Google Analytics to PostgreSQL: 2 Easy Methods
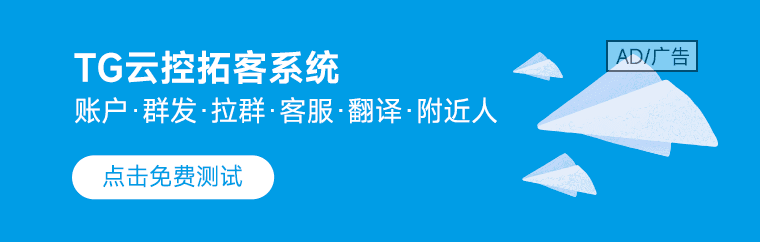
LIKE.TG 成立于2020年,总部位于马来西亚,是首家汇集全球互联网产品,提供一站式软件产品解决方案的综合性品牌。唯一官方网站:www.like.tg
Even though Google provides a comprehensive set of analysis tools to work with data, most organizations will need to pull the raw data into their on-premise database. This is because having it in their control allows them to combine it with their customer and product data to perform a much deeper analysis.
This post is about importing data from Google Analytics to PostgreSQL – one of the very popular relational databases in the market today. This blog covers two approaches for integrating GA with PostgreSQL – The first approach talks about using an automation tool extensively. Alternatively, the blog also covers the manual method for achieving the integration.
Method 1: Using LIKE.TG Data to Connect Google Analytics to PostgreSQL
LIKE.TG is the only real-time ELT No-code Data Pipeline platform that cost-effectively automates flexible data pipelines to your needs. With integration with 150+ Data Sources (40+ free sources), including Google Analytics, we help you not only export data from sources & load data to the destinations but also transform & enrich your data, & make it analysis-ready.
Get Started with LIKE.TG for FreeMethod 2: Using Manual ETL Scripts to Connect Google Analytics to PostgreSQL
Manually coding custom ETL (extract, transform, load) scripts enables precise customization of the data transfer process, but requires more development effort compared to using automated tools.
Method 1: Using LIKE.TG Data to Connect Google Analytics to PostgreSQL
The best way to connect Google Analytics to PostgreSQL is to use a Data Pipeline Platform like LIKE.TG (14-day free trial) that works out of the box. LIKE.TG can help you import data from Google Analytics to PostgreSQL for free in two simple steps:
Step 1: Connect LIKE.TG to Google Analytics to set it up as your source by filling in the Pipeline Name, Account Name, Property Name, View Name, Metrics, Dimensions, and the Historical Import Duration.
Step 2: Load data from Google Analytics to Postgresql by providing your Postgresql databases credentials like Database Host, Port, Username, Password, Schema, and Name along with the destination name.
LIKE.TG will do all the heavy lifting to ensure that your data is securely moved from Google Analytics to PostgreSQL. LIKE.TG automatically handles all the schema changes that may happen at Google Analytics’ end. This ensures that you have a dependable infrastructure that delivers error-free data in PostgreSQL at all points.
Here are a few benefits of using LIKE.TG :
- Easy-to-use Platform: LIKE.TG has a straightforward and intuitive UI to configure the jobs.
- Transformations: LIKE.TG provides preload transformations through Python code. It also allows you to run transformation code for each event in the Data Pipelines you set up. You need to edit the event object’s properties received in the transform method as a parameter to carry out the transformation. LIKE.TG also offers drag and drop transformations like Date and Control Functions, JSON, and Event Manipulation to name a few. These can be configured and tested before putting them to use.
- Real-time Data Transfer: Support for real-time synchronization across a variety of sources and destinations.
- Automatic Schema Mapping: LIKE.TG can automatically detect your source’s schema type and match it with the schema type of your destination.
Method 2: Using Manual ETL Scripts to Connect Google Analytics to PostgreSQL
In this method of moving data from Google Analytics to PostgreSQL, you will first need to get data from Google Analytics followed by accessing Google Reporting API V4 as mentioned in the following section.
Getting data from Google Analytics
Click event data from Google Analytics can be accessed through Reporting API V4. There are two sets of Rest APIs in Reporting API V4 tailor-made for specific use cases.
- Metrics API – These APIs allow users to get aggregated analytics information on user behavior based on available dimensions. Dimensions are the attributes based on which metrics are aggregated. For example, if time is a dimension and the number of users in a specific time will be a metric.
- User Activity API – This API allows you to access information about the activities of a specific user. Knowledge of the user ID is required in this case. To get the user IDs of people accessing your page, you will need to modify some bits in the client-side Google Analytics function that you are going to use and capture the client ID. This information is not exactly available in the Google developer documentation, but there is ample online documentation about it. Ensure you consult the laws and restrictions in your local country before attempting this since its legality will depend on the country’s privacy laws. After changing the client script, you must also register the user ID as a custom dimension in the Google Analytics dashboard.
