dimension tables that store the descriptive attributes of the facts. This schema is simple, easy to understand, and fast to query, as it reduces the number of joins and tables involved. However, the ideal data modeling technique for your data warehouse might differ based on your requirements. For instance, a star schema optimizes your data warehouse reporting, but it can also result in data redundancy, inconsistency, and update anomalies, as the same dimension attributes may be repeated in multiple tables. 19. Consider the modular design approach for scalability and performance Modular design is a data warehouse design principle that advocates for breaking down the data warehouse into smaller, independent, and reusable modules. This approach can improve the scalability, maintainability, and performance of the data warehouse, as well as reduce the complexity and cost of development and testing. An example of modular design is using a data vault architecture consisting of three types of tables: hubs, links, and satellites. Hubs store the business keys of the entities, links store the associations between the entities, and satellites store the attributes and history of the entities. Each table is a module that can be loaded, updated, and queried independently without affecting the rest of the data warehouse. Like dimensional modeling, following the data vault design is ideal only in certain situations. Learn more about whether you need a data vault. Monitoring and Maintenance The last in our list of data warehouse best practices is performance monitoring and periodic maintenance. The key to keeping your data warehouse running smoothly is to monitor its performance closely and fix any problems. It includes error handling, backup and recovery, and testing and debugging any changes you make. 20. Ensure smooth operations with continuous performance monitoring Performance monitoring offers crucial insights into the bottlenecks, errors, and inefficiencies of operations. Suppose you have a data warehouse that stores social media data for a digital marketing agency. You want to ensure that your it operates smoothly and reliably, delivering accurate and timely results to your users and clients. One way to do this is to implement performance monitoring in your data warehouse. It involves the following steps: Define performance metrics: You need to define and measure the key performance indicators (KPIs) for your data warehouse, such as data load time, query response time, data quality, user satisfaction, etc. You may also use tools and techniques such as benchmarks, baselines, and thresholds to set and compare the performance standards and goals. Collect performance data: You need to collect and store the performance data for your data warehouse, such as data volume, data velocity, data latency, data errors, data usage, etc. You may also use tools and techniques such as logs, alerts, and notifications to capture and report performance events and incidents. Analyze performance data: You need to analyze and interpret the performance data for your data warehouse, such as identifying and diagnosing the root causes, impacts, and trends of performance issues and anomalies. LIKE.TG: Empowering Data Warehouse Best Practices Data warehouses are powerful and valuable assets for modern businesses. Incorporating data warehouse best practices into your data warehouse management ensures a well-rounded, high-performing, and secure environment, ready to meet the evolving demands of your business. However, designing and building a data warehouse requires careful planning, implementation, and maintenance and must follow some best practices to ensure their functionality and performance. A tool like LIKE.TG is indispensable for implementing data warehouse best practices as it addresses the complexities of data management, automates processes, ensures data quality, and provides the flexibility needed to adapt to evolving business requirements. Why Choose LIKE.TG? Zero-Code ETL/ELT: Benefit: Automate dataflow creation effortlessly for seamless population of database tables. Impact: Streamline your processes, saving time and resources, while ensuring accuracy in your data transitions. Unified Metadata-Driven Solution: Benefit: Design, develop, and deploy high-volume, operational data warehouses effortlessly. Impact: Experience a unified approach to metadata-driven solutions, promoting consistency, and simplifying the development lifecycle. Built-In Validations and Checks: Benefit: Ensure the integrity of your data and data model with built-in validations and checks. Impact: Fortify your data quality, reducing errors and discrepancies, and fostering a foundation of trust in your data. Support for Different Schemas: Benefit: Embrace flexibility with support for dimensional modeling, data vault 2.0, and 3NF schema. Impact: Tailor your data warehouse to your unique needs, accommodating diverse schemas seamlessly. Data Modeler: Benefit: Craft a data model from scratch or generate one for an existing database effortlessly. Impact: Accelerate your data modeling process, promoting agility in adapting to evolving business requirements. Automated Data Pipelines: Benefit: Facilitate automated data pipelines with a rich palette of 200+ transformations and robust scheduling. Impact: Transform your data management landscape, ensuring a seamless and efficient flow from source to destination. Data Accuracy and Reliability: Benefit: Ensure data accuracy and reliability through comprehensive validation tools. Impact: Fortify your decision-making with trustworthy data, reducing the risk of errors and enhancing overall reliability. Seize the LIKE.TG advantage and implement data warehouse best practices with the ease of no-code. Start your data warehousing journey with a free 14-day trial. Build a Data Warehouse in Days - Without Any Coding! Try LIKE.TG DW Builder for free for 14 days! Simplify data integration and get real-time insights effortlessly. Request Free Trial
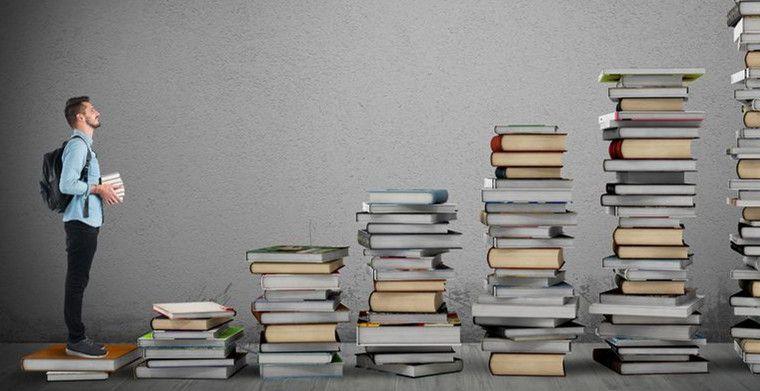
Best Azure ETL Tools For 2024
Azure SQL Data Warehouse, now called Azure Synapse Analytics, is a powerful analytics and BI platform that enables organizations to process and analyze large volumes of data in a centralized place. However, businesses need to employ the right Azure ETL (Extract, Transform, Load) tools to fully leverage its powerful capabilities. In this article, we will explore the top 5 ETL tools for Azure Data Warehouse that can help streamline data integration and improve overall analytics performance. Why Businesses Need ETL tools for Azure Data Warehouse Businesses today are generating vast amounts of data from various sources, such as social media, customer interactions, and IoT devices. However, this data is often scattered across different systems, making it difficult to consolidate and utilize effectively. This is where Azure ETL tools come into play. These tools are designed to extract data from disparate sources, transform it into a consistent format, and load it into a centralized data warehouse like Azure Synapse. By automating the data integration process, ETL tools for Azure Data Warehouse enable businesses to centralize data from multiple sources and analyze it to gain actionable insights. With the increasing need for data-driven decision-making, businesses are turning to Azure ETL tools to streamline their data integration processes. These tools provide a range of benefits, including: Efficiency:Automated ETL streamlines the process of extracting, transforming, and loading data, saving businesses valuable time and resources. Manual data integration can be time-consuming and prone to errors, whereas ETL tools for Azure Data Warehouse ensure accuracy and consistency in data processing. Scalability:As businesses grow and generate more data, Azure ETL tools can easily handle the increased volume and complexity of data. They provide scalable solutions that can adapt to changing business needs without compromising performance. Data Quality:Azure ETL tools offer built-in data cleansing and validation capabilities, ensuring that the data loaded into Azure Data Warehouse is accurate and reliable. This helps businesses make informed decisions based on trustworthy data. Integration:ETL tools for Azure Data Warehouse support integration with various data sources, including databases, cloud storage, APIs, and more. This allows businesses to consolidate data from different systems and sources, providing a holistic view of their operations. Data Transformation:Azure ETL tools provide a wide range of data transformation capabilities, such as data enrichment, aggregation, and normalization. These transformations enable businesses to derive meaningful insights from raw data and make it suitable for analysis. Top 5 ETL Tools for Azure Data Warehouse LIKE.TG LIKE.TG is a well-establishedETL/ELTsolution with native connectivity to theseAzure databases: MySQL PostgreSQL SQL Server MariaDB It also integrates with Azure Data Lake Gen 2. LIKE.TG streamlines data integration with a fully no-code approach, enabling business users to execute their data management taskseasilywhile saving significant resources. LIKE.TG has one of the best customer support teams in the market and is trusted by numerous Fortune 500 companies and SMEs for their ETL initiatives. Moreover, the pricing is flexible– usersonly pay for the modulestheyuse. LIKE.TGalsooffers additional no-code unstructured data management, data warehouse building, and API management capabilities, which come on top of its already robust ETL/ELT engine. LIKE.TG’s key features include: User-friendlyinterface Extensivenative connectors Variety of pre-built transformations. Automated data mapping Data quality and profiling. Pushdownoptimization. Git Integration. Real-timedata previews Workflow Automation Job Scheduler Matillion Matillion ETL is also a cloud-native data integration platform designed toETL data into cloud data warehouses such as Azure Synapse Analytisc, Amazon Redshift, Google BigQuery, and Snowflake. It provides a visual interface for designing data integration workflows and offers features such as data profiling, data quality checks, and error handling. Pros: Pre-built connectors for numerous data sources and applications. Seamless integration with various cloud providers. User-friendly UI. Cons: Escalating costs as usage increases. Complex updates with a lack of clear communication. Lack of clarity during debugging. Here are the best Matillion alternatives to consider. Fivetran Fivetran is an automated data movement platform thatenables ELTfrom various sources into centralized data warehouses or destinations for easier analysis and use. Its automated data pipelines ensure data replication. Fivetran also provides users with the flexibility to customize their data pipelines. Users can apply filters, basic transformations, and aggregations to their data, ensuring that only relevant and meaningful information is used. It also offers some data validation capabilities, allowing users to verify the accuracy and consistency of their data during the integration process. Pros: Incremental data synchronization for minimizing data transfer costs. Near real-time data replication. Good customer support and documentation. Cons: Steep learning-curve for non-engineers. Late alerts and notifications. Lack of data masking options. Here are the best Fivetran alternatives to consider. Stitch Data Stitch Data is a cloud-based data integration platform. Itprovides a fully managed service that enables organizationsETL without the need for infrastructure management. It was acquired by Talend in 2018. Stitch offers a simple interface for connecting and transforming data from various sources. It enables businesses to load data into an Azure data warehouse without the need for complex coding or scripting. Pros: Cheaper than some other solutions. Robust relational database replication. Cons: Below-par document store replication. Slow customer support. Difficulty writing custom SQL-scripts. Integrate.io Integrate.io is a low-code data warehousing and integration platform that’s hosted on the cloud.It enables businesses to handle big data without making hefty investments in hardware, software, or specialized staff.It offers a visual UI for designing and deploying data pipelines, making it accessible to both technical and non-technical users. Integrate.io’s data transformation capabilities and built-in data quality checks ensure the accuracy and reliability of data loaded into any data warehouse. Pros: Good customer service. Drag-and-drop UI. Highly customizable. Cons: Error logs can be more detailed. The API can be more robust. Transforming JSON and other nested formats is difficult. Choosing the right Azure ETL Tool When it comes to selecting the right ETL (Extract, Transform, Load) tool for Azure Data Warehouse, there are several factors to consider. These factors include ease of use, integration capabilities, scalability, and cost-effectiveness. Each of the tools mentioned above has its unique strengths and caters to different use cases. Let’s dive deeper into these factors to help you make an informed decision. Integration Capabilities:The most critical factor to consider is how easily users can connect to different sources and destinations using the Azure ETL tool. It should seamlessly integrate with Azure databases. The tool should also support different data formats and provide connectors for popular data sources, ensuring compatibility and ease of data movement. Ease of Use:Another essential considerations is ease of use. Some tools offer a visual interface with drag-and-drop functionality, allowing users to design and manage their workflows without writing any code. This approach is particularly beneficial for business users. Scalability:Azure Data Warehouse is designed to handle large volumes of data and support high-performance analytics. Therefore, it is crucial to choose an Azure ETL tool that can scale along with your data needs. The tool should be able to handle increasing data volumes without compromising performance. It should also support parallel processing and distributed computing to take full advantage of the Azure Data Warehouse’s capabilities. Scalability ensures that your ETL processes can keep up with the growing demands of your business. Cost-effectiveness:Cost is always an important consideration when selecting any technology solution. Different Azure ETL tools have varying pricing models, including pay-as-you-go, subscription-based, or a combination of both. It is essential to evaluate the pricing structure of each tool and consider your organization’s budget and long-term data integration requirements. Additionally, consider any additional costs associated with data storage, data movement, or other related services. A cost-effective ETL tool for Azure Data Warehouse should provide the necessary features and performance while fitting within your budget constraints. Conclusion Azure Data Warehouse is a versatile and scalable platform that empowers organizations to unlock the full potential of their data. And when it comes to ETL tools for Azure, LIKE.TG is the best option. LIKE.TG provides robust built-in connectivity to Azure databases. It also provides automated data mapping and workflow automation for streamlining ETL/ELT workflows. LIKE.TG alsooffers a highly adaptable pricing model upfront. Additionally, users benefit fromour award-winning onboardingassistanceand post-setup support. With LIKE.TG, you also gain access to: A no-code solution for buildingand populating Azuredata warehouses. A no-code API management solution. Try LIKE.TG with afree 14-day trial and discover astreamlinedapproach to ETL! Streamline Data Integration with Azure ETL Simplify data movement transformation in Azure with LIKE.TG's user-friendly, no-code ETL/ELT solution that integrates seamlessly with Azure databases. Start your FREE Trial
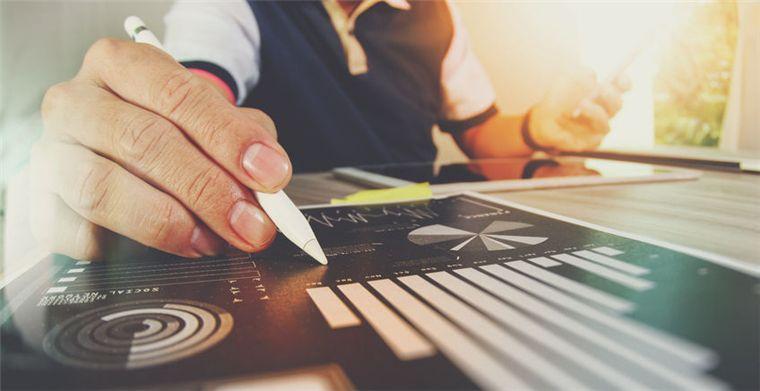
Mastering API Architecture: A Comprehensive Guide | LIKE.TG
Did you know that the average enterprise utilizes over 1,500 APIs to drive its digital operations? APIs play a pivotal role in today’s data-driven landscape. However, few know the impact API architecture has in enhancing API performance. What are APIs? An API, or application programming interface, is a software interface that dictates how different software systems communicate with each other and exchange data and functionality. Think of an API as a contractual agreement between a service provider and a consumer. The provider offers services like data, functionality, or resources, and the consumer accesses these services through the API. APIs power everyday applications and services. When you interact with social media platforms like Facebook or Twitter, APIs enable actions like posting, liking, and sharing. APIs also facilitate E-commerce experiences on platforms like Amazon or eBay, allowing users to browse, search, and conduct transactions. Even your interactions with mapping or weather apps involve APIs, facilitating access to location and weather data from diverse sources. What Is API Architecture? While API refers to the interface, API architecture involves designing and implementing APIs. In short, it allows to dictate how to structure, organize, and expose a software system’s data and functionality. An effective API architecture prioritizes creating reusable and interoperable components, focusing on the external interface rather than internal implementation details. API architecture stands apart from traditional software architecture in key aspects. It requires careful consideration of API consumers’ needs and expectations, often differing from those of API developers. An API holds several responsibilities in facilitating communication between a software system and the outside world. It defines inputs, outputs, data formats, protocols, and authentication and authorization mechanisms. A prevalent concept in API architecture is the API-first approach. This involves designing and building APIs before developing the applications that use them, yielding benefits such as a clear and consistent API contract, faster development processes, modular and reusable design, and support for a scalable and flexible architecture. However, implementing an API architecture brings challenges. It demands a high level of expertise to handle various communication aspects like security, performance, reliability, and compatibility. Effective communication and collaboration between API developers and consumers are crucial for meeting expectations and requirements. Striking a balance between the quality and quantity of the API is another challenge – the API architecture must be able to provide sufficient functionality and data without overwhelming complexity. Layers of API Architecture API architecture layers work in tandem to create a comprehensive solution. Each layer plays a distinct role, interacting seamlessly with other layers through well-defined interfaces and protocols. Data Layer The data layer enables APIs to supply and share data while maintaining data quality, ensuring security, and facilitating scalability for diverse applications and services. One study discovered that a data layer can elevate data quality by up to 50%, primarily by eliminating data discrepancies and errors. For instance, it provides a centralized point for data management, reducing the chances of inconsistent or erroneous data across different parts of the system. A well-designed data layer separates data from the business logic and presentation layers. This makes the API architecture easier to maintain and scale. It can also layer hide data access and manipulation details from the API consumers, offering a standard and consistent interface for data operations. Likewise, the data layer supports different data formats and sources, allowing you to integrate diverse data systems and create rich and varied APIs. According to one study, a data layer can enhance data collection efficiency by up to 40%, as it mitigates the need for manual coding and testing. Application Layer At the core of API architecture, the application layer handles the computing and processing of data and logic, shaping the functionality and behavior of APIs and applications. Components like microservices, serverless functions, business logic, and algorithms reside here. The application layer uses different tools such as Node.js, Python, and AWS Lambda to enhance the overall reliability, scalability, and performance of the API-related tasks and operations. For example, Node.js and Python streamline the handling of incoming data, whereas AWS Lambda, a serverless computing service, contributes to a scalable API architecture. Integration Layer Serving as the bridge, the integration layer connects and integrates data and application layers with external systems and applications. Mechanisms like API gateways, service meshes, message brokers, and event streams play a crucial role in enabling this: API Gateways: API gateways manage incoming requests to relevant backend services based on predefined rules. This ensures that the correct data and functionalities are accessed by users or other services. Additionally, API gateways contribute to the overall system performance by implementing load balancing, distributing incoming traffic across multiple servers to prevent overloading of any single server. Service Meshes: Service meshes manage communication between microservices within an application. They provide infrastructure for service-to-service communication, handling service discovery and routing. Message Brokers: Message brokers facilitate asynchronous communication between different components in a distributed system. They play a pivotal role in decoupling producers from consumers, allowing components to operate independently. Event Streams: Event streams provide a continuous flow of events that can be consumed by different components or systems. They enable real-time communication and event-driven workflows, allowing systems to react promptly to changes. Interaction Layer The interaction layer is a key part of the API architecture because it defines how the API communicates with other systems and users. It provides the specifications, formats, protocols, and tools