Data Filtering: A Comprehensive Guide to Techniques, Benefits, and Best Practices
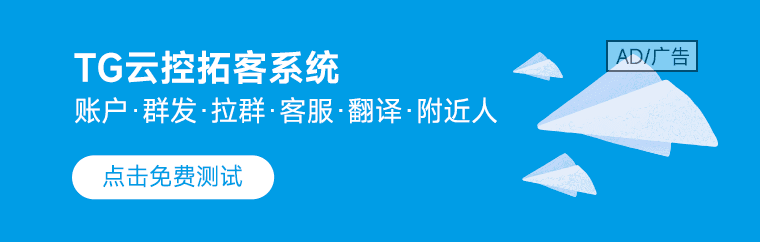
LIKE.TG 成立于2020年,总部位于马来西亚,是首家汇集全球互联网产品,提供一站式软件产品解决方案的综合性品牌。唯一官方网站:www.like.tg
Data filtering plays an instrumental role in reducing computational time and enhancing the accuracy of AI models. Given the increasing need for organizations to manage large volumes of data, leveraging data filtering has become indispensable.
What Is Data Filtering?
Data filtering is the process of narrowing down the most relevant information from a large dataset using specific conditions or criteria. It makes the analysis more focused and efficient.
Data filtering lets you quickly analyze relevant data without sifting through the entire dataset. You can filter data regardless of type, including numbers, categories, text, and complex time-series data.
Data Filtering vs. Data Sorting vs Data Sampling
While data filtering helps process large volumes of data, it is not the only method. Data sampling and sorting can also help draw insights from a large dataset. Here’s a brief overview and comparison:
- Data Filtering: Selects a subset of data based on specific criteria.
- Data Sorting: Arrange data in a specified order, either ascending or descending.
- Data Sampling: Chooses a representative subset from a larger dataset for analysis.
Parameter | Data Filtering | Data Sorting | Data Sampling |
Purpose | To narrow down data to meet specific conditions. | To organize data in a meaningful order. | To analyze a smaller, manageable subset of data that represents the whole. |
Process | Uses criteria to include or exclude data. | Rearrange data based on chosen attributes. | Randomly or systematically selects data points from the entire dataset. |
Outcome | A reduced dataset focused on relevant data points. | An ordered dataset based on specific attributes. | A smaller dataset that reflects the characteristics of the more extensive set. |
Each method can be used by itself or in combination to extract insights from large volumes of data.
What is Data Filtering Used For?
- Evaluating a Dataset: Filtering aids in exploratory data analysis by helping identify patterns, trends, or anomalies within a dataset.
- Processing Records: Data filtering streamlines workflows by processing records based on predefined criteria.
- Remove Irrelevant Data: Filtered data can help remove irrelevant data before restructuring via pivoting, grouping/aggregating, or other means.
Benefits of Using Data Filtering
Organizations prioritizing data filtering are better positioned to derive valuable insights from their data. Here is how data filtering can help you gain a competitive advantage.
- Enhances Focus: Data filtering allows you to ignore irrelevant data, enabling a sharper focus on information that aligns with their goals, which can improve the quality of insights.
- Increases Accuracy: Filtering out outliers and erroneous records contributes to a more reliable data analysis process and improves the accuracy of the results.
- Optimizes Resource Use: Working with smaller, filtered datasets can reduce the resources needed for analysis, leading to potential cost savings.
- Supports Custom Analysis: Data filtering accommodates unique analytical needs across various projects or departments by creating datasets tailored to specific criteria.
Types of Data Filtering Techniques
Data filtering techniques can help you quickly access the data you need.
Basic Filtering Methods
Basic filtering involves simple techniques like range or set membership. For example, in a database of temperatures recorded throughout a year, a range filter could be used to select all records where the temperature was between 20°C and 30°C. Similarly, a set membership filter could select records for specific months, like June, July, and August.
Filtering by Criteria
Filtering by criteria involves more advanced filtering based on multiple criteria or conditions. For instance, an e-commerce company might filter customer data to target a marketing campaign. They could use multiple criteria, such as customers who have purchased over $100 in the last month, are in the 25-35 age range, and have previously bought electronic products.
