广告优化
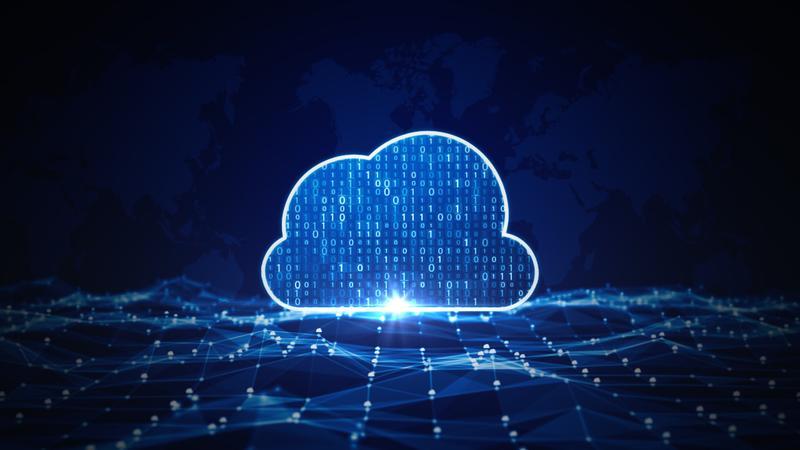
API Development: Best Practices and Strategies
API development facilitates efficient data sharing, enhances interoperability between systems, and drives digital ecosystem growth. APIs are at the core of modern applications and systems. In fact, 56% of enterprise leaders agree that APIs help them build better digital experiences and products. So, how do you develop APIs correctly, ensuring they seamlessly connect to the digital world and drive the growth of digital ecosystems? The answer lies in a series of best practices and strategies that pave the way for effective and user-friendly API development. Understanding API Development An API, or Application Programming Interface, serves as a bridge between different software systems, enabling them to communicate and share data seamlessly. APIs come in many shapes and sizes, from RESTful web services to SOAP endpoints, but the principles for creating effective APIs remain fairly consistent. Best Practices and Strategies for API Development 1. Define Clear API Objectives and Goals Every successful API starts with a clear purpose. Define the objectives and goals of your API project before writing a single line of code. What problem does your API solve, and who are the intended users? Having a well-defined scope helps you avoid feature creep, i.e., excessive features that make an API too complicated or difficult to consume, and ensures your API serves its intended purpose effectively. 2. Use Meaningful and Consistent Naming Conventions Choosing meaningful and consistent naming conventions for your API endpoints, methods, and data structures is essential. It makes your API more intuitive and user-friendly, reducing the learning curve for developers interacting with it. Be concise and maintain a standardized approach to naming. 3. Version Your APIs to Maintain Backward Compatibility As your API evolves, it’s critical to maintain backward compatibility with existing clients. Versioning your API allows you to make changes and improvements without breaking the functionality for users of older versions. Follow a clear versioning strategy (e.g., using URLs or headers) to manage these changes effectively. 4. Implement Proper Authentication and Authorization Mechanisms Security should be a top priority in API development. Implement robust authentication and authorization mechanisms to ensure that only authorized users can access your API’s endpoints. Options include OAuth, API keys, and JWT tokens, depending on your application’s requirements. 5. Document Your APIs Comprehensively Comprehensive and well-maintained documentation is the lifeblood of any API. It serves as a reference guide for developers, making it easier for them to understand how to use your API effectively. Include clear examples, use cases, and details about endpoints, requests, and response formats. 6. Provide Descriptive Error Messages and Status Codes Users should receive informative error messages and status codes when something goes wrong in an API request. Well-documented error responses help developers quickly identify and resolve issues, improving the overall user experience. 7. Validate Input Data and Handle Validation Errors Input data validation is essential for the security and reliability of your API. Validate user input to prevent common security vulnerabilities like SQL injection and cross-site scripting. Ensure that you handle validation errors and provide meaningful feedback to users. 8. Optimize API Endpoints for Performance and Scalability API performance can significantly impact the user experience. Optimize your API endpoints for speed and scalability. Use techniques like caching, pagination, and asynchronous processing to enhance performance while ensuring your API can handle increasing loads of user requests. 9. Implement Rate Limiting and Throttling to Prevent Abuse To prevent abuse or overuse of your API, implement rate limiting and throttling. Set limits on the number of API requests a user or client can make within a specific time frame. This helps maintain fair usage and protects your API from abuse. 10. Use Appropriate HTTP Methods (GET, POST, PUT, DELETE) for CRUD Operations Follow the HTTP methods (GET, POST, PUT, DELETE) convention for CRUD (Create, Read, Update, Delete) operations. This aligns your API with standard practices, making it more intuitive for other developers to work with. Understanding the Limitations Technical Complexity: API development demands expertise in various programming languages and protocols. Resource Intensive: Building and maintaining APIs can require significant resources, including development time, server capacity, and operational costs. Learning Curve: For those new to API development, there is a learning curve. It may take time to grasp the intricacies of APIs fully. Security Concerns: Ensuring robust security measures is essential. APIs can be vulnerable to attacks if not adequately protected. Versioning Challenges: Managing different versions of an API can become complex, necessitating careful planning and execution. Coding Expertise: Traditional API development often requires coding skills, which may not be the preferred approach for all projects. Consider no-code or low-code solutions to bypass this limitation. Following the best practices and strategies outlined above and acknowledging the limitations, you can create robust and user-friendly APIs that contribute to the growth and connectivity of modern enterprises and provide a seamless experience for users and developers alike. Simplify API Development with LIKE.TG Now, let’s explore how LIKE.TG’s no-code API development solution can assist you in implementing these guidelines effectively. Streamlined Development LIKE.TG API Management simplifies the API development process with its no-code environment. You can define clear API objectives and goals effortlessly. Developers can focus on business logic while the platform takes care of the technical intricacies. This ensures that your APIs are aligned with your organization’s objectives and reduces development time. Automated Documentation LIKE.TG API Management includes built-in features for creating comprehensive API documentation. You can generate clear and up-to-date documentation with ease, detailing endpoints, request-response formats, and authentication methods. This ensures that your APIs are well-documented, reducing confusion and friction for developers. Security and Versioning The platform offers robust security features, including authentication and authorization mechanisms. It also simplifies versioning, making it easy to maintain backward compatibility as your APIs evolve. LIKE.TG API Management allows you to implement these critical best practices without diving into complex coding, reducing the risk of security vulnerabilities and ensuring smooth transitions between API versions. Performance Optimization LIKE.TG API Management simplifies the process of optimizing API endpoints for performance and scalability. Its built-in features allow you to manage caching, fine-tune database queries, and integrate content delivery networks (CDNs) with ease. This ensures that your APIs are designed for efficiency and high performance, meeting the demands of large-scale enterprise applications without the need for complex coding. LIKE.TG simplifies the development process, automates documentation, and ensures robust security. With features that streamline performance optimization, rate limiting, and versioning, the no-code API development tool empowers you to create APIs that align seamlessly with your enterprise objectives. Ready to see LIKE.TG API Management in action? We’d love to show you how it works! Reach out to us at [emailprotected] to take the first step towards efficiency, security, and innovation today. Reduce API Development Time by Up To 80% With LIKE.TG Simplify API development through an intuitive, drag-and-drop interface, enabling seamless API consumption, designing, and deployment for both technical and non-technical users. View Demo
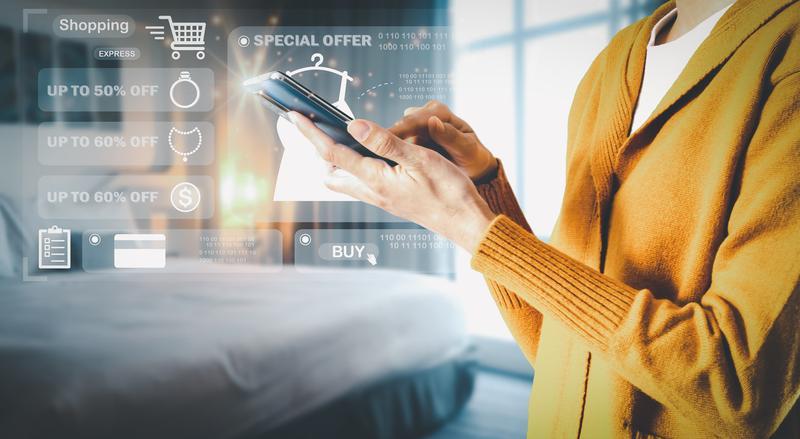
Introducing Cloud File Drop Automation with LIKE.TG
Cloud adoption has risen significantly in the past few years due to digital transformation initiatives. Businesses are migrating their operations to the cloud due to its enhanced scalability, flexibility, and agility. Gartner predicts that by 2025, over 85% of organizations will adopt a cloud-first principle.
The Shift to Cloud Computing
The global business landscape is witnessing a significant shift towards cloud computing, driven by its myriad benefits. Let’s examine some key factors that make cloud computing an ideal solution for your business.
Scalability: Cloud computing offers you the ability to scale resources up or down based on demand, which reduces the need for upfront investments, and accommodates fluctuating workloads.
Accessibility: Cloud services can be accessed from anywhere with an internet connection, promoting remote work, global collaboration, and expansion.
Disaster Recovery: Cloud providers offer robust data backup and disaster recovery solutions, reducing the risk of data loss and ensuring business continuity.
Understanding Cloud File Drop Automation
File Drop Automation is a process that automates workflows based on the addition of new files to a specific folder. This means that when a file is dropped into a designated folder, a predefined workflow is triggered automatically, eliminating the need for manual intervention. As the adoption of popular cloud services such as Amazon S3 and Microsoft Azure Blob Storage is on the rise, this functionality plays an even more important role. It involves the automation of processes when files are added to these cloud-based folders. This includes data processing, data integration, and even data analysis tasks, ensuring that the data is readily available for use across various platforms and applications.
For instance, your business might set up a workflow that automatically processes sales data whenever a new sales report is added to a specific Amazon S3 bucket. Or you might use Microsoft Azure Blob Storage to store customer feedback forms, with a workflow set up to automatically analyze and categorize this feedback whenever a new form is added. By automating these processes, your business can ensure that its data is processed quickly and accurately, allowing you to make timely, data-driven decisions.
Cloud File Drop Automation can benefit your organization through:
Enhanced Efficiency: File Drop Automation significantly reduces the time and effort required to initiate and execute workflows. By automatically triggering processes when a new file is added, it frees up resources for other critical tasks.
Improved Accuracy: With automation, the risk of errors associated with manual processes is significantly reduced, ensuring that the right processes are triggered by the right files at the right time.
Cost-Effective: By reducing the need for manual intervention and minimizing errors, File Drop Automation can lead to significant cost savings in the long run.
Real-Time Processing: File Drop Automation allows for real-time processing of data as soon as it is dropped into the cloud-based folder, enabling businesses to make timely decisions based on the most recent data.
Enhanced Collaboration: With files automatically processed and available in the cloud, collaboration among team members located in different geographical locations becomes easier and more efficient.
Cloud File Drop Automation with LIKE.TG
LIKE.TG makes it possible for you to ingest data from the cloud without spending hours in manual coding and other processes. Simply leverage the file drop automation feature to automate the processing of files in cloud-based sources such as FTP, Amazon S3, and Microsoft Azure Blob Storage. This streamlined automation enhances efficiency and reduces the risk of errors, which makes data management more accurate and reliable.
How LIKE.TG File Drop Automation Works
LIKE.TG allows you to set up a system that automatically detects when a file is dropped into a specified cloud directory, processes the data, and transfers it to a designated destination. This not only simplifies data management but also ensures that your data is always up-to-date and ready for analysis.
To utilize the cloud browser support in File Drop, you need to initially deploy the shared connection via the catalog. Once this is done, you can schedule the process to automate the workflow. This enhancement streamlines the process and makes it more efficient, further empowering businesses to leverage the power of the cloud.
Here is a step-by-step guide on how to effectively use this feature:
As a first step in this process, create a Shared Action containing a Cloud Connection to deploy it via the catalog.
Next, click on enable for catalog option and proceed to deploy the Shared Action.
Once the connection is successfully deployed and added to the catalog, check if the shared action is visible in the Connections section of the Resource Catalog to ensure it has been saved.
Next, open your dataflow. Here, we are writing data from a delimited source to an Excel destination. The file in the source is extracted from the variable where the FilePath is defined.
The variable here comes from the workflow, where the ContextInfo is typically used with the “Dropped File Path” frequency.
Now close the project and open the Scheduler. Here, amongst the Frequency options choose “When File is Dropped” and then select Browse Cloud Folders.
A dialog box will appear displaying your deployed Cloud Connection. Select any folder and set it as the directory to watch.
Enable polling and set the time interval to 30 seconds. This will ensure that the directory is checked every 30 seconds to see if a file has been dropped. Finally, save the scheduler settings and drop a file in the designated folder to verify its functionality.
Final Words
As businesses increasingly rely on data for informed decision-making, the importance of file drop automation in the cloud becomes evident. This feature equips you with a competitive edge in managing the rising volume of information while promoting agility, adaptability, and efficiency in the dynamic business landscape.
LIKE.TG’s file drop automation of cloud-based sources is an efficient and user-friendly feature that can significantly enhance your data management and transfer processes. By utilizing it, you can not only save valuable time but also reduce the likelihood of errors, ultimately leading to a boost in productivity.
To experience the benefits of this feature firsthand, download LIKE.TG’s 14-day free trial today!
Ready for a Workflow Upgrade?
Leverage LIKE.TG's new Cloud File Drop Automation to seamlessly process files dropped into your cloud-based folders. Effortlessly manage files and enhance workflow efficiency in just a few clicks.
Try it Now!
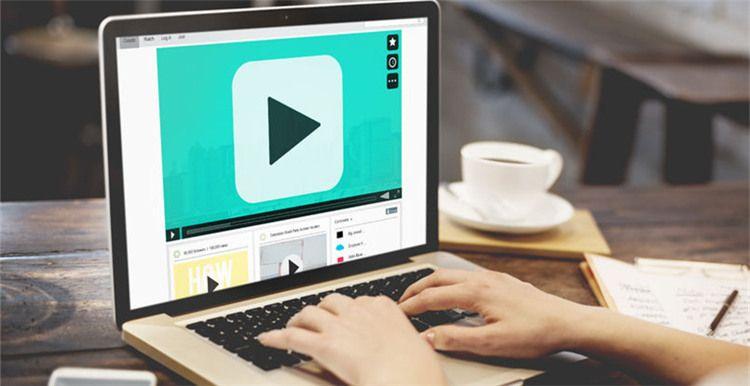
Build an Agile Data Warehouse with an Iterative Approach
If you have had a discussion with a data engineer or architect on building an agile data warehouse design or maintaining a data warehouse architecture, you’d probably hear them say that it is a continuous process and doesn’t really have a definite end. And that, in essence, is pretty much the truth. A successful data warehouse design generally uses an agile approach, iterative development methods that ensure delivering quality insights to end-users based on current business data. Fortunately, cloud data warehouse providers, like Microsoft Azure and Amazon Redshift, offer rapid flexibility and scalability options that make adopting this approach relatively easier than the rigid traditional frameworks. And consequently, having a constantly evolving architecture means you will have access to accurate, up-to-date data to fuel your analytics, allowing teams and departments to meet their respective goals. As Geoffrey Moore rightly said: “Without big data analytics, companies are blind and deaf, wandering out onto the web like deer on a freeway.” So, how can you build your own agile data warehouse design, how does this iterative data warehousing solution work, and what results can a data warehouse team of engineers and business analysts expect from it? Steps to Build a Data Warehouse Creating a More Adaptable, Responsive Data Warehouse Infrastructure In an iterative development model, the data warehouse is in a constant state of improvement and evolution. Instead of creating an architecture that answers all the queries on day one, your team focuses on the information that matters to your business users the most. You need to prioritize what’s important, put them into small manageable segments, and then take several rounds to modify your data warehouse architecture based on your business requirements. To achieve this, data teams work in multiple sprints and cycles, making changes to the ETL flows, dimensional models, and other data processes after taking feedback from business analysts and other stakeholders. After every cycle, continuous feedback ensures that these implementations provide a true picture of business performance and effectively address pain points and gaps. Build Your Data Warehouse From Scratch within a matter of days - 14-day Free Trial Try It Now! What do you need to build an agile data warehouse? Aside from a dedicated team of data architects and analysts, you’ll essentially need a data warehousing tool that offers a codeless development environment to facilitate rapid changes, allowing you to kick the rigidity out of the architecture. Main Components of LIKE.TG’s Data Warehouse Builder With that said, almost any organization can deploy an agile data warehousing solution, provided that it has the right technology stack fueling the initiative. In essence, a data warehousing toolshould provide: Source data modeling capabilities that can easily map and build relationships among your data sources Dimensional modeling functionality that can help determine facts and dimensions based on the source data and generate the schema and tables that would fit into your destination warehouse A high-performance ETL engine to simplify and accelerate data aggregation and offer an array of transformation options, like normalization, denormalization, merge, join, and more The option to switch to ELT or pushdown mode to reduce time to transform and access business data quickly Data quality modules to ensure each byte of data meets the quality standards of your organization A wide range of web applications, databases, and cloud connectors to connect and bring your business data to your data warehouse Slowly Changing Dimensions (Types 1-6) to control how different data fields are populated in database tables Support for OData service to provide secure ways to end-users to access data for reporting and analyses This is not an exhaustive list but just an overview of the major features and functionalities required. Now, you may find many solution providers that patch together several tools and build a customized package to close the functionality gaps. However, the recommended way is to opt for a unified platform that checks all these boxes to meet your data warehousing needs. Discover best practices to manage high volume data warehouses Download Whitepaper Okay, Sounds Great. But Why Should I Consider This Approach? Well, here is why: The main purpose of any agile data warehouse design implementation is to provide answers backed by trustable data based on the current business scenario. But the problem is that growing companies acquire new data sources and make changes to the information flow within apps and databases to match the pace of their increasing business needs. All these new sources and structural updates naturally have to be factored in the existing data models and reflected in the centralized repository to supply accurate, trusted data to end-users. As such, the iterative approach follows the agile methodology that allows you to design, develop, and deploy the most flexible, result-oriented architecture to build your enterprise data warehouse. You can easily include new data streams without having to revisit or rewrite ETL scripts, and that too in a matter of a few hours, if not minutes. That’s largely because modern data warehousing tools provide a code-free development environment and end-to-end process automation, allowing you to make quick changes to your flows and build better ETL processes. Not only that, you can enjoy the benefits in various scenarios as well. Whether it is planning and strategizing the data warehousing process, deploying prototypes to test its feasibility, or performing ETL testing to ensure consistent results, this agile methodology facilitates the development process at every stage. Perhaps, the real value of this approach is seen in the business benefits organizations can yield with its implementation. These include but not limited to: Faster results to meet end-user Data Warehouse business intelligence (DW BI) analytics requirements Better alignment with the evolving business needs Ability to speed up prototyping and feasibility analysis Lower TCO due to streamlined development processes Higher quality, up-to-date insights into robust decision making Reduced project complexity due to smaller, more manageable cycles The ability to identify shortcomings faster, leading to better risk management Greater transparency into the progress, performance, and challenges in each iteration Supercharge your BI initiatives and accelerate time-to-insight journey Get Started A Solution that Fits the Bill “The traditional architecture is much too slow. We no longer have the luxury of two to three months. We need to be able to tell users, ‘We can have that for you — in two to three days.'” – Rick van der Lans From source to insights – 7 steps And this is where modern data warehousing solutions, like LIKE.TG DW Builder, come in that are known to cut down development time significantly through end-to-end automation. It provides a unified data warehousing framework that enables a business to go from gathering requirements all the way to analytics via direct integration with data visualization software. Interested in creating your own data warehouse based on an agile, iterative development method? Take the first step and get a personalized live demo of LIKE.TG DW Builder.
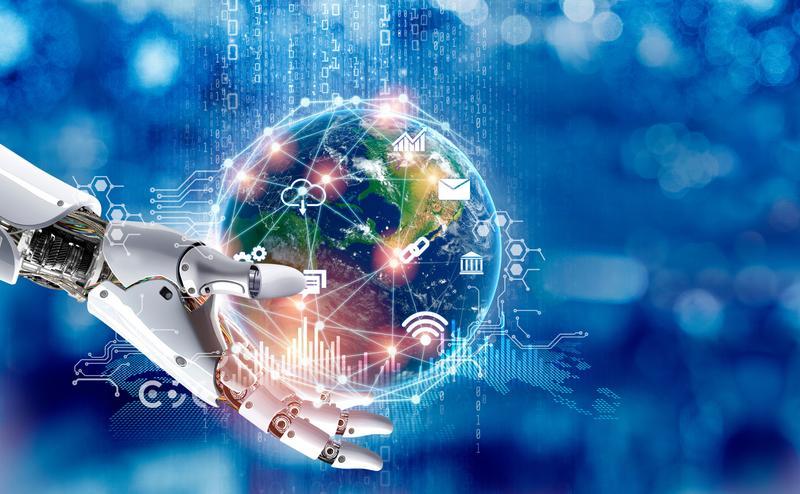
SOAP vs. REST: Which API Design is Right for Your Business?
According to Slashdata, nearly 90% of developers use APIs in some capacity. APIs enable developers to efficiently build software applications by abstracting away the complexity of low-level software layers—allowing developers to focus on core functionalities. Whether you’re a business or IT professional, understanding the nuances of API development is crucial for your organization’s success. There are two main API building methods: SOAP and REST. These methods differ significantly in their approaches and characteristics, each with its own set of advantages and considerations. What is SOAP? SOAP, or Simple Object Access Protocol, is a protocol-based approach to API development. It follows strict rules for communication, using XML as its message format. SOAP APIs are known for their structure, built-in error handling, security features, and stateful capabilities. What is REST? REST, which stands for Representational State Transfer, is an architectural style for building APIs. It’s all about simplicity and flexibility. REST APIs use various formats for message exchange, including JSON and XML. They are inherently stateless and rely on the underlying transport protocol, usually HTTP, for security and error handling. (Source: Seobility) SOAP vs. REST: Which API Suits Your Business? The way APIs handle communication, message formats, state management, error handling, and security can significantly impact your development process and the performance of your applications. SOAP, a protocol-driven approach, and REST, an architectural style, offer distinct features that are worth exploring. Communication: Protocol vs. Architectural Style SOAP is a protocol that mandates a set of rules for communication. It relies on request and response messages, typically transmitted over HTTP, SMTP, or TCP. In contrast, REST is an architectural style that doesn’t dictate a particular protocol. It takes advantage of existing protocols, primarily using HTTP methods like GET, POST, PUT, and DELETE. In an enterprise-level inventory management system real-time communication between servers and client applications is crucial. SOAP would be ideal, as it defines a clear communication protocol, ensuring that data integrity and consistency are maintained. On the other hand, if you are developing a public-facing e-commerce website, REST’s architectural style, which leverages standard HTTP methods like GET, POST, PUT, and DELETE, would provide the necessary flexibility to interact with different clients and platforms while taking advantage of existing web protocols. Message Format: XML vs. Multiple Formats SOAP exclusively uses XML for message formatting, which ensures strict structure and data typing. REST, on the other hand, allows for multiple formats, including JSON, XML, and HTML. This flexibility can be a game-changer, especially in diverse development environments. A financial application requiring accurate and strict data representation, would be best suited to SOAP. SOAP, with its reliance on XML, ensures that financial transactions are consistently formatted, reducing the chances of data interpretation errors. In contrast, if you’re developing a social media platform, REST’s support for multiple message formats like JSON, XML, and HTML allows you to cater to a wide variety of clients, including web browsers, mobile apps, and third-party integrations, making it a versatile choice. State Management: Stateless (with options) vs. Stateless SOAP can be either stateful or stateless, depending on how you configure your API. In contrast, REST is inherently stateless, which simplifies server and client communication. However, this means you may need to manage states manually if required. Consider a multi-step transactional process like booking a flight ticket. SOAP’s stateful capabilities can help maintain the session throughout the booking process, ensuring that user data is consistently available across multiple requests. If you’re building a content management system where each HTTP request is independent and doesn’t rely on previous requests, REST’s stateless nature simplifies both server and client interactions, making it suitable for systems where maintaining session states isn’t a primary concern. Error Handling: Built-in vs. Dependent on Implementation SOAP comes with built-in error handling through standardized fault messages, making it easier to pinpoint issues. In REST, error handling is dependent on the implementation, often utilizing HTTP status codes. This flexibility can be both a blessing and a curse. When developing a healthcare information exchange system, the built-in error handling of SOAP, with standardized fault messages, ensures that any errors in transmitting critical patient data are immediately and clearly addressed, enhancing patient safety. In the context of a public-facing news website, REST’s flexibility in error handling allows you to tailor error responses to suit the specific needs of various clients. While this flexibility can be advantageous, it also requires a more meticulous hand in implementation. Security: WS-Security vs. Dependent on Protocols like HTTPS SOAP provides robust security features through WS-Security, making it an excellent choice for sensitive data and regulated industries. REST relies on the underlying transport protocol, such as HTTPS, for security, which is suitable for most use cases. A banking application that deals with sensitive financial transactions would benefit from SOAP’s WS-Security’s strong encryption and authentication, ensuring that customer data is protected to the highest standards and complies with regulatory requirements. However, for a weather forecast service that provides publicly available information, relying on the underlying transport protocol’s security, such as HTTPS, is a cost-effective and suitable choice. This minimizes the complexity of security implementation for non-sensitive data. These distinct capabilities and features illustrate how the choice between SOAP vs. REST is as complex as the specific requirements and constraints of your project. Your choice should align with the goals, resources, and nature of your business. Factors to Consider When Choosing Between SOAP vs. REST When standing at the crossroads of API design decisions, i.e., SOAP vs. REST, several critical factors come into play. Your choice between SOAP and REST isn’t just a technical matter; it’s a strategic decision that impacts your project’s success. Here are some key factors to keep in mind: Nature of the Project It’s all about matching your API to your project. For example, if you’re building a big enterprise system with lots of complex processes that need to be just right, SOAP is a good pick. It’s the sturdy, reliable option. But if you’re creating a dynamic public web app or working on smaller connections, REST is a more flexible option. Required Level of Security From a data security POV, keep in mind that if your API handles processes with confidential data assets like financial transactions or personal medical records, SOAP has stronger security features that’ll keep your data safe. For non-sensitive data, REST is both more cost effective and has enough security. Expected Volume of Traffic and Scalability Needs If you’re expecting a huge crowd and loads of data, REST is the go-to choice. It’s good at handling a lot of requests without getting bogged down. But if you need to keep meticulous access records, SOAP’s is the better choice. Integration with Existing Systems Another important factor is how your new API fits in with your current systems. If your organization already uses mostly SOAP-based services, a SOAP-based API will make your life easier and vice versa with REST-based services. The Skillset of the Development Team If your development team is skilled with XML and structured data, SOAP aligns well with their existing skill set. If their expertise leans toward web technologies, REST is quicker and easier. A solution that works irrespective of technical skill is a no-code API development solution. Conclusion Your decision when evaluating SOAP vs. REST should be driven by your unique business needs, technical demands, and future aspirations. There’s no one-size-fits-all answer, and that’s perfectly okay. SOAP and REST are like different tools in a toolbox, each designed for specific tasks. So, whether you opt for SOAP or REST, it’s all about crafting an API that perfectly fits your mission, ensuring that your digital endeavors are primed for success. Contact us to learn more about how LIKE.TG, a self-service no-code API design tool, can support your API strategy.
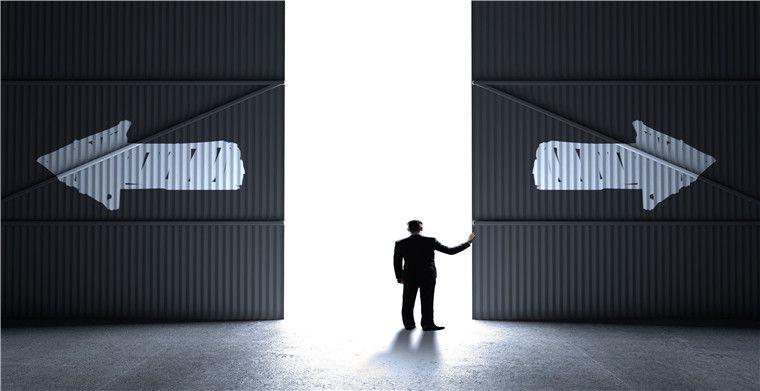
A Complete Guide to the Different Types of APIs
Although, APIs have a recent history, they have penetrated the digital ecosystem so quickly that everything today is dependent on them. From web development to mobile applications, APIs have become the building blocks of the digital world. Over the years, APIs have evolved significantly so today there are various types of APIs, each with its own purpose. Moreover, APIs are not only categorized based on their architecture but also based on their access policies. Therefore, it is crucial to understand the different nature of these APIs. If you’re confused about which API to use and when, this guide will help clear the confusion and will help you choose the right one! Let’s dive straight in. What are The Different Types of APIs? There are four main types of APIs: Public APIs Internal APIs Partner APIs Composite APIs Public APIs Public APIs, also known as external or open APIs, are APIs made available to developers, businesses, and the public by companies, organizations, or platforms. These APIs provide access to certain functionalities or data that the API provider exposes to external users or third-party developers. You can access these APIs via a form of authentication – usually an API key. Some public APIs are free while others require a payment based on the number of times the API is called. An example of a paid public API is the Google Translate API, which costs $20 for every 1 million characters translated. Internal APIs Internal APIs, also called private APIs or in-house APIs, are developed to be used within an organization. These APIs serve as the communication bridge between different components, services, or applications within a company and are not intended for external access or use by third parties. While internal APIs are flexible in their documentation and naming conventions, as they usually don’t follow the OpenAPI standard they are generally less secure than other types of APIs. Partner APIs Partner APIs are also called external APIs. These APIs facilitate communication and data exchange between different businesses, organizations, or entities and enable controlled access to certain functionalities, services, or data between partnering companies. Imagine a partnership between a cloud-based accounting software and a payment processing company. A partnership API allows the software to integrate with the payment processor’s services. The payment company provides the API to multiple companies in this way. Composite APIs Composite APIs allow you to execute multiple API requests into a single call. So, in short you can perform complex operations with a single call, reducing the number of round trips to the server and improving performance. Composite APIs are particularly useful for scenarios where multiple API calls are tightly related and need to be executed in a specific sequence. Think of an e-commerce website. There are multiple steps to making a purchase: Search for a product. Click on the result. Add to cart. Checkout. Rather than execute each call separately, it is more efficient to combine and execute them at once. API Protocols An API protocol refers to the set of rules, standards, and conventions through which communication occurs between different software components or systems. It outlines the structure and methods by which applications or services can interact and exchange data. It’s important to understand the different types of protocols as the way you create and manage your APIs is influenced by the protocol you use. The common API protocols are: REST SOAP GraphQL RPC REST APIs The full form of REST is Representational State Transfer. REST APIs use HTTP requests (GET, POST, PUT, DELETE) to interact with resources (data) via URIs. They follow stateless communication and generally use the XML format for data exchange. REST APIs are defined by: Resource-oriented:REST APIs treat data as resources,each with a unique identifier (URI or URL). Client-server architecture:REST APIs separate the client (application) from the server (API provider),allowing for independent development and deployment. Stateless:REST API interactions are stateless,meaning each request contains all the necessary information to be processed independently. Cacheable:Responses can be cached to improve performance and reduce server load. Read more about REST API When to use them: RESTful APIs are best suited for scenarios that require scalability, simplicity, and interoperability. They work well for web and mobile applications, where flexibility in data retrieval and ease of use are essential. Use them for CRUD operations (Create, Read, Update, Delete) on resources and when working in a distributed system environment. (Source: Seobility) SOAP APIs SOAP stands for Simple Object Access Protocol. These APIs use standardized XML for message formatting and typically operate over HTTP, TCP, SMTP, and other protocols. They employ a strict messaging format, including WSDL for defining the service interface. A SOAP API consists of the following components: SOAP Envelope:The outer layer of the SOAP message,containing the header and body sections. SOAP Header:Optional section that provides additional information about the message,such as routing or security information. SOAP Body:Mandatory section that contains the actual data being exchanged. SOAP Operation:A specific action that the API can perform,such as creating,retrieving,updating,or deleting data. When to Use: SOAP APIs are beneficial for complex enterprise-level applications that demand strong security measures, ACID transactions, and error handling. They’re suitable for scenarios where standards compliance and built-in mechanisms for data integrity and security are critical, such as financial transactions. They’re also protocol independent, unlike REST. Read more about SOAP APIs and how they compare to REST. GraphQL APIs GraphQL is not actually an API protocol rather it is a query language and runtime for APIs. It allows clients to request specific data structures using HTTP requests. GraphQL APIs use a single endpoint and a flexible query language with unlimited data schemas. Unlike REST APIs, which require multiple round trips to fetch the desired data from different endpoints, GraphQL allows clients to specify the exact data they need in a single request. With GraphQL, clients can specify the shape and structure of the data they require using a query, and the server responds with JSON data that matches the query, which help reduce over-fetching and under-fetching. When to Use: GraphQL is ideal when there’s a need for optimized data fetching tailored to client requirements. It suits scenarios where clients demand flexibility in data retrieval, such as in mobile applications or when dealing with complex data structures. RPC APIs RPC stands for Remote Procedure Call, and RPC APIs facilitate communication between different processes by allowing one program to execute procedures on another system. RPC protocol return a method instead of a resource. For example, an RPC protocol might return a server confirmation or an error, while a REST protocol might give a text document. There are various implementations of RPC, such as gRPC, XML-RPC, and JSON-RPC. Each has its own protocols and methodologies for handling remote procedure calls. These APIs are commonly used in building distributed systems, client-server architectures, and other scenarios where different components need to communicate over a network. When to use: RPC APIs are useful where developers need to invoke functions or procedures on remote servers. They are a suitable option for lightweight data packs and basic requests. Now that you understand the different API types, let’s look at more examples of APIs around us. APIs are All Around Us The digital world is filled with APIs of different types. They act as bridges between applications, allowing them to exchange data. Here are some examples of APIs commonly used in various aspects of our lives: Weather APIs: These APIs provide real-time and historical weather data, including temperature, precipitation, forecasts, and weather alerts. They are used by weather apps, news websites, and smart home devices to keep us informed about weather conditions. Maps and Navigation APIs: These APIs provide maps, directions, and traffic information, enabling navigation apps and location-based services. Google Maps and Apple Maps fall in this category. Social Media APIs: Social media platforms like Facebook, Twitter, and Instagram expose APIs that allow developers to integrate social media features into their applications. This enables sharing content, accessing user profiles, and managing social interactions. Payment APIs: APIs like PayPal, Stripe, and Square facilitate online and mobile payments. This allows merchants to accept payments securely and integrate payment processing into their websites or apps. E-commerce APIs: These APIs provide the building blocks for e-commerce platforms, like Amazon and eBay, enabling product catalog management, order processing, inventory tracking, and customer relationship management. Communication APIs: APIs like Twilio and Plivo enable developers to integrate SMS, voice, and messaging functionality into their applications, allowing for real-time communication and notifications. Machine Learning APIs: APIs like Google Cloud AI, Amazon Rekognition, and Microsoft Azure Cognitive Services provide machine learning capabilities for tasks like image recognition, natural language processing, and sentiment analysis. Open Data APIs: Governments and organizations around the world publish open data APIs that provide access to public datasets, enabling developers to create data-driven applications and visualizations. Choosing the Right API – Factors to Consider The type of API you choose will depend on your particular use case. However, there are certain universal principles that you need to keep in mind while picking an API such as: Documentation: Solid documentation is a big plus for both users and developers as it helps developers implement APIs efficiently and successfully. SOAP APIs have a strict set of rules, while REST are more flexible. Security: APIs should use robust encryption and authorization standards, such as OAuth 2 and SSL, to protect sensitive data. Some APIs, like RPC, are less secure than others and are only suitable for internal use. SOAP APIs provide better security. Ease of use and developer familiarity: APIs should be easy to use. Your developers should also be familiar with the chosen protocol as it simplifies development. REST is a good option as it is the most widely used protocol. API adoption: A good API is easy to acquire. The signup process should be simple with robust authentication. Another aspect of ensuring easy adoption is good chat and live support. This isn’t limited to the sign up – good support also extends to debugging errors. REST APIs are popular for being very user-friendly, being widely used in web services. Scalability:Consider the expected usage volume and traffic patterns of your API. If you anticipate high traffic or frequent requests, opt for a protocol that can handle large amounts of data efficiently. REST APIs are highly scalable, and a good option. Vendor ecosystem:Third party support is also important. Evaluate the vendor ecosystem surrounding the protocol, including the availability of third-party tools, libraries, and management platforms. Improve Your Knowledge of APIs This blog is just an introduction to some of the various types of APIs. Each API type is very complex and interesting and should be studied thoroughly. Now that you have a basic understanding, you can go ahead and learn more about each individual type in more detail. Check out our other API blogs for a more in-depth exploration: Web API vs. REST API SOAP vs. REST CRUD APIs Want to build robust APIs in a code-free environment? Check out our guide to creating APIs. Learn more about LIKE.TG’s API solution. LIKE.TG enables teams to easily and securely create, publish, and monitor APIs in a no-code way, simplifying the entire API management cycle.
