Data Quality Tools: Top 8 for 2024 & Beyond
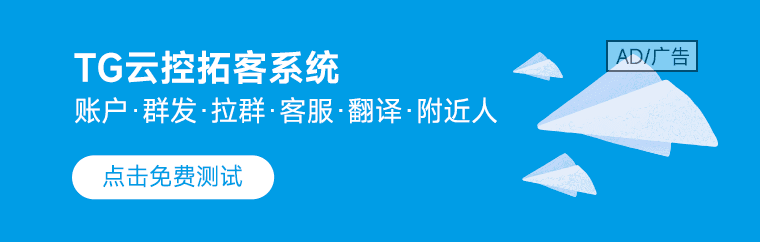
LIKE.TG 成立于2020年,总部位于马来西亚,是首家汇集全球互联网产品,提供一站式软件产品解决方案的综合性品牌。唯一官方网站:www.like.tg
While data volume is increasing at an unprecedented rate today, more data doesn’t always translate into better insights. What matters is how accurate, complete, and reliable that data is. Data quality is not trivial; it is the foundation upon which organizations make informed decisions, formulate effective strategies, and gain a competitive edge. Surprisingly, only 3% of companies currently meet basic data quality standards, highlighting the urgency for businesses to prioritize investments in data quality tools.
Data quality tools make it easier for you to deal with the challenges of modern data: volume and velocity. Using these tools, you can easily streamline data quality management and ensure you consistently get reliable insights.
In this blog, we will explore the top 8 data quality tools in the market and how you should select the right one for your business.
Top Data Quality Tools for 2024
1. LIKE.TG
LIKE.TG is a unified, zero-code platform that empowers organizations to effortlessly manage their end-to-end data management processes, including extraction, integration, warehousing, electronic data exchange, and API lifecycle management.
Its drag-and-drop, user-friendly interface allows both technical and non-technical users to leverage LIKE.TG solutions to carry out complex data-related tasks in minutes, improving efficiency and performance. LIKE.TG offers comprehensive data quality features to ensure data accuracy, reliability, and completeness.
Key Features:
- Drag-and-drop UI: LIKE.TG’s intuitive, point-and-click interface allows you to configure source and destination systems easily and create rule-driven transformations for seamless data integration and testing.
- Data Validation: LIKE.TG guarantees data accuracy and quality through comprehensive data validation features, including data cleansing, error profiling, and data quality rules, ensuring accurate and complete data.
- Variety of Connectors: LIKE.TG seamlessly connects to a wide range of on-premise and cloud-based sources, including databases, data warehouses, and data lakes. Additionally, it empowers you to build API-based connectors for extended connectivity options.
- Pre-built Transformations: It offers pre-built transformations like join, union, merge, data quality rules, etc., to help clean, transform, and integrate your data.
- Point-and-Click Navigation: LIKE.TG enables smooth navigation via point-and-click actions, letting users add, modify, and track changes for transparent data transformations.
- Interactive Data Grid: The tool offers agile data correction and completion capabilities allowing you to rectify inaccurate data. You can visualize and explore data intuitively for accuracy and consistency.
- Reusable Scripts: LIKE.TG streamlines data preparation with efficient, reusable scripts across workflows, promoting automation, efficiency, and consistency.
- Real-Time Data Health Checks: The tool allows you to monitor and adjust data in real-time for integrity, providing instant feedback on data quality
- Effortless Integration: You can seamlessly integrate cleaned data into analytics platforms or publish it as API for easy external system collaboration and insights generation.
- Workflow Automation: The tool offers workflow orchestration so that you can save time and let the software schedule automatic tasks, orchestrate data-driven processes, and much more.
2. Talend
Talend is another data quality solution designed to enhance data management processes. It uses machine learning technology to profile, cleanse, and mask data in real time and offer intelligent recommendations to address data quality issues. Its machine learning-enabled deduplication, validation, and standardization features allow its users to clean incoming records and enrich them as needed, enabling access to reliable insights.
However, Talend Data Quality may be complex to set up, particularly for non-technical users. The solution also lacks in-memory capacity, which can result in performance and speed issues, especially when dealing with large datasets of complex data transformations. Moreover, it has a higher price point than several other DQM solutions in the market.