Google Analytics APIs use oAuth 2.0 as the authentication protocol. Before accessing the APIs, the user first needs to create a service account in the Google Analytics dashboard and generate authentication tokens. Let us review how this can be done.
- Go to the Google service accounts page and select a project. If you have not already created a project, please create one.
- Click on Create Service Account.
- You can ignore the permissions for this exercise.
- On the ‘Grant users access to this service account’ section, click Create key.
- Select JSON as the format for your key.
- Click create a key and you will be prompted with a dialogue to save the key on your local computer. Save the key.
We will be using the information from this step when we actually access the API.
Accessing Google Reporting API V4
Google provides easy-to-use libraries in Python, Java, and PHP to access its reporting APIs. These libraries are the preferred method to download the data since the authentication procedure and the complex JSON response format makes it difficult to access these APIs using command-line tools like CURL
. Detailed documentation of this API can be found here. Here the python library is used to access the API. The following steps and code snippets explain the procedure to load data from Google Analytics to PostgreSQL:
- Step 1: Installing the Python GA Library to Your Environment
- Step 2: Importing the Required Libraries
- Step 3: Initializing the Required Variables for OAuth Authentication
- Step 4: Building the Required Objects
- Step 5: Executing the Method to Get Data
- Step 6: Parsing JSON and Writing the Contents to a CSV File
- Step 7: Loading CSV File to PostgreSQL
Step 1: Installing the Python GA Library to Your Environment
sudo pip install --upgrade google-api-python-client
Before this step, please ensure the python programming environment is already installed and works fine. We will now start writing the script for downloading the data as a CSV file.
Step 2: Importing the Required Libraries
from apiclient.discovery import build
from oauth2client.service_account import ServiceAccountCredentials
Step 3: Initializing the Required Variables for OAuth Authentication
credentials = ServiceAccountCredentials.from_json_keyfile_name(KEY_FILE_LOCATION, SCOPES)
# Build the service object.
analytics = build('analyticsreporting', 'v4', credentials=credentials)
Replace the key file location and view ID with what we obtained in the first service creation step. View ids are the views from which you will be collecting the data. To get the view ID of a particular view that you have already configured, go to the admin section, click on the view that you need, and go to view settings.
Step 4: Building the Required Objects
credentials = ServiceAccountCredentials.from_json_keyfile_name(KEY_FILE_LOCATION, SCOPES)#Build the service object
analytics = build('analyticsreporting', 'v4', credentials=credentials)
Step 5: Executing the Method to Get Data
In this step, you need to execute the method to get the data. The below query is for getting the number of users aggregated by country from the last 7 days.
response = analytics.reports().batchGet(body={
'reportRequests': [
{
'viewId': VIEW_ID,
'dateRanges': [{'startDate': '7daysAgo', 'endDate': 'today'}],
'metrics': [{'expression': 'ga:sessions'}],
'dimensions': [{'name': 'ga:country'}]
}]
}
).execute()
Step 6: Parsing JSON and Writing the Contents to a CSV File
import pandas as pd from pandas.io.json
import json_normalize
reports = response['reports'][0]
columnHeader = reports['columnHeader']['dimensions']
metricHeader = reports['columnHeader']['metricHeader']['metricHeaderEntries'] columns = columnHeader for metric in metricHeader:
columns.append(metric['name'])
data = json_normalize(reports['data']['rows'])
data_dimensions = pd.DataFrame(data['dimensions'].tolist())
data_metrics = pd.DataFrame(data['metrics'].tolist())
data_metrics = data_metrics.applymap(lambda x: x['values'])
data_metrics = pd.DataFrame(data_metrics[0].tolist())
result = pd.concat([data_dimensions, data_metrics], axis=1, ignore_index=True)
result.to_csv('reports.csv')
Save the script and execute it. The result will be a CSV file with the following column:
Id , ga:country, ga:sessions
Step 7: Loading CSV File to PostgreSQL
This file can be directly loaded to a PostgreSQL table using the below command. Please ensure the table is already created
COPY sessions_tableFROM 'reports.csv' DELIMITER ',' CSV HEADER;
The above command assumes you have already created a table named sessions_table.
You now have your google analytics data in your PostgreSQL table. Now that we know how to do get the Google Analytics data using custom code, let’s look into the limitations of using this method.
Limitations of using Manual ETL Scripts to Connect Google Analytics to PostgreSQL
- The above method requires you to write a lot of custom code. Google’s output JSON structure is a complex one and you may have to make changes to the above code according to the data you query from the API.