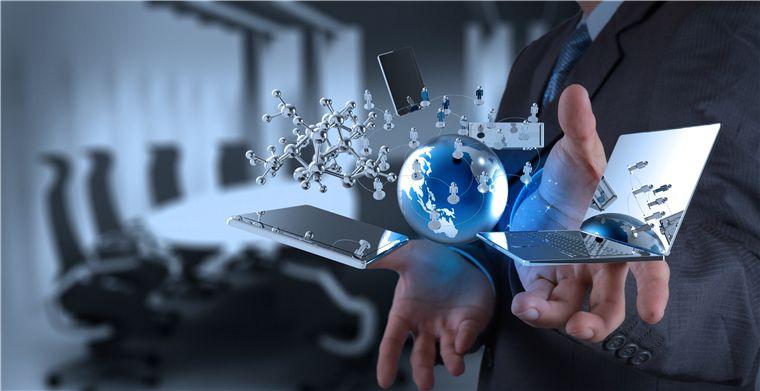
10 Best Informatica Alternatives & Competitors in 2024
Informatica, one of the key players in the data integration space, offers a comprehensive suite of tools for data management and governance. However, for reasons such as cost, complexity, or specific feature requirements, users often seek alternative solutions. In this article, we are going to explore the top 10 Informatica alternatives so you can select the best data integration solution for your organization. But before we get to it, let’s quickly get the basics out of the way and understand why there is a need for an alternative data integration solution to Informatica. What Is Informatica? Informatica is an enterprise-grade data management platform that caters to a wide range of data integration use cases, helping organizations handle data from end to end. The services it provides include data integration, quality, governance, and master data management, among others. The overall goal is to combine company-wide data into a single source of truth for BI, reporting, and analytics. Key Features of Informatica Informatica offers several features that help users with their data management and integration projects. Some of its features include: Database Management Like other data management and integration platforms, Informatica enables users to connect to and integrate data from diverse databases, for example, Oracle, SQL Server, etc. It uses the metadata-driven approach to optimize performance, streamlining the overall database operations. Data Transformation Putting aside the fact that it’s not 100% no-code, Informatica offers a rich set of transformations. These include aggregator, data cleanse, data masking, filter, to name a few. Orchestration Informatica’s Data Integration Hub makes the best use of its orchestration capabilities, coordinating data movement across different systems and applications. API Integration Besides ETL, Informatica also offers API integration features that facilitate interaction between applications and services. Access Control Informatica enables users to fine-tune access controls and manage permissions for data sets. They can also set permissions on database, domain, and security rule set nodes to authorize users to edit the nodes. Data Security As far as security is concerned, Informatica employs a range of measures tailored to its suite. These include target database credential management, encryption, access controls, and data masking. So, if the platform offers such a vast set of features, why should users then consider the different Informatica alternatives, or competitors for that matter? Let’s find out. LIKE.TG—One of the Best Alternatives to Informatica for Data Integration Looking for a powerful yet easy-to-use alternative to Informatica for data management and integration? Look no further. Try LIKE.TG. Learn More Why Consider Informatica Alternatives for Data Integration? Informatica has established itself as a key player in the data management and integration space. However, this feat is not enough for companies in today’s rapidly evolving business landscape, especially if we consider the increasing number of new SaaS startups. These businesses need dynamic solutions that are: The best fit for their use case Easy to implement, learn, and use Economical with transparent pricing Committed to exceptional customer support Additionally, with the global data integration market size set to reach USD 39 billion by 2032, numerous opportunities for innovation and growth exist, particularly in areas like real-time data integration, data quality and governance, and the integration of unstructured data and emerging technologies, making space for new players to enter the market. This means that the consumers now have a myriad of options to choose from. Here are some reasons why an Informatica alternative could make more sense for your business: Cost Considerations While informatica offers a breadth of products and services, sometimes it becomes a simple case of getting overwhelmed and paying for features that users will hardly ever use. Additionally, Informatica is relatively more expensive when compared to other options, such as LIKE.TG. The already complex pricing structure lacks transparency and their website does not offer full pricing information. Its implementation requires significant investments in hardware and infrastructure, making the overall total cost of ownership (TCO) much higher—even in the long run. High Complexity Another major challenge with Informatica is its complexity—this is more of an inherent issue with the platform. The platform is so vast and complex to use that at times even the Informatica-certified data professionals face challenges. This is primarily due to its different modules/services using vastly different user interfaces (UI). Specialized Use Cases An organization that has a highly specific use case might find that an alternative solution is better aligned with its needs. While Informatica is a comprehensive and versatile platform, its extensive feature set may include functionalities that might be unnecessary or less relevant to their unique use case. It’s like choosing a general-purpose tool with a wide range of features instead of a precision tool for a specialized job. Resource-Intensive Informatica, especially its PowerCenter, is very resource-intensive compared to its competitors, requiring significant processing power and memory. However, the performance is still not comparable. Based on reviews on popular channels, such as G2, Gartner, etc., users have frequently reported performance issues and slowdowns, raising several eyebrows. Top Informatica Alternatives to Consider in 2024 LIKE.TG LIKE.TG is an end-to-end, automated data management and integration platform powered by artificial intelligence (AI). It features a truly unified, 100% no-code UI that delivers consistent user experience across all its products. The tool enables users of all backgrounds to build their own data pipelines within minutes. LIKE.TG Data Stack The platform stands out by offering a flexible and cost-effective approach to meet the unique needs of every business. Unlike Informatica’s rigid pricing structures, LIKE.TG allows you to collaborate with their expert data solution team and cherry-pick the modules that align precisely with your use case. This ensures that you pay only for the functionalities that are essential to your operations. LIKE.TG includes: ReportMiner for unstructured data extraction in bulk Centerprise for data integration and building data pipelines Datawarehouse Builder for data warehousing EDIConnect for EDI management API management for designing, testing, publishing, and monitoring APIs The platform offers several features that easily make it one of the best Informatica alternatives. For example, with LIKE.TG, you can: Establish native connectivity to a range of data sources and destinations, both on-premises and cloud-based. Transform and shape your data the way your business needs it using pre-built transformations and functions. Build ETL and ELT pipelines without writing a single line of code. Ensure only healthy data makes it to your data warehouses via built-in data quality management. Automate and orchestrate your data integration workflows seamlessly. LIKE.TG—One of the Best Alternatives to Informatica for Data Integration Looking for a powerful yet easy-to-use alternative to Informatica for data management and integration? Look no further. Try LIKE.TG. Learn More Talend (acquired by Qlik) Talend is another data management solution that enables businesses to drive their BI and analytics. Talend’s Data Fabric is a cloud-independent platform covering the entire data lifecycle. It features data preparation, transformation, integration, as well as data quality and governance. Pros Enables users to build ETL pipelines and move data from a myriad of sources. Offers built-in transformations, including unions and joins. Offers an open-source version, making it accessible to a wide range of users and organizations. Cons Despite a visual interface, mastering all the features and capabilities takes considerable time. It’s not a completely no-code solution. Some users have reported performance issues with Talend, particularly when dealing with large datasets or complex transformations. Real-time processing capabilities are not as robust compared to some other data integration tools. Some important features, such as scheduling, are only available in the enterprise version that comes with a premium price tag. Given the limitations, and its recent acquisition, many are actively looking for Talend alternatives. Alteryx Alteryx offers a solution that allows users to access, manipulate, and analyze data without coding. It enables users to connect to a wide range of data sources and ETL data in a visual, drag-and-drop environment. Pros Its user-friendly interface makes it easy to use for most users. It excels in data blending and preparation, allowing users to clean, transform, and combine data from various sources. Multiple users can take advantage of the workflows by using the server instead of purchasing multiple individual licenses. Cons While Alteryx offers a capable reporting ability, it’s not as versatile as other competing solutions, such as Power BI. Applying logic is limited to columns—users have to work around when working at the record/cell level. Error-handling, while available, doesn’t provide greater visibility into dataflows. Additionally, users report that the error messages can be vague. Oracle Oracle Data Integrator (ODI) enables data movement and transformation in bulk. It’s primarily an ELT platform that is highly interoperable with other Oracle programs, making it a great Informatica alternative for businesses that have invested in other Oracle products and services. Businesses can use ODI with GoldenGate and unify data loading with synchronization, keeping their data warehouses up to date. Pros A great addition to technology stacks that already include other Oracle products as it integrates seamlessly within the Oracle ecosystem. Simple and straightforward UI makes it easy to use and navigate. Most use cases can be catered to in a single interface without having to switch between multiple interfaces. Cons While the tool is generally easy to use, it’s not particularly intuitive, especially when troubleshooting. The price can be prohibitive as the TCO in the long run is higher compared to competition. User review sites report frequent performance issues and suboptimal customer support, especially when it comes to Oracle Data Integrator. SnapLogic SnapLogic is an iPaaS that offers application, data, and cloud integration for enterprises. Like other tools, SnapLogic also relies on low-code/no-code approach to fast-track application and data integration. Pros Offers a dashboard to monitor configured pipelines and integrations easily. Powered by a visual UI that’s simple and easy to use and navigate. Provides support for real-time integration, as well as batch processing. Cons Users have reported that the resource utilization is not accurately displayed on the dashboard. The support for XML files is limited, especially the ones with mixed content. While it sports a visual UI, it’s not one of the most intuitive ones. Error-handling and available documentation lack depth. Ab Initio Ab Initio is an enterprise-level self-service data platform offering a range of capabilities, including batch and real-time data integration, BI and analytics, automation, as well as data quality and governance. Pros Ab Initio’s end-to-end graphical development makes it simple for users to easily process data. The ability to handle very large data sets—users can process billions of records in minutes. Overall, a feature rich ETL platform powered by parallel processing. Cons One of the biggest downsides of Ab Initio is the significant upfront licensing and infrastructure cost. While its graphical development environment is based on the low-code approach, it still has a steep learning curve for new users. Requires databases running in the background on the servers to function, adding to the already expensive solution. IBM InfoSphere DataStage IBM’s InfoSphere DataStage is a data integration platform used to design, develop, and run workflows that transform and move data. At its core, it’s an ETL tool that leverages graphical notation to build data integration solutions. Pros The solution offers high availability ands scalability—users can use multi-instance jobs that scale easily. Users can leverage complex transformations without having to write code. Metadata and data quality features are built into the solution. Cons Compared to its competitors, IBM InfoSphere DataStage is a more expensive solution. The initial setup being a complex process and the UI not being as user-friendly as the competition make it hard to justify the higher price point. Some processes can be time-consuming, for example it takes a lot of time to trigger jobs and then view logs. AWS Glue AWS Glue is another Informatica alternative, which is a serverless data integration solution offered by Amazon as part of Amazon Web Services (AWS). The overall goal with AWS Glue is to make data preparation faster and cheaper for machine learning (ML) and analytics. It does so by simplifying data integration by modernizing the ETL process using automatic provisioning and worker management. Pros AWS Glue offers a user-friendly UI that’s simple and intuitive, keeping the initial learning curve smooth. Pay-as-you-go pricing structure ensures businesses only pay for what they need. It also eliminates the need to provide capacity in advance, which simplifies scheduling. Offers advanced users the ability to create ETL scripts with custom endpoints. Cons While the UI is user-friendly, it’s not entirely beginner friendly. Some tasks will require Python and Scala expertise. While is offers support for creating ETL scripts, only two programming languages are supported: Python and Scala. AWS Glue works flawlessly as long as the user works within the AWS ecosystem. Integrating with platforms outside of Amazon is tricky. Microsoft SQL Server Integration Service As the name suggests, Microsoft SQL Server Integration Service (SSIS) is a service in Microsoft’s SQL Server software that can be used to integrate data from multiple sources. It allows users to build ETL-based enterprise-grade data integration and transformation solutions. Being Microsoft’s own service, SSIS offers superior interoperability for businesses that have invested in Microsoft’s other services, making it a great Informatica alternative. Pros Included with SQL Server licenses, making it a cost-effective option for smaller organizations already invested in the Microsoft ecosystem. Drag-and-drop interface and tight integration with SQL Server make it user-friendly for beginners and experienced developers alike. Can scale to handle large data volumes by utilizing multiple servers and parallel processing. Cons Due to limited availability of pre-built connectors, it requires scripting or custom development for some data sources and formats. Some cases require investment in additional tools due to its limited data transformation capabilities. The visual representation in the IDE sometimes does not adequately reflect the underlying code, making code review and collaboration challenging. Integrate.io Integrate.io is another Informatica alternative for businesses looking to integrate data via ETL, ELT, and CDC. It’s a cloud-based data integration platform that may be a fit for businesses of all sizes, depending on the use case. Like other visual data integration platforms, Integrate.io also offers similar features, such as pre-built connectors, transformations, etc., making it easy to use for users with varying technical expertise. Pros Offers transparent pricing based on the number of connectors used, eliminating the complex and often expensive licensing fees. Provides enterprise-grade transformation capabilities that are easy to work with. Responsive customer support that’s available 24/7 in all time zones. Cons Debugging can be a challenge, especially when fault records lack granularity and error logs lack specificity. While it offers a transparent pricing model based on flat rate pricing, the yearly costs can turn out to be higher than the alternatives. Even though the platform is low-code, the UI can be challenging for businesses without dedicated technical teams as the complexity of integrations increases. Simplify and Accelerate Data Integration With LIKE.TG Build a connected enterprise data architecture with a 100% no-code platform that simplifies and accelerates data integration. Download Free Trial Here’s Why LIKE.TG Is the Best Informatica Alternative The decision to opt for a data integration solution is not straightforward since most solutions offer similar features and cater to a wide range of use cases. Ultimately, it comes down to an organization’s unique requirements, including data volume and variety, integration complexity, desired level of data automation, security requirements, budget constraints, and future scalability, and how these requirements align with the capabilities and limitations of available solutions. In other words, it’s about finding the perfect fit. In this context, LIKE.TG stands out from the abovementioned Informatica alternatives as it offers a complete data integration and management suite with a unified user interface that delivers consistent experience throughout. This means that the platform can be used by business and technical users alike. Here are some more reasons to consider LIKE.TG as an alternative to Informatica: Simple and straightforward initial setup process significantly reduces the time required for solution deployment. 100% no-code, visual drag-and-drop UI keeps the learning curve short and smooth. Parallel processing ETL/ELT engine delivers superior performance even when dealing with high volume data sets. Pre-built connectors for a breadth of data source types, including file formats, databases, data warehouses, applications, cloud storages, web services, and file providers. The ability to instantly preview data at every step of the process with a couple of clicks. LIKE.TG offers the capability to build an entire data warehouse from scratch without writing a single line of code. The ability to build a custom connector for the required source or destination seamlessly. Proactive customer support that’s available round the clock regardless of geographic location. Don’t overpay for complexity. Get more for less with LIKE.TG. Download a 14-day free trial to get started today. Alternatively, you can get in touch with us directly at +1 888-77-LIKE.TG, where one of our data solutions experts will be happy to assist you with your use case.
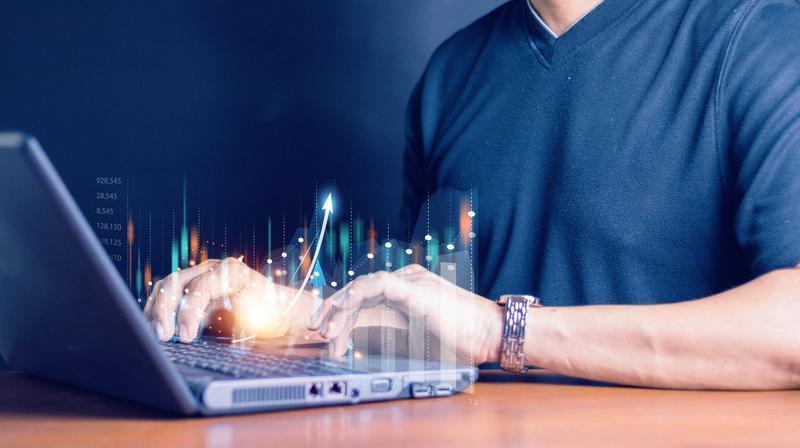
Data Pipeline Monitoring: Metrics and Best Practices
A report by KPMG indicates that only 35% of executives report a high level of trust in an organization’s use of data and analytics. The other 65% face difficulties transporting and using data. This highlights the need for effective data pipeline monitoring. Data pipeline monitoring enhances decision-making, elevates business performance, and increases trust in data-driven operations, contributing to organizational success. What is Data Pipeline Monitoring? In its simplest form, data pipeline monitoring is the ongoing process of overseeing and managing the flow of data from its source to its destination. It is a systematic process that involves tracking, validating, and ensuring the smooth operation of a data pipeline, which is a set of processes that move data from one system to another. The primary goal is to maintain the integrity and reliability of data as it moves across the pipeline. Importance of Data Pipeline Monitoring Data pipeline monitoring is crucial for several reasons: Data Quality: Data pipeline monitoring is crucial in maintaining data quality. By continuously tracking the data, errors and inconsistencies can be detected and rectified promptly. This ensures that the final data used for analysis and decision-making is accurate and reliable. Efficiency: Data pipeline monitoring identifies any bottlenecks or inefficiencies in data processing. Optimizing these processes allows data to be processed faster and more efficiently. Reliability: Regular monitoring ensures that the pipeline is functioning correctly and that data is delivered to its destination on time. Regulatory Compliance: In many industries, such as healthcare and finance, regulation govern data handling. Data pipeline monitoring ensures compliance with these regulations by providing a clear record of how data is processed and where it is stored. Benefits of Data Pipeline Monitoring Having established the significance of data pipeline monitoring, let’s explore the practical benefits it offers: Minimized Data Processing Latency Monitoring tools enable organizations to identify and address performance bottlenecks in real-time, leading to minimized data processing latency. This ensures that data moves efficiently through the pipeline, supporting timely analytics, reporting, and other critical business processes. Automation and Orchestration Monitoring can be integrated with automation and orchestration tools to trigger responses or corrective actions based on predefined conditions. This can help in automating routine tasks and ensuring the reliability of the pipeline. Enhanced Data Security Data pipeline monitoring plays a vital role in ensuring the security of sensitive information as it moves through the pipeline. By continuously monitoring access patterns, data flow, and user interactions, organizations can quickly identify and respond to any suspicious activities or potential security breaches. Data Monitoring Techniques Let’s explore some key data monitoring techniques, also known as the three pillars of data observability, for optimizing data pipeline performance: Metrics Metrics are numerical measurements that quantify various aspects of a system’s performance. They provide insights into aspects like data flow rate, error count, or processing time, offering a comprehensive understanding of the system’s efficiency, reliability, and overall health. Logs Logs are textual records that document events, errors, and activities within a system. They provide a detailed narrative of system behavior and can include information such as error messages, timestamps, and user interactions. They are essential for troubleshooting as they offer a historical record of events, helping identify and rectify any issues in the data pipeline. Traces Traces provide a detailed view of how data requests move through a specific application, helping identify any bottlenecks or issues. Their scope, however, is limited to that particular application. To gain a comprehensive view of the entire system, traces are often used in conjunction with other tools like system metrics and network monitoring solutions. Key Concepts in Data Pipeline Monitoring To effectively monitor these pipelines, it’s important to understand some key concepts that form the foundation of efficient data pipeline monitoring, helping businesses optimize their data flow and make informed decisions. These include: Data Freshness Data freshness ensures that the information used for analysis and decision-making is current, thereby enhancing the accuracy of insights. Data Distribution Data distribution describes how data is spread across different values or ranges. It involves identifying and handling missing information and unexpected spikes or deviations from the anticipated range of values to prevent skewed results. Data Volume Data volume refers to the quantity of data that is generated and processed. Efficient handling of data volume prevents data overload and underutilization, ensuring optimization of data storage and processing capabilities. Data Schema Data schema refers to the structure of data, including its organization, format, and the relationships between different data elements. A well-defined data schema is vital for accurate data analysis and plays a significant role in maintaining the integrity and quality of the data. Data Lineage Data lineage involves tracking data from its source to its destination, including all the transformations it undergoes in the process. It ensures transparency and accountability of the data, aiding in tracing any errors or inconsistencies back to their source and improving the overall quality of the data. It is also crucial for regulatory compliance and data governance. 6 Essential Metrics for Data Pipeline Monitoring Data pipeline monitoring involves six important metrics that help assess the performance, efficiency, and reliability of the data flow. These metrics are: Latency: This metric measures the time it takes for data to move from the point of entry to its destination in the pipeline. High latency can indicate bottlenecks or performance issues within the pipeline. Throughput: This measures the amount of data that can be transported through the pipeline in a given time frame. It helps in understanding the capacity of the pipeline and can indicate if scaling is required. Error Rate: This metric tracks the number of errors that occur during data processing. A high error rate can indicate issues with data quality or problems in the pipeline’s processing capabilities. Availability: This is a measure of the data pipeline’s reliability and accessibility. It quantifies the percentage of time the pipeline is functional and capable of processing data without interruptions or failures. Data Drift Detection: This metric monitors for unexpected changes in the data’s characteristics or structure over time. It helps in identifying significant shifts in the data’s statistical properties that could impact data analysis and decision-making. System Health: This involves monitoring the operational parameters of the system running the data pipeline, such as CPU usage, memory usage, and network bandwidth. It helps in identifying any system-related issues that could affect the performance of the data pipeline. How Data Pipeline Monitoring Works Data pipeline monitoring plays a crucial role in establishing the groundwork for maintaining data integrity. Its main steps include: Instrumentation Instrumentation involves embedding monitoring tools or agents at strategic points within the data pipeline architecture. These tools act as sensors, capturing relevant data and metrics as the data moves through various stages of the pipeline. Instrumentation is the first step to enable continuous monitoring. Data Collection Once the monitoring tools are in place, they continuously collect data on different aspects of the data pipeline’s operation. This includes metrics such as data throughput, latency, resource utilization, and error rates. The collected data serves as the basis for assessing the performance and health of the data pipeline. Real-time Monitoring Real-time monitoring ensures that insights into the data pipeline’s status are available immediately. Monitoring tools process and analyze incoming data in real-time, allowing for the prompt detection of issues or anomalies. This timely awareness is crucial for maintaining the efficiency and reliability of the data pipeline. Alerting System An alerting system is configured to generate notifications or alerts based on predefined criteria. For example, if the data throughput drops below a certain threshold or if an error rate surpasses a predefined limit, the alerting system triggers notifications. Alerts enable data engineers and operators to respond quickly to emerging issues. Logging Logging involves recording detailed information about events, errors, and activities within the data pipeline. Logs serve as a historical record that can be used for auditing, debugging, and understanding the sequence of events, which are essential for post-incident analysis and troubleshooting. Best Practices for Data Pipeline Monitoring Effective data pipeline monitoring requires a strategic approach to ensure the reliability, efficiency, and accuracy of data flow. Here are some best practices: Establish Clear Metrics: Identify and define the key metrics that will provide insights into the pipeline’s health and performance. These metrics will serve as the foundation of your monitoring strategy. Implement Real-Time Monitoring: Use tools and solutions that allow for real-time monitoring of your data pipelines. This enables immediate detection and resolution of issues. Automate Alerts: Set up automated alerts to be triggered when predefined thresholds are reached. This ensures prompt attention to potential issues, even when you’re not actively monitoring the dashboard. Maintain End-to-End Visibility: Ensure your monitoring strategy provides a comprehensive, end-to-end view of your data pipeline. This allows for precise pinpointing of issues and a better understanding of data flow and transformations. Prioritize Data Quality: Implement checks at various stages of the pipeline to ensure data quality. This includes validating data formats, checking for missing values, and identifying any inconsistencies. Monitor Regularly: Establish a routine practice of regularly assessing your data pipeline’s performance and seek to make incremental improvements. This will help ensure your data pipelines remain efficient, robust, and capable of handling your evolving data needs. Monitor your Data Pipelines Seamlessly LIKE.TG’s Centerprise helps you seamlessly monitor your data pipelines using a user-friendly and easy-to-use interface. Try it now! Book Free Trial Start Monitoring Your Data Effectively Managing data pipelines is integral as it provides insights into their performance, enabling organizations to promptly identify and rectify any issues that may arise. By implementing robust monitoring, organizations can enhance their data analysis and gain a competitive edge. Organizations must build a strong data monitoring strategy and foster a culture of data awareness and responsibility across all levels of the organization. This will ensure that monitoring data pipeliens is not just a one-off task but an ongoing commitment. Need help building and manage your data pipelines? Start your 14-day free trial with LIKE.TG’s Data Pipeline Builder now! Take Control of your Data Pipelines Discover how LIKE.TG's advanced features empower your team to proactively manage, optimize, and future-proof your data workflows. Download Free Trial
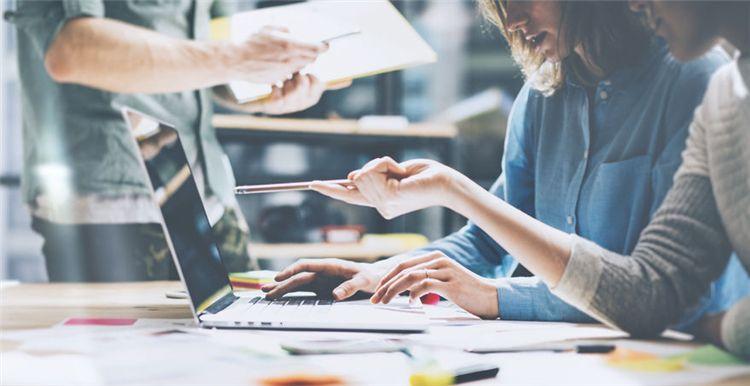
How to Build a Data Pipeline: A Step-by-Step Guide
Data plays a significant role in business growth and digital initiatives for approximately 94% of enterprises. However, the full potential of these data assets often remains untapped, primarily due to the scattered nature of the data. The solution lies in constructing a structured data pipeline, a task that can be resource-intensive and time-consuming. A simple pipeline may take weeks to build, while a more complex one could span months. Therefore, it is imperative for your organization to invest in appropriate tools and technologies to streamline the process of building a data pipeline. This blog details how to build a data pipeline effectively step by step, offering insights and best practices for a seamless and efficient development process. How To Build a Robust Data Pipeline Building a data pipeline is a multi-step process that requires careful planning and execution. Here are eight steps to build a data pipeline: Step 1: Define Your Goals The first step in building a data pipeline is to clearly outline your objectives. These could be to enable real-time analytics, facilitate machine learning models, or ensure data synchronization across systems. Your goals will guide the design, complexity, and scalability of your pipeline. Consider the specific data requirements, the frequency of data updates, and the desired speed of data processing and analysis. Step 2: Identify Data Sources Recognizing your data sources is a crucial step in building a data pipeline. Data sources can be broadly divided into six categories: Databases: Databases could be relational like MySQL, PostgreSQL, or non-relational like MongoDB, Cassandra. Cloud Storage: Data can also be stored in cloud platforms like AWS S3, Google Cloud Storage, or Azure Blob Storage. Real-time Data Streams: These are continuous data sources like IoT devices, social media feeds, or log files. File Sources: Data may originate from files, including formats such as CSV, Excel, JSON, or other file-based storage systems. APIs (Application Programming Interfaces): Data can be extracted from APIs that provide a direct connection to various web services and external platforms.” Understanding the nature, format, and volume of these data sources is essential as it impacts the subsequent stages of ingestion and transformation. Step 3: Determine the Data Ingestion Strategy Next, you need to extract, integrate and incorporate data from diverse sources into your pipeline. There are two primary methods of data ingestion: Batch Ingestion: This method involves collecting data over a specific period and processing it as a group. It’s suitable for large volumes of static data where real-time insights are not required. Real-time Ingestion: In this method, data is processed immediately as it arrives. It’s ideal for scenarios where real-time insights are crucial, such as fraud detection or system monitoring. Step 4: Design the Data Processing Plan You’ll need to clean, validate, and structure the ingested data into a format suitable for analysis. There are two main approaches to data transformation: ETL (Extract, Transform, Load): Here, you extract data from the source onto a staging server, transform it, and then load it into the destination. This approach is typically used when dealing with smaller volumes of data or when the transformed data needs to be used by multiple downstream applications. ELT (Extract, Load, Transform): In this approach, you extract data from the source, load it into the destination, and then transform it within the destination server. This eliminates the need to load data on a staging server, making it preferable for handling larger volumes of data in a big data environment. The data transformation process in ELT is similar to ETL but occurs after the data has been loaded into the destination. Step 5: Decide Where to Store the Information Next, you’ll store the processed data for further use. This could be a traditional data warehouse like Amazon Redshift for structured data, a data lake like Google Cloud Storage for semi-structured or unstructured data, or a database system like PostgreSQL for transactional data. The choice of destination depends on the nature of the data, the use case, and the specific data analytics requirements. Step 6: Establish the Workflow At this stage, it’s important to define the sequence of operations in the pipeline. This includes specifying the order of tasks, managing dependencies between tasks, handling errors, and setting up retries or notifications in case of failure. This step is crucial to ensure the smooth operation of the pipeline and to handle any issues that might arise promptly. It involves creating data validation checks and defining procedures for error handling and recovery. Step 7: Set a Monitoring Framework It’s crucial to monitor data pipeline performance to ensure it’s working as expected. This involves tracking the flow of data through the pipeline, checking for errors or bottlenecks that might slow down data processing, and monitoring resource usage to ensure the pipeline is operating efficiently. Monitoring helps detect and resolve issues before they affect the performance of the pipeline or data quality. Step 8: Implement Data Consumption Layer Finally, you’ll need to create a reliable interface through which end-users or applications access the processed data. This could be a business intelligence (BI) tool that provides data visualization capabilities, a reporting tool that generates detailed reports, or an API that allows other applications to interact with the data. The data consumption layer needs to be designed to easy access to the data. Best Practices for Building Data Pipelines Streamline Development and Deployment: Implementing Continuous Integration/Continuous Deployment (CI/CD) is key here. CI/CD automates the process of integrating code changes and deploying those changes to the production environment. This means that any updates or improvements to the pipeline can be implemented rapidly and reliably, ensuring the pipeline is always operating at its best. Maintain Consistency: Version control systems help achieve consistency in collaborative development environments. They track and manage changes to the codebase, enabling simultaneous work without overwriting changes and providing the ability to revert to previous code versions when necessary. Optimize Data Management: Data partitioning divides a large database into smaller, more manageable segments based on specific criteria, improving query performance and streamlining data management tasks. Leverage Modern Architectures: Modern software architectures like containers and microservices can significantly improve the scalability and reliability of your data pipeline. Containers encapsulate an application and its dependencies into a single unit that can run consistently across platforms. Microservices create an application as a collection of independent services, promoting better scalability and easier debugging. Ensure Data Security: As data moves through various stages of the pipeline, from extraction and transformation to loading, it’s crucial to protect it from unauthorized access and potential breaches. This involves implementing data encryption, access controls, and regular vulnerability testing to protect sensitive information. Compliance with relevant data safety regulations, such as GDPR and HIPAA, is essential, particularly in sectors dealing with personal data, such as healthcare and finance. Shifting from Manual to Automated Data Pipelines Building data pipelines manually (Python for data pipelines) is inherently a time-consuming and resource-intensive process, making it susceptible to delays, errors, and inefficiencies. The transition to automated data pipeline tools addresses these challenges, streamlining processes for greater efficiency and resource optimization. These advanced tools play a pivotal role in streamlining the entire process, offering advantages such as reduced development time, minimized errors, and enhanced scalability. By automating repetitive tasks, they allow organizations to expedite pipeline development while maintaining a high level of accuracy. Create Data Pipelines Easily with LIKE.TG LIKE.TG’s Data Pipeline Builder brings all of these automation capabilities together. It simplifies data integration, offers scalability, and comes equipped with features to maintain data security and compliance. With its user-friendly interface and powerful features, LIKE.TG’s solution reduces the effort and time needed to build a robust data pipeline, allowing businesses to focus more on leveraging their data for insights and decision-making. Building a data pipeline with LIKE.TG takes only five steps: Data Extraction: LIKE.TG allows you to extract data from a wide range of sources. This includes various databases like SQL Server, Oracle, and MySQL, file formats such as Excel, CSV, XML, and JSON, and cloud applications like Salesforce and Microsoft Dynamics 365, ensuring a comprehensive data collection process. Data Transformation: Once the data is extracted, LIKE.TG provides a variety of transformation functions that you can use to clean, format, and structure your data as per your requirements. For example, you can use these functions to remove duplicates, convert data types, or aggregate data based on specific criteria. Data Loading: After the data is transformed, LIKE.TG enables you to load it into your desired destination, be it a database, a data warehouse, or a file system. You could load the data into an SQL Server database, a cloud-based data warehouse like Amazon Redshift, or even export it as a CSV file. Automation and Scheduling: LIKE.TG also provides features for automating and scheduling your data pipeline tasks. This means that once you’ve set up your pipeline, you can have it run automatically at scheduled intervals or triggered by certain events, reducing manual effort and ensuring your data is always up-to-date. Monitoring and Error Handling: LIKE.TG provides real-time monitoring capabilities, allowing you to track the performance of your data pipeline and quickly identify and resolve any issues. Upgrade from manual to automated data pipelines today! Download LIKE.TG Data Pipeline Builder’s 14-day free trial and transform your data workflows. Build Automated Data Pipelines With Just a Few Clicks! With LIKE.TG's robust features and automation capabilities, you can effortlessly build and deploy data pipelines, saving time and enhancing efficiency. Download Free Trial
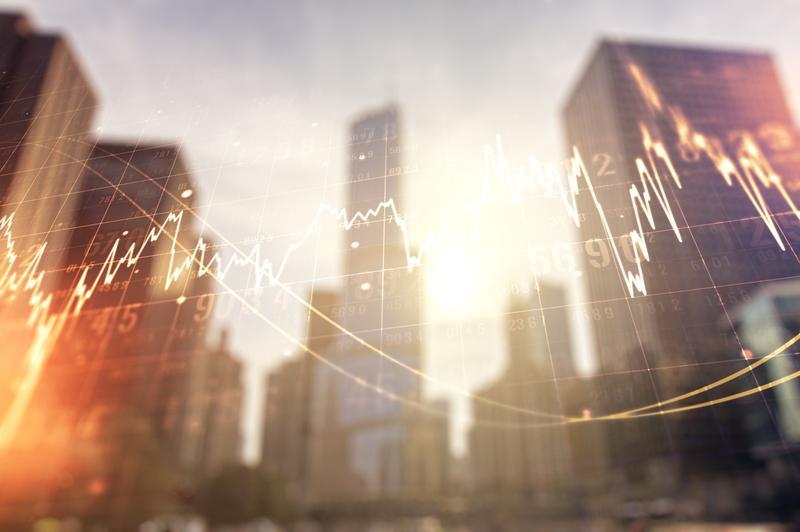
The Role of Data in Personalized Banking
With over 70% of customers emphasizing the importance of personalized offers in banking, it’s evident that people highly value tailored experiences from their financial institutions. However, despite this strong customer preference, only 14% of banks have embraced personalized banking. This reveals a significant gap between customer expectations and the services currently provided by the finance industry. Bridging this gap requires leveraging the role of data. Personalized banking experiences rely on utilizing customer information and insights derived from data. These insights are crucial in shaping services that align more precisely with individual preferences and needs. What is Personalized Banking? Personalized banking focuses on delivering unique experiences to customers based on their financial needs and preferences outlined by behaviors. This personalized approach goes beyond the one-size-fits-all approach of traditional banking services. Banks collect vast amounts of customer data, ranging from transaction history to online browsing behavior. By leveraging this data for analytics, banks can gain valuable insights into consumer behavior and provide customized recommendations and offers. Modern banks recognize the importance of personalization in every aspect of their operations—be it customer acquisition, service, onboarding, or overall engagement. Benefits of Personalized Banking Customer Satisfaction and Loyalty: Personalization enhances customer satisfaction by addressing specific financial needs, making customers feel valued and understood. This tailored approach fosters a deeper connection with the bank, leading to increased loyalty and retention rates. Banks can also offer rewards programs where customers receive discounts, cashback, or exclusive deals based on their spending patterns. Tailored Financial Advice: Banks can provide individualized financial guidance, such as personalized investment strategies based on a customer’s income, risk apetite, and goals. This not only improves customer satisfaction but also increases the likelihood of achieving financial goals. Increased Revenue Opportunities: Through data analysis, banks can identify additional services or products that align with customers’ needs, leading to cross-selling and upselling opportunities. This targeted approach benefits both customers and the bank by expanding product offerings and revenue streams. Financial Literacy and Education: Personalized banking includes providing dedicated educational materials to improve customers’ understanding of complex financial concepts. Educating customers contributes to their financial well-being and strengthens long-term relationships. Source: info.blend.com How are Banks Using Personalized Banking? Targeted Product Recommendations Banks analyze customer spending patterns and offer personalized recommendations for credit cards, loans, or investment products that align with the customer’s financial goals. For instance, imagine a customer who frequently shops at grocery stores and spends a significant portion of their income on groceries. The bank would analyze this customer’s transaction history and identify their spending patterns. Based on this information, they might recommend a credit card that offers cashback or rewards specifically for grocery purchases. This personalized recommendation not only helps the customer maximize their savings, but also strengthens their relationship with the bank. Customized Marketing Campaigns Banks personalize their marketing efforts by sending targeted offers based on customers’ transaction history and preferences. For example, a customer who frequently travels may receive offers for travel insurance or foreign currency services. Consider a customer who frequently uses their credit card to book flights and hotels. A bank utilizing personalized banking would analyze this customer’s transaction history and identify their travel-related expenses. Armed with this knowledge, the bank could tailor their marketing campaigns to offer the customer exclusive travel benefits, such as discounted travel insurance or preferential foreign currency exchange rates. By providing personalized offers that align with the customer’s interests, the bank enhances the customer’s experience and increases the likelihood of them engaging with the bank’s services. Personalized Customer Service Banks use customer data to provide personalized customer service experiences. For instance, a customer calling the bank’s helpline will be routed to a representative who specializes in their specific financial needs. Imagine a customer who recently started a small business and needs guidance on managing their business finances. With personalized banking, when this customer calls the bank’s helpline, their call is directed to a representative who specializes in assisting small business owners. This representative would have a deep understanding of the challenges and opportunities faced by small businesses, allowing them to provide tailored advice and support to the customer. Overcoming Challenges in Data-Driven Personalized Banking While data-driven banking offers numerous benefits, it also poses challenges. Banks need to address these roadblocks effectively to ensure their strategies are successfully impelemented. Legacy Infrastructure: Older data processing systems often struggle with: Utilizing Unstructured Data: Inability to process and derive insights from the rapidly growing unstructured and alternative data sets. Open Data Sharing: Lack of capabilities for open data sharing, hindering collaboration and integration with external sources. Financial institutes should invest in modernizing their infrastructure to handle unstructured data efficiently. Upgrading data integration systems and adopting advanced analytics tools can aid in this process. Additionally, fostering partnerships and embracing open APIs can facilitate seamless integration with external sources. Strict Data Regulations: Increasing concerns about data breaches have led to: Consumer Caution: Heightened consumer wariness in sharing personal data due to high-profile instances of data theft and breaches. Regulatory Compliance Burden: Stricter regulations necessitate more comprehensive measures to safeguard customer information, adding complexity and cost to operations. Implementing robust data protection measures such as advanced encryption technologies and multi-factor authentication is crucial. Banks should also regularly audit data access and invest in monitoring systems to ensure compliance. Transparent communication about data privacy policies is essential to build trust and reassure consumers about the security of their information. Access to Third-Party Data: Issues in accessing and leveraging external data arise due to: Disjointed Tools: Challenges in performing real-time analytics and democratizing financial decisions due to fragmented tools and systems. Banks can diversify their data sources by reducing dependency on single vendors or technologies. Integration of unified analytics platforms and adopting standardized tools across business units can streamline data access and enable real-time analytics for better decision-making. Data Silos: Internal challenges revolve around: Complex Workflows: Highly intricate processes hinder collaboration and smooth data flow. Disparate Technologies: Using multiple systems across various business units creates data silos, preventing cohesive insights and decision-making. Banks should focus on streamlining workflows by implementing centralized data management systems. Integration of technologies across departments and fostering a culture of data sharing and collaboration can break down silos, enabling better data flow and cohesive insights for decision-making. The Role of ETL in Personalized Banking ETL is a process of extracting data from different sources, transforming it into a standard format, and then loading it into a target system. In personalized banking, ETL plays a crucial role in enabling data-driven decision-making and delivering personalized experiences to customers. Extract The extraction phase involves gathering customer-centric data from numerous sources. This process includes pulling essential information from internal systems, such as transaction logs, customer databases, and account histories. External sources also play a vital role in enriching this data pool. These sources might include social media platforms, where individuals may engage with financial institutions or express preferences that can inform personalized services. Moreover, data from credit bureaus and other financial institutions can offer insights into credit histories, loan obligations, and other relevant financial behaviors, contributing significantly to the holistic view of a customer’s financial profile. This extracted data forms the foundation upon which various analytics and machine learning models operate. Transform During the cleansing process, data is carefully examined, and any inconsistencies, errors, or duplicates are identified and corrected. For example, if a customer’s name is misspelled in one source, the ETL process can be designed to ensure consistent customer information across all relevant datasets. This attention to detail is essential in personalized banking, as even small errors can lead to incorrect recommendations or decisions. is another critical step in the ETL process. It involves combining data from multiple sources. Integration can be time-consuming, especially when dealing with large volumes of data. However, ETL tools, such as LIKE.TG, have advanced significantly, making integration more efficient and seamless. During the transformation phase, companies can apply enrichment as an additional step. It involves enhancing the data with additional information to provide a more comprehensive view. For example, demographic data, such as age, gender, or location, can be added to customer records to enable better segmentation and targeting. Load Finally, the transformed data is loaded into a centralized data warehouse or data mart, where it can be further analyzed and utilized for personalized banking initiatives. This unified data enables banks to gain a 360-degree view of their customers, leading to meaningful insights and personalized recommendations. How Does LIKE.TG Help Financial Institutions with Personalized Banking? LIKE.TG empowers financial institutions to craft personalized banking experiences by offering a comprehensive suite of features, ensuring seamless integration, data security, compliance, and operational efficiency. Here’s how Asters cater to your needs: Intuitive Drag-and-Drop Interface: LIKE.TG streamlines operations with a no-code interface, allowing seamless data management to facilitate personalized banking solutions. Extensive Connectivity to Diverse Data Sources: Seamlessly integrate data from various sources to ensure a comprehensive view of your customer data. LIKE.TG facilitates connectivity, bridging gaps between disparate data sources for a unified perspective. Library of Pre-Built Transformations: Accelerate data processing with our pre-built transformations, effortlessly manipulating and enriching data for actionable insights. Flexible Data Pipeline Builder for ELT and ETL: Construct tailored data pipelines with an industry0grade ELT and ETL engine. LIKE.TG ensures efficient data processing from extraction to loading, meeting specific institutional needs. Simplified Data Mapping: Streamline complex data mapping processes for precision and accuracy, enabling efficient mapping of data elements. Efficient Workflow Automation: Increase operational efficiency with automated workflows, orchestrating processes seamlessly for a more personalized service. Real-Time Change Data Capture (CDC): Stay updated with LIKE.TG’s Change Data Capture feature, integrating real-time changes for swift responses and personalized services. Secure Managed File Transfer (MFT): Safeguard critical data with our Managed File Transfer capabilities, ensuring the secure flow of sensitive information within your data ecosystem. Robust Security Measures: Prioritize data security with encryption protocols, role-based access control, and publishing workflows deployed on-premise, ensuring utmost security for personalized banking data. Compliance Alignment (e.g., GDPR): LIKE.TG helps align with global data regulations such as GDPR, ensuring banking services are delivered ethically and responsibly. Ready to revolutionize your banking experience? Experience the power of personalized services with LIKE.TG. Sign up for a demo or a 14-day- free trial now to discover the future of finance!