Key Features:
- Data Profiling: Talend Data Quality utilizes machine learning to profile data in real-time automatically, swiftly identify data quality issues, detect hidden patterns, and spot anomalies ensuring accurate and up-to-date insights
- Self-service interface: The platform offers a convenient self-service interface that is equally intuitive for business users and technical experts, promoting effective collaboration across the organization.
- Talend Trust Score: The built-in Talend Trust Score provides an immediate and precise assessment of data confidence, guiding users in secure data sharing and pinpointing datasets that require additional cleansing.
- Data Security and Compliance: The tool has security and compliance features, safeguarding your data and ensuring adherence to relevant regulations.
3. IBM InfoSphere
IBM InfoSphere Information Server is a data integration platform that simplifies data understanding, cleansing, monitoring, and transformation. IBM InfoSphere Information Server enables continuous data cleansing and tracking, allowing organizations to turn raw data into trusted information.
Based on user reviews, IBM InfoSphere Information Server has some limitations, including a complex initial setup that requires technical expertise. Users have also highlighted the platform’s complexity as a potential hurdle, which may necessitate additional training or skilled personnel. Additionally, the platform’s feasibility is contingent on the organization’s size and complexity, with smaller or simpler entities may find it excessive for their needs.
Key Features:
- Performance Management: You can rely on IBM InfoSphere Information Server for monitoring and optimizing the performance of your data integration processes.
- Data Security: With its data security features, IBM InfoSphere Information Server ensures your data remains safe and protected.
- Data Integration: The platform allows you to integrate data from diverse sources, such as databases, files, and web services.
- Process Management: IBM InfoSphere Information Server also provides process management capabilities, helping you effectively oversee your data integration processes.
- Data Quality Control: You can ensure the quality of your data with the data quality control capabilities integrated into IBM InfoSphere Information Server to assess, analyze, and monitor your data’s quality effectively.
4. Data Ladder
Data Ladder is a quality control and cleaning tool that uses matching algorithms to improve data quality. It helps users to clean data and uncover missed matches from diverse sources, ensuring reliability and accuracy throughout the enterprise data ecosystem.
However, limited documentation is available for its advanced features, such as custom data profiling patterns, advanced matching options, and survivorship rule setup. Additionally, a few users have reported encountering issues with the data-matching algorithm.
Key Features:
- Data Import: Data Ladder allows you to connect and integrate data from multiple disparate sources, including file formats, relational databases, cloud storage, and APIs.
- Data Profiling: It automates data quality checks and provides instant data profile reports on blank values, data types, patterns, and other stats, revealing data cleansing opportunities.
- Data Cleansing: The tool helps eliminate inconsistent and invalid values, create and validate patterns, and achieve a standardized view across all data sources.
- Data Matching: Data Ladder enables you to execute proprietary and industry-grade match algorithms based on custom-defined criteria and match confidence levels for exact, fuzzy, numeric, or phonetic matching.
5. Ataccama ONE
Ataccama ONE is a modular, integrated platform that provides a range of data quality functionalities. With Data Governance, Data Quality, and Master Data Management combined in an AI-powered fabric, it allows businesses and data teams to grow while ensuring data trust, security, and governance.
Based on user feedback, Ataccama ONE exhibits certain limitations. Its inherent complexity has proven to be particularly challenging for beginners. Therefore, users need to have a clear understanding of technical concepts such as coding and troubleshooting, especially when dealing with large datasets. Furthermore, users experience difficulty in performing complex data transformations and managing conflicts during updates to downstream systems.
Key Features:
- Data Governance: Ataccama ONE offers data governance capabilities, enabling effective and efficient data management.
- Data Quality: With Ataccama ONE, you can leverage AI to ensure data quality by understanding, validating, and enhancing your data, preventing the influx of erroneous information into your systems, and continuously monitoring data accuracy.
- Data Catalog: The tool enables you to discover, understand, and utilize your data resources.
- Data Integration: You can integrate data from diverse sources with the data integration capabilities of Ataccama ONE.
6. Experian Aperture Data Studio
Experian is a global information services company offering data, analytics, and insights to businesses and consumers alike. Its platform, Aperture Data Studio, is a dynamic and user-friendly data management suite designed to enhance confidence in managing consumer data projects. This tool allows users of all levels to swiftly develop intricate workflows, incorporating machine-learning algorithms for automated data tagging. Moreover, it enhances data quality by utilizing meticulously curated global datasets from Experian, ensuring compliance with data standards.
