API to BigQuery: 2 Preferred Methods to Load Data in Real time
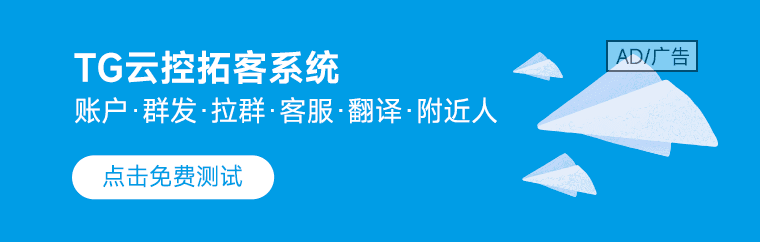
LIKE.TG 成立于2020年,总部位于马来西亚,是首家汇集全球互联网产品,提供一站式软件产品解决方案的综合性品牌。唯一官方网站:www.like.tg
Many businesses today use a variety of cloud-based applications for day-to-day business, like Salesforce, HubSpot, Mailchimp, Zendesk, etc. Companies are also very keen to combine this data with other sources to measure key metrics that help them grow.
Given most of the cloud applications are owned and run by third-party vendors – the applications expose their APIs to help companies extract the data into a data warehouse – say, Google BigQuery. This blog details out the process you would need to follow to move data from API to BigQuery.
Besides learning about the data migration process from rest API to BigQuery, we’ll also learn about their shortcomings and the workarounds. Let’s get started.
Note: When you connect API to BigQuery, consider factors like data format, update frequency, and API rate limits to design a stable integration.
Method 1: Loading Data from API to BigQuery using LIKE.TG Data
LIKE.TG is the only real-time ELT No-code Data Pipeline platform that cost-effectively automates data pipelines that are flexible to your needs. With integration with 150+ Data Sources (40+ free sources), we help you not only export data from sources & load data to the destinations but also transform & enrich your data, & make it analysis-ready.
Here are the steps to move data from API to BigQuery using LIKE.TG :
Step 1: Configure REST API as your source
- Click PIPELINES in the Navigation Bar.
- Click + CREATE in the Pipeline List View.
- In the Select Source Type page, select REST API.
- In the Configure your REST API Source page:
- Specify a unique Pipeline Name, not exceeding 255 characters.
- Set up your REST API Source.
- Specify the data root, or the path, from where you want LIKE.TG to replicate the data.
- Select the pagination method to read through the API response. Default selection: No Pagination.
Step 2: Configure BigQuery as your Destination
- Click DESTINATIONS in the Navigation Bar.
- Click + CREATE in the Destinations List View.
- In Add Destination page select Google BigQuery as the Destination type.
- In the Configure your Google BigQuery Warehouse page, specify the following details:
Yes, that is all. LIKE.TG will do all the heavy lifting to ensure that your analysis-ready data is moved to BigQuery, in a secure, efficient, and reliable manner.
To know in detail about configuring REST API as your source, refer to LIKE.TG Documentation.
Sign Up for a 14-day free trial and experience the feature-rich LIKE.TG suite firsthand.
Method 2: API to BigQuery ETL Using Custom Code
The BigQuery Data Transfer Service provides a way to schedule and manage transfers from REST API datasource to Bigquery for supported applications.
One advantage of the REST API to Google BigQuery is the ability to perform actions (like inserting data or creating tables) that might not be directly supported by the web-based BigQuery interface. The steps involved in migrating data from API to BigQuery are as follows:
- Getting your data out of your application using API
- Preparing the data that was extracted from the Application
- Loading data into Google BigQuery
Step 1: Getting data out of your application using API
Below are the steps to extract data from the application using API.
Get the API URL from where you need to extract the data. In this article, you will learn how to use Python to extract data from ExchangeRatesAPI.io which is a free service for current and historical foreign exchange rates published by the European Central Bank. The same method should broadly work for any API that you would want to use.