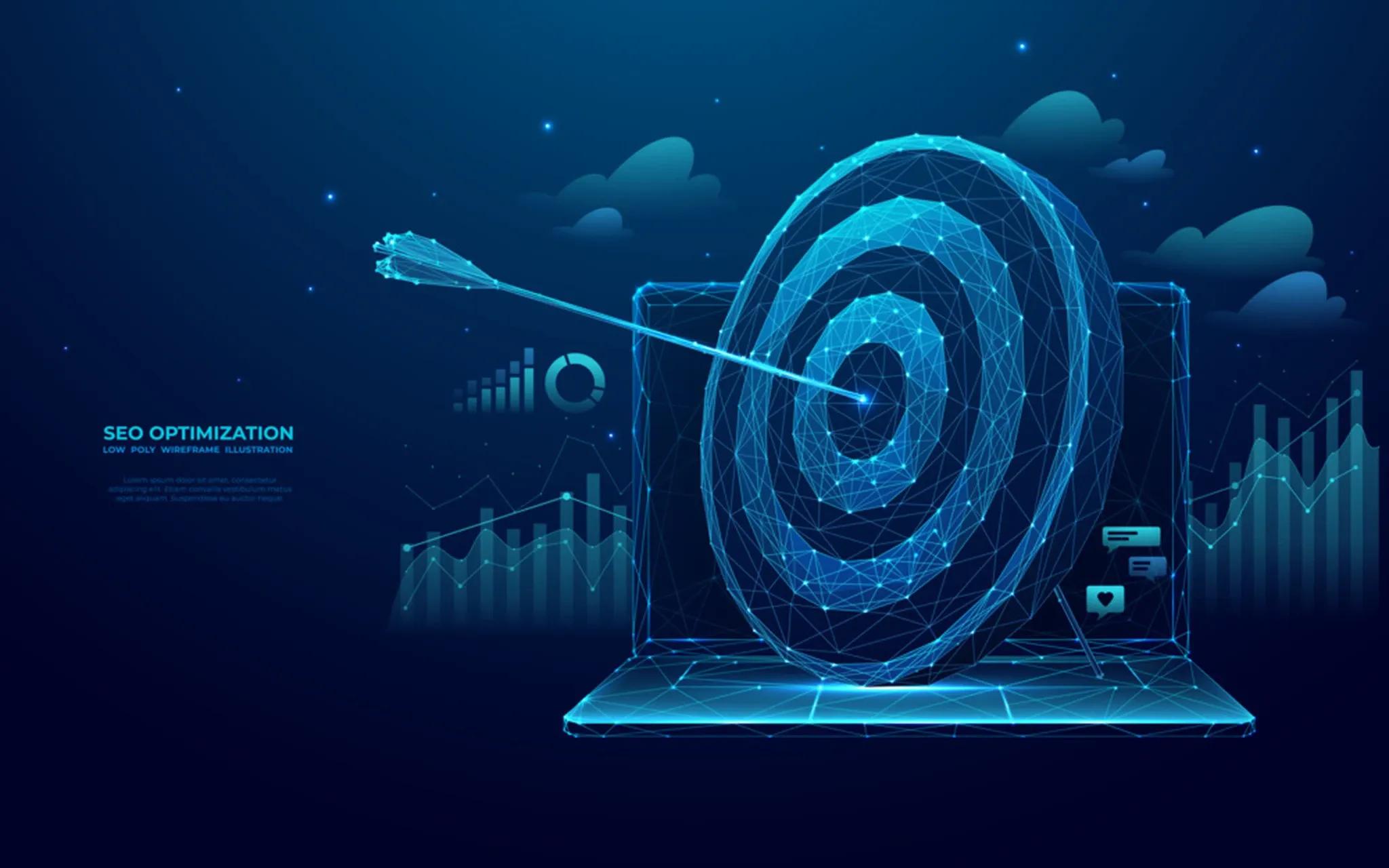
Data Profiling: Types, Techniques and Best Practices
Clean and accurate data is the foundation of organizations’ decision-making processes and is the reason why they’re heavily investing in data quality solutions. The global Data Quality Tools market was valued at $3.23 billion in 2023, and projections show that it’ll cross the $8 billion mark by 2030. Obtaining high-quality data is among the primary objectives of data preparation across different industries and sectors. This is where data profiling comes into play. It provides organizations with a general overview of all their data, which they can then use to detect errors and inconsistencies. These insights enable them to promptly rectify issues, make informed decisions, and enhance operational efficiency. Let’s dive into the specifics of data profiling and how it helps in data preparation. What is Data Profiling? Data profiling ensures that the data is in good health and fit for its intended use. It is essentially the first step in the process of managing and using data. Data profiling can uncover a range of data quality issues, such as missing data, duplication, and inaccuracies. It also highlights patterns, rules, and trends within the data. This information is crucial as it helps organizations improve data quality, streamline data transformation, and make informed decisions. Types of Data Profiling Data profiling can be classified into three primary types: Structure Discovery: This process focuses on identifying the organization and metadata of data, such as tables, columns, and data types. This certifies that the data is consistent and formatted properly. For instance, in a healthcare database, structure discovery reveals the presence of tables like “Patients” and “Appointments” with columns such as “PatientID,” “AppointmentDate,” and data types like “integer” and “date.” Content Discovery: This involves a deep dive into the actual content of the data. It examines individual data records to identify errors. For example, in a customer database, content discovery reveals that the “Phone Number” column contains numerous missing values, highlighting incomplete contact information for certain customers. Relationship Discovery: This process identifies the relationships and dependencies between different data elements. For instance, in a retail database, relationship discovery would analyze the associations between different fields and tables, such as the relationship between the ‘Customers’ table and the ‘Orders’ table, understanding how different data elements are interconnected and how they influence each other. Data Profiling Techniques Profiling data involves a variety of techniques that help analyze, assess, and understand data. Four primary techniques are: Column Profiling: This technique analyzes each column in a database. It looks at the type of data in the column, how long the data is, and if there are any empty values. A crucial part of this process is frequency analysis, which counts how often each value appears, helping to spot patterns and unusual values. Cross-Column Profiling: Here, the focus is on the relationships between different columns within the same table. It includes key and dependency analysis. Key analysis finds columns where each row has a unique value, while dependency analysis looks at how values in one column depend on values in another column. This can help find connections, overlaps, and inconsistencies between columns. Cross-Table Profiling: This method looks at relationships between different tables in a database. It includes foreign key analysis, which finds columns in one table that match up with unique key columns in another table. This helps show how data in one table is related to data in another table and can provide important information about the structure and accuracy of the database. Data Validation and Cleansing: This approach involves verifying the accuracy and quality of data against specific criteria or standards. It includes format checks, range checks, and consistency checks to ensure data is clean, correct, and logically consistent. Understanding the Difference: Data Profiling vs. Data Mining Data profiling and data mining are two distinct processes with different objectives and methodologies. Data profiling is the initial step in data preparation, focusing on understanding the data’s basic characteristics, quality, and structure. It helps identify data issues like missing values or anomalies. This helps ensure that data is clean and reliable for further use. In contrast, data mining involves exploring the data to discover hidden patterns, trends, and valuable insights using advanced techniques like machine learning. These techniques can help with different tasks including: Pattern recognition Classification and prediction Clustering Anomaly detection Association rule mining Feature selection and dimensionality reduction Text and image mining Model evaluation and optimization Data Profiling Benefits Data profiling offers a multitude of specific benefits that can significantly enhance an organization’s data management strategy. Here are some of the distinct advantages of data profiling: Informed Decision-Making: Data profiling provides a clear understanding of the available data, its quality, and its structure. This knowledge aids in making informed, data-driven decisions, thereby improving strategic planning and operational efficiency. Increased Operational Efficiency: It helps in identifying and eliminating redundant or irrelevant data. This leads to improved efficiency of data processing and analysis, resulting in faster insights, improved productivity, and a better bottom line. Risk Mitigation: Data profiling can help businesses identify potential risks and issues in their data, such as compliance violations or security threats. By addressing these issues proactively, businesses can mitigate risks and avoid costly penalties or damage to their reputation. Cost Savings: By improving data quality and efficiency, data profiling can lead to significant cost savings. Businesses can avoid the costs associated with poor-quality data, such as inaccurate decisions, wasted resources, and lost opportunities. Compliance Assurance: Data profiling can help businesses ensure compliance with industry regulations and standards. By addressing compliance issues, businesses can avoid legal complications and maintain their credibility in the market. Data Profiling Challenges Understanding the challenges and limitations of data profiling is key to ensuring data profiling methods’ effectiveness. Here are some of the data quality challenges and how to overcome them: Scalability When datasets grow and become increasingly complex over time, conventional data profiling techniques may prove insufficient for handling the growing data volume. Left unchecked, this can make data profiling tasks more resource-intensive and time-consuming — ultimately delaying data preparation and disrupting timely analysis. Organizations can address scalability issues by implementing parallel processing. Parallel processing techniques distribute profiling tasks across multiple nodes or processors. This measure improves efficiency and significantly reduces processing time for larger datasets. Resource Requirements Data profiling can require substantial computational resources such as memory, storage capacity, and processing power. Insufficient resources create performance bottlenecks and slow down profiling processes, impacting productivity and efficiency. Organizations can mitigate bottlenecks and boost productivity by optimizing resource allocation in the following ways: Investing in scalable infrastructure, such as cloud-based solutions, to ensure flexibility. Implementing dynamic resource allocation based on changing workload requirements. Complex Data Structures Modern data environments typically feature diverse data formats and structures. They also contain large amounts of semi-structured and unstructured data. Conventional data profiling techniques may not be as useful for analyzing such complex data structures, causing them to generate inaccurate or incomplete profiling results. Fortunately, enterprises can resolve this by incorporating advanced profiling techniques such as natural language processing and machine learning algorithms. These modern techniques can identify relationships and patterns within unstructured and semi-structured data and aid in obtaining more accurate profiling results. Data Privacy and Security Accessing and analyzing sensitive information — such as confidential business data and personally identifiable information — are standard components of data profiling. Maintaining data privacy and security throughout the profiling process is essential, as it helps defend data against data breaches, unauthorized access, and regulatory non-compliance. Organizations can resolve data privacy concerns by integrating data anonymization and masking techniques into their profiling methods. Doing so facilitates meaningful analysis while comprehensively protecting sensitive information. 5 Best Practices of Data Profiling When performing data profiling, organizations follow some best practices to ensure accurate results and efficient analysis: Define Clear Objectives: Clearly define the goals, objectives, and expectations to ensure it’s aligned with business needs and requirements. Choose Relevant Data Sources: Select relevant data sources based on their importance, relevance, and potential impact on decision-making processes. Establish Data Quality Metrics: Define appropriate metrics and validation rules to assess the quality and accuracy of data based on business requirements and industry standards. Document Data Profiling Results: Document and communicate the findings, recommendations, and actions taken during data profiling to facilitate understanding, accountability, and compliance. Regularly Monitor Data Quality: Implement regular data quality monitoring processes to ensure data consistency, accuracy, and compliance over time. Data Profiling Made Easier with LIKE.TG LIKE.TG's drag-and-drop, zero-code functionality simplifies the data profiling process, helping you quickly assess and understand your data. Start your free trial today! Start a Free Trial Applications of Data Profiling Data profiling finds applications in various areas and domains, including: Data Integration and Data Warehousing: Data profiling facilitates the integration of multiple datasets into a centralized data warehouse, ensuring data accuracy, consistency, and compatibility between sources. Data Migration and System Development: Before migrating data from one system to another or developing new software systems, data profiling helps identify potential data issues, detect data schema and structure, assess data distribution and patterns, and understand data dependencies and relationships. Data Analytics and Business Intelligence: By understanding the quality, structure, and relationships within data, data profiling empowers organizations to generate more accurate insights, make data-driven decisions, and enhance overall business intelligence. Data Profiling’s Role in Data Governance and Compliance Data profiling is vital in supporting organizational data governance and compliance initiatives. Data governance comprises all policies, processes, and controls that ensure data assets’ availability, integrity, and security. In contrast, compliance involves adherence to regulatory requirements and the industry standards governing data handling and usage. Here are five ways data profiling contributes to data governance and compliance: Data Quality Assessment: Data profiling serves as the first step in determining data assets’ quality. Analyzing the structure, content, and relationships within the data reveals any inconsistencies, inaccuracies, and anomalies that can potentially damage data integrity and impact compliance. Risk Identification and Mitigation Data profiling enables organizations to identify potential risk factors that can compromise data quality, privacy, and security. This can help them proactively deal with issues that can threaten compliance — such as regulatory violations, data breaches, or inaccurate reporting. Data Classification and Tagging Data profiling allows businesses to classify and tag data based on its regulatory requirements, sensitivity, and criticality. Understanding data attributes’ nature and context simplifies the application of relevant data classification policies and access controls.This helps organizations comply with privacy regulations such as the California Consumer Privacy Act (CCPA) and the General Data Protection Regulation (GDPR). Monitoring and Auditing Data profiling supports an organization’s ongoing monitoring and auditing protocols to maintain compliance with data governance policies and regulations. Creating baseline profiles of their data assets allows enterprises to observe data quality, integrity, and usage patterns consistently. It also helps them spot deviations that may warrant further investigation or corrective measures. Documentation and Reporting Data profiling results offer meaningful insights into the metadata of data assets by examining their structure and content. These insights are important for documentation and reporting initiatives. Organizations can utilize profiling reports to demonstrate their compliance with regulatory mandates, audit requirements, and internal governance policies. Conclusion As organizations continue to harness the power of data for competitive advantage, data profiling remains critical in ensuring data quality. By systematically examining and evaluating data, organizations can ensure data accuracy, reliability, and compliance, leading to more informed decision-making and better business outcomes. To ensure that high-quality data is being used for analysis, it’s crucial to invest in advanced data profiling tools. LIKE.TG stands out as a comprehensive solution that offers advanced data profiling, cleansing, and validation capabilities. It provides real-time health checks that continuously monitor your data quality as you work, providing immediate feedback on its overall health. LIKE.TG’s capabilities extend to both global and field-level data analysis, enabling early identification of irregularities, missing values, or anomalies. This proactive approach to data quality allows for timely measures to be taken to rectify any issues. LIKE.TG’s drag-and-drop visual interface empowers business users to examine and evaluate the data, facilitating necessary adjustments as needed. Therefore, LIKE.TG simplifies the data profiling process and enhances data accuracy, reliability, and overall quality, enabling improved operational efficiency and better business outcomes. Want to learn more about data profiling and how LIKE.TG streamlines the entire data prep process? Download your free whitepaper now!
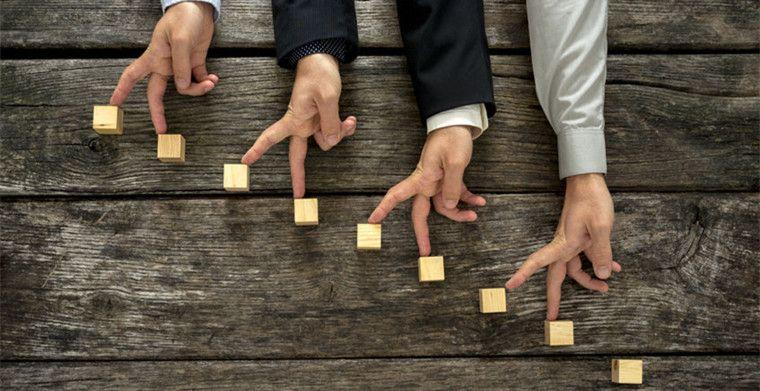
API-Led Connectivity: A Complete Guide
Today, APIs are everywhere —so much so that the global API market is set to reach USD 6263.00 million by 2028. Among the usage of APIs, API-led connectivity is a new approach, revolutionizing how systems, applications, and data interact. This disruptive technology has emerged as the backbone of seamless connectivity by playing a significant role in retrieving information from diverse sources. What is API-led Connectivity? API-led connectivity is an approach to connecting and integrating different applications, systems, and data sources using APIs as the primary building blocks. This approach organizes the development of integrations and connections between various systems based on reusable and manageable APIs. It involves creating and exposing APIs that encapsulate specific functionalities or data sets, allowing different systems to interact and share information in a secure and controlled manner. Why is API-led Connectivity Important? API-led connectivity revolutionizes digital interactions. They empower businesses to maximize the potential of their existing applications and systems. By seamlessly integrating various components through APIs, organizations can overcome traditional data silos. This fosters a more interconnected data ecosystem. Through this approach, businesses unlock numerous benefits. Firstly, they can enhance operational efficiency by breaking complex systems down into manageable parts. This not only streamlines processes but also facilitates easier integration, enabling a more agile and innovative environment. Furthermore, by promoting the flow of information across previously isolated departments or systems, valuable insights are revealed, enabling informed decision-making. Security is paramount in this interconnected landscape. APIs serve as gateways that ensure controlled access to information, safeguarding sensitive data. Ultimately, API-led connectivity doesn’t just improve internal operations. It transforms customer experiences. By leveraging APIs to optimize services, businesses create seamless digital ecosystems that deliver enhanced experiences to customers. API-Led Connectivity Architecture API-led connectivity follows a well-defined architectural approach that consists of three key components: System APIs System APIs serve as a standardized interface, exposing the functionalities of back-end systems or applications to facilitate seamless interaction with various front-end applications. They act as a bridge, enabling easy access and communication between different services ensuring that resources are readily available for utilization across diverse applications. Here are a few examples of System APIs: Operating System APIs: These APIs allow applications to interact with the operating system. For instance, Windows API or POSIX (Portable Operating System Interface) for Unix-like systems. Database APIs: Such as JDBC (Java Database Connectivity) or ODBC (Open Database Connectivity) that enable applications to communicate and interact with databases like MySQL, PostgreSQL, or Oracle. Cloud Service APIs: For example, Amazon Web Services (AWS) API, Google Cloud API, or Microsoft Azure API allow developers to access and manage cloud services like storage, computing resources, and machine learning tools. Messaging APIs: Services like Twilio offer APIs for sending and receiving SMS, MMS, and other communication services programmatically. Process APIs Process APIs simplify complex integrations by orchestrating and composing multiple system APIs. These APIs act as a higher-level abstraction layer. They simplify complex integrations by providing a unified interface to fulfill specific business processes or use cases. Process APIs ensure that the different systems and applications work together harmoniously by orchestrating the sequence and flow of data between them. The result? Streamlined business operations and improved efficiency. Here are a few examples: Order Processing: Consider an e-commerce platform that involves multiple steps—like inventory check, payment processing, shipping, and notification. A process API can orchestrate communication between inventory management APIs, payment gateways, shipping APIs, and notification services to ensure a smooth order processing flow. Customer Onboarding: Banks or financial institutions may have a complex onboarding process involving identity verification, credit checks, account creation, and notifications. A process API can orchestrate interactions between various APIs handling these tasks, ensuring compliance and a seamless experience for the customer. Travel Booking: Travel agencies often integrate with airlines, hotels, car rental services, and payment gateways. A process API can coordinate communication between these different APIs to manage flight bookings, hotel reservations, car rentals, and payment processing for a comprehensive travel booking system. Experience APIs Experience APIs focus on delivering seamless and personalized experiences to end-users, abstracting the underlying complexities of the integrated systems. They aggregate diverse data sources by acting as a unified interface, transforming this information into formats tailored for specific channels and devices. This ensures that end-users encounter consistent, optimized experiences across various touchpoints. Some examples nclude: E-commerce Platforms: Companies like Amazon or eBay leverage Experience APIs to unify product information, user preferences, and purchase history from diverse sources. These APIs ensure a seamless shopping experience across devices, recommending personalized products and streamlining the checkout process. Hospitality and Travel: Booking platforms such as Airbnb or Expedia use Experience APIs to aggregate data from multiple providers, including accommodations, transportation, and activities. This integration allows users to seamlessly plan and book entire travel experiences through a single interface. Streaming Services: Platforms like Netflix or Spotify utilize Experience APIs to collect user data, including viewing or listening history, preferences, and device information. They then leverage this data to provide personalized content recommendations, creating a cohesive and engaging user experience. How API-Led Connectivity Works The API-led connectivity approach follows a structured flow that ensures seamless integration and efficient data flow. It involves: Discovering and Creating APIs:Identify the systems and data sources that need to be integrated and define the APIs that will expose their functionalities. This involves understanding the existing landscape of applications and systems within an organization and determining the key functionalities that need to be exposed through APIs. Once the systems and data sources are identified, APIs are created to expose their functionalities, providing a standardized interface for accessing and interacting with their resources. Building and Implementing APIs:Develop and deploy the APIs, ensuring they adhere to industry standards and best practices for security, scalability, and reliability. This involves implementing the necessary logic and functionality to expose the desired functionalities of the back-end systems or applications. It is crucial to ensure that the APIs adhere to industry standards and best practices for security, scalability, and reliability. By following these standards and best practices, organizations can ensure that their APIs are secure, performant, and capable of handling the expected load. Orchestrating APIs:Use process APIs to orchestrate the sequence and flow of data between different systems and applications, ensuring they work together harmoniously. Process APIs enable the seamless flow of data between different systems and applications by defining the sequence and logic of how the APIs should interact with each other. By orchestrating the APIs, organizations can ensure that the data flows smoothly and that the systems and applications work together harmoniously to fulfill specific business processes or use cases. Managing and Monitoring APIs:Implement a robust API management platform to manage, secure, and monitor the APIs throughout their lifecycle. Organizations that implement a robust API management platform can better manage, secure, and monitor the APIs throughout their lifecycle. These solutions include versioning and lifecycle management, security and access control, rate limiting and throttling, analytics and monitoring, and developer portal management. By effectively managing and monitoring the APIs, organizations can ensure their availability, performance, and security, enabling them to deliver a seamless and reliable user experience. Benefits of Implementing API-Led Connectivity API-led connectivity offers several advantages, making it important in modern software development and integration practices: Maximizing Existing Investments: It allows organizations to connect and integrate existing applications and systems without replacing them entirely. This maximizes the value of these assets, saving time, resources, and minimizing disruptions to ongoing processes. Data-Driven Decision-Making: Utilizing APIs to integrate diverse data sources enables businesses to gain a comprehensive overview of operations and customer behaviors. This integrated approach not only facilitates informed decision-making for growth and competitive advantages but also ensures a seamless and consistent customer experience across various platforms Security and Control: APIs provide a structured way to manage data access, allowing for fine-grained control over who accesses what data and under what conditions, thus enhancing security measures. Modularity and Reusability: API-led connectivity encourages a modular approach where specific functionalities of a system or application are exposed through APIs. This modularity allows for easier reuse of these APIs across various projects and applications, fostering efficiency and consistency. Vendor Neutrality: It reduces dependency on specific technologies or vendors. APIs provide a standardized way to communicate between systems, making it easier to swap out components or technologies without major disruptions. Overcoming Challenges in API-Led Connectivity As API-led connectivity becomes increasingly prevalent, organizations face various challenges that need to be addressed for successful implementation and operation. Security Concerns One of the primary challenges is ensuring the security of the APIs. As APIs become involved in integrations, they handle sensitive data and provide access to critical systems. Therefore, implementing robust security measures is of paramount importance. Implementing strong authentication mechanisms enables organizations to verify the identity of users and systems accessing the APIs. This can involve using techniques such as API keys, OAuth, or even biometric authentication. For example, banking apps often employ biometric authentication (fingerprint or facial recognition) for accessing financial APIs. This technology verifies the user’s identity before allowing access to account details or transactions. Another crucial aspect of API security is encryption. Organizations can prevent unauthorized access and protect sensitive information by ensuring they transmit sensitive data through encrypted APIs. This can be achieved by implementing secure communication protocols such as HTTPS and using encryption algorithms like AES and DES to encrypt the data at rest. Integration Issues Integrating different systems with varying protocols, data formats, and standards can pose significant challenges. Organizations often have a mix of legacy systems, modern applications, and third-party services that need to be seamlessly integrated to enable efficient data flow. To address integration issues, organizations need to carefully plan and design their integration architecture. This involves analyzing the systems and applications to be integrated, understanding their data requirements, and identifying any potential conflicts or compatibility issues. Organizations can adopt an API-led approach to integration, where APIs act as the building blocks for connecting different systems. By designing APIs that abstract the underlying complexity of systems and provide a standardized interface, organizations can simplify the integration process and ensure seamless data flow. Furthermore, organizations can leverage integration platforms and tools that provide pre-built connectors and adapters for popular systems and applications. These tools can help streamline the integration process by providing out-of-the-box support for different protocols, data formats, and standards. How LIKE.TG Enables API-led Connectivity The future of API-led connectivity holds tremendous potential. As businesses continue to embrace digital transformation, API-led connectivity will play a crucial role in enabling seamless integration and data flow. With emerging trends such as event-driven architecture, API marketplaces, and API monetization, businesses are finding innovative ways to leverage APIs for their growth and success. As integration capabilities evolve, API-led connectivity will remain a crucial enabler for businesses seeking to stay ahead in a rapidly changing world. Enter LIKE.TG. LIKE.TG is a no-code platform for developing, testing, deploying, governing, and overseeing APIs, enabling organizations to expedite API creation and management. Crafted with an emphasis on user-friendliness, efficiency, and automation, LIKE.TG API Management reduces API development and management time by up to 80%. Integrating LIKE.TG into your API strategy can enhance your ability to adapt to evolving business requirements, refine dataflows and workflows through API-led connectivity, and ensure a seamless user experience for your customers. Want to experience the benefits of no-code API management? Sign up for LIKE.TG API Management’s14-day free trialor schedule ademowith our experts today!
