Leveraging AI to Boost Digital Marketing Performance
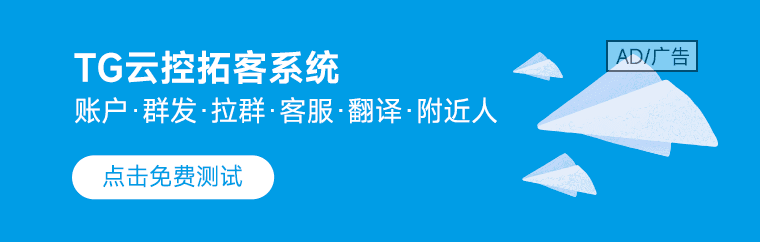
LIKE.TG 成立于2020年,总部位于马来西亚,是首家汇集全球互联网产品,提供一站式软件产品解决方案的综合性品牌。唯一官方网站:www.like.tg
I. In today's rapidly evolving digital landscape, the sheer volume of data available can be overwhelming for marketers. Traditional methods of data analysis and campaign optimization are becoming increasingly inadequate to handle the complexities. This is where Artificial Intelligence (AI) and Machine Learning (ML) step in. These technologies offer promising solutions to automate, personalize, and innovate digital marketing strategies.
II. Supervised Learning Techniques in Digital Marketing
Decision Trees
A decision tree is essentially a graphical representation of possible solutions to a decision, based on certain conditions. Each internal node of this tree-like model represents a "test" on an attribute (such as age, gender, or browsing history), while each branch is the outcome of this test, and each leaf node holds a class label (like a 'Buy' or 'Not Buy' action). In digital marketing, decision trees can serve multiple functions:
1. Customer Segmentation: Marketers can use decision trees to segment their customer base into more targeted and manageable groups, such as 'High Value,' 'Medium Value,' and 'Low Value.'
2. Campaign Optimization: By analyzing the historical data of campaigns and their success rates, decision trees can predict which types of campaigns are more likely to succeed in the future.
3. Risk Assessment: Decision trees can also evaluate the potential risks associated with different marketing strategies, enabling marketers to make informed decisions.
4. Email Targeting: By understanding customer behaviors and preferences, decision trees can help automate the process of sending targeted emails to specific customer segments, thereby improving open rates and conversions.
Naive Bayes
The Naive Bayes algorithm is a probabilistic classifier that applies Bayes' theorem with the assumption of independence between features. In layman's terms, it calculates the probability of an event occurring based on prior knowledge of related conditions. In digital marketing, the applications of Naive Bayes are vast:
1. Email Filtering: Naive Bayes can automatically classify emails into categories like 'Spam' or 'Not Spam' based on the words in the email, making it invaluable for email marketing campaigns.
2. Sentiment Analysis: This technique can analyze customer reviews and feedback to gauge public sentiment, helping brands understand their reputation in the market.
3. Customer Lifetime Value Prediction: By analyzing past customer behaviors, Naive Bayes can predict future behaviors, enabling marketers to identify potentially valuable customers and focus their efforts accordingly.
4. Churn Prediction: It can also predict the likelihood of a customer discontinuing a service or product, allowing businesses to take preventative action.
Nearest Neighbors (k-NN)
The k-Nearest Neighbors (k-NN) algorithm is a type of instance-based learning that stores all available instances (or data points) and classifies new instances based on a similarity measure. This is particularly useful in:
1. Recommendation Systems: By examining a user's past behaviors, k-NN can recommend new products or services that similar users have liked, thereby increasing cross-selling and upselling opportunities.
2. Customer Segmentation: Similar to decision trees, k-NN can also be used to segment customers into different groups based on buying behaviors, demographics, etc.
3. Fraud Detection: In financial marketing, k-NN can help in identifying suspicious activities by comparing them to historical data.
4. Personalization: Personalizing web pages and advertisements for each user based on their behavior and other similar users can drastically improve engagement and conversion rates.