- This approach is fine for a one-off data load to PostgreSQL, but in a lot of cases, organizations need to do this periodically and merge the data point every day while handling duplicates. This will force you to write a very complex import tool just for Google Analytics.
- The above method addresses only one API that is available for Google Analytics. There are many other available APIs from Google analytics that provide different types of data. An example is a real-time API. All these APIs come with a different output JSON structure and the developers will need to write separate parsers.
- The APIs are rate limited which means the above approach will lead to errors if complex logic is not implemented to throttle the API calls.
A solution to all the above problems is to use a completely managed ETL solution like LIKE.TG which provides a simple click and execute interface to move data from Google Analytics to PostgreSQL.
Use Cases to transfer your Google Analytics 4 (GA4) data to Postgres
There are several advantages to integrating Google Analytics 4 (GA4) data with Postgres. A few use cases are as follows:
- Advanced Analytics: With Postgres’ robust data processing features, you can extract insights from your Google Analytics 4 (GA4) data that are not feasible with Google Analytics 4 (GA4) alone. You can execute sophisticated queries and data analysis on your data.
- Data Consolidation: Syncing to Postgres enables you to centralize your data for a comprehensive picture of your operations and to build up a change data capturing procedure that ensures there are never any inconsistencies in your data again if you’re utilizing Google Analytics 4 (GA4) together with many other sources.
- Analysis of Historical Data: Historical data in Google Analytics 4 (GA4) is limited. Data sync with Postgres enables long-term data storage and longitudinal trend analysis.
- Compliance and Data Security: Strong data security protections are offered by Postgres. Syncing Google Analytics 4 (GA4) data with Postgres enables enhanced data governance and compliance management while guaranteeing the security of your data.
- Scalability: Growing enterprises with expanding Google Analytics 4 (GA4) data will find Postgres to be an appropriate choice since it can manage massive amounts of data without compromising speed.
- Machine Learning and Data Science: You may apply machine learning models to your data for predictive analytics, consumer segmentation, and other purposes if you have Google Analytics 4 (GA4) data in Postgres.
- Reporting and Visualization: Although Google Analytics 4 (GA4) offers reporting capabilities, more sophisticated business intelligence alternatives may be obtained by connecting to Postgres using data visualization tools like Tableau, PowerBI, and Looker (Google Data Studio). Airbyte can automatically convert your Google Analytics 4 (GA4) table to a Postgres table if needed.
Conclusion
This blog discusses the two methods you can deploy to connect Google Analytics to PostgreSQL seamlessly. While the custom method gives the user precise control over data, using automation tools like LIKE.TG can solve the problem easily.
Visit our Website to Explore LIKE.TGWhile Google Analytics used to offer free website analytics, it’s crucial to remember that the program is currently built on a subscription basis. Presently, the free version is called Google Analytics 360, and it still offers insightful data on user behavior and website traffic. In addition to Google Analytics, LIKE.TG natively integrates with many other applications, including databases, marketing and sales applications, analytics applications, etc., ensuring that you have a reliable partner to move data to PostgreSQL at any point.
Want to take LIKE.TG for a ride? Sign Up for a 14-day free trial and simplify your Data Integration process. Do check out the pricing details to understand which plan meets all your business needs.
Tell us in the comments about your experience of connecting Google Analytics to PostgreSQL!
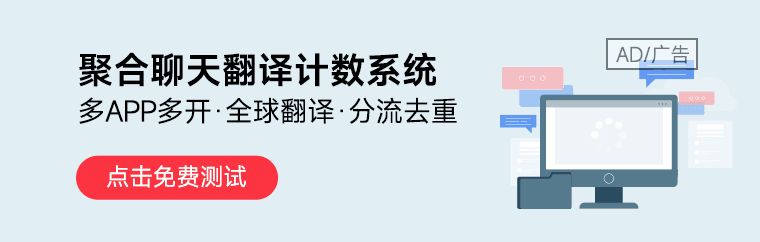
LIKE.TG 专注全球社交流量推广,致力于为全球出海企业提供有关的私域营销获客、国际电商、全球客服、金融支持等最新资讯和实用工具。免费领取【WhatsApp、LINE、Telegram、Twitter、ZALO】等云控系统试用;点击【联系客服】 ,或关注【LIKE.TG出海指南频道】、【LIKE.TG生态链-全球资源互联社区】了解更多最新资讯
本文由LIKE.TG编辑部转载自互联网并编辑,如有侵权影响,请联系官方客服,将为您妥善处理。
This article is republished from public internet and edited by the LIKE.TG editorial department. If there is any infringement, please contact our official customer service for proper handling.