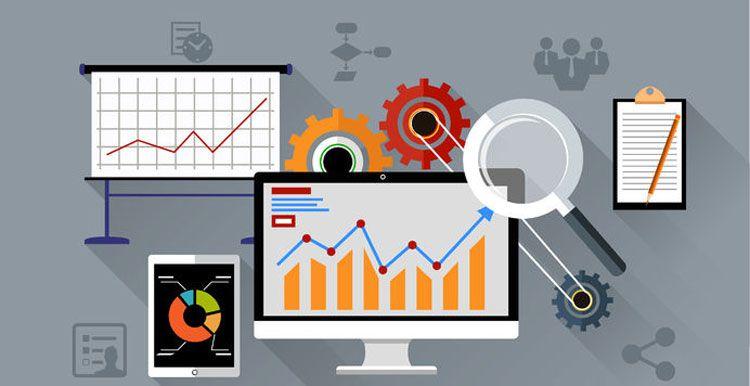
CDC for ETL Process Optimization in Finance Industry
Every day, customers produce an immense volume of data through hundreds of thousands, if not millions, of individual transactions. From managing customer transactions and financial records to dealing with regulatory requirements and risk management, data plays a crucial role in every aspect of banking operations. This data is categorized as big data, a term denoting “large, diverse sets of information that grow at ever-increasing rates.” To put this into perspective, a staggering 2.5 quintillion bytes of data is generated daily. Banks rely on Extract, Transform, Load (ETL) processes to make sense of data and extract valuable insights. These processes are critical for banks to manage and utilize their vast amounts of data effectively. However, as data volumes continue to grow and the need for real-time insights increases, banks are pushed to embrace more agile data management strategies. Change data capture (CDC) emerges as a pivotal solution that enables real-time data synchronization and analysis. Understanding ETL Processes in Banking ETL refers to the three fundamental steps in data integration. First, data is extracted from various sources, including databases, applications, and external systems. This extraction process involves identifying relevant data points and retrieving them in a structured manner. For example, customer transaction data may be extracted from a database using SQL queries, while regulatory data may be obtained from external systems through APIs. Next, the extracted data is transformed into a standardized format and cleansed of any inconsistencies or errors. This transformation phase involves applying various data manipulation techniques, such as data validation, cleansing, and enrichment. For instance, if the extracted data contains missing values or outliers, these issues are addressed during the transformation process to ensure data accuracy. Finally, the transformed data is loaded into a target system or data warehouse for reporting and analysis. The loading phase involves storing the data in a structure that facilitates easy retrieval and analysis. For example, by loading the transformed data into a data warehouse, banks can perform in-depth analysis, generate reports, and gain valuable insights. Key Challenges in Current ETL Processes Growing volume and velocity of data: With the advent of digital banking, mobile payments, and other technological advancements, banks are generating data at an unprecedented rate. This exponential data growth has created significant demands on traditional ETL processes, which struggle to keep up with the speed and scale required for real-time insights. Complexity of data sources and formats: Banks have to deal with structured and unstructured data from various sources, such as transactional databases, log files, social media feeds, and more. Each data source may have its own data format and schema, requiring careful mapping and transformation during the ETL process. Need for near real-time data integration: Traditional ETL processes typically operate on a batch basis, meaning data is extracted, transformed, and loaded in predefined intervals (e.g., daily or weekly). However, in an era where timely insights can make a significant difference, banks are looking for ways to reduce the latency in their ETL processes. Introduction to Change Data Capture (CDC) To address the challenges faced by banks in optimizing their ETL processes, CDC has emerged as a valuable tool. What is Change Data Capture? CDC captures the changes (inserts, updates, deletes) made to data at the source system level as events. These events are then propagated to the target system, where they are applied, to keep the data in sync between the two systems. Imagine a scenario where a customer updates their contact information in the bank’s online portal. Without CDC, the traditional ETL process would extract the entire customer dataset, transform it, and load it into the target system. However, with CDC, only the specific change made by the customer is captured and propagated to the target system. This targeted approach not only saves time and resources but also ensures that the data remains consistent across systems. Furthermore, CDC provides a granular view of the changes made to the data. Each change is captured as an event, which includes information such as the type of operation (insert, update, delete), the affected rows, and the timestamp of the change. This level of detail allows banks to have a comprehensive audit trail of data modifications. How CDC Works in Data Integration? CDC operates by leveraging the transaction logs or change logs present in source systems. By continuously monitoring these logs, CDC technology can identify and capture the changes as they occur. Moreover, CDC allows banks to choose between different synchronization methods, such as one-way replication or bi-directional synchronization, depending on their specific requirements. This flexibility empowers banks to tailor their data integration approach to suit their unique business needs. Enhancing ETL with CDC By incorporating CDC into their ETL processes, banks can enhance their data integration capabilities. Traditional ETL processes can be supplemented with CDC technology to capture and replicate real-time data changes. This enables banks to have a more accurate and up-to-date view of their data, leading to more meaningful insights and better decision-making. When CDC is integrated with ETL, the ETL process can be triggered by the captured data changes, ensuring that the target system is always synchronized with the source systems. This eliminates the need for periodic batch updates and reduces the latency in data integration. Furthermore, CDC can capture not only changed data but also the metadata associated with said changes. This additional information can be valuable for auditing, compliance, and data lineage purposes. Steps to Optimize ETL Processes Using CDC Optimizing ETL processes using CDC requires a systematic approach that considers the unique requirements and challenges of each individual bank. The following steps provide a general framework for banks to implement CDC in their ETL processes: Identifying Opportunities for Optimization The first step in optimizing ETL processes is to assess the current state and identify areas for improvement. Banks should conduct a thorough analysis of their existing ETL workflows, data sources, and integration requirements to pinpoint bottlenecks and inefficiencies. This assessment helps banks identify the specific areas where CDC can bring the most value. For example, banks may find that certain data sources produce a high volume of changes, making them ideal candidates for real-time replication using CDC. By focusing on these high-impact areas, banks can prioritize their optimization efforts and achieve maximum benefits. During this stage, banks should also consider the scalability and performance requirements of their ETL processes. CDC technology can address these challenges by enabling incremental updates instead of full data loads, reducing the overall processing time and resource consumption. Implementing CDC in ETL Processes Once optimization opportunities are identified, banks can proceed with implementing CDC in their ETL processes. This involves deploying CDC technology that is compatible with the bank’s data source and target systems. When selecting a CDC solution, banks should consider factors such as data source support, scalability, ease of integration, and real-time data replication capabilities. It is essential to choose CDC technology that aligns with the bank’s specific requirements and can seamlessly integrate into the existing ETL infrastructure. Additionally, banks need to establish data mapping and transformation rules to ensure that the captured changes are correctly applied to the target system. This step involves defining the mapping between the source and target data structures, handling data type conversions, and resolving any conflicts or inconsistencies. Properly configuring and setting up CDC technology is crucial for seamless data integration and synchronization. Banks should also test the CDC implementation to ensure that it meets the desired performance, reliability, and data quality requirements. Furthermore, banks should consider implementing data validation and reconciliation mechanisms to ensure the integrity and consistency of the replicated data. This involves comparing the source and target data sets to identify and resolve any discrepancies. Automated Tools for ETL and CDC in Banking Operations Adopting automated tools for ETL and CDC processes is instrumental in managing and processing vast volumes of data with precision and agility. These tools offer a sophisticated framework for extracting data from heterogeneous sources, transforming it to meet specific banking requirements, and seamlessly loading it into the target systems. Moreover, CDC capabilities ensure real-time modification tracking within critical datasets, enabling banks to promptly identify changes in transactional records, customer profiles, or compliance standards. By leveraging automated tools for ETL and CDC, banks optimize operational efficiency, streamline data workflows, and maintain a competitive edge in an increasingly data-driven financial landscape. Centerprise can make building CDC-enabled ETL pipelines easy and fast! Powered by a no-code interface, Centerprise makes creating and automating ETL pipelines super simple, with increased time and cost-savings. Give it a try! Book FREE trial Using LIKE.TG to Easily Set Up An ETL Pipeline With CDC LIKE.TG’s user centric approach, code free environment, and intuitive UI allows it to empower business users in their data-driven endeavors. Here’s a breakdown LIKE.TG’s key features for CDC-enabled ETL: Diverse Connectivity: Supports a broad range of connectors for popular databases, data warehouses, and file formats, facilitating seamless integration into the ETL process. Unified CDC Management: Consolidates CDC oversight for relational databases within a single platform, eliminating the need for separate management, and ensuring comprehensive control across all relevant databases. Built-in Transformations: Offers built-in transformation capabilities, empowering users to cleanse and manipulate data effortlessly, enhancing data quality and accuracy within CDC-enabled pipelines. Data Profiling and Quality Assurance: Provides robust data profiling and quality features, facilitating checks to ensure accuracy and reliability, which is especially crucial for real-time or near-real-time data processing scenarios. Automation and Job Scheduling: Accelerates data transfer through automation and scheduling features, optimizing the efficiency of CDC-enabled ETL pipelines. This includes scheduling near real-time job runs. By incorporating scheduling options like ‘Continuous,’ LIKE.TG ensures timely updates and synchronization across data sources. Comprehensive Security Protocols: LIKE.TG prioritizes data security through robust measures such as bearer token authentication, granular user access control, customizable role-based management, and seamless integration with Windows Active Directory. In conclusion, leveraging CDC is a pivotal strategy for banks to streamline and optimize their ETL processes. By capturing and processing only altered data, CDC minimizes redundancy, enhances efficiency, and ensures real-time synchronization across systems. Transform your bank’s data management with LIKE.TG’s powerful CDC-enabled ETL workflows. Secure, streamline, and synchronize data effortlessly. Sign up for a demo or a 14-day- free trial now!
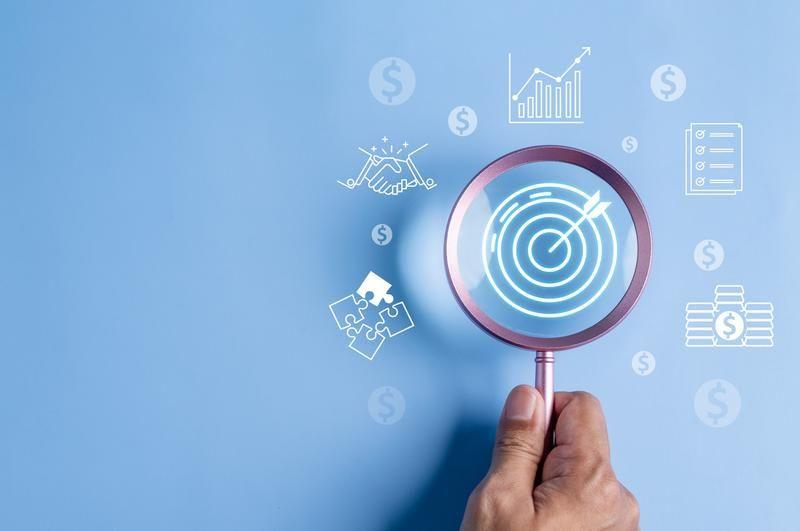
Data Vault 2.0: What You Need to Know
With rising data volumes, dynamic modeling requirements, and the need for improved operational efficiency, enterprises must equip themselves with smart solutions for efficient data management and analysis. This is where Data Vault 2.0 comes into play. It supersedes Data Vault 1.0, the original data modeling methodology specifically designed for data warehousing. With its foundation rooted in scalable hub-and-spoke architecture, Data Vault 1.0 provided a framework for traceable, auditable, and flexible data management in complex business environments. Building upon the strengths of its predecessor, Data Vault 2.0 elevates data warehouse automation by introducing enhanced scalability, agility, and adaptability. It’s designed to efficiently handle and process vast volumes of diverse data, providing a unified and organized view of information. With its ability to adapt to changing data types and offer real-time data processing capabilities, it empowers businesses to make timely, data-driven decisions. This is further substantiated by BARC’s study, which reveals that 45% of leaders have adopted Data Vault 2.0, primarily for its ability to expedite data delivery. What is Data Vault 2.0? Data Vault 2.0 is a modern data modeling methodology designed to provide a solid foundation for managing an organization’s data assets. It serves as a comprehensive framework that supports data integration, storage, and retrieval in a way that is highly adaptable, scalable, and conducive to business agility. This approach is particularly valuable in the era of big data, where organizations need to quickly adapt to changing business needs and incorporate diverse data sources. What’s New in Data Vault 2.0? In 2013, Dan Linstedt and Michael Olschimke introduced Data Vault 2.0 as a response to the evolving data management landscape, taking Data Vault 1.0 to new heights. While maintaining the hub-and-spoke structure of its predecessor, The upgrade introduces new, innovative concepts to enhance its efficiency and adaptability. Three key components of this system are the Business Vault, the Raw Vault, and the Information Mart and Data Mart layers. Raw Vault: In contrast to the Business Vault, the Raw Vault serves as the primary storage for original source data. It preserves the integrity of the data, ensuring that the original, unaltered data is always available for reference or further processing. Business Vault: This component of Data Vault 2.0 is a dedicated repository for business-specific rules and transformations. It optimizes data to meet precise business requirements, ensuring that the data is tailored to the specific needs and context of the business. Information Mart and Data Mart Layers: These layers in Data Vault 2.0 offer advanced analytical and reporting capabilities. They create a seamless connection between raw data and actionable insights, allowing businesses to easily transform their raw data into meaningful information that can drive decision-making. Understanding the Difference: Data Vault 1.0 vs Data Vault 2.0 The primary difference between the two lies in their implementation. Data Vault 2.0 adopts a groundbreaking approach by employing Hash Keys as surrogate keys for hubs, links, and satellites, effectively replacing the conventional sequence numbers. This substantially enhances data performance, scalability, and traceability. Additionally, Data Vault 2.0 introduces the concept of Business Keys, which are unique identifiers or natural keys that represent the core business entities within an organization. Data Vault 2.0 establishes comprehensive standards and guidelines for naming, modeling, loading, and documenting data. This ensures a foundation of quality, clarity, and manageability, making Data Vault 2.0 a comprehensive solution for modern data warehousing. Aspect Data Vault 1.0 Data Vault 2.0 Hash Keys Hash Keys weren’t a central concept, limiting data integrity and traceability. Prioritizes Hash Keys, ensuring data integrity and improving traceability for enhanced data security. Loading Procedures Loading procedures in Data Vault 1.0 could be complex, often involving sequence numbers, impacting efficiency. Simplifies loading procedures, enhancing efficiency and eliminating the need for complex sequence numbers. Dependencies Had significant dependencies, potentially slowing down data loading due to sequential processing. Reduces dependencies, enabling faster data processing through parallelization. Scalability Faced challenges with large datasets due to design limitations. Efficiently handles big data, making it suitable for complex datasets. Agility Less adaptable to changes in data sources and business requirements. Agile and responsive to changes, ideal for dynamic environments. Tech Agnosticism Limited flexibility with technology tools and platforms. More versatile, accommodating various technologies and platforms. The Data Vault 2.0 Advantage The need for Data Vault 2.0 stemmed from the changing dynamics of the data landscape. As organizations began to handle larger volumes of diverse data sources and adopted more agile development practices, it became evident that Data Vault 1.0, while initially effective, had certain limitations. The technology: Struggled to adapt to changing data types. Couldn’t handle vast volumes of data. Lacked real-time data processing capabilities. Didn’t align well with current technology or data governance requirements. 2.0 was developed to address the issues with the original Data Vault methodology and to better keep up with modern data management and analytics requirements. Here are some key reasons why Data Vault 2.0 was needed: Evolving Data Ecosystems: Data Vault 1.0 was developed in the early 2000s when data ecosystems were simpler and less complex compared to today. With the advent of big data, cloud computing, and advanced analytics, data architectures have become more intricate. Data Vault 2.0 was needed to better fit into these modern, complex data environments. Integration with Modern Technologies: Data Vault 1.0 was primarily designed for relational databases. Data Vault 2.0 extends its capabilities to work with big data platforms and NoSQL databases, allowing organizations to integrate a wider range of data sources. Managing Rising Data Volumes: Data Vault 2.0 offers better scalability and flexibility for handling large and diverse data volumes from various sources seamlessly. It leverages technologies like distributed computing and parallel processing to ensure efficiency. Industry Standards: Over time, industry standards and best practices for data management have evolved. Data Vault 2.0 incorporates these updated standards and aligns more closely with data governance and compliance requirements. Business-Centric Focus: Data Vault 2.0 focuses on making sure that data modeling and warehousing are closely aligned with what a company wants to achieve by introducing the concept of Business Data Vault. This helps IT and business teams work together better and ensures that data solutions really meet what the company needs. Benefits of Data Vault 2.0 Data Vault 2.0 offers three key advantages: scalability, adaptability, and auditability. Let’s explore these benefits in detail: Scalability Scalability is vital because data volumes are continually expanding, and organizations need systems that can handle this ever-growing demand. For example, consider a retail company that experiences a surge in online sales during the holiday season. Without a scalable data management system, the company may struggle to process and analyze the increased volume of transactional data efficiently. Data Vault 2.0 addresses scalability through various means: Separation of Concerns: It divides data into three layers (Raw Data, Business Data Vault, and Information Delivery Layer), allowing each layer to be scaled independently. This ensures resources can be allocated as needed, optimizing performance during high-demand periods like the holiday season. Parallelization: It encourages parallelization of data loading and processing tasks, distributing workloads across multiple servers or resources. This enhances efficiency, ensuring substantial datasets, like a surge in holiday sales, can be processed efficiently. Adaptibility Adapaptability is critical because business requirements, data sources, and data structures are subject to change. For instance, a healthcare organization may need to incorporate new types of patient data or adapt to evolving regulations. Data Vault 2.0 offers adaptability through several methods: Flexibility: Its inherent design is flexible and agile due to its modular structure of Hubs, Links, and Satellites. This allows for easy integration of new data sources, such as new patient data, without disrupting the existing structure. Future-Proofing: Its architecture is not tied to specific business rules or data sources, allowing the data architecture to evolve with changing business requirements or regulations, ensuring the healthcare organization remains compliant. Auditability Auditability is crucial for ensuring transparency, accountability, and compliance with data governance standards. Without it, organizations may struggle to track changes to data or maintain data quality. For example, in the financial sector, it is vital to track every change made to financial transaction data for regulatory compliance. Data Vault 2.0 enhances auditability through several ways: Traceability: It incorporates tracking mechanisms that capture metadata related to data sources, transformations, and data lineage. This detailed documentation ensures that every change made to financial transaction data can be traced back to its origin. Versioning: It allows for data versioning, enabling financial organizations to maintain historical records of data changes over time, which is invaluable for auditing and regulatory compliance purposes. Data Vault 2.0: A Glimpse Forward As we look towards the future, the question arises: Will Data Vault 2.0 continue to stay relevant in the face of technological advancements and the increasing volume and complexity of data? The answer is yes. Data Vault 2.0 is designed to handle the challenges of big data, integrating data from diverse sources, including social media, IoT devices, and traditional databases. Its ability to manage vast volumes of data, maintain data history, and ensure data consistency makes it ideal for large-scale businesses seeking to maintain long-term historical storage of data. To further highlight the relevance of Data Vault 2.0 in the future, let’s discuss some emerging trends in the data sphere and how this approach facilitates them: Machine Learning and Artificial Intelligence (AI): The integration of ML and AI in data management is revolutionizing the way businesses process and analyze data. Data Vault 2.0’s ability to handle high data volumes and support parallel processing ensures that data scientists and AI practitioners have access to clean, comprehensive data sets to train and refine their models. Its focus on data lineage and traceability aligns with the need for transparency and accountability in AI, especially in industries like healthcare and finance. Rise of Hybrid and Multi-Cloud Infrastructures: With businesses increasingly adopting hybrid and multi-cloud strategies, Data Vault 2.0’s compatibility with various cloud platforms and on-premises systems is a significant advantage. Its modular design allows seamless integration with diverse cloud platforms, facilitating efficient and secure data storage and processing. Self-Service Analytics: The trend toward self-service analytics is set to grow, empowering business users to explore and analyze data independently. Data Vault’s structured architecture provides a foundation for self-service analytics, allowing non-technical users to confidently navigate and query data. By fostering a data-driven culture and reducing reliance on IT for data provisioning, Data Vault accelerates decision-making and innovation within organizations. Data Vault 2.0: Utilizing Advanced Tools and Automation The implementation and maintenance of a Data Vault is an elaborate process, requiring a high level of expertise and significant time investment. However, employing advanced data warehouse tools tailored for Data Vault 2.0 can greatly simplify these processes. These tools offer the advantage of automation at every stage — from design and modeling to deployment and maintenance, significantly enhancing efficiency. Let’s delve into how these tools transform each stage of the Data Vault 2.0 process, making it more accessible and manageable for businesses. Design Stage At the design stage, advanced tools assist in creating the blueprint of the data vault. They enable the easy mapping of business concepts to data vault structures, leading to a well-structured and efficient design. The result is a time-efficient process that minimizes potential errors, ensuring a more accurate and effective design. Modeling Stage During the modeling stage, these tools automate the generation of data vault models based on the initial design. This includes the creation of hubs, links, and satellites, which are the core components of any data vault. The automation process significantly accelerates the modeling stage and guarantees consistency and accuracy in the models. Deployment Stage In the deployment stage, automated solutions streamline the process of populating the data vault with data from various sources. They automate the extraction, transformation, and loading (ETL) processes, ensuring a smooth and efficient data flow into the data vault. This accelerates the deployment process and mitigates the risk of data errors. Maintenance Stage During the maintenance stage, these solutions continue to provide value by automating routine tasks and checks. This ensures the data vault remains accurate, up-to-date, and efficient over time, reducing the manual effort required for maintenance and enhancing the overall longevity and reliability of the data vault Final Words Data Vault 2.0 emerges as a highly effective system for building agile and auditable data warehouses. Its unique data modeling methodology offers a holistic approach to designing, developing, and deploying data warehouses. As the demand for agile and auditable data warehouses grows, the role of specialized tools and automation in facilitating the effective implementation of Data Vault 2.0 becomes increasingly significant. This is where LIKE.TG comes into play. LIKE.TG is an enterprise-grade, end-to-end data management solution that enables enterprises to simplify the process of creating a Data Vault. With features that align perfectly with the principles of Data Vault 2.0, LIKE.TG provides businesses with a robust, scalable, and flexible data warehousing solution. It’s designed with user-friendliness and efficiency in mind, enabling businesses to automate the process of creating a Data Vault with just a few clicks. Elevate your data warehousing capabilities with LIKE.TG. Download our 14-day free trial today and see how easy it is to build a Data Vault.
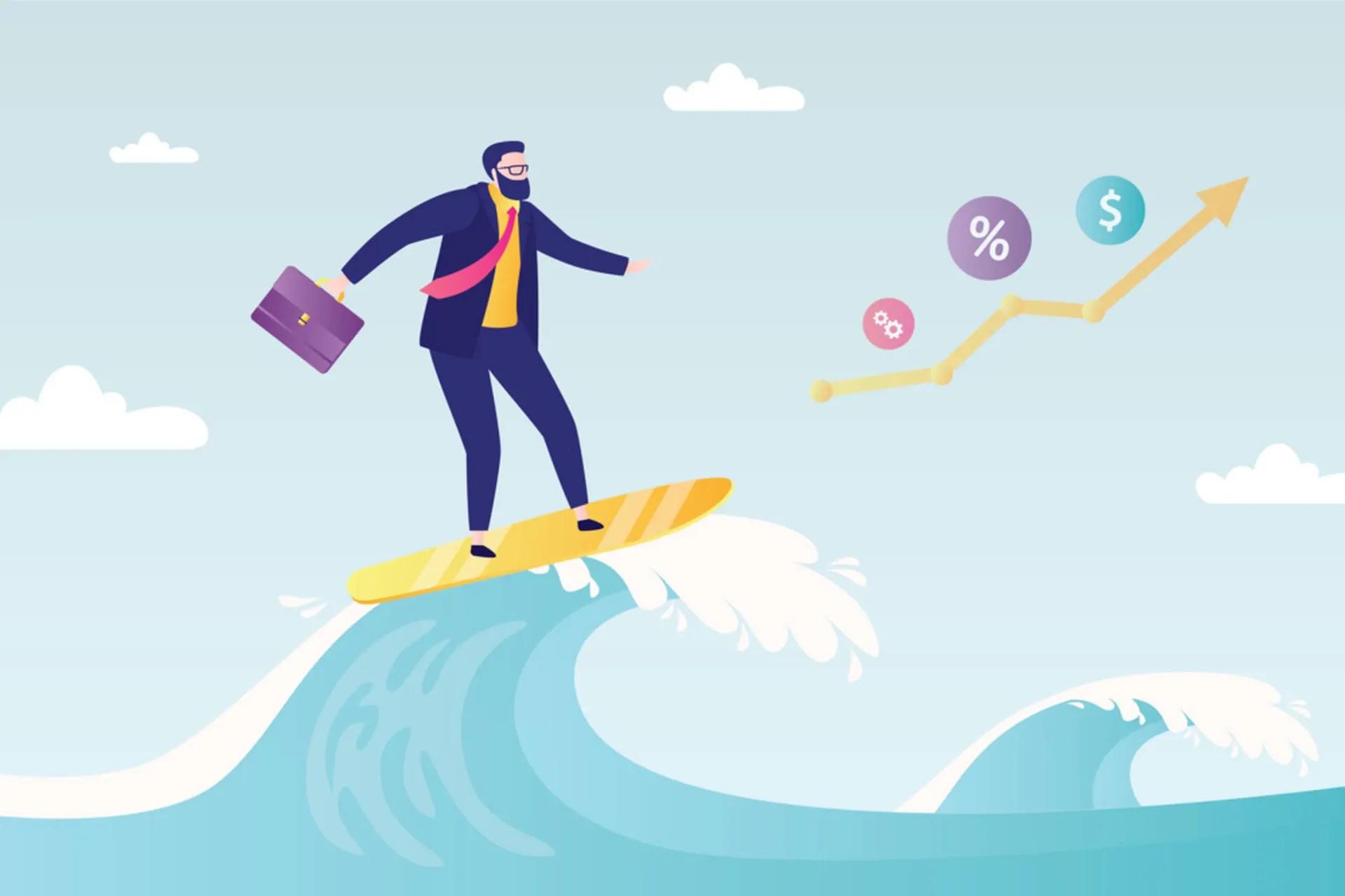
Fivetran vs. Matillion for ETL: A Detailed Comparison
As data becomes more complex and spreads across various sources and touchpoints, it is becoming increasingly difficult to combine it at the speed required by the business. Data teams usually use ETL tools to integrate data into a unified view. Thankfully, these ETL tools are also evolving along with the data needs. Today, there are a plethora of options available in the market, that choosing the right one can be a little tricky. Two established ETL tools in the market are Fivetran and Matillion. This blog will help users evaluate their pricing, features, integrations, support, and feasibility. Also, it will give you another alternative to both the solutions that you might find helpful. What is Fivetran? Fivetran is that was launched in 2012 out of Y Combinator. It took its inspiration from Fortran, a coding language developed in 1954 by John Backus. Fivetran operates as a single platform, offering data movement, transformation, and governance features. It’s fully managed on the cloud and allows users to set up and manage their data pipelines easily. The tool comes with a range of pre-built connectors to streamline data integration and operates on a usage-based pricing model. Customers pay monthly for each MAR (monthly active row) in use. What is Matillion? Matillion is also a cloud ETL tool. It was founded in 2011 in Manchester, UK. Matillion focuses on simplifying data integration and business intelligence. Matillion’s ETL offering focuses on robust transformations and connectivity. Not only do users have access to pre-built connectors, but they can also access community connectors and create their own. A distinguishing feature of Matillion is that it can run on major cloud platforms such as Amazon, Google Cloud Provider, and Microsoft Azure. It uses a credit model with three tiers: basic, advanced, and enterprise. Fivetran Vs. Matillion: Pricing Fivetran Fivetran charges users based on active rows, where each active row is counted once a month. There are 4 tiers: Free Starter Standard Enterprise It offers a free tier for low data volumes, which provides limited features for 500,000 MARs. Higher tiers allow unlimited users and API access, with users getting priority support, enterprise connectors, and advanced security in the Enterprise. Matillion Unlike Fivetran, Matillion uses progressive per-credit pricing with 3 tiers: Basic ($2/credit) Advanced ($2.5/credit) Enterprise ($2.7/credit) The credits consumed are dependent on vCore usage per hour. For every 1 hour of vCore use, 1 credit is used. The basic tier offers limited connectors, users, and security. Enterprise customers receive CDC pipelines, unlimited users, mission-critical support, auto-documentation, and more. No-code, End-to-end ETL/ELT With LIKE.TG Maximize your data's potential with LIKE.TG's no-code solution. Extract, prepare, transform, and load your data with ease with a flexible pricing structure - tailored to your specific needs. Download Free 14 Day Trial Fivetran Vs. Matillion: Services Fivetran Fivetran is purely an ELT product. For extraction, Fivetran has an extensive library of ready-made connectors. Users can find connectors based on connector type and features. In terms of replication, Fivetran supports SaaS, streaming, file, database, and SAP. It uses dbt Core for custom transformations alongside its native library. Major data warehouses, such as Redshift and Snowflake, support these models. To streamline efficiency, Fivetran automatically switches between full and incremental loads. It helps users automate the entire ETL process. It also provides auditing, schema drift handling, and incident handling. Matillion Matillion boasts a wide range of connectors to cloud sources and destinations. While the number of pre-built connectors is limited compared to Fivetran, the availability of community connectors and quick custom ones makes up for it. Users can build no/low-code pipelines based on experience and resources. The company supports various data integration initiatives: ETL/ELT Reverse ETL Data replication CDC Verdict: Both tools have robust ETL features. While Fivetran has more pre-built connectors, Matillion has community connectors, offering more flexibility in making custom options. Both tools provide a minimum code approach to building data pipelines. Fivetran Vs. Matillion: Support Fivetran Fivetran provides the usual SaaS support features: FAQs and support tickets. They also have a status page to provide a real-time service functionality check. Fivetran has a support rating of 7.9/10 on G2 and 4.4/5 on Gartner. Matillion Matillion has a dedicated support subdomain for submitting tickets. The subdomain also comes with example cases and a knowledge base. Users can also join a community for more information and tips. Matillion has a support rating of 8.8/10 on G2 and 4.3/10 on Gartner. LIKE.TG provides industry-leading support with a robust ETL and ELT platform. Try it for 14 days – totally free. Fivetran Vs. Matillion: Ease of Use Fivetran Fivetran has a user-friendly, drag-and-drop web interface that makes it easy to operate. It uses a no-code approach to ELT. The large number of connectors and cloud deployment make it easy to load and manage data. It also has role-based access, versioning, and other collaboration features. It has an 8.9/10 ease of use rating on G2. Matillion Matillion offers both no-code and low-code development. This makes it accessible to both developers and business users. Like other ETL tools, it also has a drag-and-drop GUI for creating mappings and pipelines. Mattilion also provides Git integration, version control, and role-based access for improved collaboration. Their strong documentation further helps teams use the product more effectively. It has a 9.3/10 ease of use rating on G2. Fivetran Vs. Matillion: Architecture Fivetran Hosted on AWS and Google Cloud, Fivetran is a fully managed SaaS focused on efficient Extract-Load processes. It fetches data from the user’s local environment, manages it on its cloud, and then pushes it to the customer’s cloud – keeping the 3 separate. Other core features of its design are: Incremental loading Idempotence Schema drift handling Matillion Matillion’s supports 3 architecture types: Single node: All components of Matillion run on a single VM. Single node RDS: All nodes are on a single virtual machine, but the database runs on a separate Redshift instance. Clustered: Multiple VMs are used for the nodes. Data is never pushed out of the cloud to an external server, keeping data sensitivity in mind. The focus is on carrying out transformations through the power of the cloud warehouse. Users can access the ETL client on Amazon EC2, Google Cloud, and Microsoft Azure. Fivetran Vs. Matillion: Deployment Fivetran While Fivetran mainly works fully managed on the cloud, it also offers self-deployment options. It can be deployed on-premises – users can host it on a VM or air-gapped. Hybrid deployment is also an option. This setup provides users with added flexibility. Small teams can choose the cloud option, while large corporations with strict security requirements can choose self-hosting. Matillion Users can deploy Matillion in 3 ways through the web interface: Using Environments (a target data warehouse) Using Multiple Projects (each project has one Environment) Using Multiple Instances (each Matillion VM is one Instance) There are also other deployment options through their REST API. Comparison Summary Fivetran Matillion Pricing Based on Monthly Active Rows – has 4 tiers Based on credits consumed – has 3 tiers Free Trial Yes – 14-day Yes Deployment On-cloud on-prem On-cloud on-prem, multiple instance Customer Support Support tickets, email, live status page, FAQs, documentation Subdomain for tickets, email, user community, knowledge base, Matillion Academy Customer Support Ratings 7.9/10 G2, 4.4/5 Gartner 8.8/10 G2, 4.3 Gartner Connectors 459, can ask for custom connectors 100+ pre-built, community versions and custom-building options are also available Custom API SQL coding required No SQL coding Interface No-code, drag-and-drop Low-code/no-code, drag-amd-drop Ease of Use Rating 8.9/10 G2 9.3/10 G2 Transformations Pre-built, custom transformations through dbt Core Pre-built, custom through SQL ide Connectivity All major cloud warehouses All major warehouses LIKE.TG – A Better Way to ETL LIKE.TG provides a simple yet powerful way to manage your data. It offers a no-code solution for creating enterprise-level ETL and ELT pipelines in a drag-and-drop interface. In short, it empowers both technical and non-technical teams to automate data management. LIKE.TG provides: A drag-and-drop interface for building ETL/ELT pipelines. Connectors for all major databases. OData connectivity. Automation. No-code, in-built transformations. Data quality rules. Change Data Capture. LIKE.TG is built-on industrial strength engine and can easily process large volumes of data. It has a Top Rated certification on Trustradius and multiple Fortune 500 clients. LIKE.TG is also an industry leader in User Support, boasting customer support excellence awards from leading publications like TrustRadius and G2. In terms of pricing, LIKE.TG has a flexible approach – users can choose and pay for the exact modules they need. So, no overpaying for features that are not required. Try out LIKE.TG for free with a 14-day trial, or get in touch with us for a free demo!