that enable the API to be accessed, understood, and used effectively. The interaction layer establishes a consistent and uniform way of exposing and consuming APIs, regardless of the underlying technologies or platforms. This reduces complexity and increases interoperability across different systems and applications. It also provides clear and comprehensive information about the API’s functionality, parameters, responses, and errors. This helps developers and users to learn and use the API correctly and efficiently. Management Services Layer Acting as the brain of API architecture, the management services layer oversees the lifecycle and performance of APIs and applications. These services include API design, development, deployment, versioning, governance, analytics, testing, and debugging.This layer is instrumental in providing the necessary tools and services to create, manage, and enhance APIs and applications. In the design phase, the management services layer helps in structuring APIs and applications in accordance with best practices and standards. It also facilitates the documentation and testing of APIs and applications prior to deployment. The management services layer plays a crucial role in deploying APIs and applications to different environments, be it cloud, on-premises, or hybrid. It further ensures the scalability of APIs and applications based on demand and load. Security Services Layer The security services layer is responsible for safeguarding the data and communication of APIs and applications. It employs mechanisms like encryption, authentication, authorization, auditing, and logging. Protocols and standards like SSL/TLS, OAuth, JWT, and API Keys play a critical role in the security services layer as they maintain the confidentiality, integrity, and availability of data. Components of API Architecture API architecture components offer specific services and functions for APIs and applications. Understanding these components is essential for creating, managing, and consuming APIs securely and efficiently. Let’s explore the five key components of API architecture, delving into their purpose, function, and best practices. API Gateway The API gateway serves as the entry point, managing the requests and responses between API consumers and providers. The API gateway takes care of: Load balancing: Distributing traffic among multiple servers or instances for improved performance. Caching: Temporarily storing data or responses to reduce latency and backend system load. Rate limiting: Controlling the number of requests to prevent overloading or abuse. Transformation: Converting data or messages to ensure compatibility and interoperability. API Portal The API portal acts as the interface, providing information and documentation for developers to discover and consume APIs and applications. It offers: API catalog: A directory of available APIs with descriptions, categories, and tags. API documentation: A detailed explanation of the API contract, covering inputs, outputs, data formats, protocols, authentication, authorization, errors, etc. API testing tools: Tools enabling consumers to test and validate API functionality. API client libraries: Pre-built code and functions facilitating API integration and consumption. API Management Platform The API management platform functions as the control center, overseeing the lifecycle and performance of APIs and applications. It provides services such as: API design: Allowing developers to create and define the API contract. API development: Enabling implementation and testing of API logic and functionality. API deployment: Allowing publishing, updating, versioning, staging, and rolling out of APIs and applications. API governance: Enforcing and maintaining quality, consistency, and compliance through policies, standards, and guidelines. API Security Services API security services form the protective layer, ensuring the security and safeguarding of data and communication. Mechanisms include: Encryption: Transforming data or messages into an unreadable form to prevent unauthorized access or modification. Authentication: Verifying the identity of API consumers or providers to ensure credibility. Authorization: Determining permissions and access rights to restrict unauthorized actions. Auditing: Recording and tracking activities and events for compliance with rules and regulations. Understanding these components empowers you to navigate the intricacies of API architecture, facilitating secure and efficient API management for your projects. How to Design an API Architecture? Step 1: Define the goals and requirements of the API architecture Begin by defining the goals and requirements of your API architecture. Conduct a thorough analysis of the problem domain, involving relevant stakeholders such as developers, customers, partners, and managers. Document the goals and requirements in a clear and concise manner, using tools like user stories, use cases, or specifications. Step 2: Choose the right API style and format Select the API style and format that best suits your project’s goals and requirements. Consider options like REST, GraphQL, gRPC, or SOAP, each with distinct advantages and disadvantages. Choose based on your data and application characteristics. For instance, GraphQL for flexibility, gRPC for speed and efficiency, or REST for simplicity and standardization. Step 3: Implement API Design Best Practices Adhere to the principles of API design to create a high-quality, user-friendly, and maintainable API. Embrace principles such as simplicity, consistency, usability, modularity, reliability, security, and testability. Simplicity: Avoiding complexity and confusion by having a logical structure, consistent naming, and minimal endpoints and parameters. Uniformity: Maintaining the same format, protocol, and style across all aspects of the API, such as endpoints, parameters, responses, and errors. Usability: Providing clear and accurate documentation, as well as tools and methods for testing and debugging, to enhance the user and developer experience. Modularity: Allowing for easy integration and extension by separating concerns into smaller, independent, and loosely coupled components. Reliability: Ensuring stability and consistent performance by exhibiting high availability and fault tolerance. Security: Protecting the data and communication of the API and its users by using strong encryption, authentication, and authorization mechanisms. Testability: Verifying the quality and correctness of the API by having a clear and measurable specification, and by performing comprehensive and automated testing. Step 4: Document and test the APIs Documentation and testing are crucial aspects of designing the API architecture. Create and maintain comprehensive documentation using specifications and tools like OpenAPI, Swagger, or Postman. Test the API for functionality, performance, compatibility, and security using reliable tools and frameworks, for example, Mocha, Chai, Jest, or Supertest. Step 5: Implement API security and governance policies Implement security and governance policies to protect and manage the API. Use mechanisms such as OAuth, JWT, HTTPS, or SSL for security. Govern the API through monitoring, versioning, and lifecycle management. Tools like Prometheus, Grafana, Semantic Versioning, and CI/CD methodologies aid in implementing these policies. Step 6: Monitor and optimize the API performance and quality Continuously monitor and optimize API performance and quality. Utilize metrics from monitoring, testing, and user feedback to identify and address issues, such as: Errors: Monitoring and optimizing API performance and quality can help to detect and fix errors, ensuring that the API functions correctly and reliably. Latency: Latency can affect the user experience and the performance of the dependent applications and services. Monitoring and optimizing API performance and quality can help reduce and improve latency, ensuring that the API responds quickly and smoothly. Security vulnerabilities: Security vulnerabilities can be caused by various factors, such as poor design, lack of encryption, weak authentication, or outdated dependencies. Monitoring and optimizing API performance and quality can help to identify and prevent security vulnerabilities, ensuring that the API is secure and protected. Implement best practices such as caching, compression, pagination, and throttling, and seek feedback from users and stakeholders to enhance API functionality and usability. Simplifying API Architecture Design with No-Code API architecture is the foundation of modern digital businesses. It enables data and application integration, innovation, scalability, and security across various platforms and systems. With API architecture, you can create modular and reusable solutions that meet your business needs and goals. However, designing and managing API architecture can be challenging and time-consuming. You need to consider various aspects such as data quality, performance, security, compliance, and testing. You also need to choose the right tools and technologies that suit your requirements and preferences. That’s why you need a no-code API management tool like LIKE.TG. LIKE.TG is a powerful and easy-to-use platform that helps you design, secure, publish, and deploy APIs on-premises, in the cloud, or in hybrid environments. With LIKE.TG, you can: Create APIs using a drag-and-drop interface or pre-built templates Transform data using built-in functions or custom logic Validate data using advanced data profiling features Automate workflows using job scheduling features Monitor performance using real-time dashboards and reports Secure APIs using encryption, authentication, authorization, and auditing features Publish APIs using RESTful or SOAP protocols Deploy APIs on any platform or environment LIKE.TG is the ultimate solution for API architecture design. It simplifies the complex process of creating and managing APIs without writing a single line of code. If you want to learn more about LIKE.TG, visitLIKE.TG’s websiteor contact us directly at +1 888-77-LIKE.TG. Alternatively, you can try it out with a 14-day free trial..
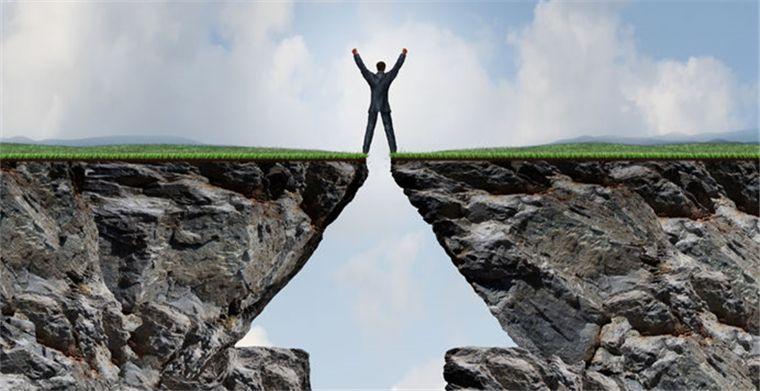
What Is Data Governance and Why It Matters? A Complete Guide
What is Data Governance? Data governance refers to the strategic management of data within an organization. It involves developing and enforcing policies, procedures, and standards to ensure data is consistently available, accurate, secure, and compliant throughout its lifecycle. At its core, data governance aims to answer questions such as: Who owns the data? Determining clear ownership ensures accountability and responsibility for data quality and compliance. What data is being collected and stored? Identifying the collected data types helps understand their significance and potential impact on the organization. How is the data used and accessed? Establishing guidelines for accessing and using data ensures it is utilized appropriately and ethically. Is the data accurate and reliable? Implementing measures to maintain data integrity ensures that the data is accurate, consistent, and trustworthy. Is the data secure? Implementing security measures to protect data from unauthorized access, breaches, or misuse is crucial for maintaining confidentiality and compliance with regulations. Learn about the dfferences between three core data governance concepts: a data catalog, data dictionary, and business glossary. Data Governance Vs. Data Management What’s the difference between data governance and data management? Data governance primarily focuses on establishing the framework, policies, and processes for ensuring data quality, integrity, security, and compliance across the organization. On the other hand, data management is the broader set of practices and activities involved in the day-to-day handling, storage, retrieval, and maintenance of data assets. While data governance sets the rules and guidelines for managing data, data management involves implementing those rules through various operational tasks such as data collection, storage, integration, cleansing, and analysis. Related: Find out how data governance helps establish effective data management. Aspect Data Governance Data Management Focus Establishing policies, standards, and processes for ensuring data quality, integrity, security, and compliance Implementing operational tasks and activities involved in handling, storing, retrieving, and maintaining data Purpose Ensuring accountability, compliance, and responsible management of data assets across the organization Efficiently handling data throughout its lifecycle to derive value and support business operations. Implementation Establishes the rules and guidelines for data management activities and provides oversight and enforcement Executes the rules and guidelines established by data governance to manage data effectively Stakeholders Business stakeholders, IT professionals, data stewards, compliance teams Data analysts, database administrators, IT professionals, data stewards, and end-users Outcome Ensures data quality, integrity, security, and compliance across the organization Ensures efficient data handling supports decision-making and business operations. The Importance of Data Governance Data governance facilitates accessibility by establishing clear guidelines for who can access the data under what circumstances. These guidelines ensure that every employee has access to data required for their roles, promoting collaboration and informed decision-making across the organization. Ensure compliance: Reliable data fuels informed choices at all levels. Trust in the information you’re using empowers you to make strategic decisions confidently. Mitigate risks: Data governance safeguards sensitive information, reducing the risk of data breaches and protecting your organization’s reputation while building trust with stakeholders and customers. Improve data quality: Effective data governance processes establish standards and protocols for data collection, storage, and management, leading to accurate, consistent, and up-to-date data. Key Stakeholders in Data Governance Data governance involves collaboration between executive leadership, IT professionals, data stewards, compliance teams, and data analysts. Adapting to evolving business needs and regulatory requirements requires continuous monitoring, assessment, and improvement. The key stakeholders in data governance include: Executive leadership: Provide direction and support to drive the strategic implementation of data governance. IT professionals: Implement and maintain technical infrastructure to support data governance initiatives. Data stewards: Ensure data quality, integrity, and compliance within specific areas or datasets. Compliance teams: Oversee adherence to regulations and standards governing data usage and privacy. Data analysts: Utilize governed data to extract insights and inform decision-making processes. Modern companies are increasingly opting for unified, no-code solutions. They provide a user-friendly platform for all stakeholders to collaborate seamlessly, regardless of technical expertise. It empowers the leadership team to define governance policies, while IT professionals, or even data stewards, can implement them efficiently due to the no-code environment. Data stewards and compliance teams can easily monitor and enforce governance standards, while data analysts can seamlessly access data for reporting and analytics, fostering effective collaboration across the organization. Related: Learn about active data governance and automated data governance. How Does a Data Governance Program Work? A Data Governance Program operates by establishing a framework that includes various processes, policies, and practices aimed at ensuring the effective management of an organization’s data assets, including: Policy Development: The program begins with developing comprehensive data governance policies that outline rules and guidelines for data management, usage, security, and compliance. These policies are typically designed in collaboration with key stakeholders across different departments and are aligned with the organization’s strategic objectives. Data Ownership and Accountability: Clear ownership and accountability for data assets are defined within the program. This step involves identifying data stewards or custodians responsible for specific datasets or domains. Data stewards uphold data quality standards, control data access, and ensure compliance. Data Standards and Metadata Management: Standardizing data formats, definitions, and naming conventions is crucial for ensuring consistency and interoperability across the organization. The program establishes processes for metadata management, including the documentation of data lineage, definitions, and usage policies. Data Quality Management: The program implements processes and tools for monitoring and improving data quality. This step involves identifying data quality issues, assessing their impact on business operations, and implementing corrective measures to address them. Continuous data profiling, cleansing, and validation are critical components of data quality management. Data Security and Compliance: Data governance ensures that appropriate security measures are in place to protect sensitive data from unauthorized access, breaches, or misuse. Compliance with relevant regulations and standards, such as GDPR, HIPAA, or PCI-DSS, is critical to data governance. The program establishes controls and processes to ensure compliance with data protection laws and industry regulations. Data Lifecycle Management: Effective data governance involves managing the entire data lifecycle from creation to archival or disposal. This step includes defining policies for data retention, archiving, and disposal based on business requirements, regulatory obligations, and data value. Monitoring and Enforcement: The program includes mechanisms for monitoring adherence to data governance policies and enforcing compliance. This stage may involve regular audits, data quality assessments, and access controls to ensure teams follow data governance policies effectively. A data governance program establishes a structured approach to managing data assets, ensuring their quality, integrity, security, and compliance throughout their lifecycle. A unified environment and no-code solutions offer advantages by providing a centralized platform for collaboration and enabling stakeholders of varying technical backgrounds to contribute to governance initiatives efficiently. What is a Data Governance Framework? A data governance framework is a detailed plan guiding your company’s implementation of data governance. Think of it as a set of rules and processes that help you manage your data smartly and organized. Following this framework lets you maintain consistency and efficiency in handling your company’s data. Data Management Body of Knowledge (DMBOK): Developed by the Data Management Association (DAMA), DMBOK provides a comprehensive guide to best practices, principles, and processes in data management, including data governance. The Data Governance Institute (DGI) Framework: DGI offers a framework that outlines the components, principles, and practices of data governance, including organizational structures, policies, and processes. Control Objectives for Information and Related Technology (COBIT): COBIT, developed by ISACA, provides a governance and management framework for IT processes, including data governance, focusing on control objectives and maturity models. Open Group Architecture Framework (TOGAF): TOGAF offers a framework for enterprise architecture, which includes aspects of data governance within its broader scope of managing organizational assets and capabilities. The benefits of a data governance framework include: Improved collaboration across the organization to manage all data assets in alignment with corporate objectives. Greater visibility into data governance efforts, leading to effective coordination and control of the data assets. Greater transparency in data management processes, ensuring clarity and accountability. Efficient monitoring of data consumption and usage, guided by a structured blueprint. A robust foundation for regulatory compliance practices, ensuring compliance with industry standards and regulations. The Importance of Data Lineage Data lineage strengthens data governance by providing transparency, control, and accountability over the organization’s data assets. Data lineage systematically tracks data from origin to its various transformations and destinations within an organization’s data architecture. It provides a detailed record of how data moves through different systems, processes, and databases. This information is crucial for ensuring data quality and understanding the impact of changes to data sources or structures. Implementing a unified solution with data lineage capabilities instead of third-party data lineage tools improves data governance. It provides a centralized platform for managing and visualizing data lineage, making it easier to trace data dependencies and identify potential issues. With this visibility, organizations can make informed decisions about data management strategies and ensure that data is used effectively and responsibly across the organization. Learn more about data lineage and the differences between data lineage and data provenance. Data Governance Best Practices A Unified Approach Toward Data Assets Establish a data governance framework involving all types of data assets across the organization, including structured databases, unstructured documents, and semi-structured data from various sources like APIs. This ensures a unified approach to managing data, reducing the risk of overlooking critical data assets and enhancing overall governance effectiveness. Involve Data Stewards Encourage participation from data analysts, engineers, and business users in governance efforts. Leverage their expertise and insights to inform governance decisions and policies. This approach fosters a sense of ownership and responsibility among practitioners, leading to more tailored and effective governance practices aligned with business needs. Integrate Governance into Workflows Integrate governance practices seamlessly into existing workflows and tools used by practitioners. Implement data quality assessments, privacy compliance checks, and access controls directly within your data management solutions to ensure that governance becomes integral to routine operations. This approach minimizes disruptions and encourages widespread adoption of governance practices throughout the organization. Establish Data Governance Metrics Define key performance indicators (KPIs) and metrics to measure the effectiveness of your data governance initiatives. This step includes tracking metrics such as data quality scores, compliance levels, and user satisfaction with data accessibility. By regularly monitoring these metrics, you can identify areas for improvement and demonstrate the value of data governance to stakeholders. Provide Ongoing Training and Education Offer continuous training and educational programs to enhance employee awareness and understanding of data governance principles and practices. This initiative may include training sessions on data management best practices, compliance requirements, and the use of data governance tools and technologies. Investing in employee development can empower your team to play an active role in upholding data governance standards. Learn more about data governance best practices. Investing in Data Governance for Success Data governance is critical to modern business operations, ensuring that data is managed effectively and responsibly throughout its lifecycle. By establishing clear policies, standards, and processes, you can promote accessibility, security, and quality of data assets, fostering collaboration, informed decision-making, and compliance with regulatory requirements. With the involvement of key stakeholders and the adoption of best practices, a robust data governance program can play a role in mitigating risks and achieving strategic objectives. LIKE.TG’s Data Governance Solution LIKE.TG’s data governance solution empowers organizations to leverage these benefits and create a single source of truth for their data. With a strong focus on data quality standards and validation processes, LIKE.TG guarantees the reliability and accuracy of data. Its data ownership initiatives ensure that responsible parties are accountable for data, while data enrichment and discovery functionalities enhance the value of the information available. This comprehensive approach supports informed decisions and strengthens their data-centric foundation for success. Want to learn more about LIKE.TG? Sign up for a demoorcontact us. Take Control of Your Data Assets! With its seamless data sharing and robust access controls, LIKE.TG Data Governance ensures the integrity and security of your data assets. Start your free 14-day trial now and elevate your data governance strategy. Schedule A Demo
