Connect Microsoft SQL Server to BigQuery in 2 Easy Methods
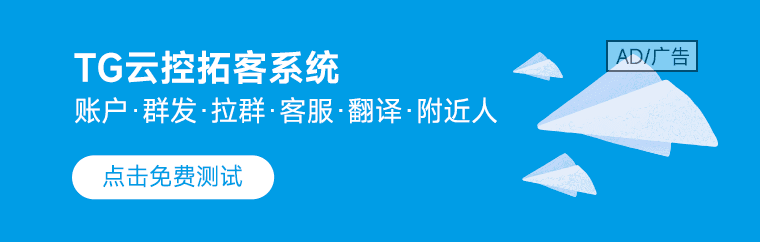
LIKE.TG 成立于2020年,总部位于马来西亚,是首家汇集全球互联网产品,提供一站式软件产品解决方案的综合性品牌。唯一官方网站:www.like.tg
Are you looking to perform a detailed analysis of your data without having to disturb the production setup on SQL Server? In that case, moving data from SQL Server to a robust data warehouse like Google BigQuery is the right direction to take.
This article aims to guide you with steps to move data from Microsoft SQL Server to BigQuery, shed light on the common challenges, and assist you in navigating through them. You will explore two popular methods that you can utilize to set up Microsoft SQL Server to BigQuery migration.
Methods to Set Up Microsoft SQL Server to BigQuery Integration
Majorly, there are two ways to migrate your data from Microsoft SQL to BigQuery.
Method 1: Manual ETL Process to Set Up Microsoft SQL Server to BigQuery Integration
This method involves the use of SQL Server Management Studio (SMSS) for setting up the integrations. Moreover, it requires you to convert the data into CSV format and then replicate the data. It requires a lot of engineering bandwidth and knowledge of SQL queries.
Method 2: Using LIKE.TG Data to Set Up Microsoft SQL Server to BigQuery Integration
Integrate your data effortlessly from Microsoft SQL Server to BigQuery in just two easy steps using LIKE.TG Data. We take care of your data while you focus on more important things to boost your business.
Get Started with LIKE.TG for FreeMethod 1: Using LIKE.TG Data to Set Up Microsoft SQL Server to BigQuery Integration
LIKE.TG is a no-code fully managed data pipeline platform that completely automates the process of not only loading data from your desired source but also enriching the data and transforming it into an analysis-ready form. Its fault-tolerant architecture ensures that the data is handled in a secure, consistent manner with zero data loss.
Sign up here for a 14-Day Free Trial!The steps to load data from Microsoft SQL Server to BigQuery using LIKE.TG Data are as follows:
- Connect your Microsoft SQL Server account to LIKE.TG ’s platform. LIKE.TG has an in-built Microsoft SQL Server Integration that connects to your account within minutes.
Click here to read more about using SQL Server as a Source connector with LIKE.TG .
- Select Google BigQuery as your destination and start moving your data.
Click here to read more about using BigQuery as a destination connector with LIKE.TG .
With this, you have successfully set up Microsoft SQL Server to BigQuery Integration using LIKE.TG Data.
Here are more reasons to try LIKE.TG :
- Data Transformation: It provides a simple interface to perfect, modify, and enrich the data you want to transfer.
- Schema Management: LIKE.TG can automatically detect the schema of the incoming data and maps it to the destination schema.
- Incremental Data Load: LIKE.TG allows you to migrate SQL Server to BigQuery data that has been modified in real-time. This ensures efficient utilization of bandwidth on both ends.
Method 2: Manual ETL Process to Set Up Microsoft SQL Server to BigQuery Integration
The steps to execute the custom code are as follows:
- Step 1: Export the Data from SQL Server using SQL Server Management Studio (SSMS)
- Step 2: Upload to Google Cloud Storage
- Step 3: Upload to BigQuery from Google Cloud Storage (GCS)
- Step 4: Update the Target Table in BigQuery
Step 1: Export the Data from SQL Server using SQL Server Management Studio (SSMS)
SQL Server Management Studio(SSMS) is a free tool built by Microsoft to enable a coordinated environment for managing any SQL infrastructure. SSMS is used to query, design, and manage your databases from your local machine. We are going to be using the SSMS to extract our data in Comma Separated Value(CSV) format in the steps below.
- Install SSMS if you don’t have it on your local machine. You can install it here.
- Open SSMS and connect to a Structured Query Language (SQL) instance. From the object explorer window, select a database and right-click on the Tasks sub-menu, and choose the Export data option.
- The welcome page of the Server Import and Export Wizard will be opened. Click the Next icon to proceed to export the required data.
- You will see a window to choose a data source. Select your preferred data source.