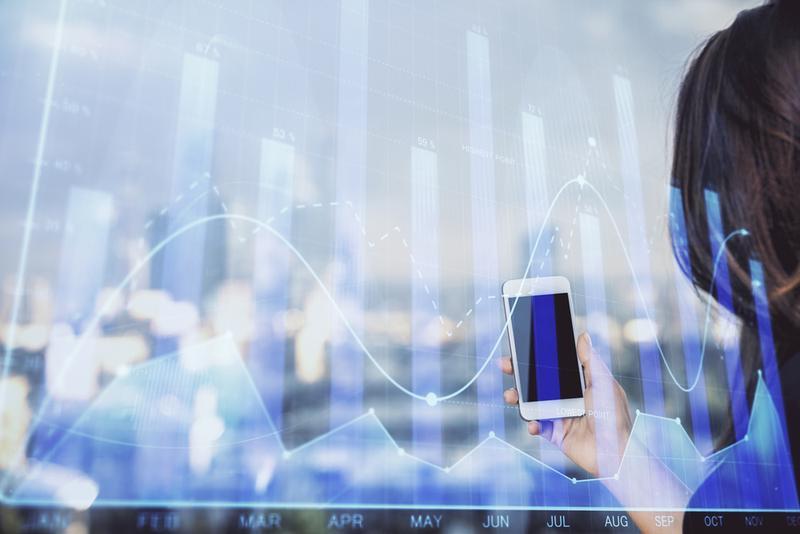
A Beginner’s Guide to Data-Driven Marketing
According to Google, nearly 7 in 10 leading marketers say their companies use data to support decision-making at all levels. Data is the driving force behind successful marketing campaigns and can make the difference between merely surviving and thriving in your industry. Consider all of the amazing data surrounding you—website clicks, customer information, the interactions. All of this data is a goldmine of insights. Now think of this data as a unified map, shaping the way you connect and engage with your audience. Using this data to enhance your campaigns forms the basis of data-driven marketing. What is Data-driven Marketing? Data-driven marketing is about leveraging data insights to make informed decisions and optimize marketing strategies. It relies on concrete facts and figures. By tapping into the wealth of information available, you can understand your audience better, predict trends, and tailor the campaigns for maximum impact. This marketing initiative requires integrating data sources into a centralized repository, where information becomes actionable intelligence. If you’re managing marketing for a healthcare provider, by integrating data from electronic health records, patient surveys, and mobile app usage, you create a comprehensive profile of patient preferences and behaviors. This enables you to send targeted health education materials, appointment reminders, and wellness tips, fostering a more personalized and effective patient communication strategy. Keep in mind that data-driven marketing isn’t just about collecting data; it’s about turning that data into actionable insights. This approach empowers marketers to make strategic decisions based on timely information, creating a more dynamic and responsive marketing strategy. Effective Data-Driven Marketing Campaigns Drive Benefits Data-backed initiatives can have a large positive effective on a company’s revenue. TaxJar, a SaaS paltform for managing taxes, analyzed their data to find that they were saving clients huge amounts of money compared to the price they were charging. This insight enabled them to triple their revenue in a year by creating a tier-based pricing model. KICKS, a cosmetics provider in Scandanavia, provide a personalized online shopping experience for each customer. This experience is based on past shopping behavior. The result: a 1000% increase in email subscribers. GreenPal, a lawncare service provider, saw a 30% increase in conversions after targeting their cost-effective plans to lower-income neighborhoods. Data-driven Marketing Use Cases The applications of data-driven marketing are vast and varied. From personalized content recommendations to targeted advertising, the possibilities are limited only by the granularity and quality of the data at your disposal. Precision in Identifying Prospects with Predictive Lead Scoring Predictive lead scoring assigns numerical values to leads based on historical data, determining the likelihood of conversion. A software company might analyze past customer data to identify patterns indicative of a high-conversion lead, such as a common location or job title. This technique allows the sales team to prioritize efforts on leads with the highest potential, optimizing resource allocation and improving conversion rates. Customizing Content through Dynamic Website Personalization Data-driven marketing enables real-time adaptation of website content based on user behavior. Using tools that analyze user interactions, a travel website can dynamically adjust content to showcase destinations and offers that align with the user’s browsing history and preferences. This level of personalization enhances the user experience and increases the likelihood of conversion. Improving Campaigns via A/B Testing with Behavioral Analytics Combining A/B testing with behavioral analytics provides a granular understanding of user responses to different campaign variations. An e-commerce platform might test two versions of a promotional email, analyzing click-through rates and purchase behavior. The insights gained enable marketers to refine their campaigns and optimize for elements that resonate most with their audience. Understanding Customer Touchpoints through Attribution Modeling Data-driven marketing allows for sophisticated attribution modeling or considering multiple touchpoints in a customer’s journey. A retailer using advanced analytics can track conversions to both the last interaction and the complete customer journey. This comprehensive understanding of touchpoints informs marketing decisions, helping allocate budgets effectively across channels that contribute most to conversions. Data-driven Marketing Best Practices Here are some straightforward strategies that can help optimize your marketing approach for the best results: Customer-Centric Approach: Place the customer at the center of your marketing efforts. Understand their needs, preferences, and pain points and tailor your strategies to provide them with value specifically, which in turn builds lasting relationships. Cross-Channel Consistency: Maintain a consistent brand message and identity across all marketing channels. Whether it’s social media, email, or traditional advertising, a cohesive brand presence reinforces recognition and trust. Agile Marketing Practices: Embrace agility in marketing by adapting quickly to changing trends and consumer behaviors. Stay responsive to market dynamics and allow for timely adjustments to campaigns and strategies. Continuous Testing and Optimization: Implement a culture of continuous improvement through testing. Regularly test and optimize various elements of your marketing mix, from ad creatives to messaging, to find what resonates best with your audience. Performance Metrics Tracking: Establish and track KPIs to measure the success of your marketing efforts. Regularly analyze metrics like conversion rates, click-through rates, and customer acquisition costs to gauge performance. Personalization at Scale: Leverage personalization techniques to connect with your audience on an individual level. Use data to tailor messages and experiences, ensuring that your marketing is relevant and resonant for every customer. Customer Feedback Integration: Actively seek and integrate customer feedback into your marketing strategies. This not only demonstrates a commitment to customer satisfaction but also provides valuable insights for refinement and improvement. Data-driven Marketing Automation Automation plays a crucial role in data-driven marketing. Once you’ve set your marketing goals and gathered data from different sources, you won’t need to deal with the manual tasks of transforming, validating, and creating a centralized repository. Thanks to automated data pipelines, these processes happen smoothly for you. Let’s say you’re collecting data from customer interactions or market trends. With automated pipelines, this data transforms and seamlessly flows into your centralized repository. These pipelines also quickly spot and fix any inconsistencies, guaranteeing the accuracy of your data. Building a centralized repository becomes a breeze as automated pipelines bring together information from various sources into a coherent format, saving you time and reducing errors. Connecting to BI tools for strategic insights becomes a dynamic, near-real-time process. Automated pipelines facilitate this connection, allowing for swift visualization of trends and actionable strategies. Picture a scenario where, with a click, your team can access up-to-the-minute insights on campaign performance or customer behavior. Automation allows your marketing team to focus on high-value tasks like interpreting transformed data and refining strategies rather than handling repetitive, manual processes. It enhances efficiency and adaptability, enabling swift responses to market shifts. Automated data pipelines move your data-driven marketing strategy forward—serving as a scalable, agile, and precise framework for sustained success. Final Word In a world where every user action holds significance, data-driven marketing becomes your catalyst for progress. It’s not just about executing campaigns; it’s about establishing connections, fostering engagement, and continually evolving. Through data utilization, you’re not merely gaining insights into your audience; you’re developing a tailored approach based on precise consumer behavior analytics. Combining data spread across many sources is the first step in analyzing customer info. ETL tools, like LIKE.TG, help gather data and prepare it for analysis. They also empower users to automate the data collection part, allowing them to focus on what matters – strategizing. Transform Your Marketing Strategy Today! LIKE.TG empowers marketers to make informed decisions by automating the data journey. Fuel your success with data-driven strategies that resonate with your audience and deliver measurable results. Learn More!
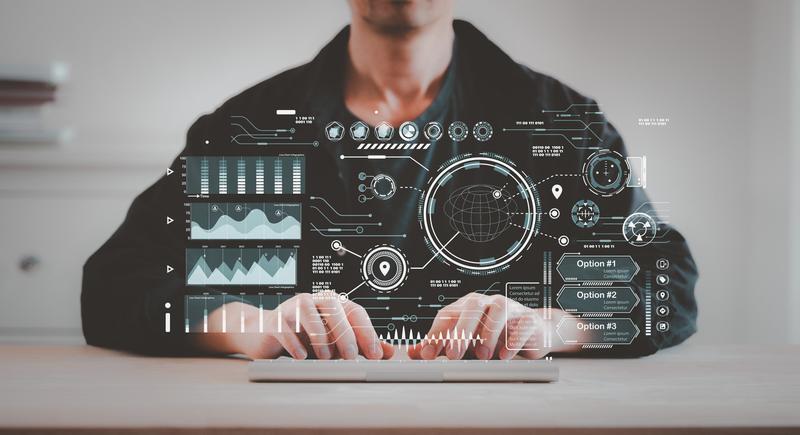
SQL Server for Data Warehouse: Optimizing Data Management and Analysis
We live in an era where organizations spend a fortune for access to the most comprehensive and most up-to-date data set to outdo their competitors. In this pursuit, they invest in the most cutting-edge technologies that capture and transform raw data into actionable intelligence, ultimately providing them with a sustainable competitive advantage. Among the key players in this domain is Microsoft, with its extensive line of products and services, including SQL Server data warehouse.In this article, we’re going to talk about Microsoft’s SQL Server-based data warehouse in detail, but first, let’s quickly get the basics out of the way.The Essential Toolkit for Automated Data WarehousingDive into the critical aspects of Data Warehouse Automation (DWA), including data modeling and data pipelining, with this guide on Automated Data Warehousing.What is a Data Warehouse?A data warehouse is a key component of an organization’s data stack that enables it to consolidate and manage diverse data from various sources. Technically speaking, data warehouses are a specialized type of database that is optimized for handling and analyzing large volumes of data to support business intelligence (BI), analytics, and reporting. Similarly, the SQL Server data warehouse is built on the foundation of the infamous SQL Server database, which is a comprehensive relational database management system (RDBMS) developed by Microsoft.An essential component of the data warehouse architecture is ETL (extract, transform, load). As part of the ETL pipeline, the first step involves data extraction to gather data sets from different sources, such as transactional databases, logs, or external data feeds. Once extracted, the data undergoes the transformation phase in a staging area, where it is cleaned, standardized, and organized into a consistent format. The loading phase transfers the transformed data into the destination, for example, a SQL Server data warehouse, often organized in a dimensional model for optimal query performance.The structured format, commonly using star or snowflake schemas, enables you to navigate and analyze the data with ease. While the ETL process is a critical part of data warehousing, a comprehensive data warehouse architecture also includes storage infrastructure, data modeling, metadata management, security measures, and relevant tools. The overarching goal of this architecture is to provide a robust foundation for analytical processing.SQL Server Data Warehouse Modeling TechniquesIn the context of a data warehouse, data modeling, or simply modeling, refers to the process of structuring and organizing data to facilitate storage, retrieval, and analysis. Let’s go through two of the most common data modeling techniques you can use to build a SQL Server data warehouse:Dimensional ModelingDimensional modeling simplifies data analysis for data and business professionals as it provides a structure that aligns well with the way users think about and analyze data in business contexts. Facts and dimensions are the main components in a dimensional data model, with primary and foreign keys being integral to establishing relationships between them.Data is organized into two types of tables in a dimensional model: fact tables and dimension tables.Fact TablesThese tables contain the quantitative data, or “facts,” that you want to analyze.Common examples include sales amounts, quantities sold, or other measurable metrics.Fact tables often have foreign key relationships with dimension tables.MeasuresThese are quantitative values or metrics, such as sales revenue, quantity sold, profit, etc., that provide the basis for analysis in a data warehouse.Measures can be aggregated using different functions like SUM, AVG, COUNT, MIN, MAX, etc. to analyze data at different levels of granularity.Measures are typically stored in fact tables and are often analyzed in the context of dimension hierarchies.Dimension TablesThese tables store descriptive information or dimensions related to the facts in the fact tables. Dimensions are the characteristics by which you want to analyze your business.Examples of dimensions might include time, geography, product categories, or customer details.Dimension tables typically have a primary key that serves as a foreign key in the fact table.You can use dimensional modeling to design and implement a SQL Server data warehouse when facilitating efficient BI processes is the overall business requirement.Data Vault ModelingIf your organization operates on a large scale and involves complex data warehousing environments, data vault modeling can offer significant gains. Even more so if data traceability, scalability, and flexibility are of prime importance. Data vault modeling combines elements from both the Third Normal Form (3NF) and star schema approaches to create a flexible and scalable data warehouse architecture.Do You Really Need a Data Vault?Data Vault 2.0 modeling methodology has gained immense popularity since its launch in 2013. Find out if your data warehouse architecture will actually benefit from a Data Vault.Learn MoreThe primary elements in data vault modeling are:HubsHubs serve as the central repositories for business keys, or identifiers, that store unique and unchanging business data and provide a solid reference point for each business entity. Think of Hubs as tables, as in 3NF but much simpler, with just a single key column and, often, some extra information for documentation. When building a SQL Server data warehouse using data vault modeling, you implement Hubs as tables in the SQL Server environment.LinksLinks are entities that establish relationships between Hubs. You need Links to connect different business entities and form associations within the data warehouse. In a sales scenario, for instance, a Link might tie together a customer Hub with a product Hub, showing you who bought what. In the context of building a SQL Server data warehouse via data vault modeling, you would implement Links as tables, which then become the active agents that handle relationships between your Hubs.SatellitesSatellites capture changes in data over time—they store historical information about your Hubs or Links. For instance, if a customer’s address changes, the Satellite table associated with the customer Hub will store the historical addresses. Just like with Links, Satellites also contribute to scalability. As your business grows and data changes, you can extend these Satellite tables without disrupting your core Hub or Link structures. Again, if you’re building a SQL Server data warehouse via data vault modeling, you would implement Satellites as tables to continually capture changes in your data.Data Warehouse SchemasData warehouse schemas define how data is organized and structured within a data warehouse. They play a crucial role in facilitating efficient querying and reporting. There are mainly three types of data warehouse schemas: star schema, snowflake schema, and galaxy schema (also known as a fact constellation).Each schema has its own advantages and trade-offs. The choice of schema depends on factors such as the nature of your data, query patterns, and performance considerations. Star schemas are commonly used for their simplicity and query performance, while snowflake schemas and galaxy schemas provide more normalization, supporting complex data structures and relationships.Star SchemaIn a star schema, you have a central fact table surrounded by dimension tables. The fact table holds your key business metrics, like sales revenue. The dimensions provide context, such as product, time, and location. It looks like a star when you draw it out, with the fact table at the center and dimensions branching out. It’s easy to understand, and because it’s denormalized, querying is efficient.Snowflake SchemaNow, imagine extending the star schema. In a snowflake schema, your dimensions get broken down into sub-dimensions or related tables. It’s like a more detailed version of the star, reducing redundancy in your data. However, the trade-off is that queries might be a bit more complex and slower due to additional joins. The name “snowflake” comes from the shape of the schema diagram, with all these branching structures.Galaxy SchemaIn a galaxy schema, you’re dealing with multiple fact tables that share dimension tables. This is handy in complex data warehouse setups with different business processes generating various metrics. The fact tables connect through shared dimensions, allowing for a flexible and comprehensive analysis of data across different processes. It’s like having multiple centers (fact tables) connected by common links (dimension tables).Why use SQL Server for Data Warehousing?SQL Server’s strength in handling relational databases makes it an excellent choice, especially when most systems and applications generating and managing data transactions within your organization are structured in a relational database format. The seamless transition of relational data into a SQL Server data warehouse simplifies the integration process and ensures compatibility across the data ecosystem. This is particularly effective in scenarios where maintaining data consistency and relationships are crucial, for instance extracting accurate insights to optimize business processes.Cut Down Data Warehouse Development Time by up to 80%Traditional data warehouse development requires significant investment in terms of time and resources. However, with LIKE.TG DW Builder, you can reduce the entire data warehouse design and development lifecycle by up to 80%. Learn more in this whitepaper.Download WhitepaperAdditionally, you can combine dimensional modeling and OLAP cubes in SQL Server Analysis Services (SSAS) to create high-performance data warehouses. Doing so reduces the need for extensive joins and computations during query execution, which leads to faster response times.Microsoft-centric EnvironmentsWhen your organization predominantly uses Microsoft technologies such as Power BI, Excel, and Azure services, leveraging SQL Server for data warehousing ensures a cohesive and integrated analytics ecosystem.Analytical Query PerformanceIn scenarios where analytical query performance is crucial, SQL Server’s columnstore index technology proves to be significantly beneficial. It excels in handling large-scale data and executing complex analytical queries, making it well-suited for data warehousing where quick and detailed analysis is the primary objective.Mixed WorkloadsSQL Server can be an excellent choice if your organization deals with mixed workloads that involve both transactional and analytical processing. Its ability to handle both types of workloads in a unified platform can simplify the overall data management process for your business.Integration of External Data SourcesWhen you need to integrate data from diverse external sources, SQL Server’s PolyBase feature can facilitate the process. This capability is particularly valuable in data warehousing scenarios where data consolidation from various platforms is a common requirement.Scalability RequirementsIf your organization is experiencing growing data volumes, it can benefit from SQL Server’s features like partitioning and parallel processing to meet scalability demands.Cloud-based Data WarehousingSQL Server seamlessly integrates with Azure services, offering flexibility and scalability in the cloud. It can be an added advantage in scenarios where you want to leverage the benefits of a cloud-based data warehousing architecture.How to Build SQL Server Data Warehouse?Building a data warehouse is a multifaceted task that involves multiple steps. However, a data warehousing tool, such as LIKE.TG Data Warehouse Builder, eliminates most of these steps, especially in the areas of schema design and SQL ETL processes—so much so that the entire process is the same regardless of the type of data warehouse.Here are the steps to build a SQL Server data warehouse:Step 1: Create a Source Data ModelFirst you need to identify and model the source data. With LIKE.TG, this is as simple as reverse engineering the source data model. Once you have the source data model, you can verify it and check for errors and warnings. Once again, this can easily be done with a click of a button.After you’re certain that you have modeled the source data correctly, all you need to do is to deploy it to the server and make it available for use in ETL or ELT pipelines or for data analytics. With LIKE.TG, this is as simple as clicking on “Deploy Data Model”, as shown below:SQL Server data warehouse: Deploying Data Model in LIKE.TGStep 2: Build and Deploy a Dimensional ModelThe next step is to build a dimensional model that serves as the destination schema for the data warehouse. You can design a model from scratch seamlessly using the “Entity” object in LIKE.TG.However, if you already have a database schema designed, you can automatically create a dimensional model using the “Build Dimensional Model” option. It allows you to decide which tables will be facts and which will be dimensions. Here’s what a dimensional model can look like in LIKE.TG’s UI:SQL Server based data warehouse: Dimensional ModelBuild a Custom Data Warehouse Within Days—Not MonthsBuilding a data warehouse no longer requires coding. With LIKE.TG Data Warehouse Builder you can design a data warehouse and deploy it to the cloud without writing a single line of code.Learn MoreNext, you can assign specific roles to the fields for each entity (or table) for enhanced data storage and retrieval. For example, you can select either of the following for dimensions:Surrogate Key and Business Key.Slowly Changing Dimension types (SCD1, SCD2, SCD3, and SCD6).Record identifiers (Effective and Expiration dates, Current Record Designator, and Version Number) to keep track of historical data.Placeholder Dimension to keep track of early arriving facts and late arriving dimensions.Once your dimensional model is built and verified, you can forward engineer it to the destination where you want to maintain your data warehouse, in this case, SQL Server, and deploy it.Step 3: Populate the Data WarehouseNow that you have your data warehouse set up, you need to build data pipelines to populate it. Once again, this is something you can easily achieve within LIKE.TG’s UI, and without writing any codes.To do so, you need to create a dataflow and start building your ETL pipelines. Let’s say you want to move customers data into your new SQL Server data warehouse, here’s what the dataflow would look like in LIKE.TG’s UI:SQL Server data warehouse: Dimensions table dataflowHere we have the source table on the left and the “Dimensional Loader” object on the right. You’ll have to use this object to move data into a table in the destination dimensional model.You’ll also need to create a dataflow to move data into the fact tables. Since the fact table contains fields from multiple source tables, the dataflow will likely be a bit different. Additionally, we can use “Data Model Query Source” since we need to extract data from multiple tables in the source model. Here’s the dataflow for the fact table:SQL Server data warehouse: Facts table dataflowFinally, execute the dataflows and start populating your SQL Server data warehouse.Step 4: Orchestrate and AutomateTo orchestrate the process, you can create a workflow and eliminate the need to execute the dataflows one by one.Workflow Automation in LIKE.TGAdditionally, you can automate the process so that the data is loaded into the data warehouse automatically.Build Your Data Warehouse Effortlessly With a 100% No-Code PlatformBuild a fully functional data warehouse within days. Deploy on premises or in the cloud. Leverage powerful ETL/ELT pipelines. Ensure data quality throughout. All without writing a single line of code.Download TrialLimitations of Setting up a SQL Server Data WarehouseSetting up a SQL Server data warehouse comes with its own set of challenges and limitations. Understanding these limitations is crucial for making informed decisions when setting up a SQL Server data warehouse. It helps you assess whether the chosen solution aligns with your organization’s specific needs and requirements.Let’s break down what this means:Learning CurveSetting up and managing a SQL Server data warehouse requires a high level of expertise. Your team might need training to effectively design, implement, and maintain the data warehouse. This includes gaining knowledge about indexing strategies, partitioning, and statistics maintenance. Additionally, familiarity with tools for monitoring and troubleshooting is also crucial for ensuring the system’s health and addressing any issues that may arise.ScalabilityWhen it comes to dealing with extremely large datasets, a SQL Server based data warehouse might face scalability issues. While the platform is designed for analytics workloads and allows horizontal scaling by adding more compute nodes, there could be challenges in handling truly massive data. In such cases, alternative solutions that specialize in distributed computing might be worth exploring to ensure seamless scalability for your data storage and processing needs.PerformancePerformance becomes a critical concern as data scales up in a SQL Server data warehouse, necessitating you divert extra attention toward query optimization and indexing. Strategically optimizing queries and implementing effective indexing mechanisms are vital to mitigate the impact of growing data volumes. The outcome is an efficient and responsive query processing within the SQL Server data warehouse environment.ComplexityBuilding a SQL Server data warehouse introduces a specific set of challenges, with complexity standing out as a notable limitation. The twists and turns surface during the design phase, where a thorough plan proves its worth in helping you craft the schema and implement effective ETL processes. Ensuring data quality further adds to the intricacy as it demands ongoing attention and validation, making the overall process even more challenging.Integration with Other SystemsIntegration with other systems is a crucial aspect when considering the implementation of a SQL Server data warehouse. In a business environment, data often resides in various sources and formats, including different databases, applications, and external data feeds. The challenge lies in harmonizing and consolidating this diverse data into the SQL Server data warehouse, as compatibility issues can come up due to differences in data formats, structures, or communication protocols between systems. So, your data teams might need to resort to custom integration efforts to bridge these gaps and establish a seamless flow of data into the data warehouse.Related: Learn about creating an SQL Server API.Data Warehouse Best Practices for SQL ServerClearly define your business requirements and goals for the data warehouse. You should also have a full understanding of the reporting and analysis needs of the end users.Choose the appropriate data modeling approach for the SQL Server data warehouse. This will be guided by and based on your business requirements. Additionally, normalize or denormalize data structures as needed.Incorporate SQL Server replication to ensure optimal and timely data distribution across the architecture.When anticipating growth, decide whether your data warehouse should be designed to scale horizontally or vertically. Consider partitioning large tables to further enhance scalability.Use modern data integration tools to build, automate, and maintain your ETL pipelines. Prioritize solutions that can help you implement parallel processing for ETL tasks to optimize performance. Always implement data quality checks during the ETL process to eliminate data health related issues.Before going live, conduct thorough testing of the data warehouse, including ETL processes, data integrity, and query performance. Similarly, validate the accuracy of reports and analytics against business requirements to ensure that the insights derived from the data warehouse align with the intended business goals.Key TakeawayBuilding a data warehouse can be a long and resource-intensive journey, and SQL Server data warehouse is no exception. However, much of the process can be shortened if you plan the process thoroughly from the outset of the project and incorporate highly capable data warehouse building solutions, such as LIKE.TG Data Warehouse Builder.If you’re looking to build a SQL Server data warehouse and time is of the essence, contact us at +1 888-77-LIKE.TG and get in touch with one of our data solutions experts for professional advice.Alternatively, you can sign up for a demo or download a 14-day free trial to test it yourself and see if it fits your requirements.Build Your Data Warehouse Effortlessly With a 100% No-Code PlatformBuild a fully functional data warehouse within days. Deploy on premises or in the cloud. Leverage powerful ETL/ELT pipelines. Ensure data quality throughout. All without writing a single line of code.Download Trial
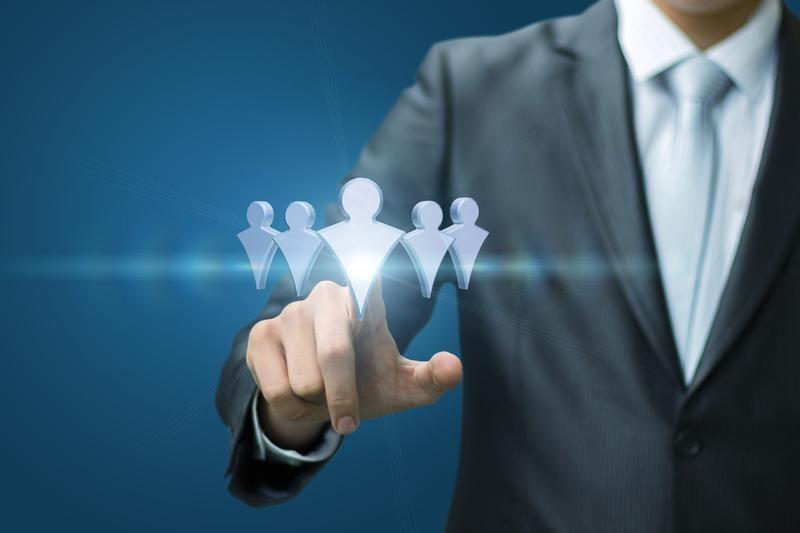
What Is Data Quality and Why Is It Important?
What Is Data Quality?Data quality is the measure of data health across several dimensions, such as accuracy, completeness, consistency, reliability, etc. It serves as the foundation upon which your data team can build a dependable information infrastructure for all your data-driven processes and initiatives—whether it’s analyzing data, extracting meaningful insights, or driving successful business strategies. In short, the quality of your data directly impacts the effectiveness of your decisions.It’s important to note that data quality goes beyond simply ticking a checkbox—it’s an ongoing commitment to preserving the accuracy and reliability of your data. In other words, high-quality data results from effective data quality management, a continuous effort to ensure that only accurate data drives all your BI and analytics efforts. It involves implementing robust processes, validating accuracy, and maintaining consistency over time, leading to a single source of truth (SSOT).Your Guide to Data Quality ManagementManaging tons of data is tough, but there's a bigger challenge: keeping your data in tip-top shape. This eBook is your guide to ensuring data quality across your organization for accurate BI and analytics.Why Is Data Quality Important?Now, let’s talk about the importance of data quality. What makes it all that important? Simply put, the healthier the data, the better the outcome.The health of your data directly affects the effectiveness of several crucial frameworks that empower your organization. Ensuring the accuracy of your data allows you to actively strengthen the very tools you use to manage and analyze it. Your data governance framework will likely fall short of enforcing access controls properly or ensuring full compliance if your data is riddled with errors and inconsistencies. The same applies to data security. Dirty data, with errors and missing information, makes it harder for your data teams to identify suspicious activity or isolate threats.The quality of data also affects the reliability and usability of your data catalog—high-quality data leads to a useful catalog, and a well-maintained data catalog facilitates effective data quality management practices.Machine learning (ML) algorithms and artificial intelligence (AI) models rely heavily on data to learn and make predictions. High-quality data with clear provenance (where it came from) makes it easier to trace the model’s reasoning and ensure its decisions are aligned with your expectations.Data regulations are prevalent across many industries, and maintaining high-quality data is essential for ensuring compliance with these legal and regulatory requirements. Failure to adhere to these standards can have serious consequences, resulting in legal repercussions and potentially damaging your organization’s reputation.Benefits of Ensuring Data QualityInformed Decision-Making: High-quality data improves decision-making. When your data is accurate and reliable, you can trust the insights derived from it, leading to more informed and strategic decisions.Operational Efficiency: Healthy data allows you to avoid costly errors. It’s an investment in streamlining your operations, improved financial performance, and a strong foundation for building customer trust. For example, accurate and complete inventory data gives you a holistic picture of your stock, preventing stockouts and ensuring smooth order fulfillment.Innovation and Competitive Advantage: High-quality data empowers organizations to identify new opportunities, adapt to changing market dynamics, and innovate faster. Consequently, it helps them stay ahead of the curve and maintain a competitive edge.Customer Trust and Satisfaction: If your data is trustworthy, it instills confidence in your brand as customers rely on accurate information. Inaccurate data erodes customer trust and satisfaction, potentially leading to customer dissatisfaction and loss of business.Efficient Resource Allocation: Whether it’s budgeting, workforce planning, or project management, accurate data ensures that resources are utilized optimally, all the while preventing waste and maximizing efficiency.Data Governance and Data QualityWhen it comes to managing your data, two crucial aspects to keep in mind are data governance and data quality. Both these concepts emphasize the fact that data is not just a chaotic mess but a well-organized and reliable asset for your organization.Think of data governance as the rulebook for data management. It sets the ground rules that define who will have access to what data, ensuring it’s handled responsibly and securely within your organization. Apart from documenting data policies, it involves implementing data stewardship programs and establishing mechanisms for resolving data-related issues. Data governance extends its influence across the entire data lifecycle—from creation to deletion.On the other hand, data quality is all about how good, or healthy, your data is. Is it accurate, consistent, and up-to-date, or is it a huge pile of mess? High-quality data means you can trust it to make informed decisions. However, to maintain top-tier data quality, you need processes that clean up errors, validate information, and keep everything in tip-top shape.Data Integrity vs. Data QualitySpeaking of maintaining data quality, we have a closely related concept called data integrity, which preserves the data throughout its lifecycle. Both these concepts complement each other as they are essential to making informed decisions and achieving desired outcomes. Suffice to say that high-quality data is achieved as a result of maintaining solid data integrity.Here are the differences between data integrity vs data quality:While data quality focuses on the overall health of your data, i.e., how well or fit it is for use, data integrity is what keeps it unchanged and consistent at all times.With data quality, the goal is to enhance the accuracy, completeness, and reliability of data for analysis and decision-making processes. On the other hand, the goal with data integrity is to prevent unauthorized alterations or distortions to ensure that the data you rely on is trustworthy and reflects the real-world scenario.Poor data quality can result in inaccuracies, inconsistencies, and incompleteness in the data set, leading to incorrect analyses and flawed decision-making. Issues with data integrity mainly stem from system failures or security breaches and can lead to loss of data, unauthorized access to sensitive information, and damage to reputation.You can address data quality issues through data profiling, cleansing, validation rules, and regular data audits. However, to maintain data integrity, you need to go a step further and implement data protection techniques, such as access controls, encryption, checksums, hashing, and version control systems.Ensure Only Healthy Data Reaches Your Data Warehouse With LIKE.TGLooking to achieve a single source of truth? The first step is to ensure that all your data assets are in optimal health. Elevate data quality with LIKE.TG.Learn MoreThe Need for a Data Quality FrameworkA data quality framework is essentially a structured approach to managing the quality of your data. It involves a set of processes, rules, standards, and tools to guarantee that your data is accurate and reliable. A data quality framework generally has the following key components:Data ProfilingStart by getting to know your data. Data profiling enables you to analyze the content, structure, and relationships within your data sets and identify inconsistencies and outliers.Data StandardizationSet clear standards for how data should be formatted and represented. Data standardization ensures consistency across your data sets, making it easier to analyze and compare information.Data CleansingData cleansing involves detecting and correcting errors in your data sets, such as missing values, duplicates, or inaccuracies.Data MonitoringKeep a watchful eye on the health of your data. Implement monitoring processes to track changes in real-time and maintain data quality.Data GovernanceEnforce accountability and a well-organized approach to maintaining data health by establishing clear roles and responsibilities. Define who’s in charge of what when it comes to data quality.How to Measure Data QualityJust like we track our physical health with regular checkups, monitoring your data’s health through quality measures is crucial. It’s the only way to confirm your information assets are fit for purpose and driving accurate insights.But how do we measure data quality?Measuring data quality isn’t a one-size-fits-all approach, but rather a tailored exploration into your data assets and their intended uses. Additionally, your organization should clearly define what “good” or “healthy” data means for its specific needs.Having said that, data quality measurement generally involves assessing data health against a number of dimensions.Data Quality DimensionsData quality dimensions serve as benchmarks to examine the health and fitness of your data, and how well they meet your requirements.Data Quality DimensionsWhile there’s no universally agreed-upon set, some of the most commonly used data quality dimensions include:Accuracy: Accuracy measures how precisely your data reflects the real world it represents. Are you confident that the recorded age of a customer is truly their age, or could it be a typo?Completeness: Completeness measures whether any essential information is missing from your data. Are there empty fields in a customer record, or missing values in a financial report?Consistency: Consistency means that your data adheres to predefined rules and formats across different platforms and systems. Are all date formats consistent? Are units of measurement used uniformly?Timeliness: Timeliness refers to the freshness and relevance of your data. Is your inventory data updated to reflect current stock levels, or is it lagging behind? Are you analyzing the latest sales figures or outdated statistics?Uniqueness: Uniqueness verifies that all records in your data set are distinct and don’t contain duplicates. Are there multiple entries for the same customer with different email addresses?Validity: Validity checks whether the data values fall within acceptable ranges and adhere to defined constraints. Are phone numbers formatted correctly? Do product prices stay within realistic boundaries?Some data quality frameworks also include relevancy, integrity, granularity, and accessibility as the relevant data quality dimensions.Data Quality MetricsOnce you’ve identified the dimensions you want to measure the quality of your data against, it’s time to translate them into specific, measurable metrics. Visualizing these metrics on dashboards allows you to track data quality over time and prioritize areas for improvement.Let’s take a look at some metrics for different data quality dimensions:Accuracy Metrics: To measure how accurate the data sets are. Examples can include:Error rate: Percentage of data points that are incorrect.Matching rate: Percentage of data points that match a known source of truth.Mean absolute error: Average difference between data points and their true values.Completeness Metrics: To measure the proportion of missing data within a data set. Examples generally include:Missing value percentage: Percentage of fields with missing values.Completion rate: Percentage of records with all required fields filled.Record count ratio: Ratio of complete records to total records.Consistency Metrics: To measure whether data adheres to predefined rules and formats. Some examples include:Standardization rate: Percentage of data points conforming to a specific format.Outlier rate: Percentage of data points that deviate significantly from the norm.Duplicate record rate: Percentage of records that are identical copies of others.Timeliness Metrics: To measure the freshness and relevance of your data. Examples include:Data age: Average time elapsed since data was captured or updated.Latency: Time taken for data to be available after its generation.Currency rate: Percentage of data points that reflect the latest information.Uniqueness Metrics: To ensure all records are distinct and avoid duplicates. Examples include:Unique record rate: Percentage of records with unique identifiers.Deduplication rate: Percentage of duplicate records identified and removed.Take the First Step Towards Enhancing Data Quality. Try LIKE.TG for Free.Ready to maximize the health of your data? Try LIKE.TG's leading platform and witness firsthand how it improves data quality, elevating your insights and decision-making.Download TrialData Quality IssuesIssues with data quality can wreak havoc on your analysis, especially if left unchecked for long. While these issues can arise due to multiple reasons, including inaccurate data entry or inconsistent data formats, it’s mostly the lack of data governance and a proper data quality framework that causes them.Here are some of the most common data quality issues:Inaccurate DataIssues related to accuracy usually stem from typos, misspellings, or outdated information. Sometimes, it’s just the data collection process that’s flawed that leads to inaccurate data. Moreover, if your data favors a certain group or excludes others, it can lead to skewed results.Incomplete DataFactors such as system integration issues and data entry errors frequently lead to omitted records and empty fields. Sometimes users overlook certain fields or fail to provide complete information, especially in forms or surveys, which also leads to incomplete data. Analyzing incomplete data leads to impaired insights and questionable decision-making.Outdated DataOutdated data is a significant data quality issue as it compromises data reliability and validity. As data ages, it becomes less reflective of the present circumstances, potentially leading to misguided analyses and decision-making. And in dynamic environments where conditions change rapidly, relying on outdated data can result in strategic missteps and missed opportunities. The consequences extend beyond mere informational discrepancies; they encompass operational inefficiencies and compromised forecasting accuracy.Duplicate DataThis issue often arises due to system glitches or during the integration of data from multiple sources. Data entry errors also contribute to duplicate data. The consequences are multifaceted, ranging from skewed analyses to operational inefficiencies. Specifically, it can lead to overestimation or underestimation of certain metrics, which impacts the accuracy of statistical analyses and business insights. As far as resource utilization is concerned, duplication not only clutters databases but also consumes valuable storage space.Inconsistent DataInconsistency in data usually results from different formats, units of measurement, or naming conventions across records. The root causes often include diverse data sources, changes in data collection methods, or evolving business processes. The consequences of inconsistent data are substantial, leading to difficulties in data integration and compromising the reliability of analyses. Decision-makers may face challenges in comparing and combining information, hindering the ability to derive cohesive insights.Beyond these issues, sometimes too much data can also lead to data quality problems—in fact, it can be a double-edged sword. This phenomenon, often referred to as data overload, occurs when there’s an overwhelming volume of information to process. It can strain resources and slow down analysis and increase the likelihood of errors.How to Improve Data QualityIdentifying data quality issues is half the work—your data team should be well-versed to resolve these issues efficiently.Improving and maintaining the health of your data sets generally begins with establishing clear data quality standards and protocols to guide the correction process. Once you’re through that, here are some steps you can take to improve data quality:Implement Data Quality ChecksData quality checks serve as a proactive measure to maintain the health of your data sets and support effective decision-making processes within your organization. Specifically, these are systematic processes that you can implement to assess and guarantee the accuracy, completeness, consistency, and reliability of your data. They involve a series of evaluations, including:Format ChecksRange ChecksCompleteness ChecksDuplicate ChecksConsistency ChecksConduct Regular Data AuditsPeriodically reviewing your data sets at scheduled intervals will enable you to identify and rectify errors, inconsistencies, and outdated information. When your team identifies and addresses data quality issues early in the data lifecycle, they can prevent the propagation of inaccuracies into analyses and decision-making processes.Appoint and Empower Data StewardsOne strategic move that you can take to maintain data health is appointing data stewards who take on the responsibility of overseeing specific data sets and addressing issues promptly. They play a crucial role in maintaining data integrity, enforcing standards, and serving as the point of contact for all data-related concerns. Empowering data stewards with the authority and resources to make decisions regarding data quality allows for a more proactive and efficient approach to managing and improving the quality of your data.Eliminate Data SilosData silos, where information may be isolated within specific departments or systems in your organization, often lead to inconsistencies and inaccuracies. By integrating data from different sources and eliminating silos, you create a more cohesive and reliable data set. This integration facilitates cross-referencing, and consistency checks, ultimately contributing to a more accurate and comprehensive understanding of your data.Use Data Quality ToolsIn addition to the steps discussed above, you can use software solutions to ensure that only healthy data populates your data warehouses. These software solutions, also called data quality tools, are designed to assess, enhance, and manage the quality of organizational data in an automated manner.Two of the most common categories of data quality tools are standalone solutions, that are only concerned with improving the quality of data sets, and integrated solutions that seamlessly incorporate data quality functionalities into broader data integration tools, such as LIKE.TG. The choice between standalone and integrated solutions will depend on your organization’s specific needs and priorities in managing and improving data quality.See It in Action: Sign Up for a DemoCurious about how LIKE.TG's platform improves data quality? Sign up for a demo and explore all the features you can leverage to get analysis-ready data without writing a single line of code.View DemoData Quality Best PracticesMaintaining data quality is an ongoing process that demands a systematic approach. It involves continuous monitoring and refinement of data-related practices to uphold data integrity and reliability. Here are some data quality best practices that you can incorporate into your data quality management framework for a more capable and reliable data ecosystem:Standardize Data FormatsConsistent data formats are vital to prevent errors and enhance interoperability. When data follows a uniform structure, it minimizes the risk of misinterpretation during analysis. To implement this, establish a standardized format for various data elements, including date formats, numerical representations, and text conventions. This way, you’ll be able to create a foundation for accurate and reliable data.Implement Data Validation RulesThe implementation of robust data validation rules serves as a frontline defense against inaccurate data. These rules act as automated checks that assess incoming data for accuracy, completeness, and adherence to predefined standards. By defining and consistently applying these rules, you ensure that only high-quality data enters the target destination system.Establish Data Governance PoliciesBy creating clear guidelines for data usage and access, you provide a framework that mitigates the risk of unauthorized changes to data sets. Regular audits and strict enforcement of these policies are essential to maintaining a secure data ecosystem. This way, you ensure that data is always accessed and utilized in accordance with established protocols.Prioritize Data RelevancePrioritizing data relevance is a strategic approach to maintaining a focused and impactful data set. Regular assessments of each data element’s importance in relation to current business objectives are crucial. Identifying and removing obsolete or redundant data enables you to streamline your data set and make it more efficient for analyses and decision-making processes.Enforce Data Lineage TrackingImplementing tools and processes to trace the origin and transformations of data throughout its lifecycle is essential. By documenting metadata, transformations, and dependencies, you create a comprehensive data lineage map. This map becomes a valuable resource for troubleshooting, auditing, and ensuring the accuracy of data-driven insights.Take the First Step Towards Enhancing Data Quality. Try LIKE.TG for Free.Ready to maximize the health of your data? Try LIKE.TG's leading platform and witness firsthand how it improves data quality, elevating your insights and decision-making.Download TrialEnsure Data Quality With LIKE.TGAs data volumes continue to grow, businesses not only require a data quality solution but also a robust tool capable of managing and integrating data at scale. It gets even better when both of these functionalities come in a single package.Enter LIKE.TG—an end-to-end data management and integration solution that seamlessly incorporates data quality features into its platform to ensure data accuracy, completeness, and reliability. With its user-friendly and consistent UI, LIKE.TG simplifies the process of enhancing data quality, taking the hassle out of the equation.Data Quality – Data health displayed in LIKE.TG’s UIWith LIKE.TG, you can:Use Data Profiling to analyze your data’s structure and quality.Use the Data Cleanse transformation to clean your data sets effortlessly.Use Data Quality Rules to validate data at the record-level without affecting the entire data set.Use automated Data Validation to quickly check your data sets against set rules.And much more—all without writing a single line of code.Ready to improve organizational data quality? Contact us at +1 888-77-LIKE.TG. Alternatively, you can also download a 14-day free trial to test it out yourself.