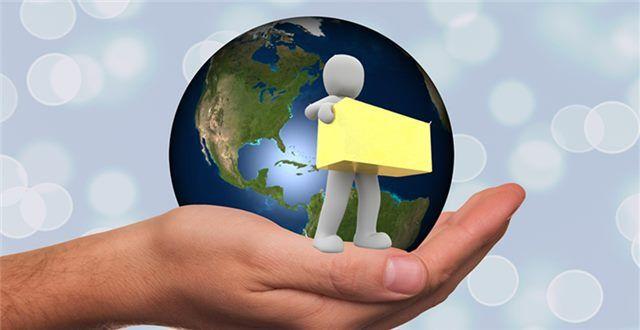
Navigating AI-Driven Claims Processing
95% of insurers are currently accelerating their digital transformation with AI-driven claims processing. Traditionally, this process involved manual steps such as claim initiation, data entry, validation, decision-making, and payout, consuming significant time and resources. However, the introduction of AI has replaced tedious manual work, enabling companies to streamline their tasks efficiently. According to a report by Market Research Future, the AI in insurance market size is expected to grow from USD 4.2 billion in 2022 to USD 40.1 billion by 2030 showing substantial growth and investment in AI. AI-powered claim processing is a one-stop solution for instant detection and rejection of fraudulent claims, delivering accurate damage estimates and risk prevention. Contribution of AI Technology in Claims Processing Implementation of AI in claim management optimizes the process and improves data accuracy by efficiently handling unstructured data. The technology can quickly analyze data from several sources, such as police reports, medical records, and witness statements. This helps insurance companies make faster and well-informed decisions. Various technologies are commonly used in AI -powered document processing, including deep learning, machine learning, and natural language processing. These technologies contribute to seamless, accurate, and efficient AI claim processing through: Deep Learning Deep learning is a sub-category of AI that suits a variety of applications in claim processing. It uses advanced algorithms to help insurance companies accurately and efficiently process claims. Deep learning aids in claim processing by analyzing images and videos. For example, in the case of a car accident, deep learning algorithms can automatically analyze images of damaged vehicles. It can determine the extent of the damage and estimate the repair costs, all without relying on structured inputs like excel files and databases. Machine Learning Machine learning plays an important role in streamlining AI claim processing. It can analyze large amounts of data to recognize patterns and trends in claims data. This data helps in predicting claim outcomes by automating some parts of the claims process. There are tools like Docsumo and Levity AI that uses ML to extract data from unstructured data sources. By using such tools, insurance firms can enhance their processing with reduced time and resources. Natural Language Processing Natural language processing (NLP) has become a vital technological tool in claim processing. It helps insurance companies automate the process of extracting and checking information from unstructured data like claim forms and emails. With NLP, insurance firms can quickly and accurately process claims, which reduces the time it takes to settle claims and keep the customers satisfied. Also, NLP allows insurers to identify fraudulent claims by analyzing language patterns and detecting inconsistencies in claims. Benefits Of Integrating AI-driven Claims Processing Integrating AI in claim processing offers numerous benefits some of these include the following. Enhanced Accuracy in Handling Unstructured Data Manual claim processing can be uncertain due to human error, misinterpretation of information, and inconsistent application of rules. As per the research, human error accounts for up to 82% of data. However, with AI techniques such as convolutional neural networks (CNNs) such errors are significantly reduced. These tools use CNNs to accurately analyze images of damaged properties or to interpret, leading to faster, and accurate claim assessments and more satisfied clients. Improved Speed of Workflows Traditional claim processing is a time-consuming task, such as manual data entry and analysis by employees. However, AI-based systems process vast amounts of data by using machine learning which reduces the time it normally takes to process a claim, particularly beneficial for handling unstructured data. These systems use techniques like natural language processing (NLP) to extract and interpret relevant information from diverse sources like images, PDFs, and emails. This makes processing claims faster, more accurate, and cheaper because it doesn’t need as many people to do the work. Better Customer Satisfaction Every organization wants to keep its customers at the forefront, but manual claim processing often delays their requests and queries. In this regard, AI-powered insurance chatbots have enhanced customer communication by providing instant assistance. This way, companies could respond to customer queries without delay. As a result, many consumers decide to renew their insurance with the same insurer. This is largely due to the convenience of receiving real-time updates on claim status, coverage details, and documentation requirements. As a result, many consumers decide to renew their insurance with the same insurer. So that they can receive real-time updates on claim status, coverage details, and documentation requirements. Compliance With Regulatory Requirements AI claim processing systems are designed to comply with regulatory requirements by ensuring the accuracy and efficiency of claim processing These systems are well-equipped with decent algorithms like anomaly detection and predictive modeling and prevent fraudulent claims. As an outcome, they abide by the security protocols and guard the privacy of sensitive information. Also, AI-powered claim processes are subject to regular audits by regulatory bodies. These audits ensure that they operate per the applicable laws and regulations. Top Predictions for the Future of AI-driven Claims Processing As more insurance companies adopt AI-based claim processing, the following years seem promising. However, the technology will keep evolving, and insurance firms will likely meet the advancements. That is why companies must identify the technologies and their potential to incorporate into their claim processes in the following years. After analyzing the contribution of AI in claim management, it’s time to acknowledge how these technologies will progress in the future. Internet of Things (IoT) for Real-Time Processing The use of Internet of Things (IoT) in real-time claim processing has the potential to revolutionize the insurance industry. In the following years, insurers will collect data for their customers in real time using IoT devices like sensors and wearables. The use of Internet of Things (IoT) in real-time claim processing has the potential to revolutionize the insurance industry. In the following years, insurers will collect data for their customers in real time using IoT devices like sensors and wearables. For example, a car sensor can detect an accident and inform the insurer automatically, who could process the claim immediately. This would save time and reduce the potential for fraudulent claims. Advanced Predictive Analytics for Customized Processing Advanced predictive analysis for customized insurance will likely pose several benefits regarding the future of claim processing. For instance, companies can analyze unstructured data from various sources such as social media, telemetry, and other platforms using predictive analysis. The technology will allow insurers to offer more personalized policies based on individual risk factors, which may reduce overall claims and costs. Collaboration of Blockchain and Artificial Intelligence Experts have predicted that the collaboration of blockchain technology with AI claim processing will result in enhanced speed and accuracy of claim records. One possible scenario is that blockchain technology can securely store and share data related to insurance claims. By creating a distributed ledger system, all parties involved in claim processes can access the same information in real-time, reducing the chances of errors and fraud. Continuous Improvement with Self-Learning It’s no secret that there will be significant improvements in AI claim processing in the coming years. According to the predictions, AI systems will keep learning from each claim processed, making the operations more efficient and authentic. These self-learning systems can adapt to new claims and evolve regulatory landscapes without disturbing the operations. This could lead to faster and better claims processing, boosting client retention rates. In addition, self-learning patterns can identify trends in claim data, while alerting insurers to recognize potential risks and measures to combat them. The Use of AR And VR In AI Claim Assessment Augmented reality (AR) and Virtual Reality (VR) are expected to provide a more immersive and interactive experience for insurers and clients. AR can be used to overlay digital information on real-world objects, allowing insurers to identify and access damage to a property quickly. On the other hand, VR can create a completely stimulated environment, enabling insurers to virtually inspect a property without physically being there. Indeed, the coming years of AI claim processing are bright, especially with these tech predictions backed by experts. Wrapping Up! Looking ahead, the integration of artificial intelligence in claims processing is set to become increasingly significant. The ability of AI to efficiently and accurately extract data from unstructured sources promises to revolutionize the insurance industry. This technological advancement will not only reduce costs for insurance companies but also enhance the customer experience by making the claims process faster and more reliable. In this context, LIKE.TG ReportMiner emerges as a powerful tool. This advanced data extraction software utilizes artificial intelligence to intelligently extract data from unstructured sources. It offers features such as automated data extraction and data validation, ensuring both efficiency and accuracy. Its ability to identify and extract relevant data points from complex sources minimizes errors, thereby enhancing the overall claims process. To experience the capabilities of LIKE.TG ReportMiner firsthand, download the 14-day free trial today. Experience the efficiency and accuracy of AI-powered claim processing with LIKE.TG! Navigating AI-Driven Claim Processing is made seamless with our automation solutions, structuring unstructured data effortlessly for your claims. Download Now
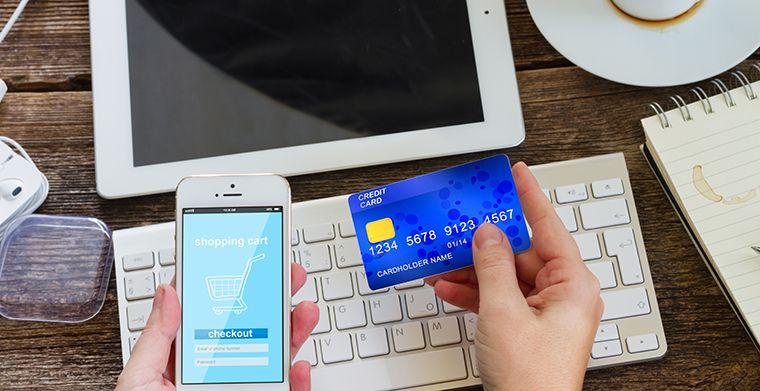
Navigating Workplace Accident Claims with LIKE.TG
The U.S. Bureau of Labor Statistics reports that the incidence rate of nonfatal workplace accidents has decreased over the years, which can be attributed to the implementation of preventive measures in private industry. Despite this positive trend, companies deal with large volumes of unstructured data that demand effective management. Addressing these complexities is easier with LIKE.TG’s unstructured data extraction solution. It allows companies to process and analyze information faster than ever, streamlining workplace accident claims processing. Introducing LIKE.TG’s Unstructured Data Extraction Solution LIKE.TG ReportMiner simplifies data extraction from various sources, especially unstructured data related to workplace accidents. Its user-friendly interface empowers users of all skill levels to effortlessly extract data without extensive IT knowledge.The solution’s main feature is its template-based extraction model. Users can create templates that instruct the tool on identifying and extracting essential data from various sources, like PDFs and scanned documents related to workplace accidents. Essential Features of Report Miner for Streamlining Workplace Accident Claims Some essential features of ReportMiner include; Automated Data Extraction LIKE.TG ReportMiner extracts essential information from complex workplace accident claims documents with great precision. The tool is also capable of handling a range of document formats, including scanned documents that can be transformed into searchable text within the tool. This feature is particularly useful before building an extraction template. It also incorporates different elements within the documents, including tables, headers, footers, key-value pairs, structured data, and any other unique sections within the document according to patterns defined by the user. This allows insurance companies to extract complex data layouts present in workplace accident claims documents, facilitating analysis. Automated Workflows Handling the various stages between initial workplace incident reporting and final resolution can be a challenging task, particularly in the context of workplace accidents. However, with LIKE.TG ReportMiner’s automated workflow functionality, organizations can automate these processes according to their requirements and thus enhance efficiency, compliance, and accuracy throughout the claims management cycle, particularly in addressing workplace incidents. The tool standardizes the data extraction process from unstructured sources and automates it to ensure consistency and accuracy in data handling, thus saving time. ReportMiner’s strength lies in its ability to automate data extraction with validation, ensuring the accuracy of data used for further processing. Data Accuracy and Validation In workplace claims, making sure that the information is correct is crucial. Therefore, LIKE.TG uses advanced techniques to extract accurate information from various sources like scanned images, text files, and PDF documents. These techniques ensure that the data extracted is free of errors and discrepancies that would otherwise arise from manual data entry processes. LIKE.TG is also capable of cross-referencing extracted information against predefined criteria. This is further beneficial in removing any inconsistencies in the data, ensuring the data extracted is highly reliable to be processed further. Claims Standardization Standardization of claims in workplace claims is essential as it ensures that all relevant information such as witness statements, injury details, and incident location follow a consistent pattern. Having standardized extraction templates ensures all the necessary information is captured in a structured and consistent manner, making it easier to analyze and process workplace claims. LIKE.TG ReportMiner standardizes claims processing to improve efficiency and clarity while enhancing communication and collaboration among different departments involved in the claims management lifecycle. Having uniform data fields across all claims ensures the key details are recorded consistently and are easily accessible for reporting and further analysis. The Strategic Benefits of Report Miner for Workplace Accident Claims Extracting valuable insights from vast amounts of data generated in workplace accident claims is now easier with LIKE.TG’s unstructured data extraction solution. It helps insurance companies minimize risks and streamline their operations.Other benefits include: Enhanced Compliance LIKE.TG ReportMiner presents a framework that complies thoroughly with the regulatory requirements and internal policies regarding workplace accident claims. LIKE.TG achieves this by implementing validation processes that involve validating the extracted data against predefined rules, regulations, and policies. It guarantees the accuracy and integrity of extracted data by using advanced algorithms and validation mechanisms. That said, all the necessary data and information concerning accident claims are captured precisely and stored in compliance with industry standards. ReportMiner provides the flexibility to adhere to regulations related to such claims, minimizing the possibility of non-compliance and legal liabilities. Greater Employee and Customer Satisfaction By automating data extraction, employees can focus on more value-added tasks. This results in an increase in employee productivity while minimizing frustration associated with repetitive tasks. Such streamlined claims processing leads to improved communication with claimants that results in higher levels of satisfaction among customers. In essence, ReportMiner contributes to a positive brand image and helps develop long-term relationships with the customers. Reduction of Manual Labor and Paperwork Manual data entry and verification processes are prone to typos, inconsistencies, and human errors, this would compromise the integrity of reports. Automation eliminates the need for manual data entry and verification. This enhances accuracy, streamlines the entire process, and reduces errors in handling workplace accident claims. ReportMiner efficiently extracts incident details, descriptions, and witness statements, saving time and minimizing the chances of errors. Step-by-Step Guide to Processing Workplace Accident Claims with ReportMiner Here, we will investigate how LIKE.TG ReportMiner streamlines the intricate workflow from initial data extraction after report submission to claim resolution, taking the batch of workplace accident claims as the use case. STEP 1: Start by importing the workplace claim PDF form into the data pipeline designer. For this, you can drag the PDF Form Source object from the toolbox to extract data from a fillable PDF file without having to create an extraction template. Step 2: To configure your file, right click on the PDF Form Source and choose Properties from the drop-down menu. A configuration window will appear; now, provide the file path (where your workplace claim PDF is stored) and click next. STEP 3: Select next and a layout builder screen will appear. Here you can modify both the labels of fields and their corresponding data types. Step 4: Then click OK. On the dataflow designer, the PDF Form Source object will display fields according to the layout specified within the PDF form. The claims information or the extracted data lacks structure initially, but it can be further utilized and analyzed by organizing it into an Excel spreadsheet. Data Cleanse transformation can be employed after extracting data to perform data cleansing, such as removing whitespaces. The final version of the extracted data is then written to the Data Destination by specifying a connection to the company’s database. The data extracted here is from a single PDF source only. It can be managed for a whole batch as well. This ensures data from each PDF file is extracted, processed and written to the destination correctly according to the predefined rules. This file presents extracted data from PDF files and arranges them accordingly. It highlights unstructured data extraction and provides a preview of the output data. Wrapping Up! LIKE.TG ReportMiner offers a valuable solution for managing workplace accident claims efficiently. With features like automated data extraction businesses can effortlessly extract and analyze unstructured data related to workplace incidents. By using ReportMiner, organizations can streamline their accident claims process, enhance data accuracy, and ultimately improve workplace safety practices. LIKE.TG ReportMiner stands as a reliable tool for navigating workplace accident claims with ease and confidence. Handle Workplace Claims Effectively with LIKE.TG! Unlock the power of unstructured data with LIKE.TG's guide using Report Miner for workplace accident claims. Streamline your process and gain valuable insights effortlessly. Start your 14-days-trial now!
