All You Need to Know About Data Aggregation
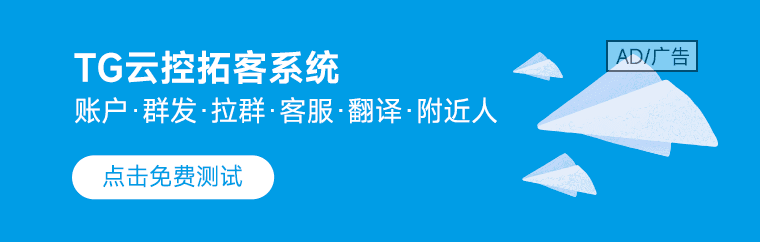
LIKE.TG 成立于2020年,总部位于马来西亚,是首家汇集全球互联网产品,提供一站式软件产品解决方案的综合性品牌。唯一官方网站:www.like.tg
Data aggregation is the process of combining and summarizing data from disparate sources into a cohesive dataset. It prepares data for analysis, making it easier to obtain insights into patterns and insights that aren’t observable in isolated data points.
Once aggregated, data is generally stored in a data warehouse. Then, you can leverage it to gain a holistic perspective on your operations and market trends, design effective risk management practices, and make more informed decisions overall.
This blog offers an in-depth look at data aggregation to help you understand what it is, how it works, and how it benefits your business when done right.
Understanding Data Aggregation
What is Data Aggregation?
Data aggregation is performed using specialized tools called data aggregators. Depending on the nature of the data and your objectives, the aggregation process can include grouping, averaging, summing, and counting. Individual records and atomic data rows are consolidated into a summarized dataset following these operations — resulting in aggregated data.
Examples of Aggregated Data
- Number of students in a given school district: The total number of enrolled students in each school district can offer insights into the state of education in different city areas.
- Number of customers by city: Instead of assessing each customer individually, a business can group its customers in a particular town to determine their shared characteristics, interests, and preferences.
- Financial reporting: Financial data is regularly aggregated for reporting purposes. For example, balance sheets, income, and cash flow statements are consolidated to create comprehensive financial reports. These reports help stakeholders understand an enterprise’s financial health.
Comparing Aggregated and Disaggregated Data
These are some of the factors that differentiate aggregated and disaggregated data from each other:
Aggregated Data | Disaggregated Data |
Replaces individual data elements with summaries that represent various groups as a whole. | Comprises individual data elements or groups that aren’t summarized or collated. |
Accelerates querying and analysis, allowing you to quickly evaluate vast data. | Features the same granularity as the original data, allowing you to assess each data point individually if needed. |
Offers a concise representation of the whole dataset, enabling you to identify patterns and trends. | Provides a detailed view that is useful for accessing and analyzing individual data records. |
What are Data Aggregators?
Data aggregators are crucial to data aggregation and management. These tools collect data from various sources and feature built-in capabilities for aggregate measurements. They utilize different techniques to collect, clean, and process data. They can also help you track data lineage to trace your aggregated data.
The Only Data Aggregation Tool You Need
Zero coding but plenty of aggregate functions and an interface that's ideal for business and technical users alike. Your free trial awaits.
Start Using LIKE.TG Today!Importance and Benefits of Data Aggregation for Businesses
1. Decision-Making
Data aggregation provides comprehensive insights that can assist you in making better strategic decisions for your organization. Consolidating, summarized data from wide-ranging sources ensures you aren’t considering just one perspective in your analysis. The result is a more detailed picture of trends, opportunities, and potential room for growth.
2. Performance Monitoring
Data aggregation facilitates you in monitoring key performance indicators (KPIs) more effectively. When you keep up with sales, revenue, operational efficiency, and customer satisfaction, you can assess your business’ performance capably and course-correctly to achieve your goals.
3. Risk Management
Aggregating your data helps identify potential risks and take the necessary steps to mitigate them. Including various sources in your data aggregation process lets you determine potential anomalies and threats. You can then take decisive, proactive measures to protect your business.