III. Applications in Digital Marketing
Ad Targeting
The power of AI-driven algorithms in digital advertising cannot be overstated. AI sifts through enormous sets of user data—such as browsing history, social media interactions, and purchase behavior—to display the most relevant ads to each user. This level of personalization ensures that businesses aren't wasting ad dollars on uninterested audiences and significantly boosts conversion rates. Moreover, machine learning algorithms continually update their data models based on user interaction with ads, leading to increasingly effective targeting over time.
Social Media Marketing
In today's interconnected world, a robust social media presence is critical for any brand. Advanced machine learning algorithms can analyze vast amounts of data from likes, shares, comments, and even the time spent on posts to offer insights into consumer behavior. This data helps brands craft more engaging and effective social media campaigns. Additionally, AI tools can also help in identifying social media influencers who align with your brand's image and values, creating opportunities for partnerships and collaborations.
Content Creation
The content creation landscape has been dramatically changed by AI. Natural language generation algorithms can automatically produce high-quality articles, blog posts, or video scripts based on a set of predefined rules or templates. This doesn't just speed up the content creation process; it also ensures a consistent brand voice and frees up human resources for more strategic tasks.
Chatbots for Improved Customer Experience (CX)
Chatbots, powered by conversational AI, offer real-time, automated customer service, improving overall customer experience. These chatbots are capable of understanding natural language and can handle a variety of tasks from answering frequently asked questions to guiding a customer through a purchase. This 24/7 service can greatly improve customer satisfaction while reducing the need for large customer service teams.
Predictive Analytics
Predictive analytics employ machine learning algorithms to analyze historical data and predict future outcomes. In digital marketing, this means anticipating customer behaviors, market trends, and even potential risks. With predictive analytics, businesses can create highly personalized marketing campaigns, optimize inventory levels, and even predict sales trends, allowing for more data-backed decision-making processes.
In conclusion, supervised learning techniques and their applications in digital marketing offer a potent combination for businesses aiming to scale their efforts, optimize their campaigns, and stay ahead in a fiercely competitive landscape. With the proper implementation of these technologies, marketers can look forward to more effective campaigns, higher ROIs, and an overall more streamlined marketing operation.
IV. Case Study
An example of a company that has successfully utilized AI/ML in their marketing strategies is Netflix. The streaming service uses machine learning algorithms to analyze viewer preferences and habits, subsequently recommending shows and movies that are likely to be of interest, thereby increasing viewer engagement and subscription rates.
V. Implementing AI/ML in Digital Marketing
Quality and Unbiased Data
Quality data is the backbone of any AI/ML project, especially in digital marketing where consumer behavior is rapidly evolving. The data used to train AI models should be accurate, up-to-date, and comprehensive. Let's explore why quality and unbiased data are crucial:
1. Data Completeness: Incomplete data can lead to misinterpretation and consequently to faulty predictions. For instance, if the data doesn't account for all age groups, the model may be biased toward a specific demographic.
2. Data Accuracy: Incorrect data can compromise the model's performance. For example, incorrect labeling of customer reviews as 'positive' when they are 'negative' could severely affect sentiment analysis.
3. Data Bias: Biased data will produce biased models. This is particularly problematic in marketing where understanding diverse consumer behavior is critical. For example, if the training data is skewed towards a particular gender or age group, the model will make predictions largely beneficial to that group but not necessarily for others.
4. Data Relevance: Data should be aligned with the current trends and consumer behavior. For example, consumer preferences changed dramatically during the COVID-19 pandemic, and models trained on pre-pandemic data would likely be ineffective.
5. Data Volume: While quality trumps quantity, a large volume of quality data can significantly improve the model's performance by enabling it to identify complex patterns and make accurate predictions.
Iterative Training and Testing
Implementing AI/ML in digital marketing is not a 'set and forget' process but an ongoing endeavor. The steps are often cyclical—comprising phases of training, testing, evaluation, and re-training. Here's how it works:
1. Initial Training: Start with a 'training set' of historical data to teach the model about the relationships between different variables.