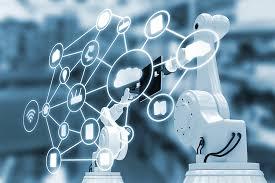
Workplace Claims: A Close Look at the Importance of Quick Settlements
Workplace claims are legal actions or complaints that employees set forth against their employers due to violations of employment laws or contractual agreements. In recent times, employees feel encouraged to speak up for their rights with no workplace harassment, discrimination or unjust treatment. This increased awareness has raised legal standards and regulatory frameworks and thus, employees feel more empowered to report instances of harassment and discrimination. The resulting increased number of claims necessitates advanced automation tools to handle them properly. Such a situation demands automation of the extraction process, categorizing, and data analysis from diverse sources like surveys, employee reports, and incident logs. This helps the insurance companies in gaining actionable insights swiftly and accurately. Such efficiency and responsiveness can address all workplace grievances and promote a healthy work environment. Significance of Unstructured Data in Workplace Claims In workplace claims unstructured data plays a significant role. Here is how: Provides Contextual Insights Unstructured data is a valuable resource in workplace claims because it provides context and depth to the issues at hand. Different data sources, such as chatlogs and emails, can serve as evidence for any workplace claim. For example, if an employee alleges mistreatment, the company can examine communication records to verify the claims. Similarly, in cases of unfair termination, reviewing documents may reveal discrepancies in treatment. This approach ensures that decisions regarding the claim are fair, impartial, and based on information. Presenting concrete evidence enhances the credibility and transparency of the resolution process. Highlights Systematic Issues Unstructured data serves as a source for highlighting trends within a company, especially in the context of workplace claims. For example, if a significant number of claims relate to unfair treatment or discrimination, analyzing communication patterns and document trails can help identify underlying cultural or organizational issues. Such insight can be better achieved with automated data extraction as it enables companies to efficiently sift through large volumes of data. This enables the stakeholders to address root causes effectively, implement targeted interventions, and nurture a more inclusive and respectful workplace culture. Manages Risks Proactively Proactive risk management means companies can take measures to prevent a situation long before it becomes a serious issue. In the context of workplace claims, unstructured data such as chatlogs and emails can help organizations see potential problems in the early stages. Using automated data extraction solutions, they can quickly gather and analyze the information to identify trends and warning signs. For instance, if many employees complain about the same issue, such as unfair treatment, the company can assess the extracted information and address it before it becomes a problem. This approach is less costly in the long run. The Importance of Streamlined Claims Processing through Automation Claims processing facilitated by automation is of utmost importance in handling workplace claims effectively. Here’s why: Ensures Data Integrity Automation promotes data integrity, particularly with unstructured data and preserving data integrity is essential to ensure the reliability of information used in decision-making processes. When claims are processed manually, they are at a higher risk of human error and accidental data loss during handling. However, automation reduces such risks as these procedures implement standardized processes and protocols data management and preservation. Automated systems are almost always secured with strong security measures to protect data from tampering and unauthorized access. Furthermore, automation protects sensitive information and maintains its integrity throughout its lifecycle. Greater Cash Flow Traditional claims processing methods involve lengthy administrative procedures, which incur significant costs and consume a lot of time too. However, if the same process is automated, it would streamline the entire claims process all the way from initial submission to resolution. This results in better and more efficient outcomes. Such swift resolutions facilitated by automation also alleviate the financial strain caused by prolonged settlement negotiations. With a reduced turnaround time, companies can minimize the resources expended on legal fees and can maintain a healthy financial position. That said, a company with enhanced cash flow would be better able to reinvest resources into core business activities, research, development, and talent acquisition. Promotes Compliance with Legal Obligation The best practice to protect the company’s reputation during workplace claims is compliance with legal obligations, laws, industry standards, and regulations. Automation plays an important role in ensuring companies adhere to regulatory timelines. They help organizations meet the deadlines set by regulatory bodies and legal frameworks. Such automated systems also allow for better tracking and interpreting of legal requirements. Automation also facilitates the implementation of standardized processes that reduces the risks of errors, which could otherwise lead to regulatory non-compliance. Helps Save Time and Resources Resolving workplace claims is time-consuming. It demands both time and money. However, with automation, companies can ensure faster claims processing and quick settlements. This would avoid lengthy disputes and reach mutually acceptable resolutions in almost no time. By automating the key aspects of the claims resolution process, like data collection, analysis, and communication, companies can significantly reduce the time and resources required to address workplace claims. With quick settlements, the resources can be allocated efficiently, and the disruptions of business operations can also be avoided. Adaptability and Agility Using automated systems, companies can enhance the speed and accuracy of claim resolutions. It also facilitates the implementation of measures to prevent the recurrence of workplace issues. Analyzing data trends and patterns enables organizations to identify potential areas of concerns. Companies that embrace automated systems show adaptability and agility in responding to challenges in the workplace and other changing market dynamics. Ensuring that workplace claims get addressed swiftly, organizations can focus on the real deal. How Technology is Facilitating Quick Settlements Traditionally, claims management has remained a time-consuming activity and complex process for enterprises. Modern technology has optimized this process, but it can now be done better through automated data extraction solutions. This allows for quick settlements, which is advantageous. AI-Powered Data Extraction: Technology-enabled claim settlements are now eased through automation. AI has made unstructured data processing easier. Using AI, companies can automatically extract bulk data from different sources such as documents, forms, and emails. In workplace claims processing, AI powered data extraction can be employed to handle tasks like data entry and validation. This speeds up claim processing and reduces manual work. Optical Character Recognition (OCR): OCR digitizes and extracts text from documents, including scanned images and PDFs. This technology proves beneficial in the rapid conversion of physical documents into editable and searchable digital files. Pattern Recognition: Efficient settlement procedures rely on the effective use of pattern recognition, particularly through Machine Learning (ML) algorithms. These algorithms are essential for speeding up the resolution of workplace claims by continuously improving their ability to understand and organize data patterns in documents. By analyzing processed documents ML algorithms become better at recognizing patterns, making it faster to identify valuable information. Wrapping Up! While unstructured data can be challenging for workplace claim processing, automated data extraction solutions, such as LIKE.TG ReportMiner, have significantly optimized the system. By effortlessly handling various data sources and supporting multiple formats, ReportMiner reduces the manual effort required, thereby streamlining the entire data extraction process. Moreover, by leveraging advanced AI technology, ReportMiner saves time and enhances accuracy. This streamlined approach benefits employee satisfaction and workplace harmony and expedites workplace claims settlements, ultimately benefiting both employees and employers alike. Ready to experience the efficiency of LIKE.TG ReportMiner? Sign up for our 14-day trial and request a personalized demo today to witness how it can simplify your workflow! Automate Your Claims with LIKE.TG Discover the power of efficient data extraction with LIKE.TG's Report Miner! Download your free trial today. Download Now
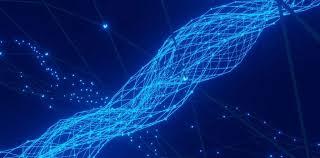
BigQuery vs. Redshift: Which One Should You Choose?
Considering BigQuery vs. Redshift for your data warehousing needs? This guide is for you. Both BigQuery and Redshift stand as leading cloud data warehouse solutions each offering a multitude of features catering to multiple use cases. Google’s BigQuery offers seamless scalability and performance within its cloud platform, while Amazon’s Redshift provides great parallel processing and tuning options. Let’s simplify the decision-making process by breaking down the differences between Redshift vs. BigQuery, helping you find the perfect fit for your business. What is Google BigQuery? Google BigQuery is a part of Google’s overall cloud architecture, the Google Cloud Platform (GCP). It operates as a serverless and fully managed service, eliminating the need for managing infrastructure and allowing businesses to prioritize data analysis and insight generation. Google BigQuery is built on top of Dremel technology, which is a query service that enables users to run SQL-like queries. At its core, Dremel uses a distributed execution model that distributes the workload across multiple nodes within Google’s infrastructure. BigQuery is among the initial major data warehouses, featuring exceptional query performance. Using Google’s infrastructure and technologies, such as Capacitor, Juniper, Borg, and Colossus, BigQuery can execute complex analytical queries against massive datasets within seconds. What is Amazon Redshift? Amazon Redshift is the first-ever cloud data warehouse that offers a fully managed, petabyte-scale service. Redshift is designed to manage large datasets and complex analytical queries with high performance. Amazon acquired the primary source code for Redshift from ParAccel, which is a company that was developing the ParAccel Analytic Database (a PostgreSQL-based database). Redshift is built on the PostgreSQL fork but has a lot of unique capabilities. For instance, Redshift has a unique column structure and makes use of distribution styles and keys for data organization. Since Redshift is designed to handle large amounts of data, companies can scale the data warehouse up or down based on their requirements to easily accommodate data volumes. Moreover, there are no charges incurred when the warehouse is idle, which means you pay only for what you use. Build your data warehouse seamlessly, without a single line of code. Discover how LIKE.TG's DW Builder transforms data integration with native connectivity to BigQuery and Redshift. Check Demo Now! BigQuery vs. Redshift: Architecture When comparing Google BigQuery to Amazon Redshift in terms of architecture, there are some key differences to consider. Firstly, BigQuery operates on a serverless architecture, while Redshift offers greater overall control.In BigQuery, Google manages all the aspects of the warehouse, including provisioning, scaling, and maintenance. But it abstracts the users from the BigQuery infrastructure. With this approach, users can focus on processing massive datasets without having to worry about infrastructure management. The resources are allocated automatically depending on the number of queries you execute. On the other hand, Amazon Redshift follows a more traditional architecture that is based on a cluster of nodes. This architecture includes a leader node that takes care of client connection and query execution, while multiple compute nodes store and process data. Redshift uses a massive parallel processing (MPP) architecture to parallelize and distribute the queries across compute nodes. Redshift generally allows you to have better control over your resources so you can manage tasks, including scaling, patching, and backup. BigQuery vs. Redshift: Scalability Scalability is mainly limited by three major factors that is lack of dedicated resources, continuous ingestion, and tightly coupled storage and compute resources. BigQuery has a serverless architecture and it automates resource provision and scaling. Therefore, scaling is well-planned and well-structured in the case of BigQuery. It generally works on either on-demand pricing or flat-rate pricing. In the on-demand pricing model, assigning slots (compute resources) is fully controlled by BigQuery, whereas the flat-rate pricing model reserves slots in advance. The auto-scaling capability generally suits companies with fluctuating data volumes or unpredictable workloads. In contrast, Amazon Redshift cannot distribute the load across clusters even with RA3. This limits its scalability. To support the query concurrency, it can scale up to 10 clusters easily; however, Redshift can handle only 50 queued queries across all clusters. Though Redshift is scalable, its manual cluster management approach requires monitoring and configuration adjustments that would potentially introduce complexity. In a test run by an independent researcher, it was found that BigQuery was significantly faster than Redshift when dealing with a large dataset, which may suggest better scalability for BigQuery. However, note that several of these tests have been performed, and deciding on a clear winner is easier said than done. BigQuery vs. Redshift: Performance Comparing the performance of Redshift and BigQuery involves considering factors like concurrency, optimization techniques, query speed, and data processing capabilities. Considering that both BigQuery and Redshift are run by tech giants the differences in their performance are negligible. The columnar storage format and distributed execution model of BigQuery enable parallel processing of queries across multiple servers that results in rapid data retrieval and analysis. Moreover, its automatic query optimization features, including execution plans and dynamic query reordering, enhance query performance and efficiency. This minimizes latency and maximizes throughput. That said, BigQuery is a great solution for real-time analytics and interactive querying cases where speed and responsiveness are of considerable importance. BigQuery also has a built-in caching mechanism that automatically caches the results of every query for 24 hours, which can significantly speed up repeated queries. However, for small, ad-hoc queries, BigQuery may be slower than Redshift due to its reliance on distributed computing. On the other hand, the Amazon Redshift is manufactured on a massively parallel processing (MPP) architecture that allows it to perform well for data warehousing and analytical workloads. Redshift has more tuning options than many others, but you cannot expect it to deliver much faster compute performance than other cloud data warehouses. Redshift also offers workload management features, including query queues and concurrency scaling, to prioritize and manage query execution based on user-defined criteria. However, its manual cluster management approach may introduce overhead in terms of cluster configuration and maintenance, impacting its overall performance. Redshift vs. BigQuery: Which One to Choose? When choosing between the two, companies should assess their preferences and requirements before picking any of these data warehouses. Here are a few use cases to help you decide. When to Use Google BigQuery Large-Scale Data Analytics: BigQuery’s serverless architecture and ability to handle petabytes of data make it an ideal choice for large-scale data analytics. Data Exploration: BigQuery is designed for ad-hoc analysis and data exploration. It allows users to perform SQL-like queries on big datasets. Real-Time Analytics: BigQuery supports real-time analytics through its streaming API, making it perfect for analyzing live data. Integration with Google Ecosystem: If your organization already uses Google Cloud Platform services, using BigQuery can provide seamless integration. When to Use Amazon Redshift: Complex Query Execution: Redshift maintains a strong performance when executing complex, compute-heavy queries. Its column-based storage and MPP architecture are designed for this purpose. Data Warehousing Operations: Redshift is ideal for traditional data warehouse operations, where the primary requirement is storing structured and semi-structured data. Predictable Pricing: If predictable pricing is a priority, Redshift may be a better choice as its pricing is per node, which can often be more predictable and affordable. Integration with AWS Ecosystem: If your organization is already invested in the AWS ecosystem, using Redshift can simplify data warehousing operations. The Path Forward: Future-Proof Data Warehousing For future-proof data warehousing, it’s significant to select a solution that can adapt to evolving data demands and analysis technologies. Here is what you can expect from Redshift and BigQuery in the future. BigQuery’s Petabyte Scale: BigQuery has the capability to manage large datasets without any hassle. No matter if it requires dealing with customer transaction data or years of dealing with billions of sensor readings from IoT devices, BigQuery can handle all that efficiently by accommodating your data needs. This scalability is advantageous for enterprises that expect sustained growth in their data volumes over time. Redshift’s Real-time Options: Despite its emphasis on batch processing, Redshift offers real-time analytics functionalities through its integration with Amazon Kinesis Firehouse. With this, near real-time data ingestion into Redshift becomes possible. This turns out beneficial in cases that require immediate insights, like stock price monitoring and fraud detection.While this feature addresses some real-time needs, those looking for instantaneous analytics at scale will find BigQuery a more suitable option due to its inherent design for better performance for low-latency queries and real-time processing. Choosing the Right Future-Proof Platform Choosing the ideal data warehousing solution for future-proofing your infrastructure depends upon the specific needs and priorities of your organization. Here’s a guide to help you pick the right one: Looking for AI/ML Integration? Choose BigQuery as it stands out for seamless integration with Google’s AI and machine learning tools like Vertex AI and TensorFlow. This native integration allows for easy analysis and enables the development of ML models directly within the data warehouse environment. Want to Focus More on Real-time Analytics? BigQuery emerges as a superior choice. It features serverless architecture and automatic scaling, achieving real-time insights with minimal latency. That’s difficult with Redshift as it may require additional configuration and management overhead to handle real-time data effectively. Have Significant Investments in AWS? Consider Redshift as it offers tight integration with other AWS services. By using Redshift, you can ensure seamless interoperability and maximize the benefits of existing AWS infrastructure. Looking for a Completely Serverless Architecture? BigQuery is the optimal choice. It runs on a fully serverless architecture that eliminates the need for any kind of server management. That said, scalability and resource allocation become easier. Considering the Integration of Unstructured Data? Go ahead with Redshift with Spectrum, as it has better capabilities for analyzing some forms of unstructured data. However, if the data primarily consists of unstructured formats like text and images, BigQuery will be a better option as it provides better built-in features for handling such unstructured data. Working with Massive Datasets? BigQuery will be your perfect companion as it excels at handling massive datasets. It can manage large data volumes and ensures optimal performance and scalability even with your growing data needs. Evaluating these considerations and aligning them with your business’s objectives and requirements will help you choose a future-proof data warehousing platform that positions you to continue to leverage the power of data for years to come. LIKE.TG Provides Native Connectivity to Redshift and BigQuery This wraps up our “BigQuery vs. Redshift” comparison. Both platforms offer high-performance and scalable cloud data warehousing, each with its own set of features, pricing models, and usability. Being supported by tech giants like Amazon and Google, either option is a solid choice. However, selecting the one that fits your data warehousing needs is essential. LIKE.TG provides native support for both BigQuery and Redshift. Whether you’re migrating an existing data warehouse or creating a new one, our no-code platform, LIKE.TG DW Builder, enables you to design, develop, and deploy enterprise-grade data warehouses quickly and efficiently. Start your 14 days trial now! Start Your DW Migration Now with LIKE.TG! Migrate to any of your favorite data warehouses through LIKE.TG DW Builder. Get in touch with us today to get your 14-day free trial. Start your 14-days-trial now!