Filtering by Time Range
Temporal filters work by selecting data within a specific time frame. A financial analyst might use a time range filter to analyze stock market trends by filtering transaction data to include only those that occurred in the last quarter. This helps focus on recent market behaviors and predict future trends.
Text Filtering
Text filtering includes techniques for filtering textual data, such as pattern matching. For example, a social media platform might filter posts containing specific keywords or phrases to monitor content related to a specific event or topic. Using pattern matching, they can filter all posts with the hashtag #EarthDay.
Numeric Filtering
Numeric filtering involves methods for filtering numerical data based on value thresholds. A healthcare database might be filtered to identify patients with high blood pressure by setting a numeric filter to include all records where the systolic pressure is above 140 mmHg and the diastolic pressure is above 90 mmHg.
Custom Filtering
Custom filtering refers to user-defined filters for specialized needs. A biologist studying a species’ population growth might create a custom filter to include data points that match a complex set of conditions, such as specific genetic markers, habitat types, and observed behaviors, to study the factors influencing population changes.
These techniques can be applied to extract meaningful information from large datasets, aiding in analysis and decision-making processes.
Data Filtering Tools and Software
Data filtering can be performed via manual scripting or no-code solutions. Here’s an overview of these methods:
Filtering Data Manually
Manual data filtering often involves writing custom scripts in programming languages such as R or Python. These languages provide powerful libraries and functions for data manipulation.
Example: In Python, the pandas library is commonly used for data analysis tasks. A data scientist might write a script using pandas to filter a dataset of customer feedback, selecting only entries that contain certain keywords related to a product feature of interest. The script could look something like this:
Python
import pandas as pd
# Load the dataset
df = pd.read_csv(‘customer_feedback.csv’)
# Define the keywords of interest
keywords = [‘battery life’, ‘screen’, ‘camera’]
# Filter the dataset for feedback containing the keywords
filtered_df = df[df[‘feedback’].str.contains(‘|’.join(keywords))]
Using No-Code Data Filtering Software
No-code data filtering software allows you to filter data through a graphical user interface (GUI) without writing code. These tools are designed to be user-friendly and accessible to people with little programming experience. With Regular Expressions capabilities, you have the flexibility to write custom filter expressions.
Example: A bank’s marketing department wants to analyze customer transaction data to identify potential clients for a new investment product. The data includes various transaction types, amounts, and descriptions. The team is particularly interested in clients who have made large transactions in the past year that may indicate an interest in investment opportunities.
Using a no-code data filtering tool, the marketing team can filter records that contain terms like ‘stock purchase,’ ‘bond investment,’ or ‘mutual fund’ in their transaction description field. They also set a numeric filter to include transactions above a certain amount. The tool’s GUI allows them to easily input these parameters without writing complex code.
The result is a filtered list of clients who meet the criteria, which the bank can then use to target their marketing campaign for the new investment product.
Feature | Manual Filtering (Python/R) | No-Code Data Filtering with Regular Expressions | ||
Ease of Use | Requires programming knowledge | User-friendly with intuitive GUI | ||
Pattern Matching | Complex filter expressions need coding | Simplified filter implementation | ||
Learning Curve | Steep requires learning syntax | Minimal, often with helpful tutorials | ||
Speed of Setup | Time-consuming script development | Quick setup with immediate results | ||
Accessibility | Limited to those with coding skills | Accessible to non-technical users | ||
Maintenance | Requires ongoing script updates | Often includes automatic updates | ||
Scalability | Can be less efficient for large datasets | Designed to handle big data efficiently | ||
Cost Efficiency | Potential for higher long-term costs | Cost-effective with subscription models | ||
Collaboration | Less collaborative, more individual-focused | Encourages collaboration with shared access |
Best Practices for Effective Data Filtering
It’s essential to follow the best practices below to ensure that data filtering is as effective and efficient as possible:
Define Clear Objectives
Having clear goals for what you want to achieve with data filtering. Before you begin, ask yourself:
- What specific insights am I trying to obtain?
- Which data is relevant to my analysis?
- How will the filtered data be used?