According to user reviews, Aperture Data Studio has certain performance limitations, particularly when dealing with large datasets. While the tool’s ease of use facilitates rapid adoption, it also poses a potential risk of losing control over the assets being created and may lead to unintentional duplication of effort and data inconsistencies.
Key Features:
- Data Profiling: Aperture Data Studio offers data profiling capabilities, enabling a better understanding of your data and identification of potential data quality issues.
- Data Matching: It includes advanced data matching features, utilizing both proprietary and well-established matching algorithms to help you accurately match and deduplicate your data.
- Data Integration: The tool facilitates data integration from various sources, including Hadoop clusters, to consolidate isolated data sets in a single customer view.
- Workflow Management: Aperture Data Studio enables the creation of sophisticated workflows that incorporate machine learning algorithms for automating data tagging and enrichment.
7. OpenRefine
OpenRefine (formerly known as Google Refine) is an open-source tool for data quality management. Using this tool, you can identify and rectify data issues, apply data transformations and perform data exploration. It has a variety of features for data cleansing and standardization to ensure accuracy and consistency.
However, the tool has certain limitations to consider. Firstly, Undo/Redo functionality lacks mid-history undo capability and may lead to unintended data loss when applying new operations. Reusing and sharing workflows can be difficult due to the lack of error handling in operation sequences and adapting workflows to projects with different column names.
Key Features:
- Faceting: OpenRefine enables you to navigate and analyze extensive datasets efficiently. This allows you to filter and view specific portions of your data, making it easier to detect patterns and trends swiftly.
- Clustering: The tool aids in resolving inconsistencies within your data by merging similar values using intelligent techniques, minimizing duplicates, and ensuring better consistency throughout the dataset.
- Reconciliation: OpenRefine enables you to match your dataset with external databases through reconciliation services to enhance the accuracy and completeness of your data by linking it to reliable external sources.
- Infinite Undo/Redo: This feature allows effortless movement to prior dataset states, revisiting the entire operation history for experimentation with data transformations and quickly reversing changes when needed.
8. Informatica
Informatica is a modern enterprise cloud data management solution that ensures the accuracy of data within a single environment. With capabilities for transforming, profiling, integrating, cleansing, reconciling data, and managing metadata, it enables businesses to drive innovation and growth by making the most of their critical assets.
A significant limitation of Informatica is the difficulty users face when debugging workflows and mappings. Additionally, many users have expressed frustration with Informatica’s error messages, finding them hard to comprehend or cryptic, leading to potential delays in issue resolution and decision-making.
Key Features:
- Data Integration: Informatica’s primary strength lies in data integration. It can fetch data from various heterogeneous systems and transfer it to other business processes and users within your organization.
- Data Quality: With Informatica’s data quality features, you can gain insights into your data’s condition, validate and enhance it, prevent the inclusion of inaccurate data into systems, and continuously monitor data quality.
- Safe Data Exchange: Informatica ensures the secure data exchange in Business-to-Business interactions, offering complete visibility throughout the entire process.
- Parallel Processing: One of Informatica’s notable capabilities is parallel processing, which allows the concurrent execution of multiple processes, resulting in faster computation and execution.
Criteria for Selecting the Right Data Quality Tools
You must carefully evaluate the capabilities and features of a data quality management (DQM) tool and match them against specified criteria to ensure it matches your organization’s requirements.
The following criteria stand out as crucial in the selection process:
- Scalability and Performance:
You must ensure that the chosen tool can effectively handle your current data volume and be able to accommodate future growth. Look for a robust data quality tool that can process large datasets efficiently without compromising overall system performance. Additionally, consider one that offers real-time data processing capabilities for time-sensitive insights.
- Data Profiling and Cleansing Capabilities:
You must assess whether a tool provides comprehensive data profiling features. This will allow you to gain insights into data quality, detect anomalies, and understand data distribution patterns. Look for a tool with advanced cleansing capabilities to correct errors, standardize formats, remove duplicates, and validate data.
- Data Monitoring Features:
Consider tools that go beyond one-time solutions and provide continuous data monitoring features. Select a tool that allows you to track data quality metrics, set up alerts for anomalies, and establish data lineage to comprehend data origins and transformations over time.
- Seamless Integration with Existing Systems:
Ensure compatibility with your data sources, databases, data warehouses, and business intelligence platforms to facilitate a smooth implementation process without disrupting your established workflows. Look for a data quality tool that offers easy-to-use connectors or APIs for seamless integration with your existing IT infrastructure to minimize implementation workout.