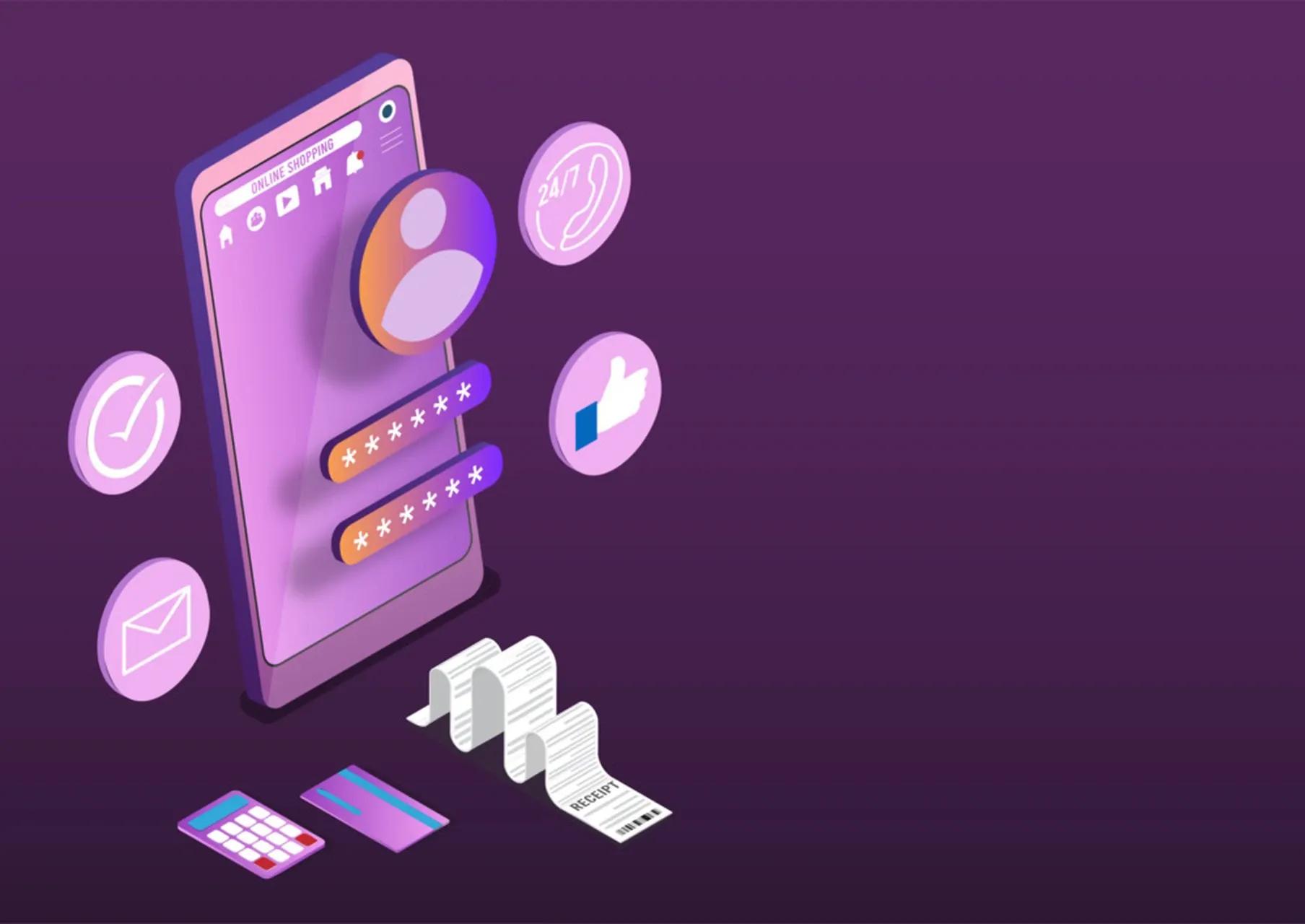
Data Centralization – A Guide to Modernizing Financial Analytics
What is Data Centralization? Data centralization is the process of consolidating data from multiple sources into a single, centralized location, usually a database, cloud data warehouse, or a data lake. Centralizing data makes it more accessible, secure, and helps achieve a single source of truth for improved decision-making. Organizations gain a complete view of their financial situation by consolidating data from various systems, including accounting software, and CRM tools. A closely related concept here is of distributed data. What is the Difference Between Centralized Data and Distributed Data? The main difference is in how they are stored. In a centralized repository, all the data resides in a single location, while in distributed systems the data is spread out. Some characteristics of both methods: Centralized Data: Access to the data is typically controlled by a central authority or server. Examples of centralized data systems include traditional databases managed by a single server or data warehouses where data is consolidated for analysis. Distributed Data: In a distributed data system, data is spread across multiple locations or nodes within a network. There is no single central authority controlling all data; instead, data may be replicated or partitioned across different nodes. Access to the data and processing may occur locally on each node, reducing the need for centralized coordination. Examples of distributed data systems include peer-to-peer networks and distributed databases like DynamoDB. Key Differences: Control: Centralized data has a single point of control, while distributed data may have multiple points of control or none at all. Location: Centralized data is stored in one or a few locations, whereas distributed data is spread across multiple locations or nodes. Access: Accessing centralized data typically requires interacting with a central authority, while distributed data may allow for more decentralized access and processing. Scalability and Fault Tolerance: Distributed data systems are often more scalable and fault-tolerant due to their decentralized nature, whereas centralized systems may face limitations in these areas. Network Dependency: Distributed data systems rely heavily on network communication between nodes, while centralized systems may have less reliance on network performance for data access. The Shift from Siloed Data to Centralized Data Many organizations still operate with siloed data, limiting their ability to harness analytics’ power fully.Siloed datarefers to information that is segregated or compartmentalized within an organization and stored in separate databases or systems managed by individual departments or teams. In such cases, data isn’t easily accessible or shared across the organization. Siloed data often results from a combination of factors, including disparate systems, inconsistent data formats, varying access permissions, or lack of integration, i.e., different departments using their own databases without integrating them into a unified system. These factors collectively lead to challenges in data management. Siloed Data Challenges Organizations face several hurdles due to decentralized data. These challenges include: Legacy Systems:Outdated systems make it difficult to get the data you need into yourdata warehouse. Divergent data sources can lead to conflicting information, undermining accuracy and reliability. Analysis Difficulties:Data in diverse and scattered sources requires extensive effort to consolidate and interpret, limiting data analytics capabilities. Timely Decision-making Impediments:Data consolidationand reconciliation delays hinder prompt decision-making, which puts your company at a disadvantage to those able to process in real time. Imagine a big organization with many departments, each responsible for its own financial data. The marketing department has its own set of spreadsheets tracking advertising expenses and campaign performance. The sales department has a CRM system that records customer transactions and revenue. The finance department has its own accounting software to manage financial statements. The result? With data scattered across these silos, it’s challenging to gain a holistic view of the organization’s operations. The solution: Data centralization. The Benefits of Data Centralization Data centralization has been growing in importance, and rightly so—given the several benefits it offers: Improved Decision-Making:Data centralization enables everyone in the team to get a holistic view of the data they work on. For example, finance teams gain a comprehensive understanding of cash flow, revenue streams, and financial metrics. Having the most up-to-date information and a complete picture of all your data allows for more accurate forecasting and strategic decision-making. Enhanced Efficiency:Data centralization streamlines business operations by eliminating manual data gathering from disparate sources. In finance, it would mean speeding up the monthly and quarterly reporting exponentially. Hence, data centralization boosts efficiency and productivity, allowing professionals to focus on strategic analysis. Data Integrity and Compliance:Centralizing data leads to enhanceddata integrity.It does so by maintaining data consistency and minimizing errors and discrepancies in the data sets. Additionally, complying with regulatory requirements is much easier when your data is organized and accessible. Simplified Data Analysis and Reporting: Data centralization lays the foundation for advanced analytics. With all relevant data in one place, organizations can use advanced analytics techniques, such as predictive modeling and ML, to uncover valuable insights. It’s easier to perform data analysis and generate meaningful reports with all the data in one place. Analysts can access a unified dataset without the need for complex data integration or reconciliation processes. Scalability and Flexibility:As organizations grow, centralization provides the scalability and flexibility needed to accommodate increasing data volumes and changing business requirements. The centralized repository can easily be expanded or modified to adapt to new data sources and analytical needs. Enhanced Data Security: Centralizing data facilitates better security measures and access controls as a single, centralized repository is easier to manage. Organizations can implement centralized security policies, encryption standards, and authentication mechanisms to protect sensitive data from unauthorized access. Improved Data Quality: Centralizing data improves its quality. During the centralization process data is cleansed and standardized based on strict company standards. This helps create a single repository of accurate and timely data, ensuring teams and management have more trustworthy data for analysis, potentially saving them hundreds of thousands of dollars in erroneous reporting and forecasting. Increased Cost Savings: Centralizing data increases cost savings by reducing duplication of efforts as all data is present in a single location. This deduplication also minimizes the need for redundant infrastructure and optimizes data management processes. Steps to Centralize Organizational Data Centralizing data requires careful planning and execution. Let’s explore the key steps organizations should consider: Assessing Your Current Data Infrastructure: Before centralizing data, evaluate your existing data infrastructure. Identify and document the current systems and tools, assessdata quality, and identify any gaps or redundancies. For example, during the assessment, you may discover that different departments within your organization use multiple data sources, resulting in data duplication and inconsistencies. Define Data Centralization Goals: Clearly define the goals and objectives of centralizing organizational data. Determine what benefits you aim to achieve, and how centralization aligns with your organization’s broader objectives. Are you hoping to achieve improved data quality? Or does your business require streamlined compliance? These are some questions your data centralizing plan should have answers to. Develop a Data Governance Framework: Establish a framework to govern the centralized data effectively. Define data ownership, responsibilities, access controls, and security policies. Implement data quality standards, metadata management practices, and data lifecycle management processes. A data governance framework acts as a guide to managing data. Select Centralized Data Architecture: Choose the appropriate centralized data architecture based on your organization’s needs. Consider options such as cloud data warehouses, data lakes, master data management (MDM) systems, or centralized databases. Also, evaluate factors like data volume, velocity, variety, and the complexity of analytical requirements. Data Integration and Migration: Develop a strategy for data integration and migration. Implement data integration tools, ETL processes, or your preferred method for efficient data movement. Choosing the Right Centralization Tools: Selecting the appropriate tools and technologies is critical for successful data centralization. Consider solutions that align with your organization’s specific needs, such as data warehouses,data integration platforms, or cloud-based analytics platforms. Collaborate with IT and finance teams to identify the most suitable tools that integrate seamlessly with existing systems. A well-planned selection process ensures compatibility, scalability, and security. For instance, if your organization wants to keep track of large volumes of historical data, you may opt for a data warehouse tool that can handle the storage and complex querying requirements efficiently. Ensure Data Security and Compliance: Implement robust security measures and compliance controls to protect centralized data from unauthorized access, breaches, or misuse. This is especially important as a single, centralized repository can very well turn into a single point of failure. Encrypt sensitive data, implement access controls, audit trails, and monitoring mechanisms. Establish Data Standards and Metadata Management: Next, define data standards, naming conventions, and metadata management practices to ensure consistency, and usability of centralized data. Document data definitions, lineage, and relationships to provide context and facilitate data discovery and understanding. Provide Data Access and Analytics Capabilities: Enable easy access to centralized data for users across the organization. Implement self-service analytics tools, data visualization platforms, or BI (Business Intelligence) solutions to empower users to derive insights and make data-driven decisions. Monitor and Maintain Centralized Data Infrastructure: Continuously monitor and maintain the centralized data infrastructure to ensure performance, reliability, and scalability. Monitor data quality and address issues promptly to optimize resource utilization. Iterate and Improve: Regularly review and iterate on your centralized data strategy based on the changing business requirements and technological advancements. Continuously improve processes, tools, and governance practices to maximize the value derived from centralized data. The Future of Financial Analytics: A Centralized Approach Financial institutions have traditionally relied on fragmented data sources and siloed analytics systems. However, in today’s fast-paced and data-driven environment, data centralization and integration from various sources — such as internal systems, external market data providers, and evenunstructured data, such asincome statements, cashflow statements and balance sheets — is crucial for a comprehensive view of the financial landscape. The shift from siloed to centralized financial analytics is imperative for organizations looking to thrive in the modern business landscape. Data centralization coupled with modern technology enables businesses to access comprehensive insights that drive strategic decision-making, improve financial performance, and capitalize on new opportunities. Embracing a centralized approach to financial analytics is not just a wise investment—it is a necessary step toward building a sustainable and competitive future. LIKE.TGoffers a no-code enterprise-grade solution for creating and managing automated data pipelines. The platform’s capabilities span a wide range, from reading various file sources and database providers to supporting diverse file formats and transfer protocols. With over 50 connectors, integration across popular databases like Oracle, SQL Server, and cloud platforms like AWS S3, Google Cloud, and Azure becomes seamless. Users can trust LIKE.TG to load data into various destinations, including flat files, cloud data warehouses and database destinations. Designing and scheduling dataflows for automated execution becomes straightforward with our built-in job scheduler, allowing complex task sequences to be easily visualized and implemented. Ready to see it in action? Sign up for ademoor download a14-day- free trialnow! Centerprise Can Make Your Data Centralization Process Fast and Easy – Try it Today! Automate the process using LIKE.TG Centerprise’s simple drag-and-drop interface, connect to all the popular databases and cloud platforms, design and schedule dataflows and workflows… and more – all without writing any code! Centralize All Your Data Now - 14 Day Free Trial!
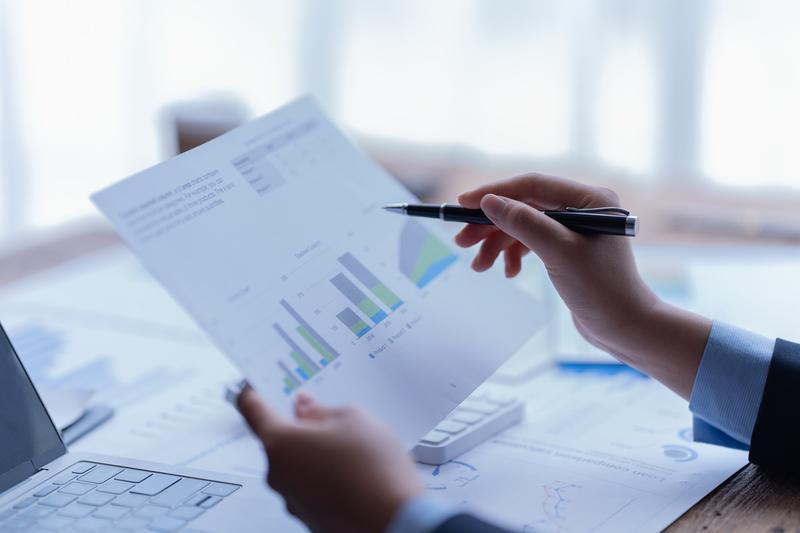
What Is Data Management? A Comprehensive Guide
What Is Data Management?Data Management DefinedData management is the process of ingesting, collecting, storing, organizing, governing, and protecting data to achieve organizational goals. It is the proactive approach organizations must take to comprehend and safeguard their data throughout its lifecycle. It also involves creating and enforcing policies and guidelines to keep data practices consistent and coherent across the organization.Managing data is more than simply handling and sorting it; it’s about implementing robust systems, or frameworks, that validate, secure, and provide a clear understanding of the information at hand. However, this does not mean that it’s just an enterprise-level concern—for that, we have enterprise data management. Even small businesses stand to enhance their revenue, productivity, and customer experience with effective data management and efficient business processes; and this is why organizations need an effective data management strategy.Why is Data Management Important?Businesses need healthy data for business intelligence (BI) and analytics, training AI and machine learning models, and other data-driven initiatives. Relying on unrefined data to power business decisions is like setting sail without a map (or a well-defined plan in this case). Think of it this way: When you have a lot of raw information—whether it’s numbers, text, or pictures—you must structure it and improve its quality before you can use it. This is precisely what data management helps organizations with; it keeps business data in order so that it’s easier to find, use, and protect.Today, businesses have several reasons to manage data effectively, particularly as they are compelled to deal with an unprecedented growth in the amount of data they create and collect. This is also why organizations resort to using data management platforms. Whether it’s to improve the performance of marketing campaigns, forecast demand, or unlock hidden opportunities for innovation, businesses need an effective framework to manage data and make the right decisions.However, if the data is managed poorly, that will mean that the requisite information is not only disorganized but also inaccurate and inaccessible to decision-makers. Relying on this data leads to wasted resources, frustrated employees, and ultimately, flawed decisions that hinder business growth.Here are some more reasons why data management is important for businesses:Effective data management streamlines processes and reduces redundancies to improve the overall efficiency of business operations.It keeps critical business data backed up and accessible for disaster recovery.It enables businesses to comply with relevant regulatory requirements, for instance, data privacy and security.Provides a standard procedure to properly archive and dispose of data and optimize data storage costs.When data is organized and accessible, different departments can work cohesively, sharing insights and working towards common goals.Craft an Effective Data Management StrategyA robust data management strategy is a prerequisite to ensuring the seamless and secure handling of information across the organization. Download this whitepaper and create an end-to-end data management strategy for your business.Data Governance vs. Data ManagementOne of the key points to remember is that data governance and data management are not the same concepts—they are more different than similar. Yet, both go hand in hand when it comes to using data to achieve organizational goals.Whereas data governance involves a broader set of activities, such as establishing policies and procedures, that guide and oversee how an organization uses its data, data management is all about its implementation. It essentially supports the overall data governance framework.The table below summarizes data governance vs. data management:Data GovernanceData ManagementFocusStrategic and organizational aspects of data.Tactical and operational aspects of data.ScopeEstablishing policies, standards, and oversight.Implementation of processes and day-to-day tasks.ObjectiveEnsure data is healthy and secure.Efficient and effective handling of data.ActivitiesPolicy creation, enforcement, and monitoring.Data collection, storage, processing, and usage.Long-term viewAligns data-driven initiatives with organizational goals.Addresses immediate data handling requirements.ExamplesDefining data ownership, access controls.Database administration, data cleansing.Learn more about data governance and management.Who Is Responsible for Managing Data in an Organization?Managing data in an organization is a collaborative effort involving several roles, each with specific areas of focus. Here’s a general breakdown of key personnel responsible for the management of data in an organization:Leadership and StrategyIn larger organizations, a chief data officer (CDO) is generally responsible for overseeing the entire data strategy. They define the vision, establish data governance policies, and ensure alignment with business goals.Governance and OversightThe data governance council, a cross-functional group with representatives from IT, business units, and data governance specialists, collaborates on defining data policies, standards, and procedures, ensuring compliance.Technical ImplementationData architects design the architecture for storing, accessing, and integrating data across different systems. They ensure scalability, security, and performance of the data management infrastructure. Database administrators (DBAs) manage and maintain the organization’s databases, ensuring smooth operation while maintaining data integrity.Data Ownership and UseData stewards are business-unit representatives who own and are accountable for the accuracy and quality of specific data sets. They understand the data needs of their department and ensure data is used appropriately. Data analysts analyze data to extract insights and inform business decisions. They may also be involved in the cleansing, transformation, and management of data. With advanced skills in statistical analysis and machine learning, data scientists develop models and algorithms to uncover hidden patterns and trends within the data, enabling more sophisticated data-driven decision making.Data SecurityThe IT security team implements security measures, access controls, and data protection protocols to safeguard sensitive information.The Data Management ProcessUnlike a one-off data migration project, data management is not a one-time process—it’s an ongoing endeavor. As businesses evolve, so does their data. Regular updates and maintenance are essential to provide data that is relevant, secure, and, most importantly, aligned with the ever-changing needs and objectives of the organization. Finally, as data ages and becomes obsolete, there are procedures for archiving or appropriately disposing of it.Here’s what the data management process generally looks like:Collecting Data: The process begins with the collection of raw data from various sources. This could be customer interactions, transactions, or any other relevant information. Once collected, the data needs a home, so it’s stored in databases, data warehouses, or other storage systems, making it easily accessible.Storing Data: This step involves selecting and maintaining the appropriate infrastructure to house your organization’s information. It also includes decisions about storage types, such as relational databases, NoSQL databases, or cloud data warehouses. Considering the volume and type of data your organization handles, you must also determine the optimal storage capacity.Structuring Data: Raw data often lacks a defined structure, so it must be structured in a way that makes sense, usually through databases with defined tables and relationships. This step also involves cleaning and transforming the data so that it’s standardized and consistent.Improving Data Quality: Data quality is fundamental to ensuring accurate and reliable information. Start by implementing robust data validation checks during the data entry phase to prevent errors. Collaborate with data owners and users to establish and enforce data quality standards. Utilize data cleansing tools and techniques to correct inaccuracies and inconsistencies.Securing Data: Protecting data from unauthorized access or loss is a critical aspect of data management, which involves implementing security measures such as encryption, access controls, and regular audits. Organizations must also establish policies and procedures to guarantee data quality and compliance.Accessing Data: This is where you establish access controls to ensure that individuals have appropriate permissions based on their roles within the organization. Implementing encryption and authentication mechanisms further helps in protecting sensitive data during transmission and storage.Consuming Data: With the data now processed and secured, organizations can perform analysis, whether it’s through statistical methods, machine learning algorithms, or other analytical tools, and make reliable business decisions.Data Management Types, Components, and FunctionsBeing a broad concept, data management can have several types of aspects, or functions, that reflect the diverse ways organizations manage data. Here’s a look at the different types of data management functions:Big Data ManagementGrowing data volumes compel organizations to invest in scalable data management solutions. Today, this means they should have the necessary resources and infrastructure to be able to deal with big data—large volumes of structured and unstructured data—efficiently. This also includes maintaining data quality while facilitating easy access to the needed data.Master Data ManagementAnother widely used strategy for managing data is master data management (MDM). It focuses on maintaining a consistent and accurate version of master data, such as customer information or product details, across an organization. MDM leads to data that remains a reliable and trustworthy asset for the organization.Database ManagementInvolves the organization, storage, and retrieval of data using database management systems (DBMS). Database management is the process of creating and maintaining databases. It allows users to manage data, maintain its integrity, and optimize performance. There are several types of DBMS, with two being the most common ones: relational databases (also called SQL databases) and non-relational databases (also called NoSQL databases). Other DBMS include object-oriented database management systems, columnar databases, graph databases, etc.Learn more about the types of databases.Data IntegrationThe goal with data integration is to consolidate data into a centralized repository. Organizations rely on scalable data pipelines to integrate their data to make reliable decisions and drive growth. Two of the most widely used data integration techniques are extract, transform, and load (ETL) and extract, load, and transform (ELT).Data Warehousing and Data LakesData warehouses and data lakes serve as essential components in the modern data management architecture.Data warehousing is all about consolidating data into a central data warehouse—or an enterprise data warehouse in case of a large organization—for various BI and analytics initiatives. Although organizations widely use ETL pipelines for this purpose, ELT has gained considerable traction lately. Data warehousing is especially important in managing data with a defined structure for streamlined data retrieval.A data lake, on the other hand, takes an unstructured approach to data storage. Unlike relational databases with predefined schemas, data lakes can store vast amounts of data in its original format, regardless of structure. This includes text documents, images, sensor data, social media feeds, and more. Data lakes are increasingly being used for big data management.Data Quality ManagementData management lays a strong emphasis on data quality management. The goal is to provide business leaders with easy access to accurate data for reliable decisions. Breaking down data silos and building a single source of truth (SSOT) are some prerequisites that organizations must do right to ensure data accuracy.Data GovernanceData governance provides strategic oversight and a framework to uphold data as a valuable asset, managing it in a way that aligns with organizational goals and industry best practices. It maintains data quality, consistency, and compliance with regulations.Data ModelingData modeling is all about creating abstract representations of how data should be organized, structured, and related within a database or information system. It serves as a blueprint that guides the design and implementation of databases and facilitates the storage and retrieval of data in a logical and efficient manner.Business Intelligence and AnalyticsModern data management systems are equipped with features and components that enable you to analyze data, generate insights, and make forecasts. Specifically, BI offers a comprehensive overview of historical and real-time data. It transforms raw data into actionable insights, equipping you to make informed decisions with a forward-looking perspective.On the other hand, analytics enables you to sift through information, identify patterns, and extract valuable details that contribute to a granular understanding of your business landscape. Together, BI and analytics form a pivotal component of your data management strategy to enhance decision-making.Explore the Best Data Management Tools and SolutionsWith so many data management tools and solutions to choose from, it's easy to get overwhelmed. Here's a list of the best data management solutions to help you make an informed decision.Learn MoreData Management BenefitsStaying competitive in a dynamic business landscape means organizations must base their decision-making on healthy data. Effective data management sets up organizations for success, here’s how:Improved Decision-MakingEffective management of data allows organizations to track and monitor various aspects of their operations. With access to a well-managed and integrated data repository, executives make more informed and timely decisions. Consequently, the increased visibility into relevant data allows for a better understanding of market trends, customer behavior, and internal performance, facilitating strategic and data-driven decision-making.Enhanced Operational EfficiencyAn effective data management strategy becomes a foundation for reliable and cohesive information by streamlining processes and workflows. This way, everyone works with the most up-to-date and accurate data. As a result, not only does operational efficiency improve, but the entire team can also respond more promptly to challenges and opportunities, contributing to an agile and responsive work environment.Improved Data SecurityThe repercussions of data loss and cyber threats continues to keep organizations on their toes. This is why managing data also extends to protecting sensitive information. With data security being a key data management component, it involves setting up access controls, encryption, and authentication mechanisms permitting only authorized individuals to access and modify data. Regular monitoring and auditing of data activities further enhance data security by detecting and addressing potential threats proactively.Better ComplianceManaging data isn’t just about keeping data organized; it’s as much about following the rules. Industry standards and regulations set clear guidelines for handling data, and following them helps organizations avoid hefty fines. But that’s not all. By handling data responsibly, organizations show they take protecting sensitive information seriously. This builds trust with stakeholders and strengthens the organization’s reputation for ethical and responsible practices.Lower CostsOrganizations with effective data management processes see a significant reduction in costs due to optimized utilization of resources. For example, businesses can substantially reduce storage costs by eliminating redundant and obsolete data, so that only relevant and valuable information is stored. Additionally, efficient data processing and retrieval mechanisms streamline operational workflows, which in turn lead to increased productivity and decreased labor costs.Simplify Data Management With LIKE.TG's Unified PlatformExplore the full breadth of LIKE.TG's unified data management platform. From unstructured data management and integration to designing APIs, to building a custom data warehouse, to EDI management—all with a consistent user interface and experience.Learn MoreData Management ChallengesWhile there are many benefits of data management, its implementation can be a complex endeavor. Here are some challenges that become obstacles when managing data in an organization:Accessing Organizational DataA significant aspect of data management involves knowing your organization’s data sources and where the business data resides. However, many companies struggle in the identifying the most critical data elements. More than 42% of tech professionals surveyed face difficulty locating organizational data.The solution is first to determine the analytics and reporting objectives. Based on these objectives, craft a comprehensive data management strategy to collect, prepare, store, and consume data efficiently. The goal should be to make organizational data accessible across the organization.Rising Data VolumesData collection has increased vastly due to the growing digitalization of information. It is pushed further by the increasing use of social media and smartphones, with IoT systems being another significant driver of big data. However, the limited storage capacity of on-premises systems is a big obstacle in data management, especially when it comes to big data. Continuously rising data volumes require additional storage space and advanced storage systems, which lead to higher costs.Many businesses move their data to the cloud to overcome for cheaper storage. Consequently, cloud-based data warehouses have become a necessity for storing large amounts of data.Consolidating Data Across SystemsTech companies use multiple information systems, including accounting software, CRM, and ERP systems. Each department usually has its own data repository, resulting in siloed systems. The contrasting systems can lead to data duplication and lack of synergy, which impact data analyses.Tip:ano-code data integration platformis the best option for preparing a centralized data warehouse.Managing Unstructured DataMore than 80% of business data is available in anunstructured format. This data contains valuable insights that can drive effective decision-making. For instance, emails from potential leads contain information, including their location, budget, and use case, which helps salespeople design more personalized and relevant demos.However, unstructured data is not machine-readable and must be structured, i.e., in columns and rows for reporting and analysis. To leverage unstructured data to their advantage, companies must use tools and technology that enable them to gather and manage data, whether it’s structured or unstructured.Maintaining Data QualityAccording to asurvey by Forrester, 80% of IT professionals cite poor-quality data as a major challenge to data management. With businesses facing an onslaught of data from various directions, many data sets inevitably contain missing or incorrect information. Leaving these issues unattended leads to misleading insights and poor decision-making. Using relevant data quality metrics helps measure data health by identifying duplicate values, missing fields, and null values.Ensuring Data SecurityRising data stores increase the risk of cyber-attacks and data leaks. Large volumes of data need protection both during rest and transmission. A data governance framework is usually the answer, however developing and implementing comprehensive data governance policies and procedures requires substantial resources. Maintaining consistency and adherence to these policies across diverse data sources and systems requires organizations to determine the sensitivity and regulatory requirements of data, manage access controls and implement encryption methods, all the while ensuring consistency in data governance practices across different departments.Handling Multiple Data SolutionsMany organizations use separate toolsfor different data management processes, such as extraction, integration, warehousing, etc. Using various data solutions leads to data silos and inefficient data management. Additionally, using multiple software has the following disadvantages:Lack of connectivity between systemsHigher total costs associated with multiple toolsEach tool has varying compliance levelsLack of consistent UIUnified data management solutions help address and overcome these challenges.Take the First Step to Unified Data Management With LIKE.TGWhether it's unstructured data management and integration, API management, building a custom data warehouse, or EDI management, streamline data management from end-to-end with LIKE.TG's 100% no-code platform. Try for Free.Download TrialData Management Best PracticesOrganizations can effectively tackle the challenges of managing data discussed above if they incorporate some best practices into their overall data strategy. Here are some data management best practices:Implement a centralized data access strategy with clear protocols for data retrieval, access control, and discovery to streamline information flow.Implement a layered security approach with encryption, access controls, and regular audits to safeguard sensitive data.Invest in scalable data storage solutions to efficiently manage growing data volumes.Incorporate effective data integration tools and processes streamline data flow and enhance interoperability.Utilize data management tools that can handle unstructured data in bulk.Establish a robust data quality management framework and processes to keep data health in check.Create a comprehensive data governance framework to ensure consistent and secure management of data across the organization.Leveraging Data Management Tools for SuccessGiven the proliferation of data and organizations’ growing need fordata democratization, leveraging data management tools is no longer optional; it’s essential for success. Amodern data management toolempowers organizations to:Tame the data deluge by organizing, cleansing, and integrating data from various sources while ensuring its accuracy, consistency, and accessibility.Break down data silos to dismantle information barriers and enable self-service analytics.Enhance data-driven decision-making at all levels by offering user-friendly interfaces that make it easier for everyone, regardless of technical expertise, to explore and analyze data.Ensure data security and compliance with built-in data governance features that protect sensitive information and guarantee adherence to data privacy regulations.How LIKE.TG Helps Businesses with its Data Management PlatformLIKE.TG provides a unified data management platform with a consistent user interface and experience.LIKE.TG – Unified Data ManagementWhether its unstructured data management, integrating data from multiple sources via ETL, ELT, or CDC, building a custom data warehouse from scratch, building and publishing APIs, or EDI management, LIKE.TG empowers its users to accomplish everything without writing a single line of code.With the ability to connect to a wide range of source and destination systems, whether they are on on-premises or in the cloud, users get a truly seamless experience when moving and integrating data across systems.Streamline your data management initiatives with a reliable partner. Get in touch with one of our data solutions experts at +1 888-77-LIKE.TG to discuss your use case. Alternatively, you can download a 14-day free trial to test our data management suite and see if it fits your requirements.Take the First Step to Unified Data Management With LIKE.TGWhether it's unstructured data management and integration, API management, building a custom data warehouse, or EDI management, streamline data management from end-to-end with LIKE.TG's 100% no-code platform. Try for Free.Download Trial
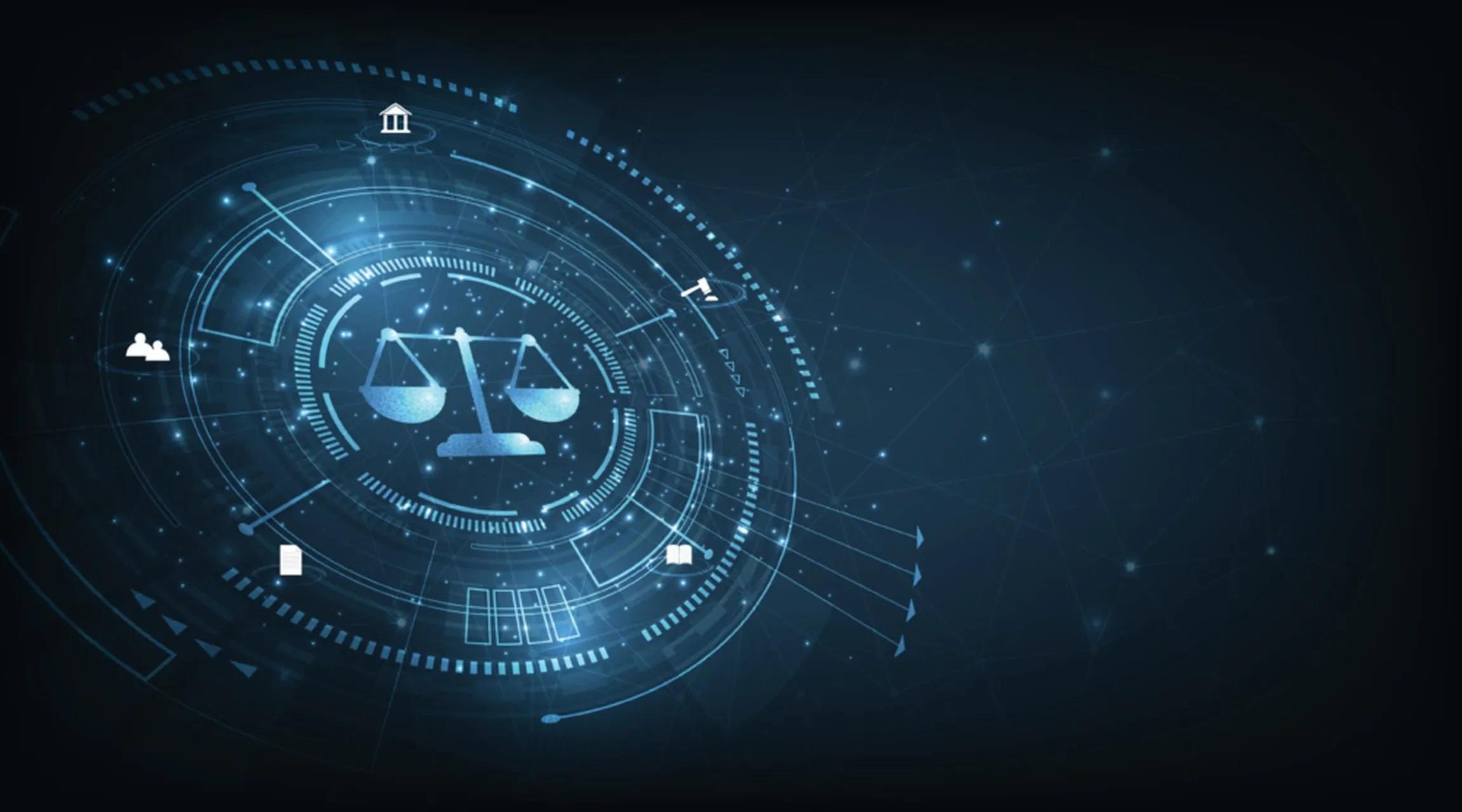
Data Vault vs. Data Mesh: Choosing the Right Data Architecture