- In the Server name dropdown list, select a SQL Server instance.
- In the Authentication section select authentication for the data source connection. Next, from the Database drop-down box, select a database from which data will be copied. Once you have filled the drop-down list select ‘Next‘.
- The next window is the choose the destination window. You will need to specify the location from which the data will be copied in the SQL server. Under the destination, the drop-down box selects the Flat File destination item.
- In the File name box, establish the CSV file where the data from the SQL database will be exported to and select the next button.
- The next window you will see is the Specify Table Copy or Query window, choose the Copy data from one or more tables or views to get all the data from the table.
- Next, you’d see a Configure Flat File Destination window, select the table from the source table to export the data to the CSV file you specified earlier.
- At this point your file would have been exported, to view the exported file click on preview. To have a sneak peek of the data you just exported.
- Complete the exportation process by hitting ‘Next‘. The save and run package window will pop up, click on ‘Next‘.
- The Complete Wizard window will appear next, it will give you an overview of all the choices you made during the exporting process. To complete the exportation process, hit on ‘Finish‘.
- The exported CSV file will be found in Local Drive, where you specified for it to be exported.
Step 2: Upload to Google Cloud Storage
After completing the exporting process to your local machine, the next step in SQL Server to BigQuery is to transfer the CSV file to Google Cloud Storage(GCS). There are various ways of achieving this, but for the purpose of this blog post, let’s discuss the following methods.
Method 1: Using Gsutil
gsutil is a GCP tool that uses Python programming language. It gives you access to GCS from the command line. To initiate gsutil follow this quickstart link. gsutil provides a unique way to upload a file to GCS from your local machine. To create a bucket in which you copy your file to:
gsutil mb gs://my-new-bucketThe new bucket created is called “my-new-bucket“. Your bucket name must be globally unique. If successful the command returns:
Creating gs://my-new-bucket/...To copy your file to GCS:
gsutil cp export.csv gs://my-new-bucket/destination/export.csvIn this command, “export.csv” refers to the file you want to copy. “gs://my-new-bucket” represents the GCS bucket you created earlier. Finally, “destination/export.csv” specifies the destination path and filename in the GCS bucket where the file will be copied to.
Method 2: Using Web Console
The web console is another alternative you can use to upload your CSV file to the GCS from your local machine. The steps to use the web console are outlined below.
- First, you will have to log in to your GCP account. Toggle on the hamburger menu which displays a drop-down menu. Select Storage and click on the Browser on the left tab.
- In order to store the file that you would upload from your local machine, create a new bucket. Make sure the name chosen for the browser is globally unique.
- The bucket you just created will appear on the window, click on it and select upload files. This action will direct you to your local drive where you will need to choose the CSV file you want to upload to GCS.
- As soon as you start uploading, a progress bar is shown. The bar disappears once the process has been completed. You will be able to find your file in the bucket.
Step 3: Upload Data to BigQuery From GCS
BigQuery is where the data analysis you need will be carried out. Hence you need to upload your data from GCS to BigQuery. There are various methods that you can use to upload your files from GCS to BigQuery. Let’s discuss 2 methods here:
Method 1: Using the Web Console UI
- The first point of call when using the Web UI method is to select BigQuery under the hamburger menu on the GCP home page.
- Select the “Create a new dataset” icon and fill in the corresponding drop-down menu.
- Create a new table under the data set you just created to store your CSV file.
- In the create table page –> in the source data section: Select GCS to browse your bucket and select the CSV file you uploaded to GCS – Make sure your File Format is set to CSV.
- Fill in the destination tab and the destination table.
- Under schema, click on the auto-detect schema.
- Select create a table.
- After creating the table, click on the destination table name you created to view your exported data file.
- Using Command Line Interface, the Activate Cloud Shell icon shown below will take you to the command-line interface. You can also use the auto-detect feature to specify your schema.
Your schema can be specified using the Command-Line. An example is shown below
bq load --autodetect --source_format=CSV --schema=schema.json your_dataset.your_table gs://your_bucket/your_file.csvIn the above example, schema.json refers to the file containing the schema definition for your CSV file. You can customize the schema by modifying the schema.json file to match the structure of your data.
There are 3 ways to write to an existing table on BigQuery. You can make use of any of them to write to your table. Illustrations of the options are given below
1. Overwrite the data
To overwrite the data in an existing table, you can use the --replace flag in the bq command. Here’s an example code:
bq load --replace --source_format=CSV your_dataset.your_table gs://your_bucket/your_file.csvIn the above code, the --replace flag ensures that the existing data in the table is replaced with the new data from the CSV file.