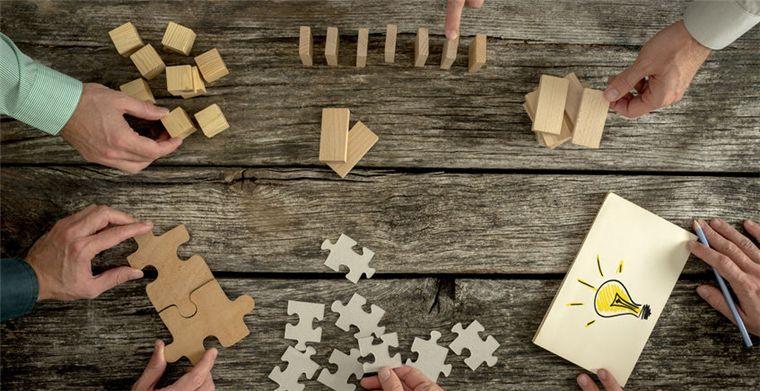
ETL Testing: Processes, Types, and Best Practices
ETL testing is a set of procedures used to evaluate and validate the data integration process in a data warehouse environment. In other words, it’s a way to verify that the data from your source systems is extracted, transformed, and loaded into the target storage as required by your business rules. ETL (Extract, Transform, Load) is how data integration tools and BI platforms primarily turn data into actionable insights. During an ETL process, ETL tools extract data from a designated source, transform it into a structure and format supported by the destination system, and load it into a centralized storage location, typically a cloud data warehouse or data lake. ETL testing comprises different tests conducted at different stages of the ETL process. These tests validate and verify the data to ensure accuracy and minimize data loss. This blog offers an in-depth discussion on ETL testing and its types, its necessity, the steps it entails, and how to do it right. What is ETL Testing? Data now heavily impacts businesses at all levels, from everyday operations to strategic decisions. This growing role has driven the global business intelligence (BI) and analytics tools market to an estimated value of nearly $17 billion. The data contained in a data warehouse is often your enterprise’s only source for generating insights and creating actionable strategies. Therefore, it must only contain accurate, reliable, and up-to-date data if it’s to serve as an effective single source of truth for your business. Automation significantly minimizes the likelihood of errors during ETL, which are otherwise prevalent in a manual ETL pipeline. However, automation must be supplemented with additional validation measures — and this is where ETL testing is useful for the following reasons: It mitigates errors left undetected by automation. It ensures the centralized data’s health and quality. It acts as an independent layer of verification, offering further assurance that the automated ETL process is functioning correctly and producing the expected results. Database Testing vs ETL Testing Database testing is a data validation procedure similar to ETL testing, but the similarities end there. Here are some differences between the two: ETL Testing Database Testing Typically performed on data in a data warehouse. Generally performed on transactional systems. Primary purpose is to ensure that the data being tested is moving as it’s supposed to. Aims to ensure that all data follows the data model’s predefined rules. Verifies that source counts match the counts in the destination. Verifies the absence of orphan records. Checks for duplications in the loaded data. Checks for redundant tables, missing data in columns, and database normalization. Why We Need ETL Testing You can consider skipping ETL testing if you have a simple data flow with minimal transformations, and your source and target systems are stable and reliable. However, assessing your data processes’ complexity should be a standard practice before forgoing testing — this can help you avoid data inconsistencies and errors downstream. There are many instances when ETL testing proves valuable, some of these include: After data integration or migration projects are completed. When loading data into a newly configured data warehouse for the first time. When adding a new data source to an existing data warehouse. During data movement and transformation. When there are suspected errors in ETL processes preventing them from running normally. When there’s a lack of confidence in data quality in either a source or the destination. The ETL Testing Process Testing protocols are subjective and customizable to an enterprise’s requirements and processes. As such, there isn’t a one-size-fits-all model for ETL testing. However, ETL testing typically comprises the following steps: 1. Understanding Business Requirements Designing an effective ETL testing process requires understanding your organization’s business requirements. This involves examining its data models, business workflows, reports, sources and destinations, and data pipelines. This understanding enables ETL testers to know what they’re testing and why. 2. Data Source Identification and Validation In the next part of the ETL testing process, identify your source data and perform schema checks, table validation, and other initial checks. This is to ensure that the ETL testing process aligns with the requirements identified by studying your business model and workflows. 3. Creating and Executing Test Cases Source-to-target mapping and test case design are the next steps, and often include the following: Transformations based on business test cases SQL scripts for conducting source-to-target comparisons Execution flows Following the preparation and validation of these elements, test cases are executed in a staging environment. Typically, testers can’t use sensitive production data, which necessitates the availability ofreal-time synthetic data. You can create this data manually or through test data generation tools. 4. Data Extraction and Reporting In the next step, perform the ETL tests according to business requirements and use cases. During test case execution, identify the different kinds of errors or defects, try to reproduce them, and log them with adequate details and screenshots. In the reporting stage, you can record defects onto specialized Defect Management Systems and assign them to designated personnel for fixing. For successful resolution of errors, analysts need to provide the following: Sufficient documentation for reproducing the test case Relevant screenshots A comparison between the actual and expected results for each test case 5. Applying Transformations Next, you must ensure that data is adequately transformed to match the destination data warehouse’s schema. Besides validating the data flow, you’ll also check the data threshold and alignment. The goal here is to verify that the data type for each table and column matches the mapping document. 6. Loading Data into The Data Warehouse You’ll do a record count check both before and after moving the data from the staging environment to the data warehouse. Verify rejection of the invalid data and acceptance of the default values. 7. Re-Testing the Bug (Regression Testing) After fixing the bug, retest it in the same staging environment to verify that it doesn’t have any traces left. Regression testing also helps ensure that no new defects occur while correcting the previous one. 8. Summary Report and Test Closure In the final step, close reports detailing the defects and test caseswith comments and all related documentation. Before closing the summary report, test its options, filters, layout, and export functionality. The summary report details the testing process and its results, and lets stakeholders know if and why a step wasn’t completed. Types of ETL Testing Each step of the ETL testing process involves different types of tests, some of which are: 1. Production Validation and Reconciliation This test validates the order and logic of the data while it’s loaded into the production systems. It compares the production system data with the source data to prevent non-compliance, data errors, or faults in the schema. 2. Source-to-Target Validation This test checks that the source system data count matches the data loaded in the destination system/warehouse. 3. Metadata Testing This test matches the data types, indexes, lengths, constraints, schemas, and values between the source and target systems. 4. Completeness Testing This verifies that all source data is loaded into the destination system without duplication, repetition, or loss. 5. Transformation Testing When multiple transformations are applied to one type of data, this test helps confirm that all data has transformed consistently based on the applicable rules. 6. Accuracy Testing Following the completion of all transformations, the data’s accuracy is verified. There can be changes in the data’s formats and schema, but the information and its quality should not change during the transformations. 7. Data Quality Testing This testing type focuses on data quality to identify invalid characters, precisions, nulls, and patterns. It reports any invalid data. 8. Report Testing This type of testing checks the data in the summary report, determines if the layout and functionality are appropriate, and performs calculations for additional analytical requirements. 9. Application Migration Testing Application migration testing verifies whether the ETL application is functioning properly following migration to a new platform or box. 10. Data and Constraint Checks This testing technique checks the datatype, length, index, and constraints. Common Challenges in ETL Testing There are several factors that can disrupt or adversely affect the ETL testing process. Below are the challenges that ETL testers encounter the most: Complex Data Transformations and Processes: Applying multiple transformations to expansive datasets can be a complicated and time-intensive procedure. The same happens when too many complex data integrations and business processes exist. Poor Data Quality: ETL testing requires accurate, clean, and high-quality data for the best results. Poor input data quality can affect the reliability of the process. Resource-Intensive: ETL testing can become resource-intensive when large, complicated source systems are involved. Decreased Performance: Big data volumes can hinder processing or end-to-end performance, ultimately affecting data accuracy and completeness. Changes in Data Sources: Any changes in the data sources can potentially impact data accuracy, completeness, and quality. Personnel Requirements: Enterprises need people with experience in ETL and data expertise to ensure the design and implementation of robust ETL testing processes. ETL Testing Best Practices The following best practices can help you optimize your ETL testing processes: 1. Working with Business Test Cases It’s not enough to develop a functional ETL testing process. It also needs to cater to varying business requirements that are different for every organization. An ETL testing process should complement your existing workflow, not disrupt it. 2. Using Clean Source Data Clean source data is a fundamental requirement for an effective ETL testing process. Rather than leaving it for the end, you should start ETL testing with clean source data to save time and obtain better results. 3. Testing for Efficiency Working with BI tools necessitates consistent access to updated data, so you should ensure both data completion and quick data delivery. Optimize the ETL testing process for accelerated testing and speedy results. 4. Automation While full automation is ideal, even partial automation is better than zero automation. Automated tools like LIKE.TG allow you to streamline the ETL testing process while resolving the common challenges associated with manual work. Experience Faster and More Reliable ETL Automation LIKE.TG's all-in-one ETL solution is what your enterprise needs for streamlined ETL testing. Ensure top-notch data quality at all times while enjoying no-code convenience. Get started today! Start My FREE Trial Choosing The Right ETL Testing Tool Here are a few factors you should keep in mind when choosing an ETL testing tool for your business: Intuitive Interface An intuitive interface makes it easy for you to design and implement ETL process. Look for a graphical interface with drag-and-drop functionality for enhance ease-of-use and accessibility. Automated Code Generation Automated coding can eliminate the effort and time it takes for manually coding and developing processes. It can also significantly reduce errors. Built-in Data Connectors Your ETL testing tool of choice should be equipped with built-in data connectors. These connectors can improve data access and make it easier to work with your go-to file formats, databases, legacy systems, or packaged applications. Content Management Features Content management capabilities enable rapid context switching between ETL development, testing, and production environments. Debugging Features An ETL testing tool with powerful debugging features can help you keep up with data flows in real time and quickly create reports on row-by-row behavior. Conclusion Accurate data analysis is crucial for any organization wanting to leverage its data to get ahead in its industry. When done right, ETL testing can boost an organization’s confidence in its data by improving its integrity and reliability. The resulting business intelligence is sound and helps mitigate business risk. Additionally, automated ETL testing tools boost a data warehouse’s stability and performance faster than manual coding, make the ETL process more organized and manageable, and enable the rapid identification and correction of anomalies in the ETL process.Businesses today are moving away from manual ETL testing and integrating automated ETL testing solutions into their workflow to reap these benefits. Yours can too. LIKE.TG is an ETL automation solution with built-in features for ETL testing, integration, validation, transformation, and more. Its ETL automation and advanced profiling capabilities allow users to reconcile data at every step of the ETL process easily. Verifying data quality and identifying errors is easier with LIKE.TG’s simple, no-code, drag-and-drop interface. Find out how LIKE.TG can transform your ETL procedures and help you maintain data quality for accurate analyses. Reach out to our team or start your FREE trial today.