Data volume continues to soar, growing at an annual rate of 19.2%. This means organizations must look for ways to efficiently manage and leverage this wealth of information for valuable insights. A solid data architecture is the key to successfully navigating this data surge, enabling effective data storage, management, and utilization. Enterprises should evaluate their requirements to select the right data warehouse framework and gain a competitive advantage. That’s where Data Vault and Data Mesh come into play- each offering distinct approaches to managing and leveraging data. To decide between the two, it’s essential to understand the evolving landscape of data architecture, the unique characteristics of each approach, and the practical applications that best suit specific business needs. Understanding the Modern Data Architecture Data architecture shapes how organizations collect, store, process, and leverage their data assets. It serves as the foundational framework that accommodates the diverse and ever-growing data streams originating from various sources, rendering traditional approaches obsolete and paving the way for future-ready data systems. Modern data architecture is characterized by flexibility and adaptability, allowing organizations to seamlessly integrate structured and unstructured data, facilitate real-time analytics, and ensure robust data governance and security, fostering data-driven insights. Think of data architecture as the blueprint for how a hospital manages patient information. It ensures that data from different departments, like patient records, lab results, and billing, can be securely collected and accessed when needed. In a modern data architecture, all this information is integrated into a central electronic health record (EHR) system. The EHR system simplifies data retrieval for healthcare providers, leading to faster diagnoses, streamlined billing, and better patient care while also allowing for scalability and compliance with evolving regulations. Selecting the right data architecture depends on the specific needs of a business. There is no one-size-fits-all solution, and the choice of architecture must align closely with an organization’s unique characteristics. Factors like data complexity, scalability, organizational culture, compliance obligations, available resources, and overall business goals should be considered to determine the right fit, enabling an organization to unlock the true value of its data assets. Data Vault vs Data Mesh: An Overview Now that we’ve established the importance of data architecture in today’s digital landscape let’s delve into two prominent approaches: Data Mesh and Data Vault. Data Vault: Data Vault architecture is an agile and flexible data modeling methodology used in data warehousing to handle complex and evolving data environments. It was developed by Dan Linstedt and has gained popularity as a method for building scalable, adaptable, and maintainable data warehouses. Core Principles: Hubs: Hubs represent core business entities with unique identifiers. Links: Links connect hubs to show relationships between business entities. Satellites: Satellites provide detailed, descriptive information about the entities represented by hubs. Data Vault emphasizes audibility and historical data tracking, making it well-suited for industries with regulatory compliance requirements and well-defined data structures, such as finance and healthcare. These sectors often have strict regulatory compliance requirements that demand secure storage of historical data, such as financial transactions or patient records. Data Vault’s ability to provide a clear audit trail of data sources, transformations, and usage over time ensures organizations can meet these regulatory demands effectively. Data Mesh: Data Mesh is a relatively new concept in the field of data architecture and management. It was introduced by Zhamak Dehghani and focuses on decentralizing data ownership and management in large, complex organizations. This approach is well-suited to the complexity of modern data ecosystems, where data is spread across various entities. Domain-Oriented Ownership: Data ownership is decentralized, with individual domains or business units responsible for managing their data to ensure context and expertise alignment. Data as a Product: Data is curated and delivered with clear interfaces, treating it as a valuable product that can be self-served by other teams. Self-Serve Data Infrastructure as a Platform: A shared data infrastructure empowers users to independently discover, access, and process data, reducing reliance on data engineering teams. Federated Computational Governance: Governance standards are collaboratively applied across domains, ensuring data quality, security, and compliance while allowing for domain-specific customization. Data Mesh is well-suited for industries with complex and decentralized data sources, such as e-commerce and manufacturing, because it provides a flexible framework that aligns with the diverse nature of their data streams. In these industries, data originates from various channels and often requires real-time analysis and scalability. Data Mesh’s decentralized approach empowers domain-specific teams to manage their data efficiently, ensuring data quality, adaptability, and agility to meet industry-specific challenges effectively. Data Vault vs Data Mesh: A Comparison Let’s compare the two approaches to uncover the differences and similarities between them for improved understanding: Differences: Infrastructure Data Vault typically relies on a centralized infrastructure, often involving a data warehouse or similar centralized storage system. This centralized infrastructure simplifies data integration and management but may require significant initial investment. In contrast, Data Mesh suggests a more distributed infrastructure approach, where individual domains manage data products. While this can reduce the need for a centralized infrastructure, it may necessitate investments in domain-specific tools and services. According to BARC, more than 90% of companies believe establishing domain-oriented ownership is relevant. Scalability Data Vault achieves scalability by integrating new data sources into the centralized architecture, allowing for centralized control. In contrast, Data Mesh facilitates scalability by enabling domains to scale their data products and services independently. This decentralized approach can be more flexible in handling varying data volumes and requirements across different domains. Data Ownership and Responsibility Data Vault centralizes data ownership, strongly emphasizing data lineage and traceability. In this approach, the data warehousing team is typically responsible for ensuring data quality and consistency. In contrast, Data Mesh decentralizes ownership, placing the responsibility on individual domains. However, governance remains essential in a Data Mesh approach to ensure data quality and compliance with organizational standards. Collaboration and Cross-Functionality While both approaches encourage collaboration among data professionals, Data Vault does not inherently emphasize cross-functional teams. It primarily focuses on centralized data management. Conversely, Data Mesh actively encourages cross-functional teams, promoting collaboration between data engineers, data scientists, and domain experts to ensure that data products align with business needs and goals. Use Cases Choosing between a Data Vault and a Data Mesh often depends on specific use cases. Data Vault is well-suited for scenarios that require rigorous historical tracking, data integration, and data quality assurance. It excels in situations where a centralized and structured approach to data management is necessary. In contrast, Data Mesh is particularly relevant for organizations with a distributed data landscape, where data is generated and used by multiple domains or business units. It thrives in environments where agility, autonomy, and collaboration among domain teams are essential for driving insights and innovation. Similarities: Data Integration Both Data Vault and Data Mesh address the challenge of integrating data from diverse sources within an organization. They acknowledge the need to combine data from various systems and make it accessible for analysis. Data Quality Both approaches emphasize data quality and governance. Data Vault includes mechanisms for data quality control within the centralized data repository, while Data Mesh promotes data product quality through decentralized ownership. Flexibility While they differ in their degree of flexibility, both Data Vault and Data Mesh aim to provide solutions that are adaptable to changing data requirements. Data Vault achieves this through versioning and change management, while Data Mesh relies on domain teams to adapt their data products. Data Democratization Both approaches aim to improve data accessibility and availability for users across the organization. Data Vault does this by creating a centralized repository accessible to authorized users, while Data Mesh encourages decentralized data ownership and access to foster data democratization. Use of Modern Technologies Both Data Vault and Data Mesh often leverage modern technologies such as cloud computing, containerization, and orchestration to support their respective architectures. Aspect Data Vault Data Mesh Approach A centralized approach to data warehousing, which consolidates data into a centralized repository. A decentralized approach that promotes distributed data ownership and autonomy suited for modern, distributed data ecosystems. Core Components Utilizes Hubs, Links, and Satellites to provide a structured and organized data architecture. Employs Domain Ownership and Data Products to distribute data ownership and provide agility in data management. Historical Tracking Strong emphasis on capturing and maintaining historical data changes for analytical purposes. Lesser emphasis on historical tracking, focusing more on domain-specific data products. Scalability Horizontal scalability achieved by adding data sources centrally to the existing architecture. Vertical scalability, allowing domains to scale their data products independently based on their needs by adding more resources to individual microservices or components. Flexibility Offers adaptability to evolving data sources while maintaining a consistent structure. Highly adaptable to changes in data types, sources, and business requirements. Data Ownership Centralized data ownership and control within a central data warehousing team. Decentralized data ownership, placing responsibility within individual domains or business units. Collaboration Encourages collaboration primarily within data teams. Promotes cross-functional collaboration between data professionals and domain experts. Data Governance Enforces centralized data governance and control policies. Requires domain-specific governance frameworks to maintain data quality and standards. Data Quality Emphasizes strong data quality assurance practices. Data quality can vary between domains, necessitating domain-specific efforts. Data Security Implements centralized security measures and controls. Requires domain-specific security considerations to safeguard data. Discoverability Centralized metadata management simplifies data discoverability. Domain-specific data discovery tools and processes are employed. Resource Allocation Concentrates resources on the central data warehouse and associated teams. Distributes resources across domains, necessitating careful resource planning. Adaptation to Variety Best suited for structured data, predefined schemas, and traditional data sources. Adaptable to diverse data types, sources, and unstructured data. Cultural Shift Requires limited cultural change, aligning with traditional data warehousing practices. Requires a cultural shift towards domain-oriented collaboration and ownership. Use Cases Well-suited for use cases requiring historical tracking, structured data, and centralized data management. Relevant for use cases in diverse and distributed data environments where agility, autonomy, and collaboration among domains are essential. Key Factors for Data Vault vs Data Mesh Implementation The decision to choose the right architecture depends on several factors. Some of them include: Data Complexity Data complexity encompasses various aspects, such as data types, sources, and relationships. Understanding data complexity is vital when selecting a data management approach. Data Mesh’s adaptability may be preferable for highly complex data landscapes, while Data Vault is better suited for structured and well-defined data. Organizational Culture An organization’s culture plays a significant role in its data management approach. It is crucial to assess whether it leans more centralized or decentralized and its readiness for change and experimentation. Data Vault better fits centralized cultures valuing control, while Data Mesh fosters decentralization, collaboration, and innovation. Compliance Obligations Compliance, including data privacy regulations and industry standards, substantially impacts their data management choices. It’s crucial to ensure that their approach aligns with compliance requirements. Data Vault offers centralized control and auditing for compliance-driven environments, while Data Mesh may require robust governance mechanisms to meet regulatory obligations. Cost Considerations Organizations must evaluate the overall cost implications covering software, hardware, cloud services, personnel, and ongoing maintenance expenses. They should assess which approach aligns better with the organization’s budget and financial objectives. Data Mesh’s cloud-native approach may have different cost dynamics compared to Data Vault’s traditional data warehousing model. A thorough cost analysis is pivotal in making the right choice. User Training Organizations must assess user training needs when choosing between Data Vault and Data Mesh. Each approach demands unique skill sets and workflows from data analysts, scientists, and business stakeholders. Data Mesh may require training in domain knowledge and collaboration due to its cross-functional focus, while Data Vault may necessitate expertise in traditional data warehousing and ETL processes. A study by Eckerson Group reveals that only 65% of Data Vault adopters report receiving training on the Data Vault 2.0 solution, highlighting a potentially critical gap and the significance of user training. Overall Business Goals An organization’s business goals should serve as a guiding principle in its data management approach. The organization must determine whether it aims for efficiency, agility, innovation, or a combination of these factors. Data Vault is well-suited for efficiency and structured reporting, while Data Mesh aligns with innovation and rapid adaptation to changing business needs. Can Data Vault and Data Mesh Co-exist? Data Vault and Data Mesh are not mutually exclusive; instead, they can be used together to create a robust data architecture. These two concepts address different aspects of data management and can be used in tandem to manage modern data ecosystems effectively. While Data Vault primarily focuses on the technical aspects of data organization, Data Mesh emphasizes the organizational and cultural aspects of effective data management. They can coexist by serving different but complementary roles within the organization’s data management strategy. For instance, an organization might employ a Data Vault to consolidate and manage structured data from multiple sources within a centralized data warehouse. Concurrently, it could embrace Data Mesh principles for handling decentralized, domain-specific data sources that don’t neatly fit into the centralized warehouse model. This hybrid approach offers organizations the flexibility and scalability needed to manage both structured and unstructured data while optimizing data quality, accessibility, and governance across the organization. A Final Word The choice between Data Vault vs Data Mesh, or a combination of both is about tailoring the data strategy to an organization’s unique needs. Data Vault brings structure and governance to your data, ensuring reliability and consistency. On the other hand, Data Mesh introduces agility and decentralization, allowing for flexibility in managing diverse data sources. It’s not an either-or decision, but rather finding the right blend that suits your specific requirements. Striking this balance empowers organizations to harness the power of their data, not only to meet their immediate needs but also to navigate the ever-evolving data landscape with confidence, ultimately achieving their long-term objectives. When it comes to finding the right data architecture, LIKE.TG stands out as a trusted provider. It offers a unified, metadata-driven approach, making it the go-to choice for organizations looking to efficiently build, manage, and optimize their data warehousing architecture. With LIKE.TG’s no-code solution, businesses can easily design, develop, and deploy high-volume data warehouses in days, enabling them to stay ahead in today’s data-driven landscape. Learn more about how LIKE.TG Data Warehouse Builder simplifies data management! Build Your Data Warehouse Effortlessly With a 100% No-Code Platform Build a fully functional data warehouse within days. Deploy on premises or in the cloud. Leverage powerful ETL/ELT pipelines. Ensure data quality throughout. All without writing a single line of code. Learn More!
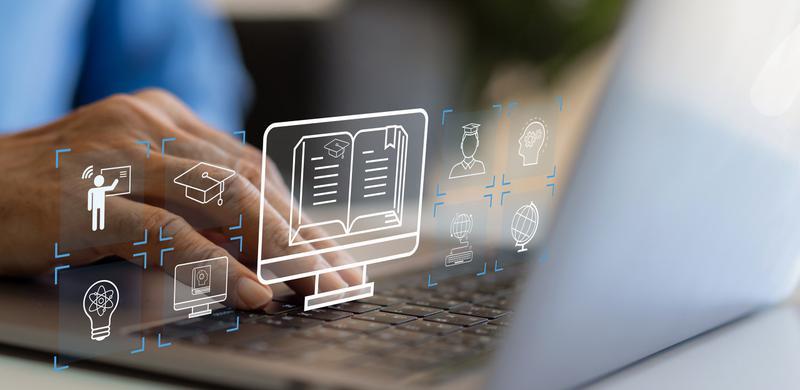
Building data pipelines in Python—Why is the no-code alternative better?
Python’s versatility, intuitive syntax, and extensive libraries empower professionals to construct agile pipelines that adapt to evolving business needs. Python seamlessly automates workflows, manages complex transformations, and orchestrates smooth data movement, creating a foundation for efficient and adaptable data processing in diverse domains. Data Pipelines in Python A data pipeline is a set of automated procedures that facilitate the seamless flow of data from one point to another. The primary objective of a data pipeline is to enable efficient data movement and transformation, preparing it for data analytics, reporting, or other business operations. Python is widely used in the creation of data pipelines due to its simplicity and adaptability. A data pipeline in Python is a sequence of data processing elements, where each stage takes data from the previous stage, performs a specific operation, and passes the output to the next stage. The primary objective is to extract, transform, and load (ETL) data from various sources and in various formats into a single system where it can be analyzed and viewed together. Python data pipelines are not limited to ETL tasks. They can also handle complex computations and large volumes of data, making them ideal for: Data cleaning Data transformation Data integration Data analysis Python’s simplicity and readability makes these pipelines easy to build, understand, and maintain. Furthermore, Python offers several frameworks like Luigi, Apache Beam, Airflow, Dask, and Prefect, which provide pre-built functionality and structure for creating data pipelines, which can speed up the development process. Key Advantages of Building Data Pipelines in Python Flexibility: Python’s extensive range of libraries and modules allows for a high degree of customization. Integration Capabilities: Python can seamlessly integrate with various systems and platforms. Its ability to connect to different databases, cloud-based storage systems, and file formats makes it a practical choice for constructing data pipelines in varied data ecosystems. Advanced Data Processing: Python’s ecosystem includes powerful data processing and analysis libraries like Pandas, NumPy, and SciPy. These libraries allow for complex data transformations and statistical analyses, enhancing the data processing capabilities within the pipeline. Python Data Pipeline Frameworks Python data pipeline frameworks are specialized tools that streamline the process of building, deploying, and managing data pipelines. These frameworks provide pre-built functionalities that can handle task scheduling, dependency management, error handling, and monitoring. They offer a structured approach to pipeline development, ensuring that the pipelines are robust, reliable, and efficient. Several Python frameworks are available to streamline the process of building data pipelines. These include: Luigi: Luigi is a Python module for creating complex pipelines of batch jobs. It handles dependency resolution and helps in the management of a workflow, making it easier to define tasks and their dependencies. Apache Beam: Apache Beam offers a unified model that allows developers to construct data-parallel processing pipelines. It caters to both batch and streaming data, providing a high degree of flexibility. This adaptability makes Apache Beam a versatile tool for handling diverse data processing needs. Airflow: Airflow is a systematic platform that defines, schedules, and monitors workflows. It allows you to define tasks and their dependencies and takes care of orchestrating and monitoring workflows. Dask: Dask is a versatile Python library designed to perform parallel computing tasks with ease. It allows for parallel and larger-than-memory computations and integrates well with existing Python libraries like Pandas and Scikit-Learn. Prefect: Prefect is a modern workflow management system that prioritizes fault tolerance and simplifies the development of data pipelines. It provides a high-level, Pythonic interface for defining tasks and their dependencies. How to Build Python Data Pipelines: The Process Let’s examine the five essential steps of building data pipelines: 1. Installing the Required Packages Before you start building a data pipeline using Python, you need to install the necessary packages using pip, Python’s package installer. If you’re planning to use pandas for data manipulation, use the command “pip install pandas”. If you’re using a specific framework like Airflow, you can install it using “pip install apache-airflow”. 2. Data Extraction The first step is to extract data from various sources. This can involve reading data from databases, APIs, CSV files, or web scraping. Python simplifies this process with libraries like ‘requests’ and ‘beautifulsoup4’ for web scraping, ‘pandas’ for CSV file reading, and ‘psycopg2’ for PostgreSQL database interaction. 3. Data Transformation Once the data is extracted, it often needs to be transformed into a suitable format for analysis. This can involve cleaning the data, filtering it, aggregating it, or performing other computations. The pandas library is particularly useful for these operations. Notably, you can use `dropna()` to remove missing values or `groupby()` to aggregate data. 4. Data Loading After the data has been transformed, it is loaded into a system where it can be analyzed. This can be a database, a data warehouse, or a data lake. Python provides several libraries for interacting with such systems, including ‘pandas’ and ‘sqlalchemy’ for writing data to an SQL database and ‘boto3’ for seamless interaction with Amazon S3 in the case of a data lake on AWS. 5. Data Analysis The final stage is analyzing the loaded data to generate insights. This can involve creating visualizations, building machine learning models, or performing statistical analysis. Python offers several libraries for these tasks, such as `matplotlib` and `seaborn` for visualization, `scikit-learn` for machine learning, and `statsmodels` for statistical modeling. Throughout this process, it’s important to handle errors and failures gracefully, ensure data is processed reliably, and provide visibility into the state of the pipeline. Python’s data pipeline frameworks, such as Luigi, Airflow, and Prefect, provide tools for defining tasks and their dependencies, scheduling and running tasks, and monitoring task execution. The No-Code Alternative to Building Python Data Pipelines Python, while offering a high degree of flexibility and control, does present certain challenges: Complexity: Building data pipelines with Python involves handling various complex aspects such as extracting data from multiple sources, transforming data, handling errors, and scheduling tasks. Implementing these manually can be a complex and time-consuming process. Potential for Errors: Manual coding can lead to mistakes, which can cause data pipelines to fail or produce incorrect results. Debugging and fixing these errors can also be a lengthy and challenging process. Maintenance: Manually coded pipelines often require extensive documentation to ensure they can be understood and maintained by others. This adds to development time and can make future modifications more difficult. The process of building and maintaining data pipelines has become more complex. Modern data pipeline tools are designed to handle this complexity more efficiently. They offer a level of flexibility and adaptability that is difficult to achieve with traditional coding approaches, making data management more inclusive, adaptable, and efficient While Python remains a versatile choice, organizations are increasingly adopting no-code data pipeline solutions. This strategic shift is driven by the desire to democratize data management, foster a data-driven culture, ensure data governance, and streamline the pipeline development process, empowering data professionals at all levels. Advantages of Using No-Code Data Pipeline Solutions Opting for an automated solution for no-code data pipelines presents several advantages such as: Efficiency: No-code solutions expedite the process of building data pipelines. They come equipped with pre-built connectors and transformations, which can be configured without writing any code. This allows data professionals to concentrate on deriving insights from the data rather than spending time on pipeline development. Accessibility: No-code solutions are designed to be user-friendly, even for non-technical users. They often feature intuitive graphical interfaces, enabling users to build and manage data pipelines through a simple drag-and-drop mechanism. This democratizes the process of data pipeline creation, empowering business analysts, data scientists, and other non-technical users to construct their own pipelines without needing to learn Python or any other programming language. Management and Monitoring Features: No-code solutions typically include built-in features for monitoring data pipelines. These may include alerts for pipeline failures, dashboards for monitoring pipeline performance, and tools for versioning and deploying pipelines. Leveraging LIKE.TG’s No-Code Data Pipeline Builder LIKE.TG is a no-code solution that’s transforming the way businesses handle their data. The advanced data integration platform offers a comprehensive suite of features designed to streamline data pipelines, automate workflows, and ensure data accuracy. Here’s a look at how LIKE.TG stands out: No-Code Environment: LIKE.TG’s intuitive drag-and-drop interface allows users to visually design and manage data pipelines. This user-friendly environment reduces dependency on IT teams and empowers non-technical users to take an active role in data management, fostering a more inclusive data culture within the organization. Wide Range of Connectors: LIKE.TG comes equipped with pre-built connectors for various data sources and destinations. These include connectors for databases like SQL Server, cloud applications like Salesforce, and file formats like XML, JSON, and Excel. This eliminates the need for complex coding to establish connections, simplifying the data integration process. Pre-Built Transformations: LIKE.TG provides a wide array of data transformation functions. These include transformations for merging, routing and pivoting/unpivoting among others. These operations enable users to cleanse, standardize, and enrich data as per their business requirements, ensuring that the data is in the right format and structure for analysis. Data Quality Assurance: LIKE.TG offers advanced data profiling and data quality features. Users can set predefined rules and check data against these rules to ensure its accuracy and reliability. This feature helps maintain data integrity, ensuring that your business decisions are based on high-quality data. Job Scheduling and Automation: The platform allows users to schedule jobs and monitor their progress and performance. Users can set up time-based or event-based triggers for tasks, automating the data pipeline process and ensuring the timely execution of data jobs. LIKE.TG’s No Code Platform Take the first step towards efficient and accessible data management. Download your 14-day free trial of LIKE.TG Data Pipeline Builder and start building pipelines without writing a single line of code!
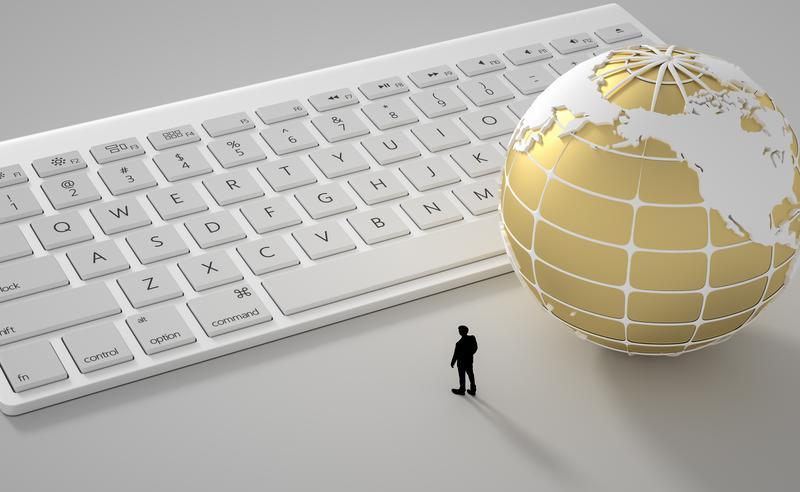
Airbyte vs Fivetran vs LIKE.TG: Which is the Better Solution in 2024?
With close to 200 different solutions for data integration out there, choosing the right one is like stepping into a bustling market with a myriad of vendors—each promising seamless connectivity. Except, you have to do your own due diligence to identify the solution that caters to your organization’s needs most effectively. So, in this article, we’re going to compare Airbyte vs Fivetran vs LIKE.TG, three of the best data integration tools, to help you make an informed decision. Airbyte vs Fivetran vs LIKE.TG: Overview Airbyte Finally, Airbyte is primarily an open-source data replication solution that leverages ELT to replicate data between applications, APIs, data warehouses, and data lakes. Being an open-source solution means users can customize and extend their pipelines. Like other data integration platforms, Airbyte features a visual UI with built-in connectors. Fivetran Fivetran is a cloud-based data movement platform that relies solely on ELT to move data between two systems. It’s an automated data synchronization solution that syncs data from different databases, APIs, applications, and other structured data sources into data warehouses and lakes for analytical purposes. Fivetran can be an ideal solution for businesses that only need to move data between sources and destinations without massively transforming it. LIKE.TG LIKE.TG is an end-to-end data integration and management platform that features a 100% no-code UI that delivers a consistent user experience across its suite of applications. The result? A simple, easy-to-use platform that allows all users, regardless of their technical expertise, to build fully automated data pipelines based on ETL or ELT. These data pipelines enable users to establish native connectivity to a wide range of endpoints, including files such as PDFs, XML, Excel, etc., databases and data warehouses, whether on-premises or in the cloud, data lakes, cloud storages, web applications, as well as data visualization and BI tools, consolidating their company-wide data. Manage All Your Data in a Single, Unified Data Management Platform Empower your data journey with LIKE.TG Data Stack—a comprehensive suite of solutions! Whether it's unstructured data management, data integration, API management, managing EDI workflows, or building a bespoke data warehouse from the ground up, LIKE.TG has you covered. Learn More Comparing Airbyte vs Fivetran vs LIKE.TG LIKE.TG Fivetran Airbyte Data Integration Uses both ETL and ELT to integrate data across the organization. Also supports change data capture (CDC) for replication. Does not offer ETL. Instead, uses ELT to ‘move data’ between systems. Also supports CDC for replication. Does not offer ETL, instead uses ELT to sync data across applications, databases, etc. Also supports CDC for replication. Data Warehousing Offers the ability to build a fully functional data warehouse from scratch without writing a single line of code in addition to supporting native connectivity to popular data warehouses. Fivetran’s data warehousing is limited to offering connectivity to popular data warehouses only. Airbyte’s data warehousing is limited to offering connectivity to popular data warehouses only. API Management Apart from the ability to connect to and integrate APIs, LIKE.TG offers a single platform that caters to the entire API lifecycle—from design to publishing. API management is not offered by Fivetran. Instead, it requires integration with external services in order to enable users to design, share, and test API requests. Only offers Airbyte API, that provides an interface to users to “programmatically” control the Airbyte platform. Does not offer API management. Unstructured Data Extraction LIKE.TG offers a complete data extraction solution capable of extracting not only structured data but also semi-structured and unstructured data, including PDFs, invoices, reports, etc., that too in bulk. LIKE.TG leverages AI to simplify and accelerate unstructured data extraction. Fivetran only replicates structured data. It does so by understanding the source data structure and mapping it to a destination schema of tables and columns. Although it has only recently started extracting text from documents, Airbyte does not offer full-fledged unstructured data management. Additionally, Airbyte users can extract text from documents stored only on Amazon S3 and Google Drive. EDI Management LIKE.TG has a dedicated platform for no-code EDI management. No support for EDI files or management whatsoever. No support for EDI files or management whatsoever. Connectors and Integrations Offers native, bidirectional connectors for a range of sources and destinations, including files, databases, data warehouses, data lakes, cloud storages, and web applications. Offers connectors for files, applications, databases, data warehouses. Fivetran’s website states that support for data lakes is in development. Like LIKE.TG and Fivetran, Airbyte also offers a long list of connectors. However, most of its source connectors are either in beta or pre-beta. Custom Connectors Along with native connectors for popular sources and destinations, LIKE.TG offers the ability to build custom connectors seamlessly. Fivetran offers custom connector development through cloud functions. It also offers its “By Request” program, albeit only for Lite connectors. Users can use Airbyte Connector Development Kit for custom connector development. However, they need technical expertise to build them. Data Quality LIKE.TG offers comprehensive data quality features embedded into its platform. Together, they ensure data accuracy, reliability, and completeness. Being an ELT-based platform, Fivetran does not provide any dedicated data quality features. Airbyte does not offer any built-in data quality features. Instead, it relies on external services. Transformations LIKE.TG offers a plethora of pre-built transformations. There’s no need to integrate with dbt, or any other service, to transform data. Since it’s an ELT tool, the Fivetran relies on dbt heavily to transform data. Being a data replication solution, Airbyte also relies heavily on external services for data transformation. Customer Support LIKE.TG has won several awards for outstanding customer support, highlighting its commitment to supporting all its customers 24/7. Although Fivetran claims to provide 24/7 support, some popular forums, such as reddit, state that the quality of customer support is not up to the industry standards. Airbyte provides enterprise-level support only to its enterprise customers. Build Data Pipelines Effortlessly With LIKE.TG’s Automated Data Integration Solution Building and maintaining ETL/ELT pipelines does not have to be complex and time-consuming. Leverage LIKE.TG's 100% no-code solution for seamless data integration. Get Your Free Trial! Airbyte vs Fivetran vs LIKE.TG: Data Integration Airbyte Airbyte is primarily a data replication tool that enables its users to extract data from their source systems and load it into their target systems. In other words, Airbyte facilitates data extraction and loading between systems, with transformation being optional and only possible, via external services or integrations, after the data is loaded into the destination system. Therefore, Airbyte can be a good solution for businesses that only need to sync data between their systems without substantially transforming it. It is certainly not the solution of choice for organizations looking for an end-to-end data integration solution, especially those that frequently need to transform their data before moving it to their data warehouses or lakes. Fivetran Similar to Airbyte, Fivetran lacks broader data management capabilities as it relies on ELT to “move” data between systems. Put simply, the platform’s focus is on moving data from a data source to a target destination as quickly as possible. This means that the data can only ever be transformed once it’s in the destination system. While this can be beneficial in some scenarios, for example, for SaaS companies that want to analyze data from their applications, the solution is far from ideal for large enterprises that need to transform and integrate all their data to achieve a single source of truth. LIKE.TG Whereas Fivetran and Airbyte rely on ELT to replicate and sync data, LIKE.TG uses both the ETL and ELT data integration techniques to integrate data in the true sense. With LIKE.TG, users have the flexibility of choosing between either, depending on their requirements. LIKE.TG’s pre-built transformations simplify and accelerate the ETL pipeline development process significantly, enabling users to take charge of their own data integration projects without relying too much on IT. TL;DR: If your business use case requires that you transform your data before loading it into the destination system, consider LIKE.TG. On the other hand, if the sequence of data transformation is not a major factor, it ultimately comes down to how much of a hassle your data teams are willing to get into and your budget. While both Fivetran and Airbyte support post-loading data transformation, you’ll have to use dbt, which can increase your costs significantly, especially if you have multiple developers working on your data integration pipelines. With LIKE.TG, however, there’s no need for any sort of external integrations for data transformation. Airbyte vs Fivetran vs LIKE.TG: Data Warehousing Airbyte As far as data warehousing is concerned, Airbyte only supports it in terms of providing connectivity to sources and destinations. This means that while Airbyte users can extract and load data into data warehouses supported by the platform, there’s no way to create a custom data warehouse using the platform. Fivetran Fivetran, being a data movement platform, majorly supports data warehouses as destinations. This means it doesn’t support all major data warehouses as sources, which can be a significant downside for many potential customers. LIKE.TG Not only does LIKE.TG offer native, bi-directional connectors for major data warehouses, but it also provides a platform to build a custom data warehouse from scratch without writing a single line of code. The user-friendly and intuitive UI keeps the process simple for business and technical users alike. TL;DR: If your business requirements are limited to loading data into a data warehouse, then it’s mostly a question of how effectively and efficiently a solution caters to them. However, if you anticipate building a data warehouse in the future, choose LIKE.TG. Build a Custom Data Warehouse Within Days—Not Months Building a data warehouse no longer requires coding. With LIKE.TG Data Warehouse Builder you can design a data warehouse and deploy it to the cloud without writing a single line of code. Learn More Airbyte vs Fivetran vs LIKE.TG: Data Quality Airbyte Unlike other data integration tools, like LIKE.TG, Airbyte does not provide built-in functionality for data quality management. Instead, it relies on external services, such as dbt and re_data, to identify data quality issues on data ingestion pipelines. In some instances, users need to use multiple different dbt packages to transform their data the way they want, which can quickly become tedious. Fivetran Fivetran also relies on dbt for data transformation and, therefore, any improvements to data quality. The fact that it’s a simple data movement platform means that its users can only improve the quality of their data sets once they are in the destination systems. While this may appeal to some users, this separation is not always an ideal solution. For instance, some data transformations require complex logic or intricate operations that are more efficiently handled closer to the source during the extraction or loading phase. Additionally, post-loading transformations consume resources in the destination system, which might lead to increased costs, especially when dealing with large data sets. LIKE.TG In contrast to both Airbyte and Fivetran, LIKE.TG offers comprehensive data quality features built right into its platform. This means that there’s no requirement for any external integrations to improve data quality. Users can easily clean, validate, and standardize data, apply custom data quality rules to their data sets even at the record level, and get insights into data quality issues via data profiling and by creating detailed logs for erroneous records. TL;DR: If your organization requires improving data quality before loading data into its enterprise data warehouse, opt for a data management solution with built-in data quality management, such as LIKE.TG. Airbyte vs Fivetran vs LIKE.TG: Customer Support and Documentation Airbyte Airbyte offers varying levels of customer support for its offerings—community, professional, and enterprise. Airbyte’s users that opt for open-source version are mostly on their own when it comes to fixing bugs and finding workarounds. It offers its enterprise-level support only to its enterprise customers. Airbyte only offers limited support hours, i.e., 12 hours per day, 5 days a week. While Airbyte does maintain documentation, it’s certainly not amongst the more extensive ones. Fivetran Compared to Airbyte, Fivetran offers 24/7 support for all its customers via a dedicated “team of technical specialists.” Fivetran support is available via email, call, and live chat. Additionally, Fivetran claims 1 hour response time for its customers using either the Standard, Enterprise, or Business Critical plans. However, Fivetran users on popular forums like Reddit frequently report subpar customer support from Fivetran. Fivetran also maintains documentation on its website that can help users with their basic questions. LIKE.TG LIKE.TG is recognized for its outstanding customer support and has consistently received awards for its relentless pursuit of customer satisfaction. It offers the same level of 24/7 customer support to all its customers. Users can get support over the phone, via email, as well as through live chat. LIKE.TG also provides additional on-demand training highly tailored to its customers’ most immediate needs. Additionally, business users can benefit from LIKE.TG’s Data Academy and hone their data skills as they continue to use its products. LIKE.TG’s documentation covers its entire suite of products, their functionalities, and use cases in detail. TL;DR: With the growing reliance on third-party data integration solution providers, organizations expect proactive customer support. This means timely responses to queries, proactive issue resolution, and a customer-centric approach that aligns with their evolving needs. While all three offer varying levels of customer support, LIKE.TG stands out with its unwavering commitment to customer satisfaction, proven by its award-winning track record. Airbyte vs Fivetran vs LIKE.TG: Pricing Airbyte Airbyte’s open-source version is free for basic use cases with limited volume and features, but users must pay for the infrastructure needed to operationalize the connectors. For Airbyte Cloud, the company uses volume-based pricing, which depends on the number of rows and the amount of data replicated. Finally, Airbyte Self-Managed will have users paying for support and enterprise features while hosting Airbyte on their own infrastructure. Calculating the actual total cost of using Airbyte can be very difficult, given its use of “credits.” One credit costs $2.5; however, it’s not immediately clear how many rows or how much data synced from what kind of source will lead to the consumption of 1 credit. Fivetran Compared to Airbyte, and in addition to its free, albeit basic, version, Fivetran uses a different pricing mechanism—MAR or monthly active rows. MARs are, in fact, the unique identifiers, or primary keys, that Fivetran uses to track transfers from a user’s source system to their destination each month. These keys are counted separately for each account, destination, connector, and table. This means that users are charged for monthly distinct primary keys that are inserted, updated, or deleted via its connectors. Additionally, Fivetran has multiple pricing plans, which vary by the number of connectors, the security and governance features, and sync frequency. Once again, the problem here is the formidable challenge of accurately estimating the actual cost of using the platform. LIKE.TG In contrast to Airbyte and Fivetran, LIKE.TG offers 100% transparency when it comes to pricing. It follows an annual license-based pricing model where the customers are fully aware of how much they will be charged. The cost of this license mostly depends on the number of connectors, the computing requirements, and number of users. The license can be renewed every year. TL;DR: If you are associated with a small business with a limited number of rows and amount of data to integrate, volume-based pricing can be very cost-effective as long as you continue to monitor your usage. However, if you have multiple sources and destinations with frequent data movement, volume-based pricing can easily get out of hand. In this case, considering a solution with more scalable and predictable pricing, such as flat-rate or subscription-based options, will certainly be more economical. Manage All Your Data in a Single, Unified Data Management Platform Empower your data journey with LIKE.TG Data Stack—a comprehensive suite of solutions! Whether it's unstructured data management, data integration, API management, managing EDI workflows, or building a bespoke data warehouse from the ground up, LIKE.TG has you covered. Learn More The LIKE.TG Advantage Compared to Airbyte and Fivetran, LIKE.TG offers a unique value proposition to businesses of all sizes. It’s an end-to-end data platform that caters to multiple aspects of data integration and management, covering diverse use cases. On the other hand, Airbyte and Fivetran only cater to a few use cases, all of which are based on ELT. From data integration to API management to building a custom data warehouse, LIKE.TG provides a unified and 100% no-code platform for all your data management needs. Its ability to transform data and improve its quality without requiring any further integrations significantly simplifies the process of building data pipelines. All of this is complemented by LIKE.TG’s award-winning customer support. If you’re looking to implement an end-to-end data integration solution for your organization, try LIKE.TG. Download a 14-day free trial or sign up for a demo.