API URL = https://api.exchangeratesapi.io/latest?symbols=USD,GBP. If you click on the URL you will get below result:
{ "rates":{ "USD":1.1215, "GBP":0.9034 }, "base":"EUR", "date":"2019-07-17" }
Reading and Parsing API response in Python:
a. To handle API response will need two important libraries
import requests import json
b. Connect to the URL and get the response
url = "https://api.exchangeratesapi.io/latest?symbols=USD,GBP" response = requests.get(url)
c. Convert string to JSON format
parsed = json.loads(data)
d. Extract data and print
date = parsed["date"] gbp_rate = parsed["rates"]["GBP"] usd_rate = parsed["rates"]["USD"]
Here is the complete code:
import requests
import json
url = "https://api.exchangeratesapi.io/latest?symbols=USD,GBP"
response = requests.get(url)
data = response.text
parsed = json.loads(data)
date = parsed["date"]
gbp_rate = parsed["rates"]["GBP"]
usd_rate = parsed["rates"]["USD"]
print("On " + date + " EUR equals " + str(gbp_rate) + " GBP")
print("On " + date + " EUR equals " + str(usd_rate) + " USD")
Step 2: Preparing data received from API
There are two ways to load data to BigQuery.
- You can save the received JSON formated data on JSON file and then load into BigQuery.
- You can parse the JSON object, convert JSON to dictionary object and then load into BigQuery.
Step 3: Loading data into Google BigQuery
We can load data into BigQuery directly using API call or can create CSV file and then load into BigQuery table.
- Create a Python script to extract data from API URL and load (UPSERT mode) into BigQuery table. Here UPSERT is nothing but Update and Insert operations. This means – if the target table has matching keys then update data, else insert a new record.
import requests
import json from google.cloud
import bigquery url = "https://api.exchangeratesapi.io/latest?symbols=USD,GBP"
response = requests.get(url)
data = response.text
parsed = json.loads(data)
base = parsed["base"]
date = parsed["date"]
client = bigquery.Client()
dataset_id = 'my_dataset'
table_id = 'currency_details'
table_ref = client.dataset(dataset_id).table(table_id)
table = client.get_table(table_ref) for key, value in parsed.items(): if type(value) is dict: for currency, rate in value.items(): QUERY = ('SELECT target_currency FROM my_dataset.currency_details where currency=%', currency) query_job = client.query(QUERY) if query_job == 0: QUERY = ('update my_dataset.currency_details set rate = % where currency=%',rate, currency) query_job = client.query(QUERY) else: rows_to_insert = [ (base, currency, 1, rate) ] errors = client.insert_rows(table, rows_to_insert) assert errors == []
- Load JSON file to BigQuery. You need to save the received data in JSON file and load JSON file to BigQuery table.
import requests import json from google.cloud import bigquery url = "https://api.exchangeratesapi.io/latest?symbols=USD,GBP" response = requests.get(url) data = response.text parsed = json.loads(data) for key, value in parsed.items(): if type(value) is dict: with open('F:Pythondata.json', 'w') as f: json.dump(value, f) client = bigquery.Client(project="analytics-and-presentation") filename = 'F:Pythondata.json' dataset_id = ‘my_dayaset’' table_id = 'currency_rate_details' dataset_ref = client.dataset(dataset_id) table_ref = dataset_ref.table(table_id) job_config = bigquery.LoadJobConfig() job_config.source_format = bigquery.SourceFormat.NEWLINE_DELIMITED_JSON job_config.autodetect = True with open(filename, "rb") as source_file: job = client.load_table_from_file(source_file, table_ref, job_config=job_config) job.result() # Waits for table load to complete. print("Loaded {} rows into {}:{}.".format(job.output_rows, dataset_id, table_id))
Limitations of writing custom scripts and developing ETL to load data from API to BigQuery
- The above code is written based on the current source as well as target destination schema. If the data coming in is either from the source or the schema on BigQuery changes, ETL process will break.
- In case you need to clean your data from API – say transform time zones, hide personally identifiable information and so on, the current method does not support it. You will need to build another set of processes to accommodate that. Clearly, this would also need you to invest extra effort and money.
- You are at a serious risk of data loss if at any point your system breaks. This could be anything from source/destination not being reachable to script breaks and more. You would need to invest upfront in building systems and processes that capture all the fail points and consistently move your data to the destination.
- Since Python is an interpreted language, it might cause performance issue to extract from API and load data into BigQuery api.
- For many APIs, we would need to supply credentials to access API. It is a very poor practice to pass credentials as a plain text in Python script. You will need to take additional steps to ensure your pipeline is secure.