4. Increased Efficiency
Data centralization is one of the crucial components of data aggregation. Storing all relevant information in a single accessible location reduces the time and resources required to access and analyze data, which enhances efficiency.
5. Better Insights
Aggregated data unlocks deeper insights into your business. It spotlights vital factors such as your market dynamics, operations, and current and potential customer base. Analyzing aggregated data allows you to unravel correlations, trends, and patterns to drive growth.
6. Competitive Edge
Data aggregation gives you a competitive advantage over other businesses in your niche. You can make data-driven decisions in less time and with better accuracy. Over time, this edge allows you to adapt and respond to changing market patterns more effectively than others.
Data Aggregation Types and Techniques
There are various types of data aggregation. Your requirements and how you plan to use the data will determine which approach suits your use case.
Temporal
As the name suggests, temporal aggregation summarizes data over specified time intervals. These intervals can be as short as hours or days and as long as months or years. This aggregation type is preferable to conduct trend or pattern analysis over time.
Temporal aggregation is extensively utilized in time-series modeling, financial analysis, and economic forecasting. You can use it to identify seasonality or cyclical patterns in your data.
Spatial
Spatial aggregation is helpful to summarize your data based on geographical locations or other spatial units — such as postal codes, towns, cities, states, or countries. You can use spatial aggregation to analyze spatial patterns, identify regional differences in data, or perform geographic analyses. It’s commonly used in urban planning and geographic information systems (GIS).
Hierarchical
Hierarchical aggregation arranges data into hierarchical structures like groups, subgroups, categories, and subcategories. This aggregation is useful for cases requiring drill-down analyses or hierarchical reporting.
These are some uses of hierarchical aggregation in a few industries:
- Finance: Evaluating financial data by transaction, account type, and branch.
- Manufacturing: Overseeing the production cycle by SKU, production line, and factory.
- Government: Using regional and administrative level demographic data to guide decision-making.
- Healthcare: Reviewing patient data by medical condition/diagnosis, department, and hospital.
- Supply Chain: Optimizing distribution and inventory levels by studying customer, route, and warehouse/storage facility details.
Techniques of Data Aggregation
Data aggregation techniques are critical in data summarization and analysis. These techniques range from simple processes to more complex operations, enabling you to analyze vast data volumes efficiently.
Here are ten common data aggregation techniques:
- Grouping: Arrange data in groups based on specified criteria, such as grouping purchase orders by department or product type.
- Summation adds numerical values for calculating totals, for example, determining the total purchase expense in a cycle.
- Counting tallies the occurrences or records, such as the number of transactions every day in a purchases dataset.
- Averaging calculates the average value or mean of a set of numbers. This technique is useful for determining the average purchase amount or the average number of items in a purchase order.
- Minimization identifies the lowest value of an attribute across a group of records, such as the smallest purchase amount each day.
- Maximization determines an attribute’s highest or largest value across a group of records, such as the most significant purchase amount each day.
- Median calculation finds the middle value in a sorted list of numerals and provides a measure of central tendency.
- Mode calculation finds the value that occurs most frequently in a group of records. In a purchases dataset, we can summarize each city’s most common product category.
- Weighted averaging averages values and assigns different weights to each of them. This technique is useful for calculating weighted average prices or scores.
- Hierarchical aggregation aggregates the data at multiple granularity levels in a hierarchical structure. For example, aggregating sales data by city, country, or region.
How to Aggregate Data: Key Steps Involved
1. Define Your Objectives
You need to define your objectives for data aggregation before starting the aggregation process. Identify your uses for the aggregated data and determine how it can aid you in making decisions and achieving your goals.
2. Collect Your Data
For effective data aggregation, you must collect data from relevant sources. Besides being relevant, your data must be complete, up-to-date, and accurate. Automated tools can help you streamline data collection and eliminate the errors associated with manual processes.