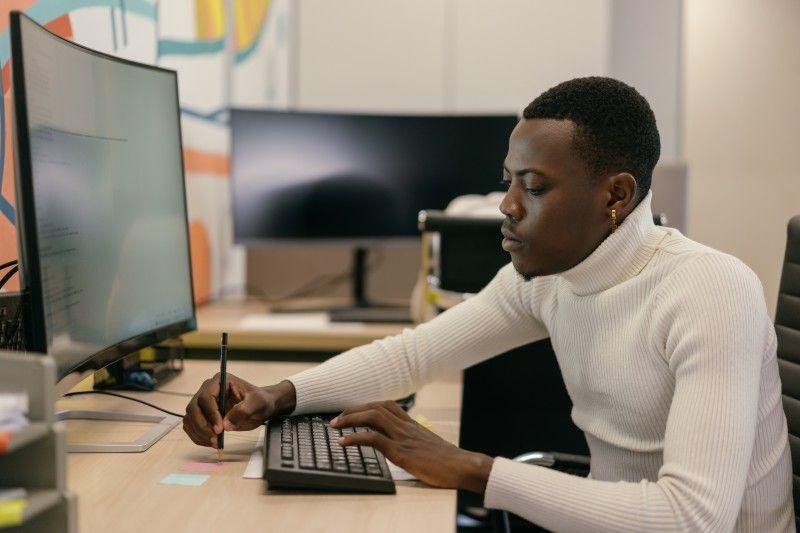
How to Implement an API Strategy in 2024
Every customer interaction, from an online click to an in-store purchase is part of the web of customer data. This web is a rich source of insights into customer behavior, preferences, and needs. The key to untangling this is Customer 360, or gaining a comprehensive view of the customer journey. According to Gartner, Inc., only 14% of organizations have successfully achieved a 360-degree view of their customers even though successfully implementing Customer 360 can empower businesses to deliver personalized experiences, foster customer loyalty, and drive growth. What is Customer 360 and Why it Matters Customer 360 is a strategic business approach that involves integrating and consolidating customer data from various departments and touchpoints within an organization to create a unified and comprehensive customer profile. Consider each department within an organization as a piece of a puzzle. Marketing holds demographic and behavioral data, sales manages contact details and lead interactions, and customer support handles service requests. Individually, these pieces offer limited insights, much like separate puzzle pieces that don’t reveal the full picture. A customer 360 approach, however, connects and integrates these various data sets, fitting them into place to create a single source of truth. By breaking down data silos, it helps businesses understand the entire customer journey, enabling personalized experiences, informed decision-making, and improved collaboration across departments. Customer 360 Use Cases and Real-World Applications Personalized Marketing Customer 360 allows businesses to tailor their marketing efforts to individual customers, enhancing the effectiveness of marketing campaigns and improving customer engagement. For example, Spotify uses a 360-degree view of its customers listening habits, preferences, and search history to curate personalized playlists. This enhances user engagement and satisfaction. Enhanced Customer Service By providing a comprehensive view of a customer’s history with the company, Customer 360 enables customer service teams to offer personalized and effective service. Predictive Analytics Using a holistic view provides a wealth of data that can be analyzed to predict future customer behavior and trends. Netflix, for example, uses predictive analytics to recommend shows and movies based on a user’s viewing history and preferences. This strategy has proven to be highly effective, with 80% of Netflix watches sprouting from its recommendation engine. Improved Sales Strategies With insights from a 360 view of data, sales teams can identify potential upsell or cross-sell opportunities. Amazon, the global e-commerce giant, analyzes a customer’s purchase history and browsing behavior to suggest relevant products. One of their most successful features is the “Frequently bought together” recommendation. This data-driven approach allows Amazon to cross-sell and upsell products that a customer is likely to be interested in, leading to increased sales and customer satisfaction. Customer Retention This approach allows businesses to track a customer’s entire journey and experience with the company, revealing potential issues or areas of dissatisfaction. Sephora, the beauty retailer, tracks purchase history and product preferences to offer personalized product recommendations and rewards, increasing customer loyalty. Campaign Optimization Customer 360 enables businesses to optimize their marketing campaigns based on comprehensive customer insights. A great example of this is the “Share a Coke” campaign by Coca-Cola. The company leveraged a 360-degree view of customer data to discover that personalized bottles were a hit with consumers. Consequently, they expanded the range of names on bottles and emphasized personalization in their marketing, which led to a 2% rise in U.S. sales. Customer 360 Tools and Technologies These tools and technologies are designed to aggregate, integrate, and analyze customer data from multiple sources to create a comprehensive and unified view of each customer. These tools play a crucial role in enhancing customer experiences, improving customer engagement, and supporting data-driven decision-making. Here are some key technologies commonly used in building and implementing Customer 360 solutions: Customer Relationship Management (CRM) Systems CRM systems are software platforms designed to manage and analyze customer interactions throughout their lifecycle. These platforms serve as comprehensive hubs for customer data, consolidating information such as contact details, purchase history, and communication preferences. CRM systems optimize customer relationships, streamline sales processes, and enhance overall customer service. By offering a unified perspective on customer interactions, CRMs empower organizations to make informed decisions that positively impact customer engagement and satisfaction. Examples of CRM systems include Salesforce, HubSpot, and Microsoft Dynamics 365. Customer Data Platforms (CDPs) CDPs, as specialized tools, concentrate on creating a consolidated and unified customer profile by integrating data from diverse sources. These platforms are specifically tailored to centralize customer data and provide a holistic view of interactions and behaviors. CDPs excel in delivering personalized marketing campaigns and refining customer segmentation based on a thorough understanding of individual preferences. Unlike general-purpose CRM systems, CDPs are laser-focused on optimizing the use of customer data for targeted and effective marketing efforts. Examples of CDPs include Segment, Tealium, and Exponea. Data Integration Platforms Data integration platforms play a foundational role in unifying data from various sources, encompassing departments like sales, marketing, and customer support. These platforms leverage ETL processes or middleware to harmonize and consolidate data, as well as eliminate data silos. By ensuring a seamless flow of information across the organization, data integration platforms contribute to a unified and coherent dataset. Examples of data integration platforms include Informatica, Talend, and LIKE.TG. Implementing Customer 360 Implementing a customer 360 view is a multi-step process that enables businesses to gain a comprehensive understanding of their customers to enhance customer experiences and drive sustainable growth. Here’s a step-by-step guide to help you successfully implement Customer 360 in your organization: Identify Data Sources: Start by identifying all potential sources of customer data within your organization. This could include CRM systems, sales data, customer service interactions, social media engagement, website analytics, and email marketing responses. Collect Data: After identifying your data sources, gather the customer data. This should include demographic details, transaction history, communication records, online behavior, customer feedback, and any other relevant data that provides insight into the customer’s relationship with your business. Integrate Data: Next, integrate the collected data into a centralized system. This involves consolidating data from different sources and ensuring uniformity in its format. A unified view is pivotal for a comprehensive understanding of each customer. Implement Customer Identity Resolution: This critical step involves accurately linking each piece of customer data to the respective individual customer. Advanced algorithms are used to match these diverse data points, effectively consolidating the scattered data into a unified view of each customer’s journey. Analyze Data: With a complete and accurate picture of each customer, analyze this data using visualization tools like PowerBI and Tableau to find patterns and trends. This helps to understand customer behavior and preferences, turning raw data into meaningful insights. The insights derived from the data analysis are then leveraged to inform and shape business strategies. This could involve personalizing marketing efforts, enhancing customer service, or tailoring products or services to meet the specific needs and preferences of the customers. Creating a Comprehensive Customer 360 View with LIKE.TG When unified and analyzed, the wealth of customer data forms a comprehensive Customer 360 view, offering invaluable insights into customer behavior, preferences, and needs. However, the key to unlocking these insights lies in effectively breaking down data silos and integrating this scattered data into a cohesive, actionable view. LIKE.TG, a leading provider of data integration and management solutions, simplifies this process. With its robust capabilities and user-friendly interface, LIKE.TG streamlines the creation of a customer 360 view, turning complex data into clear insights. Here’s how LIKE.TG facilitates the process: 1. Data Collection: LIKE.TG enables the seamless collection of data from a wide array of sources, including CRM systems, social media platforms, customer feedback, and transactional data. With its extensive range of connectors, including database, cloud, CRM, ERP, and file connectors, LIKE.TG ensures seamless data extraction. Data Sources in LIKE.TG 2. Data Profiling: LIKE.TG allows businesses to profile their customer data to identify trends, patterns, and anomalies. This process aids in understanding customer behavior and predicting future trends. Data Profiling in LIKE.TG 3. Data Quality Assurance: LIKE.TG prioritizes the accuracy and reliability of the data. It offers features for data cleansing, validation, and deduplication, ensuring the data used to build the Customer 360 view is accurate, consistent, and up-to-date. Defining data validation rules in LIKE.TG 4. Data Transformation: LIKE.TG provides tools for mapping, transforming, and converting data types and formats. This includes transformations like aggregation, filtering, normalization, and pivoting/unpivoting, which help convert raw data into a format suitable for analysis and interpretation, facilitating the extraction of meaningful insights. Sample Transformations in LIKE.TG 5. Data Integration: LIKE.TG employs various techniques, such as merging, joining, and reconciling, to seamlessly combine and consolidate data from diverse sources and build a unified and cohesive dataset. Combining datasets using Join transformation in LIKE.TG 6. Data Loading: LIKE.TG’s robust data loading capabilities support high-volume data loading from various sources into a central repository such as a data warehouse like Amazon Redshift or a data lake like Azure Data Lake. Some Sample Destinations in LIKE.TG 7. Analytics and Reporting: Finally, LIKE.TG offers analytics and reporting capabilities for immediate customer insights. For a more comprehensive analysis, data can be seamlessly integrated with leading BI tools such as PowerBI and Tableau. Alternatively, businesses can utilize LIKE.TG’s proprietary analytics add-on for a cohesive, user-friendly data analysis experience. The Next Step In navigating the complexities of a dynamic market, adopting a Customer 360 strategy becomes imperative for maintaining a competitive edge and achieving sustainable success. By following these steps with LIKE.TG, you can establish a robust Customer 360 view, allowing your organization to gain actionable insights, personalize customer interactions, and foster stronger relationships based on a holistic understanding of customer data. Ready to transform your customer data into actionable insights? Get started with LIKE.TG’s 14-day free trial today. Ready to Gain a Holistic Customer 360 View? Discover LIKE.TG Data Pipeline Builder, with its advanced data integration capabilities that can transform your customer understanding. Learn More!
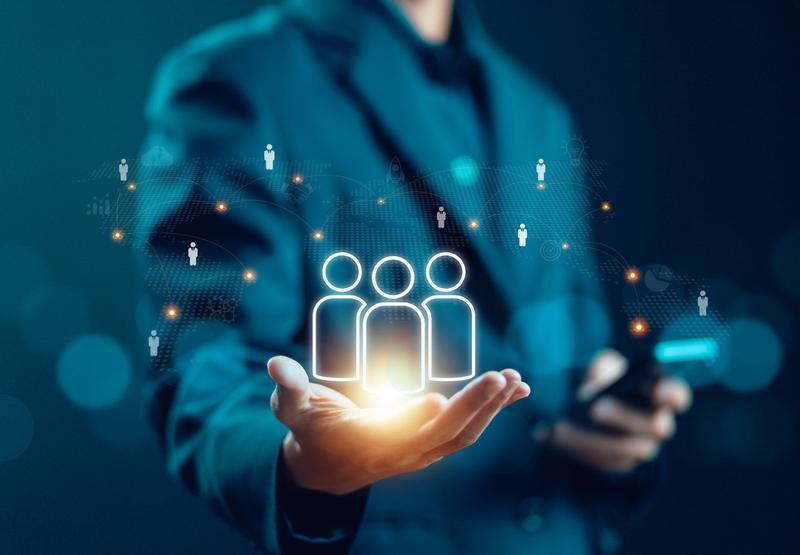
Customer 360: What It Is and How to Implement It?
Every customer interaction, from an online click to an in-store purchase is part of the web of customer data. This web is a rich source of insights into customer behavior, preferences, and needs. The key to untangling this is Customer 360, or gaining a comprehensive view of the customer journey. According to Gartner, Inc., only 14% of organizations have successfully achieved a 360-degree view of their customers even though successfully implementing Customer 360 can empower businesses to deliver personalized experiences, foster customer loyalty, and drive growth. What is Customer 360 and Why it Matters Customer 360 is a strategic business approach that involves integrating and consolidating customer data from various departments and touchpoints within an organization to create a unified and comprehensive customer profile. Consider each department within an organization as a piece of a puzzle. Marketing holds demographic and behavioral data, sales manages contact details and lead interactions, and customer support handles service requests. Individually, these pieces offer limited insights, much like separate puzzle pieces that don’t reveal the full picture. A customer 360 approach, however, connects and integrates these various data sets, fitting them into place to create a single source of truth. By breaking down data silos, it helps businesses understand the entire customer journey, enabling personalized experiences, informed decision-making, and improved collaboration across departments. Customer 360 Use Cases and Real-World Applications Personalized Marketing Customer 360 allows businesses to tailor their marketing efforts to individual customers, enhancing the effectiveness of marketing campaigns and improving customer engagement. For example, Spotify uses a 360-degree view of its customers listening habits, preferences, and search history to curate personalized playlists. This enhances user engagement and satisfaction. Enhanced Customer Service By providing a comprehensive view of a customer’s history with the company, Customer 360 enables customer service teams to offer personalized and effective service. Predictive Analytics Using a holistic view provides a wealth of data that can be analyzed to predict future customer behavior and trends. Netflix, for example, uses predictive analytics to recommend shows and movies based on a user’s viewing history and preferences. This strategy has proven to be highly effective, with 80% of Netflix watches sprouting from its recommendation engine. Improved Sales Strategies With insights from a 360 view of data, sales teams can identify potential upsell or cross-sell opportunities. Amazon, the global e-commerce giant, analyzes a customer’s purchase history and browsing behavior to suggest relevant products. One of their most successful features is the “Frequently bought together” recommendation. This data-driven approach allows Amazon to cross-sell and upsell products that a customer is likely to be interested in, leading to increased sales and customer satisfaction. Customer Retention This approach allows businesses to track a customer’s entire journey and experience with the company, revealing potential issues or areas of dissatisfaction. Sephora, the beauty retailer, tracks purchase history and product preferences to offer personalized product recommendations and rewards, increasing customer loyalty. Campaign Optimization Customer 360 enables businesses to optimize their marketing campaigns based on comprehensive customer insights. A great example of this is the “Share a Coke” campaign by Coca-Cola. The company leveraged a 360-degree view of customer data to discover that personalized bottles were a hit with consumers. Consequently, they expanded the range of names on bottles and emphasized personalization in their marketing, which led to a 2% rise in U.S. sales. Customer 360 Tools and Technologies These tools and technologies are designed to aggregate, integrate, and analyze customer data from multiple sources to create a comprehensive and unified view of each customer. These tools play a crucial role in enhancing customer experiences, improving customer engagement, and supporting data-driven decision-making. Here are some key technologies commonly used in building and implementing Customer 360 solutions: Customer Relationship Management (CRM) Systems CRM systems are software platforms designed to manage and analyze customer interactions throughout their lifecycle. These platforms serve as comprehensive hubs for customer data, consolidating information such as contact details, purchase history, and communication preferences. CRM systems optimize customer relationships, streamline sales processes, and enhance overall customer service. By offering a unified perspective on customer interactions, CRMs empower organizations to make informed decisions that positively impact customer engagement and satisfaction. Examples of CRM systems include Salesforce, HubSpot, and Microsoft Dynamics 365. Customer Data Platforms (CDPs) CDPs, as specialized tools, concentrate on creating a consolidated and unified customer profile by integrating data from diverse sources. These platforms are specifically tailored to centralize customer data and provide a holistic view of interactions and behaviors. CDPs excel in delivering personalized marketing campaigns and refining customer segmentation based on a thorough understanding of individual preferences. Unlike general-purpose CRM systems, CDPs are laser-focused on optimizing the use of customer data for targeted and effective marketing efforts. Examples of CDPs include Segment, Tealium, and Exponea. Data Integration Platforms Data integration platforms play a foundational role in unifying data from various sources, encompassing departments like sales, marketing, and customer support. These platforms leverage ETL processes or middleware to harmonize and consolidate data, as well as eliminate data silos. By ensuring a seamless flow of information across the organization, data integration platforms contribute to a unified and coherent dataset. Examples of data integration platforms include Informatica, Talend, and LIKE.TG. Implementing Customer 360 Implementing a customer 360 view is a multi-step process that enables businesses to gain a comprehensive understanding of their customers to enhance customer experiences and drive sustainable growth. Here’s a step-by-step guide to help you successfully implement Customer 360 in your organization: Identify Data Sources: Start by identifying all potential sources of customer data within your organization. This could include CRM systems, sales data, customer service interactions, social media engagement, website analytics, and email marketing responses. Collect Data: After identifying your data sources, gather the customer data. This should include demographic details, transaction history, communication records, online behavior, customer feedback, and any other relevant data that provides insight into the customer’s relationship with your business. Integrate Data: Next, integrate the collected data into a centralized system. This involves consolidating data from different sources and ensuring uniformity in its format. A unified view is pivotal for a comprehensive understanding of each customer. Implement Customer Identity Resolution: This critical step involves accurately linking each piece of customer data to the respective individual customer. Advanced algorithms are used to match these diverse data points, effectively consolidating the scattered data into a unified view of each customer’s journey. Analyze Data: With a complete and accurate picture of each customer, analyze this data using visualization tools like PowerBI and Tableau to find patterns and trends. This helps to understand customer behavior and preferences, turning raw data into meaningful insights. The insights derived from the data analysis are then leveraged to inform and shape business strategies. This could involve personalizing marketing efforts, enhancing customer service, or tailoring products or services to meet the specific needs and preferences of the customers. Creating a Comprehensive Customer 360 View with LIKE.TG When unified and analyzed, the wealth of customer data forms a comprehensive Customer 360 view, offering invaluable insights into customer behavior, preferences, and needs. However, the key to unlocking these insights lies in effectively breaking down data silos and integrating this scattered data into a cohesive, actionable view. LIKE.TG, a leading provider of data integration and management solutions, simplifies this process. With its robust capabilities and user-friendly interface, LIKE.TG streamlines the creation of a customer 360 view, turning complex data into clear insights. Here’s how LIKE.TG facilitates the process: 1. Data Collection: LIKE.TG enables the seamless collection of data from a wide array of sources, including CRM systems, social media platforms, customer feedback, and transactional data. With its extensive range of connectors, including database, cloud, CRM, ERP, and file connectors, LIKE.TG ensures seamless data extraction. Data Sources in LIKE.TG 2. Data Profiling: LIKE.TG allows businesses to profile their customer data to identify trends, patterns, and anomalies. This process aids in understanding customer behavior and predicting future trends. Data Profiling in LIKE.TG 3. Data Quality Assurance: LIKE.TG prioritizes the accuracy and reliability of the data. It offers features for data cleansing, validation, and deduplication, ensuring the data used to build the Customer 360 view is accurate, consistent, and up-to-date. Defining data validation rules in LIKE.TG 4. Data Transformation: LIKE.TG provides tools for mapping, transforming, and converting data types and formats. This includes transformations like aggregation, filtering, normalization, and pivoting/unpivoting, which help convert raw data into a format suitable for analysis and interpretation, facilitating the extraction of meaningful insights. Sample Transformations in LIKE.TG 5. Data Integration: LIKE.TG employs various techniques, such as merging, joining, and reconciling, to seamlessly combine and consolidate data from diverse sources and build a unified and cohesive dataset. Combining datasets using Join transformation in LIKE.TG 6. Data Loading: LIKE.TG’s robust data loading capabilities support high-volume data loading from various sources into a central repository such as a data warehouse like Amazon Redshift or a data lake like Azure Data Lake. Some Sample Destinations in LIKE.TG 7. Analytics and Reporting: Finally, LIKE.TG offers analytics and reporting capabilities for immediate customer insights. For a more comprehensive analysis, data can be seamlessly integrated with leading BI tools such as PowerBI and Tableau. Alternatively, businesses can utilize LIKE.TG’s proprietary analytics add-on for a cohesive, user-friendly data analysis experience. The Next Step In navigating the complexities of a dynamic market, adopting a Customer 360 strategy becomes imperative for maintaining a competitive edge and achieving sustainable success. By following these steps with LIKE.TG, you can establish a robust Customer 360 view, allowing your organization to gain actionable insights, personalize customer interactions, and foster stronger relationships based on a holistic understanding of customer data. Ready to transform your customer data into actionable insights? Get started with LIKE.TG’s 14-day free trial today. Ready to Gain a Holistic Customer 360 View? Discover LIKE.TG Data Pipeline Builder, with its advanced data integration capabilities that can transform your customer understanding. Learn More!
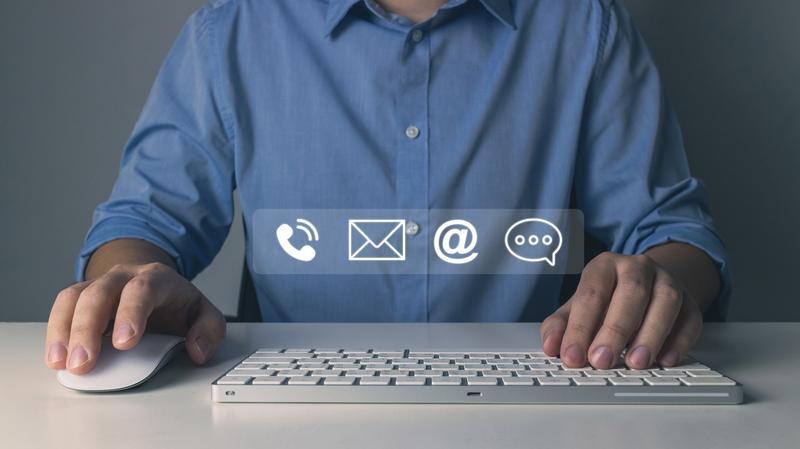
What Is Data Movement? The Complete Guide
What is Data Movement? Data movement is the process of transferring data from one place to another. This process is typically initiated when there are system upgrades, consolidations, or when there is a need to synchronize data across different platforms for business intelligence or other operational purposes. Data movement ensures that the right data is available at the right place and at the right time. The key, however, is to transfer data seamlessly without impacting system performance, allowing businesses to continue their operations smoothly while the data is being moved. Types of Data Movement Data movement can be categorized into several types based on the purpose and specific requirements of the data transfer. Common methods include Extract, Transform, and Load (ETL), Extract, Load, and Transform (ELT), data replication, and Change Data Capture (CDC). Each of these methods serves a unique purpose and is chosen based on factors such as the volume of data, the complexity of the data structures, and the need for real-time data availability. Let’s delve into each of these techniques in more detail: 1. Data Migration: Data migration is the process of moving data from one system, location, or format to another. This may be driven by factors such as system upgrades, cloud adoption, or the need to consolidate data from different sources. Data migration requires careful planning to ensure data integrity, consistency, and minimal downtime during the transition. The migration process often involves data cleaning to remove redundant, obsolete, or inaccurate data, ensuring that only high-quality, relevant data is moved to the new system. 2. Data Replication: Data replication involves creating an exact copy of data from one location and moving it to another. This is used to ensure consistency between redundant resources, often in distributed systems. It’s commonly used in disaster recovery, as it ensures business continuity in case of system failures. Data replication also improves data availability, providing real-time data for analysis and enhancing system performance by distributing the load across multiple systems. 3. ETL: ETL is a type of data movement where data is extracted from the source, transformed to fit operational needs, and then loaded into the target destination. The transformation process may involve the restructuring, cleaning, and formatting of data to align it with the standards and requirements of the intended target system or data warehouse. This phase ensures data consistency, quality, and compatibility. Finally, the loaded data, now transformed, is placed into the designated tables or structures within the target system, typically a data warehouse optimized for reporting and analytics. 4. ELT: ELT is similar to ETL, but the order of operations is different. In ELT, data is extracted, loaded into a staging area, and then transformed. This method is particularly beneficial when handling large volumes of data, as it leverages the processing power of the target system, allowing for efficient and flexible transformations. ELT is well-suited for cloud-based data warehouses and big data solutions, where high processing power can expedite the data transformation process after loading. 5. CDC: Change data capture (CDC) is a technique designed to identify and capture changes made to data over time. It tracks additions, updates, and deletions within a dataset, creating a log of modifications. CDC is particularly valuable for keeping data synchronized between source and target systems efficiently. By capturing only the changes made to the data, CDC minimizes the processing load and network bandwidth requirements, making it an effective strategy for real-time data integration and replication. Purpose of Data Movement With the rapid advancements in technology and the increasing volume of data generated by organizations, data movement has become a critical process. It plays a pivotal role in migrating data to modern systems as part of IT operations’ modernization and extension. As organizations upgrade their legacy systems to leverage advanced technologies, data movement ensures a seamless transition, preventing data loss and enabling full utilization of modern system capabilities. Data movement finds its use in various scenarios within an organization. Here are some key use cases: Data Archiving: Data archiving is the process of moving data that is no longer actively used to a separate storage device for long-term retention. Data movement comes into play here as it helps transfer this inactive data from primary storage systems to an archive. This frees up valuable space in the primary storage so that the archived data can be accessed and retrieved when needed. Database Replication: This involves creating and maintaining multiple copies of the same database, often in different locations. Data movement is integral to this process as it ensures that any changes made to one database (like adding, modifying, or deleting data) are simultaneously reflected in all other copies. This maintains consistency across databases and enhances data availability and reliability. Cloud Data Warehousing: Cloud data warehousing involves storing an organization’s data in a cloud-based data warehouse. Data movement is used to transfer data from the organization’s on-premises systems to the cloud warehouse. This not only provides the benefits of scalability and cost-efficiency associated with cloud storage but also enables real-time data analysis and insights. Hybrid Data Movement: In a hybrid IT environment, organizations use a combination of on-premises and cloud-based systems. Hybrid data movement involves transferring data between these systems as needed. This way, data is always available in the right place at the right time, regardless of whether it’s stored on-premises or in the cloud. Benefits of Data Movement Data movement offers several benefits to an organization and contributes to the overall efficiency and effectiveness of its data management strategy. Here are some key benefits: Facilitates Data Consolidation: Data movement allows organizations to consolidate data from various sources into a single, unified system. By simplifying data management, it ensures consistency and accuracy of data, and provides a comprehensive view for better decision-making. Optimizes System Performance: Data movement enhances system performance by managing data load effectively. It transfers inactive or seldom-used data from primary to secondary or archive storage, reducing the burden on the primary system and improving system speed and efficiency. Improves Data Protection: Data movement is integral to the process of data backup and recovery. By creating backups of data, organizations can safeguard their data against potential loss or damage due to system failures or data breaches, enhancing data security and ensuring business continuity. Enables Data Synchronization: In a multi-cloud or hybrid cloud environment, data movement helps synchronize data across different platforms, ensuring that all systems have the most up-to-date and consistent data, thereby improving data reliability. Enhances Data Availability: In the context of database replication, data movement ensures that data is consistently available across multiple locations, enhancing data availability and reliability, particularly in distributed systems. Risks Involved in Data Movement While data movement is crucial for many aspects of data management and IT operations, it does come with certain risks. Here are some of the potential risks involved in data movement and the ways in which they can be overcome: Data Loss: One of the most significant risks while moving data between systems is data loss, which can occur due to multiple reasons, such as technical glitches, human error, or system failures. To mitigate this risk, it’s crucial to have a robust data backup strategy in place. Regular backups ensure that a recent copy of the data is always available for recovery. Data Breaches: Moving data sets, particularly sensitive ones, can expose them to potential security threats. If not properly secured, data could be intercepted during transit, leading to data breaches. To prevent data breaches, organizations should take measures to encrypt their data during transit. Additionally, secure data transfer protocols should be used, and access to the data should be strictly controlled. Data Corruption: Data can get corrupted during the movement process due to various reasons, such as software bugs, hardware failures, or sudden power outages, rendering it unusable. One way to this is to perform data integrity checks before and after the data movement process. Any discrepancies should be immediately addressed to ensure the accuracy of the data. Compliance Violations: If data is not moved in compliance with data protection and privacy regulations, it can result in legal penalties and damage to the organization’s reputation. Organizations should be well-versed with relevant regulations and ensure that their data movement processes are compliant. Downtime: Data movement, particularly large-scale data migration, can lead to system downtime, disrupting business operations. However, most downtimes can be avoided by scheduling data movements during off-peak hours. Also, using a phased migration approach where data is moved in small batches helps keep the transition smooth. The Need For a Data Movement Tool How to Select One? Organizations today handle data that’s not only increasing in complexity but also in size, necessitating the need for data movement tools. With data often scattered across various sources and systems, a data movement tool helps consolidate and integrate this data, ensuring it’s available where and when it’s needed. These tools also facilitate the seamless migration of data during system upgrades, optimize storage management through data archiving, and enhance data availability in distributed systems. Choosing the right data movement tool is a critical decision that can significantly influence an organization’s data management capabilities. Here are some specific factors to consider: Data Connectivity: The tool should be able to connect to various data sources, both on-premises and cloud-based. At the same time, it should support different data types and formats. Scalability: As an organization’s data volume grows, the tool should be able to scale to meet these increasing needs. It should be able to handle data volume without compromising performance. Compatibility: The solution should be compatible with the organization’s existing systems and platforms. It should be able to handle the types of data sources the organization works with and support the data formats in use. Security: Given the sensitive nature of data, the data movement tool should have robust security features. This includes encryption for data in transit, secure access controls, and compliance with relevant data security standards. Real-Time Data Movement: For businesses requiring real-time insights, the tool should be capable of moving data in real-time or near real-time. Support and Documentation: When dealing with complex data movement tasks or troubleshooting issues, it is crucial to look for a solution that provides comprehensive customer support and documentation to provide the necessary assistance. Conclusion As businesses continue to evolve and expand, the volume, velocity, and variety of data they handle are set to rise exponentially. This makes data movement an increasingly crucial aspect of any data management strategy. Effective data movement ensures that data from various sources is consolidated, transformed, and made readily accessible for real-time decision-making. Although it does not inherently play a direct role in maintaining data quality and ensuring regulatory compliance, it does offer the opportunity to account for these processes, facilitating seamless data integration. LIKE.TG, with its comprehensive suite of features, is an effective data movement tool. It seamlessly integrates with existing systems, offering robust data transformation capabilities, extensive connectivity options, and near real-time data movement, making it a reliable choice for businesses of varying sizes. By integrating LIKE.TG into their data management strategy, organizations can effectively navigate their current data landscape and prepare for the future, thereby enhancing operational efficiency and overall business performance. Try LIKE.TG out with a free 14-day trial today and elevate your data management strategy! Start Your Data Movement Journey Today Leverage LIKE.TG's extensive connectivity options that enable seamless integration with a variety of platforms, databases, and applications, facilitating smooth and effective data movement across diverse systems. Download Free Trial