Clear objectives guide the filtering process, ensuring the results align with your analytical or operational goals.
Understand Data Structure and Format
A thorough understanding of the data’s structure and format is essential. Consider the following:
- Is the data structured, semi-structured, or unstructured?
- What are the data types of the columns I’m interested in?
- Are there any relationships between the data points that need to be preserved?
Understanding these aspects helps apply the most appropriate filters and prevents potential issues such as data loss or misinterpretation.
Utilize Multiple Filters for Complex Analysis
For complex analysis, a single filter might not be sufficient. Instead, use a combination of filters to drill down into the data:
- Apply a range filter followed by a categorical filter to narrow your dataset.
- Use text filters with numeric filters to further segment the data.
Multiple filters can provide a more nuanced view of the data, revealing deeper insights.
Validate Results and Adjust Filters as Needed
Regular validation of filtering results is essential to ensure accuracy. After applying filters, check if:
- The results meet your initial objectives.
- The filtered data makes sense in the context of your goals.
- Any anomalies or unexpected results need investigation.
If the results aren’t satisfactory, adjust the filters and re-validate. This iterative process helps refine the filtering strategy to produce the best possible outcomes.
Adhering to these best practices helps maximize the effectiveness of data filtering, leading to more reliable and actionable insights.
Data filtering significantly enhances the computational efficiency of training AI models, improving their accuracy. The advent of no-code data filtering tools has further streamlined this process, enabling you to develop AI systems that are not only more precise but also more efficient.
How LIKE.TG’s No-Code Data Filtering Saves 80% of Your Time
LIKE.TG Dataprep is a no-code data filtering tool that eliminates the need for complex coding, streamlines repetitive tasks, ensures consistency across projects, and offers immediate insights into data health, collectively saving up to 80% of the time typically spent on data preparation. It offers:
- Drag-and-Drop Interface uses Point-and-Click fields to filter data, simplifying data preparation.
- Dataprep Recipes standardize data preparation across multiple datasets, significantly reducing time and effort.
- Data Health Visuals provide immediate visual feedback on the quality of your data, allowing you to quickly identify and address issues such as inconsistencies or missing values.
- Real-Time Grid provides a dynamic dataframe that updates in real-time as data is transformed within the platform, giving you an interactive view of the data and illustrating the immediate effects of data manipulation.
- Automated Dataflows: reduce the need for manual intervention.
- Intuitive Filter Expressions perform complex pattern matching through the user-friendly interface, saving time on writing and debugging code.
- Prebuilt Connectors enable quick integration with various data sources.
- Advanced Data Validation and Profiling ensure data accuracy and consistency, allowing you to validate data against predefined rules and profile data for quality analysis.
Ready to transform data management and save valuable time? Try LIKE.TG Dataprep, the all-in-one data preparation tool that simplifies data filtering, integration, and transformation.
Start your journey with LIKE.TG Dataprep today and revolutionize how you work with data!
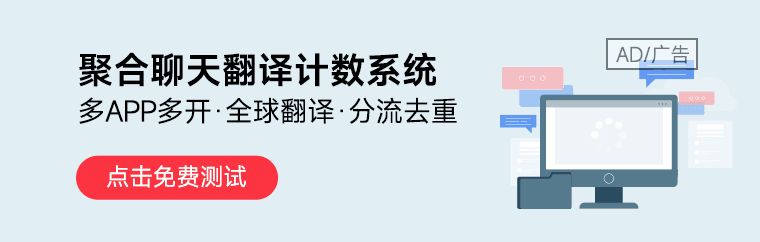
现在关注【LIKE.TG出海指南频道】、【LIKE.TG生态链-全球资源互联社区】,即可免费领取【WhatsApp、LINE、Telegram、Twitter、ZALO云控】等获客工具试用、【住宅IP、号段筛选】等免费资源,机会难得,快来解锁更多资源,助力您的业务飞速成长!点击【联系客服】
本文由LIKE.TG编辑部转载自互联网并编辑,如有侵权影响,请联系官方客服,将为您妥善处理。
This article is republished from public internet and edited by the LIKE.TG editorial department. If there is any infringement, please contact our official customer service for proper handling.