3. Enhance Data Quality
Next, enhance your data’s quality to improve its reliability. At this stage, you can implement data validation checks, enforce data governance policies and procedures, perform data profiling, and standardize data formats and values to keep your datasets consistent.
4. Aggregate Data
Once you’ve collected your data and undergone the necessary procedures to improve its quality, you can start aggregating it. As discussed above, your aggregation process may involve grouping or summarizing your data — depending on your requirements.
5. Validate The Results
Lastly, you’ll need to validate the results of the data aggregation process. Compare the aggregated data with your source data, identify any discrepancies that need attention, and fix them as required.
There's a Faster Way of Aggregating Data
Numerous businesses are already benefiting from LIKE.TG's enterprise-grade data platform. Yours can be next. Find out how LIKE.TG can cater to your unique use case.
Speak to Our TeamChallenges in Data Aggregation
- Missing or Incomplete Data: Incomplete data can disrupt aggregation and generate inaccurate or skewed results. Implement effective strategies such as imputation techniques or data sampling to counter its impact on the aggregated results.
- Handling Large Volumes of Data: Aggregating vast amounts of data is a resource-intensive process that can affect performance. Consider turning to scalable data aggregation solutions to easily process and analyze massive datasets.
- Addressing Privacy and Security Concerns: You may encounter privacy and security concerns when aggregating confidential data. Protect sensitive data throughout the aggregation process by implementing data privacy and security measures such as access controls, encryption, and anonymization methods.
- Managing Data Sources and Integration: Since data aggregation involves working with numerous sources, data integration can often be challenging owing to each source’s different format, semantics, and structure. A comprehensive data integration process can help you ensure seamless data compatibility regardless of your sources.
Effective Data Aggregation: Considerations and Best Practices
Focusing on these key considerations and best practices helps ensure effective and reliable data aggregation that results in quality data:
-
Ensuring Data Quality and Consistency
Data quality and consistency are of the utmost importance when aggregating data. Remember to monitor and validate your data to ensure it remains accurate, complete, and relevant.
-
Choosing The Right Data Aggregation Methods
You should also choose the correct aggregation methods, which will vary depending on the nature of your data and your objectives for your analysis. Data complexity, granularity, and volume are crucial when selecting a data aggregation technique.
-
Documenting All Processes and Underlying Assumptions
When aggregating data, document all processes and assumptions you use to obtain the aggregated results. Maintaining comprehensive documentation ensures transparency and repeatability, besides being valuable for troubleshooting and auditing.
-
Performing a Sensitivity Analysis
Conducting a sensitivity analysis determines how variations or uncertainties in the data affect the aggregated results. A typical sensitivity analysis involves the following:
- Identifying the key parameters or variables that can impact the aggregated data.
- Modifying those parameters within reason to simulate varying conditions.
- Analyzing how changes in these variables affect the aggregated results.
- Evaluating the aggregated results’ reliability under various scenarios.
- Documenting the sensitivity analysis process to gain insights into the aggregated data’s reliability.
-
Data Governance and Compliance
Inadequate data governance and compliance procedures can risk your data security, quality, and integrity. Prioritizing adherence to data governance standards keeps your business compliant with data regulations, improves auditability and transparency, and maintains data integrity.
-
Scalability and Performance
Your organization’s data aggregation should have scalability and performance at its core. These factors account for further growth and expansion. Additionally, they maintain aggregation efficiency even with growing datasets, improve productivity and mitigate bottlenecks, ensure optimal resource utilization, and future-proof your data aggregation process.
-
Data Quality Assurance
Data quality is central to every data management process. In data aggregation, focusing on maintaining data quality has multiple benefits. It ensures access to accurate insights, mitigates potentially expensive errors, improves decision-making, and elevates your organization’s reputation by proving your commitment to excellence.