2. Testing: Once the model is trained, test it on a different 'test set' of data to assess its predictive accuracy.
3. Evaluation: Use metrics such as accuracy, precision, and recall to evaluate the model's performance.
4. Retraining: As new data comes in or when the model's performance starts to decline, go back to the training phase. AI models can lose their effectiveness over time if they're not regularly updated to reflect new trends and data patterns.
Ensemble Techniques
Ensemble methods involve combining several machine learning techniques into one predictive model to decrease variance, increase robustness, and improve accuracy. Some popular ensemble methods used in digital marketing include:
1. Bagging: Bootstrap Aggregating involves creating multiple subsets of the original data, training a model on each, and then averaging the prediction result.
2. Boosting: This technique adjusts the weight of an observation based on the last classification. If an observation was classified incorrectly, it tries to increase the weight of this observation in the next round.
3. Random Forest: This is an ensemble of Decision Trees, generally trained with the 'bagging' method. Random forests are great for dealing with overfitting.
4. Stacking: This involves the combination of various classification (or regression) techniques with the help of another classifier (or regressor) to integrate the predictions.
VI. Measuring Performance in AI-Driven Digital Marketing
Key Metrics
The effectiveness of AI-driven digital marketing initiatives should be measured against key performance indicators (KPIs) such as:
1. Click-Through Rate (CTR): A measure of how effective your ads are at encouraging consumers to take an action. A higher CTR usually indicates more relevant and engaging content.
2. Conversion Rate: This indicates the percentage of visitors who take a desired action, like making a purchase or signing up for a newsletter, compared to the total number of visitors.
3. Return on Investment (ROI): This quantifies the profitability of your digital marketing efforts. It's calculated by dividing the net profit from the marketing activity by the total marketing cost.
A/B Testing
A/B testing is invaluable for understanding the effectiveness of AI-driven marketing strategies in comparison to traditional methods. By running parallel campaigns—one driven by AI and another by conventional methods—you can assess which performs better in real-time. This not only validates the ROI of your AI investment but also provides insights into areas that may require further optimization.
In conclusion, the implementation and performance measurement phases are critical in adopting AI and machine learning for digital marketing. Ensuring quality data, adopting iterative training cycles, and using ensemble techniques can significantly improve the effectiveness of your AI models. Performance measurement using key metrics and A/B testing offers a quantitative approach to assess and refine these models continuously. With these robust practices in place, marketers are better equipped to navigate the complex and ever-changing landscape of digital marketing.
VII. Conclusion
The growing complexity and dynamism of the digital marketing landscape make AI and ML not just helpful but increasingly essential tools. To stay competitive in this data-driven age, marketers must continuously update themselves on these technological advancements and understand how to integrate them effectively into their strategies.
In summary, AI and ML offer unprecedented opportunities to revolutionize digital marketing, from automating mundane tasks to providing valuable customer insights. Therefore, for digital marketers, the question is not whether to integrate AI and ML, but how quickly they can do it to gain a competitive advantage.
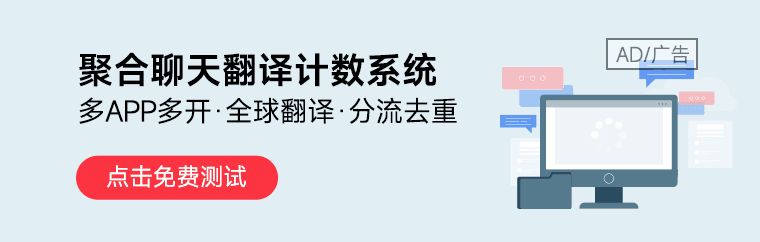
想要了解更多内容,可以关注【LIKE.TG】,获取最新的行业动态和策略。我们致力于为全球出海企业提供有关的私域营销获客、国际电商、全球客服、金融支持等最新资讯和实用工具。住宅静态/动态IP,3500w干净IP池提取,免费测试【IP质量、号段筛选】等资源!点击【联系客服】
本文由LIKE.TG编辑部转载自互联网并编辑,如有侵权影响,请联系官方客服,将为您妥善处理。
This article is republished from public internet and edited by the LIKE.TG editorial department. If there is any infringement, please contact our official customer service for proper handling.