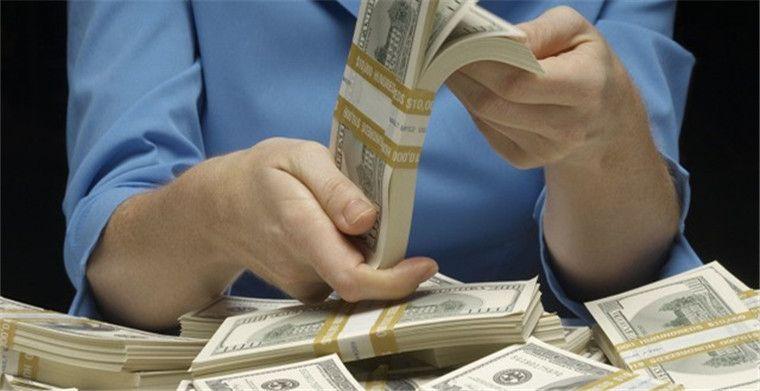
Automated Claims Processing: A Comprehensive Guide
Claims processing is a multi-faceted operation integral to the insurance, healthcare, and finance industries. It’s a comprehensive procedure that involves carefully examining a claim. Claim processing is not a single-step process; instead, it involves multiple stages, each serving as a critical control point to ensure the accuracy and fairness of the claim resolution. Industries are increasingly adopting automated claims processing to boost efficiency and accuracy in this critical function. According to a Statista study, 25% of insurance companies are exploring the possibility of transitioning to automation for claims processing in the foreseeable future. This technology-driven approach uses advanced tools to automate the traditionally manual stages of claims processing. It offers a more streamlined, accurate, and time-efficient method of handling claims. While it significantly reduces the potential for human error, it also retains the need for human oversight in complex decision-making scenarios. This automation marks a significant step forward in claims management, offering the potential for improved efficiency and customer satisfaction. How Does Claims Processing Work? Claim Submission: The claims process is initiated when the policyholder files a First Notice of Loss (FNOL) to the insurance company. This step is the policyholder’s first communication with the insurer regarding a loss, providing initial details about the incident. Digital technology simplifies this step, allowing policyholders to submit their FNOL through digital platforms such as mobile apps or online portals, enhancing accessibility and efficiency. Claim Review: Upon receipt of the FNOL, the claim handler meticulously reviews the policy to determine the coverage. This stage thoroughly examines the policy terms and conditions and the claim’s specifics. The review process is significantly streamlined by automation, which detects crucial policy terms and cross-references the claimant’s details with external databases, ensuring a comprehensive and accurate review. Claim Verification: The insurer then proceeds to authenticate the claim by collecting additional data. This step may include damage assessments, incident photographs, witness statements, or relevant health documentation. The verification process is expedited by automation, which gathers data from various sources, enhancing the speed and precision of the process. Claim Decision: The next step involves the insurer evaluating whether to approve or deny the claim based on the policy terms and the verified claim details. Automation aids this stage by applying pre-set guidelines to establish the payout and coverage. However, a provision for human intervention is maintained for final checks to ensure fairness and accuracy, combining automation’s efficiency with humans’ nuanced decision-making ability. Payment Disbursement: Once the claim is approved, the insurer initiates the payment to the claimant. This step involves calculating the final settlement amount and arranging for its disbursement. The payment process is enhanced by automation, which uses digital payment methods, ensuring swift transactions and clear records, thereby enhancing transparency and traceability. Claim Data Analysis: After the completion of the claims process, the insurer can conduct an in-depth analysis of the claims data. Automation tools facilitate this analysis by providing structured data for easy examination and interpretation. The analysis offers valuable insights, enabling insurers to spot trends, detect potential fraudulent activities, and identify areas for process enhancement. Manual vs Automated Claims Processing Imagine a scenario where a policyholder is involved in a car accident. In a traditional manual claims processing system, the policyholder first must report the accident to the insurance company, often by phone or email. They would then need to fill out a detailed claim form and gather supporting documents such as photographs of the damage, a copy of the police report, and any medical reports if there were injuries. Once these documents are submitted, a claims handler at the insurance company takes over. The handler manually reviews each document, extracting necessary information such as the date, time, and location of the accident, the extent of the damage, and the cost of any medical treatments. This extraction process is time-consuming and requires meticulous attention to detail to ensure no information is missed. The claims adjuster then verifies the extracted information. They cross-reference the accident details with the police report, check the medical bills against the treatment details provided by the hospital, and compare the photos of the damage with the auto repair shop’s estimate. This manual verification process is tedious and prone to human error. The Shift to Automation Insurance companies increasingly seek ways to automate claims processing to mitigate these challenges. Automation speeds up the process and enhances accuracy and efficiency, reducing costs, minimizing errors, and improving customer satisfaction. According to McKinsey, automation can potentially reduce the expenses associated with a claims journey by up to 30%. A key technology driving this transformation is Intelligent Document Processing (IDP). IDP combines the power of artificial intelligence and Optical Character Recognition (OCR) to extract data from unstructured documents, such as claims forms. In claims processing, IDP can significantly speed up the process by automating tasks such as data extraction from claim forms and claim verification. For instance, OCR technology can scan and extract data from claim forms, regardless of their formats or sources. The artificial intelligence component of IDP ensures the accuracy of this process, enabling the system to learn from experience, identify patterns, make connections, and even understand the context of the extracted data. By harnessing the power of IDP, insurance companies can deliver quicker and more accurate claim decisions, enhancing their service and improving customer satisfaction. Here’s a comparison table to illustrate the differences: Manual Claims Processing Automated Claims Processing Speed Slower due to human intervention at each step Faster due to automation at each step Accuracy Prone to human error Higher accuracy due to automated checks Efficiency Less efficient due to manual handling More efficient due to streamlined processes Cost Higher operational costs Lower operational costs Data Analysis Limited and time-consuming Structured data for easy and quick analysis Transparency and Traceability Limited Enhanced due to digital records Who Benefits from Claims Processing Automation? Automated extraction of unstructured data has reshaped the insurance industry, bringing about significant improvements in four key areas: Claims Processing: Automation allows for swiftly extracting pertinent information from various data sources, making manual data entry redundant. Reducing errors and accelerating the claims process leads to substantial cost savings for insurance companies and expedites claim settlements for policyholders. Additionally, real-time tracking of claims, an automated feature, provides clients with unprecedented transparency. Claims Investigation: Identifying patterns and anomalies in vast amounts of unstructured data has proven invaluable in claims investigation. Such proactive measures minimize losses due to fraudulent claims and ensure that policyholders’ premiums are not unjustly inflated to cover the cost of fraud. The integrity of the insurance system is maintained, protecting the interests of honest policyholders. Claims Management: Automation revolutionizes the claims management process by easily compiling relevant information from various data sources. This efficient system improves resource allocation and decision-making for insurance companies. It keeps policyholders informed about the status of their claims, resulting in a more organized and efficient claims management process. Customer Service: Extracting relevant information from unstructured data sources, such as emails and social media posts, enables insurance companies to respond to customer inquiries quickly and accurately. This process leads to higher customer satisfaction and enhances the overall customer experience. Furthermore, personalized communication with customers, another feature facilitated by automation, adds another layer to the customer service experience. Benefits of Automated Claims Processing Automated claims processing brings forth numerous benefits, enhancing efficiency, accuracy, and customer satisfaction in claims processing. Some notable benefits include: Accelerated Claims Lifecycle: Automated claims processing significantly reduces the time it takes from the initial submission of a claim to its final settlement. It swiftly extracts and processes relevant information from unstructured data, such as medical reports, accident scene photographs, or witness testimonies. This acceleration of the claims lifecycle improves operational efficiency and enhances the claimant’s experience by providing quicker resolutions. Intelligent Claims Triage: Automated systems can categorize claims based on their complexity or potential risk by analyzing unstructured data. Simple claims can be fast-tracked for immediate settlement, while complex or high-risk claims can be routed to experienced adjusters for detailed investigation. This intelligent triage optimizes resource allocation and ensures that each claim receives the appropriate level of attention. Claims Surge Management: In situations where there is a surge in claims volume, such as after a natural disaster or a large-scale accident, automated claims processing is invaluable. The system can handle large claims simultaneously, extracting data from various sources and processing claims swiftly. Therefore, insurance companies can efficiently assist customers and maintain consistent service levels. Enhanced Fraud Detection: Given that an estimated 5-10% of insurance claims are fraudulent, claims processing automation becomes critical. It helps claim adjusters detect patterns and anomalies in claims data that may signal fraudulent activity. They can also use this technology to cross-reference the claim data with historical data, policy terms, and industry benchmarks. Any discrepancies or unusual patterns are flagged for further investigation, enabling insurance companies to mitigate risks, reduce losses, and maintain the integrity of their operations. Use Cases of Automated Claims Processing While automated claims processing benefits insurers and policyholders, its impact extends beyond these advantages. This technology has been instrumental in streamlining operations across various industries, primarily: Healthcare Insurance The healthcare sector often grapples with the complexity and volume of insurance claims. In this context, automated claims processing is crucial. The automated system is designed to extract and process data from medical reports and hospital bills. It then cross-references each claim against the specific health insurance policy terms, verifying covered treatments, policy limits, and deductibles, ensuring that each aspect of the claim aligns with the policy provisions. Automation ensures accurate, fair, and timely claim settlements, significantly enhancing the efficiency of the healthcare insurance process. Auto Insurance The auto insurance industry, with its diverse range of claims from minor damages to total loss, requires a robust and efficient system for claims processing. Automated claims processing can efficiently manage data from accident reports and repair bills, determining whether the claim is valid and within the policy’s coverage. This step is crucial in maintaining the integrity of the insurance process and preventing fraudulent claims. Subsequently, the system calculates the settlement amount, reducing processing time, minimizing human errors, and improving customer trust in the auto insurance process. Employment Insurance Workers’ compensation claims, a significant component of employment insurance, can be complex due to the intricate nature of the data involved. Automated claims processing simplifies this process by extracting relevant data from injury reports, medical documents, and employment records. It verifies each claim against the workers’ compensation policy and calculates the compensation amount, expediting the claims process, ensuring accurate compensation, and improving employee satisfaction. Best Practices for Automating Claims Processing Transitioning to an automated claims processing system can significantly enhance an organization’s operational efficiency. However, to maximize the benefits of automation, it’s essential to adhere to certain best practices. These guidelines can help ensure a successful transition and optimal utilization of the automation system: Set Clear Objectives: Successful automation begins with clear and defined objectives. An organization should pinpoint the specific outcomes it aims to achieve with automation, such as reducing claim resolution time, enhancing accuracy, or elevating customer service levels. Choose the Right Technology: The selection of automation technology should be tailored to the organization’s specific needs, with the capacity to integrate with existing systems and handle the complexity of the claims process. Prioritize Data Security: Given the sensitive nature of data involved in claims processing, it’s essential for the chosen solution to adhere to data security standards and regulations, safeguarding customer information. Train Staff: Despite automation, the human element remains vital. Therefore, staff training on the new system is necessary to ensure effective usage and prompt issue resolution. Regularly Review and Update: As technology and business needs evolve, it’s essential for an organization to regularly review and update the automation system, ensuring it continues to meet changing needs and stays abreast of technological advancements. The 3 Must-Haves of an Automated Claims Processing Solution While implementing automation in claims processing, selecting a solution that meets specific needs and boosts operational efficiency is crucial. The ideal solution can significantly streamline the claims process. Here are three key features to consider during selection: System Interconnectivity: A solution should seamlessly integrate with important systems such as CRM, policy administration, and billing. This integration facilitates smooth data flow, eliminates data duplication, and boosts efficiency, leading to quicker and more accurate claim resolutions. Diverse Data Management: An automation system should be adept at handling a vast amount of unstructured and disparate data. A system with this capability enables faster and more precise data processing, crucial for extracting necessary information for claim resolution and accelerating the claims process. Unified Data Access: An automation system should centralize data from various sources into a single repository. A system with this feature promotes better collaboration, quicker decision-making, and constant system availability, all of which are key in streamlining the claims process and enhancing its efficiency. Conclusion Integrating automated claims processing with a focus on managing unstructured data marks a pivotal advancement in the insurance industry. This sophisticated approach streamlines cumbersome processes and enables insurers to extract valuable insights from previously untapped sources. Organizations can enhance efficiency, accuracy, and customer satisfaction by leveraging cutting-edge technologies like artificial intelligence and natural language processing. However, successful implementation requires adopting a robust solution. This is where LIKE.TG steps in. It offers a comprehensive suite of features to meet the unique demands of insurers. From seamless integration with existing systems to powerful data extraction capabilities, LIKE.TG equips insurance organizations with the necessary tools to thrive in today’s data-driven environment. To learn more about LIKE.TG’s solution, download our 14-day free trial today! Ready To Transform Your Claims Process? Learn how LIKE.TG ReportMiner, an advanced unstructured data extraction solution with AI-powered data extraction capabilities, can streamline claims processing. Download Free Trial
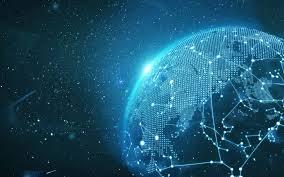
Top 7 AWS ETL Tools in 2024
Amazon Web Services (AWS) ETL refers to a cloud-based set of tools and services that help extract data from different sources, make it usable, and store it in a way that makes it easy to analyze and make decisions based on it. AWS ETL tools offer a unique advantage for businesses seeking to streamline their data processes. These tools are efficient, scalable, and adaptable, making them ideal for a wide range of industries, from healthcare and finance to retail and beyond. According to a recent report by Gartner, more than half of businesses plan to move towards industry cloud platforms by 2028. This shift reflects a new approach to utilizing cloud technologies as a strategic asset, and a utility for innovation and competitive differentiation.AWS is helping businesses prepare for the future by leveraging cloud technology. It is at the forefront of this transformation, particularly in optimizing data workflows through AWS ETL tools. In this article, we’ll discuss all about AWS ETL tools, exploring the best options available in 2024 to help you make an informed decision. What are AWS ETL Tools? AWS offers a range of ETL tools to help businesses integrate and manage their data. These tools make it easy for businesses to collect, process, and analyze data, turning it into useful insights. There are four key AWS ETL tools with unique benefits that can help businesses improve their data management. 1. AWS Data Pipeline AWS Data Pipeline is a web service that helps make it easier to move and transform data across different AWS storage, compute services, and on-premises sources. It has a simple interface that lets the user drag and drop data sources and destinations onto a visual canvas. With AWS Data Pipeline, a user can create ETL data pipelines by defining specific connection attributes. AWS Data Pipeline offers an automation feature, which lets the user schedule the data workflows to run automatically. This means that the data is always up-to-date and ready for analysis. This level of automation streamlines operations and makes data processing more efficient. The tool is fault-tolerant, meaning it’s built to handle failures and it automatically retries tasks that encounter errors. AWS Data Pipeline also provides customizable notifications to keep the user informed of their data workflows’ status. Finally, AWS Data Pipeline can manage resources automatically and provide pre-built templates for complex data handling scenarios, freeing businesses from the technical complexities of data processing. 2. AWS Glue This serverless ETL platform is designed to automate the data preparation work, making it a hassle-free process. By pointing AWS Glue to the data stored within AWS, it automatically identifies data, infers its structure, and catalogs the metadata in the AWS Glue Data Catalog. The automatic discovery and organization of data make it instantly ready for ETL processes, and accessible for querying, making sure that analytics pipelines are insight driven. AWS Glue has a user-friendly GUI that allows users to create data transformation pipelines with minimal disruption, even if they don’t have deep coding knowledge. As users visually assemble the ETL job, AWS Glue generates the necessary PySpark code behind the scenes, making it easy to use. The Glue Data Catalog and automated schema discovery streamline the ETL process by organizing data metadata centrally, making it easy to find, access, and transform. 3. AWS Lambda AWS Lambda is a serverless computing service offered by Amazon Web Services (AWS) that executes code in response to various events, automatically handling the underlying computing resources. It enables users to extend AWS services with custom logic or develop backend services without server management. Lambda supports multiple programming languages, including Python, Node.js, Java, Ruby, Go, and Power Shell, providing flexibility based on users’ preferences. As an ETL tool, AWS Lambda can process and transform data seamlessly. By leveraging Lambda functions, users can design ETL pipelines to extract data from different sources, transform it according to specific requirements, and load it into target destinations. Lambda responds to various events, such as HTTP requests via Amazon API Gateway, object modifications in Amazon S3 buckets, and table updates in Amazon DynamoDB, triggering the execution of user-defined code to perform desired actions. 4. AWS Glue DataBrew AWS Glue DataBrew is a tool that simplifies and speeds up the data preparation process for analysis and machine learning. It is like having a skilled assistant who takes care of all the data preparation tasks. With DataBrew, users can easily pull in data from different sources and clean it up, fix errors, and transform it into a more useful format using an intuitive interface. This tool saves time and makes data preparation accessible to everyone, regardless of coding skills. After refining the data, DataBrew can automate these steps. This means that when similar data is received next time, DataBrew will remember the process and repeat it automatically, without any user intervention. It is a tool for businesses that want to use their data for making decisions or building machine learning models but don’t want to get slowed down in the technicalities of getting the data ready. AWS Data Pipeline Vs AWS Glue AWS Glue is a modern, serverless service that focuses on automation and integration within the AWS ecosystem. It offers an easy and efficient way to process and transform data. On the other hand, AWS Data Pipeline is a more traditional workflow service that automates the movement and transformation of data. Feature AWS Data Pipeline AWS Glue User Interface Drag-and-drop; Web-based management console; CLI Visual and code-based GUI Data Processing Batch Batch and streaming Scaling Manually scalable to handle large datasets Automatically scales on demand Execution Environment Can be used on AWS cloud or on-premises Serverless; fully managed by AWS Integration with AWS Services Integrates with AWS storage and database services Extensive integration with AWS services, including direct support for data stores and analytics services Orchestration Supports scheduling, dependency tracking, and error handling Advanced job management, including triggers, and workflow orchestration with AWS Glue DataBrew Schema Management No native schema discovery or management Automated schema discovery, cataloging, and management with AWS Glue Data Catalog Transformation Capabilities Custom scripts for data transformation Supports both built-in transformations and custom scripts. Pricing Charged based on the frequency of activities and where they run Hourly rate for ETL jobs and crawlers, with a minimum charge; additional charges for Data Catalog and DataBrew usage Top 8 Tools to ETL Data in AWS Choosing an ETL Tool for AWS that meets your business requirements can be a difficult task, especially if we consider the range of AWS ETL tools available in the market. To simplify this process, we have compiled a list of the best AWS ETL Tools. 