- User-Friendly Interface:
You should opt for a data quality tool with an intuitive and user-friendly interface, enabling your teams to adopt and leverage the tool’s features quickly. A straightforward implementation process is essential, and you should aim for tools that do not require extensive technical training and accelerate the onboarding process.
- Flexibility and Customization Options:
Flexibility and customization are paramount, considering the diverse data types and requirements that your organization deals with. Look for a data quality tool that allows you to create custom data quality rules, workflows and adapt to changing data quality requirements as your organization evolves.
- Vendor Support and Community:
Evaluating the vendor’s reputation and support is essential for your selection process. Prioritize vendors with a track record of providing excellent customer support, regular updates, and bug fixes. Additionally, consider tools with an active user community or forum, as it signifies a solid user base and the availability of shared knowledge and resources.
- Pricing and Licensing Options:
You must consider the data quality tool’s pricing models and licensing options. Different tools may offer various pricing structures, such as subscription-based models or charges based on data volume or features used. Choosing a pricing plan that aligns with your organization’s budget and expected data usage is crucial.
Best Practices for Implementing Data Quality Tools
Implementing data quality tools effectively ensures that your organization can derive maximum value from its data and make informed decisions. Here are some essential steps and best practices to guide you through the process:
- Clearly Define Requirements
Before selecting and implementing data quality tools, clearly define your organization’s specific data quality requirements. Identify the types of data quality issues you frequently encounter, the data sources that need improvement, and the desired outcomes. Having a clear understanding of your needs will guide you in choosing the right tools.
- Thoroughly Evaluate Tools
Conduct a comprehensive evaluation of various data quality tools available in the market. Compare their features, functionalities, scalability, ease of use and compatibility with your existing data infrastructure. Look for tools that align best with your organization’s needs and seamlessly integrate them into your data management processes.
- Start Small; Scale Gradually
When implementing data quality tools, start with a pilot project or a small segment of your data. This approach allows you to test the tool’s effectiveness and identify any potential challenges or adjustments needed. Once you are confident in the results, gradually scale up the implementation across more significant datasets.
- Involve Stakeholders and Experts
Include key stakeholders in the decision-making process, such as data analysts, data engineers, and business users. Their input is valuable in understanding specific data quality pain points and in ensuring that the selected tools align with their requirements. Additionally, consider seeking advice from data quality experts or consultants to make informed choices.
- Provide Training and Support
Train your team members on how to use the data quality tools efficiently. Offer workshops or training sessions to familiarize them with the tool’s functionalities and best practices for data validation and cleansing. Moreover, establish a support system where users can seek assistance when facing challenges during tool adoption.
Final Words
Data quality is an ongoing commitment towards excellence, shaping every decision in a data-driven ecosystem. By adopting data quality tools, organizations embed a culture of data excellence into their core operations, ensuring that data remains trustworthy and consistent throughout its lifecycle. Consequently, data teams can focus on analyzing the data and extracting insights instead of spending excessive efforts on manually cleaning and reconciling data.
Is your data holding you back? Unleash its true potential with LIKE.TG. Schedule a personalized demo!
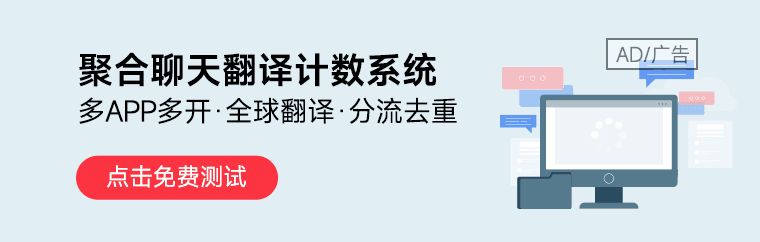
现在关注【LIKE.TG出海指南频道】、【LIKE.TG生态链-全球资源互联社区】,即可免费领取【WhatsApp、LINE、Telegram、Twitter、ZALO云控】等获客工具试用、【住宅IP、号段筛选】等免费资源,机会难得,快来解锁更多资源,助力您的业务飞速成长!点击【联系客服】
本文由LIKE.TG编辑部转载自互联网并编辑,如有侵权影响,请联系官方客服,将为您妥善处理。
This article is republished from public internet and edited by the LIKE.TG editorial department. If there is any infringement, please contact our official customer service for proper handling.