-
Engaging with Stakeholders
Like many other organizational activities, data aggregation is most effective when you incorporate suggestions and input from stakeholders. Engaging with them while developing your data aggregation process helps you better understand varying departmental requirements, cultivate trust and buy-in, facilitate adoption and ownership, and foster collaboration to ensure alignment.
Tools for Data Aggregation
There are different kinds of data aggregation tools and technologies available to help you with data integration. Here’s a closer look at each of them:
Spreadsheet Software
Spreadsheet software provides elementary data aggregation functionality. You can organize, manipulate, and analyze data in tabular format within them. While they’re great for basic aggregation and ad-hoc analysis, a lack of advanced features prevents them from supporting large-scale data processing and automation requirements.
For instance, a small business owner can use Microsoft Excel or Google Sheets to aggregate sales data from different store locations to calculate total revenue for the quarter.
Database Management Systems
Database management systems (DBMS) allow you to store, query, and aggregate large volumes of structured data. SQL databases support relational data aggregation, and NoSQL databases efficiently handle unstructured and semi-structured data.
For example, if a financial institution needs to aggregate and analyze transactions from different branches to find possible fraudulent activity, it can use a DBMS.
Business Intelligence Platforms
Business Intelligence (BI) platforms offer advanced data aggregation and visualization features so you can analyze and present business data. Some of these features include reporting tools, dashboards, and data modeling.
These platforms support complex data aggregation and analysis tasks across finance, operations, and marketing.
For example, a retail company’s marketing department can use a BI platform for aggregating customer data from various channels — email, website, and social media. This aggregated data can help create customer segmentations and profiles, leading to more targeted marketing campaigns.
Programming Languages and Libraries
Programming languages can be powerful — albeit more difficult— tools for aggregating data. These languages feature extensive libraries for manipulating and aggregating data and performing statistical analyses.
Consider a data scientist using Python and working with genomic data for a research project. They’ll use the panda’s library to analyze this data to identify genetic markers linked to a disease or medical condition.
Data Integration Platforms
Data integration platforms like LIKE.TG provide data aggregation solutions for collecting, cleansing, and integrating data from disparate sources. With features such as data connectors, transformation tools, and built-in workflow automation functionality, these platforms streamline data aggregation while maintaining data quality.
For instance, a retail business can use LIKE.TG to summarize purchases across different cities Calibri (Body) in a purchase orders dataset to find information such as average, max, min, purchase amounts, and number of purchases across different cities. This aggregated data can help the business craft a more targeted marketing strategy.
Uses of Data Aggregation
Data aggregation is essential across different industries, seven of which are:
1. Financial Services
By consolidating financial data from various sources, data aggregation enables thorough financial analysis and leads to informed investment decisions. Specific applications of data aggregation in finance include:
- Risk Management: Evaluating credit risk, operational risk, and market risk.
- Portfolio Management: Assessing asset allocation, portfolio performance, and investment strategy.
- Regulatory Compliance: Ensuring compliance with Markets in Financial Instruments Directive 2014 (MiFID 2), the Dodd-Frank Act, Basel III, and other regulations.
2. Retail and E-Commerce
Analyzing aggregated data from different channels helps businesses in the retail and e-commerce domain with the following applications:
- Inventory Management: Maintaining optimum stock levels, minimizing stockouts, and lowering carrying costs.
- Sales Analysis: Evaluating product performance, sales trends, and customer behavior.
- Customer Segmentation: Developing targeted marketing campaigns, offering more personalized recommendations, and boosting customer retention rates.
3. Healthcare
Aggregating and evaluating medical data helps healthcare facilities with the following:
- Improving Patient Care: Creating customized treatment plans to provide more targeted care and lead to better patient outcomes.
- Population Healthcare Management: Identifying patterns in population health, disparities in healthcare accessibility, and disease outbreaks. Studying aggregated data can also aid in developing community healthcare initiatives.
- Streamlining Clinical Research: Aggregated clinical trial data enables researchers to analyze patient demographics, treatment efficacy, and safety profiles for drug development and regulatory approval.