1. LIKE.TG LIKE.TG is a comprehensive data integration platform that simplifies and accelerates your data processes with a focus on automation and user-friendliness. It empowers both business and technical users to manage complex data workflows without extensive IT dependency. Seamlessly integrate with AWS, enhancing your data quality and ETL processes using LIKE.TG’s cloud capabilities. Ensure the integrity and cleanliness of your data before storing it in AWS services with advanced data quality management features. Efficiently transform data using pre-built transformations and functions, eliminating the need for extensive coding expertise. Create and manage ETL pipelines on AWS effortlessly with an intuitive drag-and-drop interface. Extract valuable information from unstructured data sources directly into AWS with AI-powered data extraction capabilities. Easily ingest data from various sources and formats into AWS using built-in connectors. Optimize your data processing tasks on AWS by automating and orchestrating data workflows, ensuring timely data availability. Enhance data mapping from source to destination with an AI-powered auto mapper, crucial for maintaining data quality and consistency in AWS environments. 2. AWS Glue AWS Glue is a fully managed ETL service that automates the preparation of your data for analytics, offering a seamless way to organize, cleanse, and ultimately transform your data. Pros AWS Glue’s serverless architecture eliminates the need to manage infrastructure, allowing user to focus on analyzing the data. It offers cost-effectiveness, billing based on the resources utilized. AWS Glue simplifies ETL job creation through a user-friendly interface and automatic code generation. Cons The service offers limited control over compute resources, which might not align with all project requirements or specific compute needs. AWS Glue supports only Python and Scala for scripting, potentially complicating the integration of existing ETL scripts written in other languages. 3. AWS Data Pipeline AWS Data Pipeline is a web service for data processing and transfer among AWS, on-premises, and storage sources. Pros The AWS Data Pipeline offers support for a range of AWS databases and provides an intuitive control interface It creates resources and clusters only when necessary. Data is protected both at rest and in motion through AWS’s access control mechanisms. Cons Managing multiple installations and configurations on compute resources within data pipelines can be challenging Beginners may find the representation of preconditions and branching logic complex. For users requiring data from external services, AWS Data Pipeline may not be the most suitable choice. 4. Stitch (acquired by Talend) Stitch simplifies data replication from various sources to your data warehouse, enabling efficient and secure data analytics. Pros The platform’s setup and user-friendly interface makes your data pipeline creation quick. With support for over multiple data sources, you can integrate a diverse range of data into your analysis workflows. Stitch’s adherence to SOC 2, HIPAA, and GDPR ensures your data processes meet security and privacy standards. Cons The platform’s limited customization may restrict the ability to tailor data pipelines to complex or specific business needs. Its transformation capabilities are limited, potentially necessitating additional tools for complex data manipulation. Stitch provides limited monitoring options, posing challenges for businesses that need real-time monitoring and alerts. 5. Integrate.io Integrate.io is a cloud-based platform that simplifies data integration from multiple sources. It streamlines the process of aggregating and transforming data for your analytics needs. Pros It enhances data transformation processes, facilitating quick and efficient workflows for handling data. The tool simplifies integrations, particularly with Salesforce environments, improving the data consolidation tasks. Its security features, including encryption and SOC 2 compliance, safeguards the data throughout the ETL process. Cons The platform’s basic error logging can make troubleshooting complex issues more difficult. The interface might present challenges as you navigate more sophisticated data pipeline configurations. A few aspects of platform features are not fully integrated. 6. Talend (acquired by Qlik) Talend offers a solution for your ETL needs, facilitating data extraction, transformation, and loading with its open-source platform. Pros It offers cost savings and customization possibilities with its open-source nature, making it an economical choice for the data integration tasks. The connectors allow easy linking to various data sources and applications, improving integration capabilities. It offers drag-and-drop functionality that simplifies ETL process creation and management. Cons Talend presents a steep learning curve for newcomers to ETL or data integration. While Talend boasts a large user community, its support options are more limited than traditional ETL tools. Talend can face performance challenges with very large data sets or complex transformations. 7. Informatica Informatica streamlines data integration and governance across various source systems, enhancing data warehousing and business intelligence efforts. Pros Its graphical interface facilitates the ETL process design, session monitoring, and overall task management with ease. The platform can manage multiple data types, including queued messages and unstructured data, ensuring complete data integration. With Informatica’s centralized web-based platform, organizations can achieve data governance and reductions in administrative costs. Cons The Workflow Monitor lacks a sorting feature, making it difficult to distinguish between essential and non-essential folders. The Repository Manager does not support moving items between folders or importing XML export files. Developing mappings and workflows is not possible with the current toolset. How to Select the Right AWS ETL Tool When it comes to AWS ETL tools, there are many options out there, all claiming to be the best for the data processing needs. It can be overwhelming to choose the right one. To help make an informed decision, let’s explore the most important factors to consider: Data Sources It Supports The tool should offer broad support for various data sources. This includes traditional databases like MySQL or PostgreSQL, cloud services, and even streaming data platforms. For example, a company using a mix of on-premises databases and cloud-based services will benefit from an ETL tool that can seamlessly integrate data from all these sources. Data Replication Features The ability to replicate data efficiently is essential. The tool should provide real-time data replication capabilities, ensuring that data is continuously synchronized between source and destination. This is important for businesses that rely on up-to-date data for real-time analytics and decision-making. For instance, an e-commerce platform may need to constantly replicate data to analyze customer behavior and inventory levels in real-time. Zero Coding Additionally, for businesses aiming to minimize coding effort or eliminate it entirely, no-code AWS ETL tools are a valuable option. These tools provide a user-friendly interface where users can create, manage, and monitor ETL jobs without writing a single line of code. Drag-and-drop interfaces and pre-built connectors for common data sources and destinations make these tools accessible to users regardless of their technical expertise. An example would be a marketing team without programming skills needing to integrate customer data from various platforms for analysis; a no-code ETL tool would enable them to set up and manage these integrations effortlessly. Customer Support Service Good customer support is vital. The ETL tool should come with comprehensive support options, including 24/7 live support, extensive documentation, and community forums where users can exchange tips and solutions. This is particularly important for businesses that may encounter complex integration scenarios or need guidance on optimizing their ETL processes. A company might prefer a tool with a reputation for responsive and helpful support to quickly resolve any issues that arise. Pricing The cost of the ETL tool is a significant consideration. Pricing structures vary widely, from subscription-based models to pay-as-you-go plans based on the volume of data processed. Businesses should look for transparent pricing that aligns with their data usage patterns and budget constraints. For small startups or companies with fluctuating data processing needs, a tool offering scalable pricing or a free tier for lower volumes of data could be most beneficial. Concluding Thoughts Picking the right AWS ETL tool is key for companies looking to use their data efficiently. These tools not only streamline data processes but also empower organizations to make informed decisions based on accurate insights. As you explore the best options for your data management needs, consider how features like automation, scalability, and data quality align with your goals. LIKE.TG stands out as the leading AWS ETL tool, offering unparalleled ease of use, automation capabilities, and comprehensive data quality features. Experience LIKE.TG’s transformative power for yourself with a free 14-day trial or schedule a demo to see how it can streamline your data processes and drive your business forward. Accelerate Your Data Processes Simplify the complex process of Data Processing. Try LIKE.TG for free for 14 days and optimize your ETL. Experience how LIKE.TG streamlines data processes, and optimize your workflows. Request a Demo
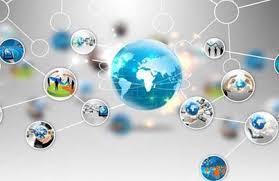
Automate Tax Form Data Extraction in 5 Easy Steps
A Smartsheet report found that over 40% of workers spend at least a quarter of their workweek manually extracting data. Tax specialists in many organizations spend hours or even days sorting through piles of paper or PDF documents, looking for relevant information, and entering it into spreadsheets or databases. That’s a lot of time and money wasted on a tedious and error-prone process. Fortunately, there is a better way to handle tax form data extraction. With LIKE.TG ReportMiner, you can automate the entire process and save yourself time, money, and hassle. Why Is Tax Form Data Extraction Important? Tax form data extraction, especially from documents like W-2, 1099, and 1098, is crucial for individuals, businesses, tax professionals, lenders, financial institutions, researchers, and analysts for various reasons: Accurate Tax Filing:Automating data extraction ensures accurate and timely tax return filing for individuals and businesses, reducing the risk of penalties, interest, and audits from the IRS. Enhanced Services:Tax professionals and accountants can provide better services to clients with accurately extracted data, like tax planning, compliance, optimization, and resolution. Risk Reduction:Lenders and financial institutions can accurately verify borrower income and creditworthiness, reducing the risk of default and fraud. Insightful Analysis:Researchers and analysts can study economic and social trends based on tax data like income distribution, tax burden, incentives, and evasion. Automating tax form data extraction is essential as it: Saves Time Resources:Reducing manual effort and errors in data entry while increasing processing speed. Improves Accuracy Consistency:Standardizing data extraction models minimizes variations in data quality. Enhances Compliance Security:Ensuring extracted data meets tax regulations and is securely stored. How LIKE.TG Transforms Tax Form Data Extraction LIKE.TG ReportMiner is a powerful unstructured data extraction solution that automates and streamlines tax form data extraction. LIKE.TG ReportMiner captures and converts unstructured data into a structured format. It leverages advanced OCR and AI capabilities to extract data from any document, including scanned PDFs, digital PDFs, and other unstructured sources. See LIKE.TG's Tax Form Data Extraction in Action Simplify and streamline your tax form data extraction process with LIKE.TG's powerful automation tools. Schedule a demo to see how LIKE.TG can transform your processes. Schedule a Demo With LIKE.TG ReportMiner, you can: Create and reuse extraction templates: You can easily create and reuse extraction templates for different tax forms using the intuitive drag-and-drop interface. You can create extraction templates for common tax formats, such as W-2, 1099, 1040, and more. They can build these templates using ReportMiner, customize them based on their specific needs and reuse on several docs with the same format. You can also define the rules and logic for data extraction, such as data types, formats, validations, and transformations. Extract data from multiple sources: You can extract data from multiple sources, such as scanned PDFs, digital PDFs, images, and other unstructured documents. With high accuracy and speed, you can use the built-in OCR engine to recognize and extract data from scanned or image-based documents. You can create extraction templates with a few clicks, so they no longer have to provide patterns and create data regions and fields manually. This reduces the manual work in creating an extraction template, especially helpful when documents have complex or extensive layouts. Integrate and export data to multiple destinations: You can integrate and export the extracted data to various destinations, such as databases, files, BI tools, or other applications. You can use the built-in connectors to connect to popular data sources and destinations, such as SQL Server, Oracle, Excel, CSV, XML, JSON, and Power BI and Tableau APIs. You can also use the REST API connector to integrate with other custom or third-party applications. Solution Demonstration: How LIKE.TG Automates End-to-End Tax Form Data Extraction Step 1: Creating the Project The first step is to create an LIKE.TG project. For this use case, you don’t need to add source file to the project either. With LIKE.TG, you can process any unstructured file, such as scanned PDFs, digital PDFs, forms, .TXT, .CSV and other unstructured documents. For this example, you will use a digital PDF file that contains W-2 forms. Figure 1 Step 2: Email Handling Automation To access W2 tax form data, you must monitor incoming emails for W2 tax form attachments, move them to a designated folder, and mark them as read. You can automate this by building and scheduling a data pipeline. This will ensure timely processing of W2 forms upon receipt, streamline data extraction workflow, facilitate centralized attachment management, and enhance email management. To do this, you will need to: Configure an email source in the dataflow artifact of LIKE.TG ReportMiner, where you configure the email connection. Dataflow to download attachments in a local folder from Unread emails with “Forms” in the Subject (this dataflow is scheduled to run continuously): Figure 2 Set the filter criteria to select only the emails with ‘Forms’ in the subject line. Figure 3 In the Email Source object, enable the option to download attachments and specify the folder path where you want to store the downloaded attachments. Email attachments automatically appear in the chosen folder: Figure 4 The Excel destination in the dataflow shown in Figure 2 stores paths for all downloaded attachments: Figure 5 Step 3: Building Report Model for the Tax Form The next step is to build a report model for the W2 tax form so that you can define the data fields, data types, extraction rules. This will enable precise extraction of W2 from data fields, enhancing the quality and reliability of extracted information. To do this, you will need to: Add a new report model to the project. You can add a sample W2 tax form file as the report layout and adjust the page settings and margins as needed. Typically, users must specify the regions they want to extract data from and the data fields within the regions. However, LIKE.TG’s advanced AI-powered extraction engine allows you to create W2 extraction template with a single click: Figure 6 When you click Build using AI on the previous screen, the following appears: Figure 7 After the above processing ends, we have the AI built report model ready: Figure 8 Step 4: Data Pipeline for Processing a Single Tax Form The next step is to create a data pipeline for processing a single W2 tax form to extract data from W2 tax form PDFs, standardize numeric fields, validate tax information, generate validity fields, and transfer processed data to a destination database. This will ensure consistent and accurate extraction of critical information from W2 forms, enhance data consistency and readability, ensure data accuracy and compliance, facilitate W2 tax form status monitoring and error identification, and enable centralized storage of extracted W2 data. To do this, you will need to: Create a new dataflow in LIKE.TG and add the report model you created in the previous step as the source object. Add a transformation object, such as DataCleanse to clean the data, such as removing characters like $, unwanted whitespaces, replace null numerics with zeros etc. Figure 9 Add a validation object and apply business rules to validate tax information, such as checking for missing values, matching with reference data, validating tax rates, etc. Figure 10 Add a new field to the tax record indicating the validity status of each W2 tax form, such as valid, invalid, or pending, based on the validation results. Figure 11 Add a destination object, such as a database table or a flat file, and use it to transfer the processed data to a destination database for secure storage and retrieval. The following is a preview of the data loaded into the db when a single W2 tax form is processed: Figure 12 Step 5: Workflow Automation The final step is to automate the entire data extraction workflow so that you can trigger processing of W2 tax form files each whenever a new file appears in the email attachment folder you have prepared. This will automate attachment retrieval, ensure a continuous data flow for processing, facilitate easy access to processed attachments, streamline processing by automating file handling, ensure efficient file management, and enable real-time database updates. To do this, you will need to: Create a new workflow in LIKE.TG and add ContextInfo. ContextInfo takes context information of the scheduled job runtime. Here it will get the filepath that will trigger this flow. Add a dataflow task and use it to execute the dataflow you created in the third step to process a single W2 tax form. Add a file system task and use it to delete the processed W2 tax form file from the attachments folder after the dataflow execution. Figure 13 The workflow triggers the processing of W2 tax forms as your team receives them in the email attachments folder. Upon completion, the workflow will automatically store records from each email attachment in the destination database: Figure 14 Each time your workflow receives a new W2 form attachment via email, it goes into processing (seen by the change in name). Figure 15 After a W2 tax form is processed, it will be deleted from the folder, making sure only fresh files appearing in the folder are processed: Figure 16 Schedule the workflow to run on file drop trigger in the attachment folder. Automation’s Impact on Tax Form Data Extraction Reduce tax form data extraction time: Automated tax form data extraction allows you to reduce the data extraction time from hours to minutes by automating and streamlining the process. You can process over 100,000 tax forms in a fraction of the time and meet the deadlines and regulations for tax filing and reporting. With LIKE.TG, your team can reduce time spent on data extraction by up to 80%. Improve data quality and accuracy: Automated tax data form extraction allows you to improve the data quality and accuracy by 90%, using built-in data cleansing and validation features. You can ensure the completeness and correctness of the extracted data and avoid any errors or penalties. Save costs and resources: Automated tax data form extraction allows you to save costs by eliminating the need for manual data entry or complex and expensive software. As a result, your team can reduce labor and operational costs and increase the productivity and efficiency of the staff. Unlock the Power of Automated Tax Form Data Extraction Discover how automation can revolutionize your tax form data extraction process. With LIKE.TG, gain unparalleled efficiency and accuracy. Sign up now to explore a free 14-day trial and experience the difference. Sign Up for a Free Trial With automated tax form extraction, you can extract data from tax forms quickly, easily, and accurately, leveraging data for reporting, analysis, or compliance. Your company can acheive improved customer satisfaction and retention, giving a competitive edge in the market. LIKE.TG ReportMiner is a powerful data extraction solution that handles any tax form and unstructured data source. Whether you need to extract data from W-2, 1099, 1040, or any other tax form, LIKE.TG ReportMiner can do it in minutes. No coding or scripting is required. Just point and click. Imagine the hours you could save and the errors you could avoid with this automation. If you are interested in trying LIKE.TG ReportMiner for yourself, you can start a free 14-day trial today or schedule a custom demo with our experts and get a personalized walkthrough of LIKE.TG ReportMiner’s features and capabilities. Don’t miss this opportunity to take your data extraction to the next level with LIKE.TG ReportMiner.