API to BigQuery: Use Cases
- Advanced Analytics: BigQuery has powerful data processing capabilities that enable you to perform complex queries and data analysis on your API data. This way, you can extract insights that would not be possible within API alone.
- Data Consolidation: If you’re using multiple sources along with API, syncing them to BigQuery can help you centralize your data. This provides a holistic view of your operations, and you can set up a change data capture process to avoid discrepancies in your data.
- Historical Data Analysis: API has limits on historical data. However, syncing your data to BigQuery allows you to retain and analyze historical trends.
- Scalability: BigQuery can handle large volumes of data without affecting its performance. Therefore, it’s an ideal solution for growing businesses with expanding API data.
- Data Science and Machine Learning: You can apply machine learning models to your data for predictive analytics, customer segmentation, and more by having API data in BigQuery.
- Reporting and Visualization: While API provides reporting tools, data visualization tools like Tableau, PowerBI, and Looker (Google Data Studio) can connect to BigQuery, providing more advanced business intelligence options. If you need to convert an API table to a BigQuery table, Airbyte can do that automatically.
Additional Resources on API to Bigquery
- Read more on how to Load Data into Bigquery
Conclusion
From this blog, you will understand the process you need to follow to load data from API to BigQuery. This blog also highlights various methods and their shortcomings. Using these two methods you can move data from API to BigQuery. However, using LIKE.TG , you can save a lot of your time!
Move data effortlessly with LIKE.TG ’s zero-maintenance data pipelines, Get a demo that’s customized to your unique data integration challenges
You can also have a look at the unbeatable LIKE.TG Pricing that will help you choose the right plan for your business needs!
FAQ on API to BigQuery
How to connect API to BigQuery?
1. Extracting data out of your application using API
2. Transform and prepare the data to load it into BigQuery.
3. Load the data into BigQuery using a Python script.
4. Apart from these steps, you can also use automated data pipeline tools to connect your API url to BigQuery.
Is BigQuery an API?
BigQuery is a fully managed, serverless data warehouse that allows you to perform SQL queries. It provides an API for programmatic interaction with the BigQuery service.
What is the BigQuery data transfer API?
The BigQuery Data Transfer API offers a wide range of support, allowing you to schedule and manage the automated data transfer to BigQuery from many sources. Whether your data comes from YouTube, Google Analytics, Google Ads, or external cloud storage, the BigQuery Data Transfer API has you covered.
How to input data into BigQuery?
Data can be inputted into BigQuery via the following methods.
1. Using Google Cloud Console to manually upload CSV, JSON, Avro, Parquet, or ORC files.
2. Using the BigQuery CLI
3. Using client libraries in languages like Python, Java, Node.js, etc., to programmatically load data.
4. Using data pipeline tools like LIKE.TG
What is the fastest way to load data into BigQuery?
The fastest way to load data into BigQuery is to use automated Data Pipeline tools, which connect your source to the destination through simple steps. LIKE.TG is one such tool.
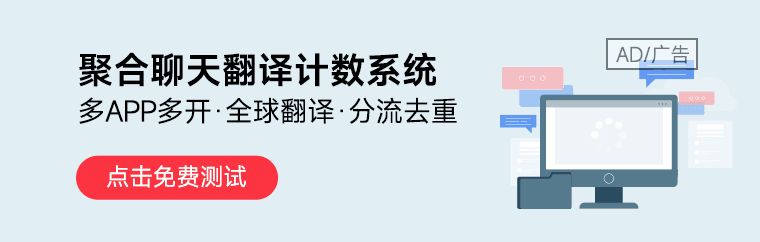
LIKE.TG 专注全球社交流量推广,致力于为全球出海企业提供有关的私域营销获客、国际电商、全球客服、金融支持等最新资讯和实用工具。免费领取【WhatsApp、LINE、Telegram、Twitter、ZALO】等云控系统试用;点击【联系客服】 ,或关注【LIKE.TG出海指南频道】、【LIKE.TG生态链-全球资源互联社区】了解更多最新资讯
本文由LIKE.TG编辑部转载自互联网并编辑,如有侵权影响,请联系官方客服,将为您妥善处理。
This article is republished from public internet and edited by the LIKE.TG editorial department. If there is any infringement, please contact our official customer service for proper handling.