4. Manufacturing
Different types of aggregated data in the manufacturing sector serve different uses:
- Production Planning: Assessing aggregated production data to optimize resource utilization, decrease downtime, and improve manufacturing processes.
- Supply Chain Management: Aggregated supply chain data enables businesses to monitor supplier performance, check inventory levels, and identify and correct supply chain bottlenecks.
- Quality Control: Aggregated quality control data aids in identifying flaws, determining their root causes, and applying corrective measures.
5. Marketing and Advertising
Studying the insights gained from various kinds of aggregated data helps marketing teams with the following:
- Campaign Performance: Aggregated marketing campaign data is useful for measuring ROI, assessing customer engagement, and optimizing advertising spend.
- Market Research: Aggregated consumer data (such as survey responses, social media posts, and online activity) reveals market trends and consumer preferences.
- Customer Analytics: Aggregated customer data allows marketing personnel to create more accurate customer profiles and personas for targeted messaging.
6. Transportation and Logistics
A variety of data is available for aggregation in the transportation and logistics sector. A few examples and uses include:
- Fleet Management: Aggregated vehicle and driver data helps optimize routes, lower fuel consumption, and increase fleet efficiency.
- Supply Chain Visibility: Aggregated logistics data aids in tracking shipments, monitoring delivery times, and inventory management.
- Demand Forecasting: Aggregated demand data can help predict future transportation needs, alleviate supply chain disruptions, and optimize resource allocation.
7. Government/Public Sector
Data is available abundantly in the government/public sector — and in the context of data aggregation, here are a few ways they can leverage it:
- Policy Development: Aggregated economic, social, and demographic data can guide policy development, impact resource allocation, and help identify and resolve common public welfare challenges.
- Emergency Response: During emergencies, aggregated real-time data enables prompt response coordination and facilitates timely assistance.
- Performance Measurement: Aggregated government program data is crucial for evaluating program effectiveness, tracking outcomes, and maintaining accountability.
Conclusion
Aggregation offers you a uniquely comprehensive view of your data. It reveals insights that individual data points can’t deliver on their own. To derive maximum value from your data, you should design a well-planned aggregation process that aligns with your organizational requirements — and choose the right data aggregation tool.
With its award-winning, highly acclaimed no-code interface and robust data management capabilities, LIKE.TG is the intuitive, user-friendly solution to your data aggregation problems. You can ingest data from various sources, use the Aggregate transformation object, and apply aggregate functions, such as Sum, Count, First, Last, Min, Max, Average, Var, or Standard Deviation, and load data to your preferred destination.
LIKE.TG helps you make the most of your data by aggregating it for high-level insights. Start your FREE trial today, or contact our team for more information.
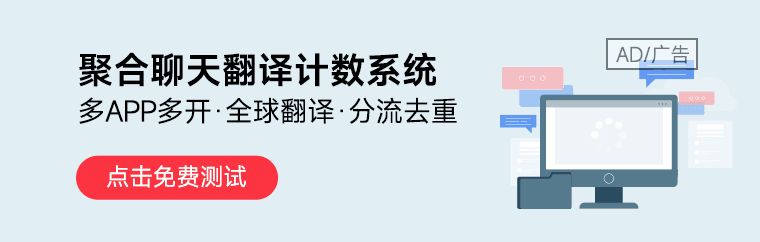
现在关注【LIKE.TG出海指南频道】、【LIKE.TG生态链-全球资源互联社区】,即可免费领取【WhatsApp、LINE、Telegram、Twitter、ZALO云控】等获客工具试用、【住宅IP、号段筛选】等免费资源,机会难得,快来解锁更多资源,助力您的业务飞速成长!点击【联系客服】
本文由LIKE.TG编辑部转载自互联网并编辑,如有侵权影响,请联系官方客服,将为您妥善处理。
This article is republished from public internet and edited by the LIKE.TG editorial department. If there is any infringement, please contact our official customer service for proper handling.