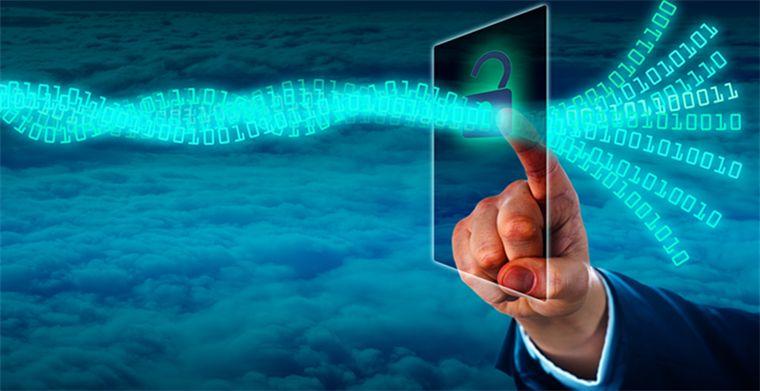
How to Automate Data Extraction from Patient Registration Forms in Healthcare
Automating data extraction from patient registration forms in healthcare is crucial to enhancing patient care efficiency, accuracy, and overall quality. Over 71% of surveyed clinicians in the USA agreed that the volume of patient data available to them is overwhelming. This abundance of data highlights the importance of streamlining the extraction process. Manual extraction is time-consuming and prone to errors, hindering patient safety. This blog discusses the importance of data extraction in healthcare, the benefits of using AI-powered tools, and how LIKE.TG can help automate patient information extraction from registration forms to make it faster and more efficient. The Role of Data in Patient Care Patient data includes a wide range of information, such as names, contact details, demographics, medical history, medications, allergies, and test results. Access to this data enables healthcare providers to get a holistic view of the patient’s health, make informed decisions, and deliver personalized care. By extracting data from medical records, healthcare providers can identify patterns and trends in patient outcomes. This information can then be used to develop evidence-based treatment protocols and guidelines. Furthermore, data extraction allows for the integration of patient data from various sources, such as electronic health records, laboratory systems, and wearable devices. This integration enables healthcare professionals to comprehensively understand the patient’s health status, facilitating more accurate diagnoses and tailored treatment plans. The Importance of Automating Data Extraction in Healthcare Manual data extraction is a labor-intensive process that often leads to inconsistencies and inaccuracies. Human errors, such as transcription mistakes and data misinterpretations, pose significant risks to patient safety. Also, the high volume of patient registration forms amplifies the inefficiency of manual data extraction processes. Additionally, manual data extraction limits the scalability of healthcare systems. As the demand for healthcare services grows, efficient, scalable data management solutions become increasingly important. With automation, healthcare providers can streamline data extraction processes, allowing faster and more reliable access to patient information. Using an Automated AI-Powered Data Extraction Tool In the healthcare industry, automation has optimized various aspects of healthcare operations. It involves leveraging cutting-edge technologies like artificial intelligence (AI) to streamline the data extraction process. Sophisticated algorithms and software solutions can automatically scan and extract data from documents and forms, eliminating the need for manual intervention. These technologies can recognize and interpret various forms of data, such as patient demographics, medical history, and test results. Using an automated AI-powered data extraction tool in healthcare has numerous benefits. Some of these benefits include: Faster Data Extraction: The adoption of automation in healthcare expedites the retrieval of critical patient information, proving particularly invaluable in time-sensitive situations. This acceleration in data access plays a pivotal role in streamlining care delivery, making it more efficient and effective. Higher Accuracy: Implementing automated data extraction processes in healthcare significantly enhances precision by eliminating the potential for manual errors. This not only improves patient safety but also contributes to more favorable treatment outcomes. Cost Savings: The integration of automated processes in healthcare not only boosts efficiency but also leads to substantial cost savings. By minimizing reliance on manual labor, healthcare organizations can allocate resources more judiciously, resulting in improved financial outcomes. Improved Data Management and Analysis: Automated data extraction serves as a catalyst for centralizing patient information and fostering better organization. This centralized data not only enhances the overall management of healthcare data but also facilitates insightful analysis, aiding informed decision-making on population health trends, treatment effectiveness, and resource allocation. Enhanced Decision-Making: Automation empowers healthcare providers with data-driven insights, ushering in a transformative era in decision-making. This innovative approach revolutionizes choices in patient care, resource allocation, and overall strategy, ultimately leading to improved outcomes and enhanced patient experiences in the healthcare industry. LIKE.TG: The Perfect Tool for Automating Data Capture from Patient Registration Forms LIKE.TG is an advanced AI-powered solution designed to streamline and automate the process of data extraction from patient registration forms. With its advanced features and user-friendly interface, LIKE.TG is the perfect tool for healthcare organizations seeking efficiency and accuracy in managing patient information. Key features of LIKE.TG: Intelligent Data Extraction: LIKE.TG’s AI-driven engine efficiently retrieves information from diverse document layouts by identifying the required fields. It adeptly handles variations across different layouts, ensuring swift and precise extraction. Batch Processing: LIKE.TG’s batch processing feature simultaneously supports data extraction from multiple documents and forms. This feature streamlines the overall extraction process for enhanced efficiency and productivity. Real-Time Processing: LIKE.TG’s Scheduler incorporates the File Drop feature, enabling the solution to process and extract information from a file as soon as it appears in a folder, facilitating real-time processing. Advanced Data Preparation: LIKE.TG has built-in data cleansing and validation capabilities, ensuring the extracted information undergoes thorough scrutiny for accuracy and integrity. Error Handling and Logging: LIKE.TG offers logging capabilities to capture and track any errors or issues encountered. Integration with External Systems: LIKE.TG ReportMiner seamlessly integrates with external systems or databases, enabling the direct loading of extracted data into preferred destinations. Steps to Automate Data Extraction from Patient Registration Forms Let’s take a use case in which patient data is contained within a PDF form, as shown below. This patient registration form is fillable and has radio buttons, checkboxes, drop-down menu, and text boxes. LIKE.TG’s power can be leveraged to perform the data capture process from multiple such forms. One such form is shown below. Step 1: Configure the PDF Form Source Within LIKE.TG’s main designer, drag and drop a PDF Form Source object on the designer and provide the file path for one of the forms we want to process. With this, we have defined our PDF form as the source. Step 2: Perform Data Transformations and Apply Data Mapping in the Dataflow The form collects the first name and last name in different fields. Use an Expression transformation to merge the two fields to get the full name. Use the same transformation to extract a full address field from the multiple address fields in the form. The PDF form extracted day, month, and year separately. To get a full date, use the built-in function ToDate available in the toolbox. Once all the data transformations are applied, map the extracted data to an Excel destination (check the append to file option to have data from all the forms written to the same file as we run this data pipeline for each form we want to process). Step 3: Preview Data Instantly Preview the output to see if data has been extracted correctly. Step 4: Configure File System Item Source Object to Extract Data from All Files in a Folder Every healthcare provider must extract data from multiple patient registration pdf forms. To automate this process of extracting data from all the pdf forms in a folder, use the file system item source object and provide the folder path. Map this file system object to a workflow object that defines the processing sequence for each PDF form. The inner workflow defines the execution of the data pipeline we created to extract data followed by the file action to move the processed file to a dedicated folder once the data extraction process is complete. Step 5: Schedule to Run This Process Automatically Use the Job Scheduler to execute the entire process weekly. This way, all new patient registration forms collected over a week will be processed automatically without manual intervention. The screenshot below shows the preview of the Excel destination file, which has all the extracted data after the execution of the workflow. Conclusion The adoption of LIKE.TG ReportMiner, with its intelligent data extraction capabilities and user-friendly interface, empowers healthcare providers to overcome the challenges associated with manual methods. By following the outlined steps, healthcare providers can seamlessly integrate LIKE.TG ReportMiner into their workflows, ensuring the automated extraction of vital patient information and paving the way for a more efficient and data-driven healthcare system. Experience the power of LIKE.TG with a 14-day free trial or a personalized demo with our experts—unlock the potential of AI-driven form processing today!

How to Load Data from AWS S3 to Snowflake
According to a study by Statista, the cloud storage market was valued at $90.17 billion in 2022 and will reach a value of $472.47 billion by 2030. These figures indicate a growing shift toward cloud computing and data storage solutions. A typical scenario in modern data management involves data transfer from cloud storage to cloud-based computing platforms. Amazon’s Simple Storage Service (S3) is among the go-to options for the former, and businesses trust Snowflake for the latter. As a result, S3 to Snowflake data transfers are common for businesses looking to benefit from the synergy of S3’s scalable storage and Snowflake’s top-notch data warehousing features. This blog discusses the S3 to Snowflake data loading process and explains two ways of setting it up so you can pick the right one. Let’s get started. Amazon S3 Overview Amazon S3 is an object storage service by Amazon Web Services (AWS). It’s backed by the scalable storage infrastructure supporting Amazon’s massive global e-commerce network. As a high-speed, web-based cloud storage service, Amazon S3 creates online backups of data and applications on AWS. It’s well-suited to meet data storage requirements of any scope from different industries. Integration with Other AWS Services: You can link S3 to other AWS security and monitoring services such as Macie, CloudTrail, and CloudWatch. Directly linking vendor services to S3 is also an option. Easy and Secure Data Transfer: You can transfer data to S3 over the public internet through S3 APIs. Amazon S3 Transfer Acceleration can help you transfer data more quickly, and AWS Direct Connect creates a private connection between S3 and your data center. Use Cases: Amazon S3 is a good storage option for organizations of varying sizes thanks to its scalability, security, availability, and performance capabilities. Typical S3 use cases include the following: Data storage Data archiving Document storage Data backup Log file storage Software delivery Website hosting Data lakes and data warehouses Snowflake Overview Snowflake is a fully managed Software-as-a-Service (SaaS) started in 2012 and launched in 2014. It offers a single platform for tackling users’ data warehousing, data lakes, data science, data application and development, and data engineering requirements. It also supports the secure sharing and consumption of real-time or shared data. This cloud-based data warehousing solution simplifies the storage, processing, and analysis of structured and semi-structured data. Snowflake’s separation of computing and storage distinguishes it from conventional data warehouses. This allows you to allocate resources to different functions independently based on your requirements. Here are some key features of Snowflake: No Hardware and Limited Software: As a true SaaS offering, Snowflake isn’t burdened by virtual or physical hardware you would have to install or configure. There’s also virtually no software that you’ll need to set up. Hassle-Free Maintenance: Snowflake undertakes all maintenance, updates, tuning, and management tasks — easing the administrative burden on users. Cloud Provider Agnosticism: Snowflake is known for being cloud provider agnostic. It’s available on AWS, Azure, and GCP and retains its functionality and end-user experience on each of them. How to Load Data from S3 to Snowflake? There are two ways you can load data from S3 to Snowflake: manual or automated. Below, we’ll discuss manual data transfer using the COPY INTO command and automated data transfer using a third-party integration tool such as LIKE.TG. Manual Data Transfer from S3 to Snowflake The following steps are involved in manually setting up a data transfer between S3 and Snowflake: 1. Setting Up the Amazon S3 Bucket If you don’t already have an Amazon S3 bucket, create one where your data is stored. Verify that Snowflake has the necessary permissions to access this bucket. You may need to set up an AWS Identity and Access Management (IAM) role, grant it the required permissions, and attach it to the Snowflake account. 2. Configuring Snowflake External Stage Log in to your Snowflake account. Create an external stage using the Snowflake web interface or SQL commands. Mention the Amazon S3 bucket path as the location for the external stage. 3. Create Snowflake Table Define a Snowflake table schema that matches the data structure of your Amazon S3 files. Make a table in Snowflake for the data transferred from S3. 4. Set Up Snowflake Integration Create a Snowflake integration object to establish a connection between Snowflake and Amazon S3. Specify necessary details, such as the AWS access key, AWS secret key, and the external stage associated with the S3 bucket. 5. Grant The Required Permissions Ensure that the Snowflake user or role has the necessary permissions to access the external stage and load data into the Snowflake table. 6. Load Data into Snowflake Use the COPY INTO command in Snowflake to load data from the external stage (Amazon S3) into the Snowflake table. Specify the source file format, the destination table, and other relevant options. 7. Monitor and Troubleshoot Monitor the data transfer process to ensure smooth, error-free operations. Check Snowflake and Amazon S3 logs for error messages and troubleshoot in case of any errors. If you wish to automate the data transfer from S3 to Snowflake, you can set up a schedule using Snowflake’s task scheduler or a third-party scheduler tool. Define a schedule for executing the COPY INTO command to load new data from Amazon S3 into Snowflake at specified intervals. AWS S3 to Snowflake Data Transfers. Simplified. Enjoy complete control over your AWS S3 to Snowflake pipelines without writing a single line of code. Try LIKE.TG for two weeks and experience the difference for yourself. Start My FREE Trial The Limitations of Manual Data Transfer from AWS S3 to Snowflake Manually loading data from AWS S3 to Snowflake may appear simple but it still has certain limitations. Below are some of the major ones you should know about: Data Transfer Costs: Transferring vast data volumes from S3 to Snowflake can quickly increase data transfer costs. These costs can add up over time and become prohibitively expensive. Network Latency: Network latency can impact data transfer speeds in an S3 – Snowflake transfer. This depends mainly on the geographical distance between your Snowflake instance and the AWS region with your S3 bucket, and your network connectivity. Data Security: Both AWS and Snowflake offer mechanisms to ensure secure data transfer, such as IAM roles and HTTPS encryption. However, misconfigured protocols or gaps in security methods can potentially expose confidential information during transit. Data Format Compatibility: Snowflake supports formats such as JSON, CSV, Avro, and Parquet. However, you can face problems if your S3 data is in a format that Snowflake doesn’t support directly — this will necessitate preprocessing or transforming the data before loading. Scalability and Concurrency: Snowflake is equipped with powerful concurrency and scalability features. However, it’s wiser to optimize your data-loading processes and resource allocation to mitigate performance bottlenecks, slower data loading, and disrupted services. Error Handling and Monitoring: Data-loading processes are prone to errors due to network issues, data format incompatibility, or lack of required permissions. You’ll have to implement comprehensive error-handling mechanisms to identify and mitigate such problems promptly. Regulatory Compliance: Based on the nature of your data and applicable regulatory requirements, ensuring compliance with data governance standards such as the General Data Protection Regulation (GDPR), Health Insurance Portability and Accountability Act (HIPAA), and Payment Card Industry Data Security Standard (PCI DSS) can require substantial effort on your part. Automated Data Transfer from AWS S3 to Snowflake Using LIKE.TG 1. Browsing Cloud Files First, note that you can browse cloud files in an Excel source or any other file source in LIKE.TG. 2. Setting Up Cloud Connections Selecting the ‘Browse Cloud Files’ option will take you to a browser where you can set up cloud connections. Here, you can set up Amazon S3 by selecting ‘Add New Connection’ and inputting credentials, as seen below. 3. Choosing The Right File Next, you’ll choose the file you want to access in AWS S3. The selected file will appear in the source file path as follows: 4. Check the File Layout When you check the layout of the file in LIKE.TG, this is how it’ll show up: Once configured, the source object will look like this: 5. Setting up Snowflake Next, you’ll set up Snowflake by configuring a Database Destination object with Snowflake credentials: Along with the Snowflake connection, you can optionally configure a Staging Area. In the image below, the Amazon S3 bucket serves as the staging area. You have now configured Snowflake as the Destination Objectand the Dataflow will appear as shown below: 6. Mapping, Previewing Output, and Running The Dataflow Next, you’ll create mappings from the Source Object (S3) to the Destination Object (Snowflake): ‘Preview Output’ for the Destination Object will look like this: Lastly, when you run the Dataflow using the ‘Start Dataflow’ button, LIKE.TG will write the data to the destination, completing the S3 to Snowflake data transfer. Manual S3 to Snowflake Data Transfers vs Automated Transfers in LIKE.TG As seen above, setting up manual S3 to Snowflake data transfers is a valid option. However, the process can be complicated, especially for non-technical users. To recap, these are the steps involved: S3 bucket setup IAM role creation External stage creation in Snowflake Setting up table schema and making a table in Snowflake Creation of a Snowflake integration object Loading data from S3 into the Snowflake table using the COPY INTO command Monitoring and troubleshooting the data transfer process as needed In contrast, LIKE.TG simplifies S3 to Snowflake data transfers with its intuitive interface, drag-and-drop functionality, and ease of use. It lets you conveniently create and automate data transfers in a few clicks, regardless of your technical proficiency. Conclusion LIKE.TG streamlines end-to-end data management for a range of industries. Its powerful, enterprise-ready solution allows you to design, deploy, and automate S3 to Snowflake pipelines — with zero coding required. Find out how you can use LIKE.TG to transform your data transfer processes. Reach out to our team or start your FREE trial today!