2. Append the table
To append data to an existing table, you can use the --noreplace flag in the bq command. Here’s an example code:
bq load --noreplace --source_format=CSV your_dataset.your_table gs://your_bucket/your_file.csvThe --noreplace flag ensures that the new data from the CSV file is appended to the existing data in the table.
3. Add a new field to the target table. An extra field will be added to the schema.
To add a new field (column) to the target table, you can use the bq update command and specify the schema changes. Here’s an example code:
bq update your_dataset.your_table --schema schema.jsonIn the above code, schema.json refers to the file containing the updated schema definition with the new field. You need to modify the schema.json file to include the new field and its corresponding data type.
Please note that these examples assume you have the necessary permissions and have set up the required authentication for interacting with BigQuery.
Step 4: Update the Target Table in BigQuery
GCS acts as a staging area for BigQuery, so when you are using Command-Line to upload to BigQuery, your data will be stored in an intermediate table. The data in the intermediate table will need to be updated for the effect to be shown in the target table.
There are two ways to update the target table in BigQuery.
- Update the rows in the final table and insert new rows from the intermediate table.
In the above code, final_table refers to the name of your target table, and intermediate_data_table refers to the name of the intermediate table where your data is initially loaded.
2. Delete all the rows from the final table which are in the intermediate table.
DELETE FROM final_table WHERE id IN (SELECT id FROM intermediate_data_table);In the above code, final_table refers to the name of your target table, and intermediate_data_table refers to the name of the intermediate table where your data is initially loaded.
Please make sure to replace final_table and intermediate_data_table with the actual table names, you are working with.
This marks the completion of SQL Server to BigQuery connection. Now you can seamlessly sync your CSV files into GCP bucket in order to integrate SQL Server to BigQuery and supercharge your analytics to get insights from your SQL Server database.
Limitations of Manual ETL Process to Set Up Microsoft SQL Server to BigQuery Integration
Businesses need to put systems in place that will enable them to gain the insights they need from their data. These systems have to be seamless and rapid. Using custom ETL scripts to connect MS SQL Server to BigQuery has the following limitations that will affect the reliability and speed of these systems:
- Writing custom code is only ideal if you’re looking to move your data once from Microsoft SQL Server to BigQuery.
- Custom ETL code does not scale well with stream and real-time data. You will have to write additional code to update your data. This is far from ideal.
- When there’s a need to transform or encrypt your data, custom ETL code fails as it will require you to add additional processes to your pipeline.
- Maintaining and managing a running data pipeline such as this will need you to invest heavily in engineering resources.
- BigQuery does not ensure data consistency for external data sources, as changes to the data may cause unexpected behavior while a query is running.
- The data set’s location must be in the same region or multi-region as the Cloud Storage Bucket.
- CSV files cannot contain nested or repetitive data since the format does not support it.
- When utilizing a CSV, including compressed and uncompressed files in the same load job is impossible.
- The maximum size of a gzip file for CSV is 4 GB.
While writing code to move data from SQL Server to BigQuery looks like a no-brainer, in the beginning, the implementation and management are much more nuanced than that. The process has a high propensity for errors which will, in turn, have a huge impact on the data quality and consistency.
Benefits of Migrating your Data from SQL Server to BigQuery
Integrating data from SQL Server to BigQuery offers several advantages. Here are a few usage scenarios:
- Advanced Analytics: The BigQuery destination’s extensive data processing capabilities allow you to run complicated queries and data analyses on your SQL Server data, deriving insights that would not be feasible with SQL Server alone.
- Data Consolidation: If you’re using various sources in addition to SQL Server, synchronizing to a BigQuery destination allows you to centralize your data for a more complete picture of your operations, as well as set up a change data collection process to ensure that there are no discrepancies in your data again.
- Historical Data Analysis: SQL Server has limitations with historical data. Syncing data to the BigQuery destination enables long-term data retention and study of historical trends over time.
- Data Security and Compliance: The BigQuery destination includes sophisticated data security capabilities. Syncing SQL Server data to a BigQuery destination secures your data and enables comprehensive data governance and compliance management.
- Scalability: The BigQuery destination can manage massive amounts of data without compromising speed, making it a perfect solution for growing enterprises with expanding SQL Server data.
Conclusion
This article gave you a comprehensive guide to setting up Microsoft SQL Server to BigQuery integration using 2 popular methods. It also gave you a brief overview of Microsoft SQL Server and Google BigQuery. There are also certain limitations associated with the custom ETL method to connect SQL server to Bigquery.
With LIKE.TG , you can achieve simple and efficient Data Replication from Microsoft SQL Server to BigQuery. LIKE.TG can help you move data from not just SQL Server but 150s of additional data sources.
Visit our Website to Explore LIKE.TGBusinesses can use automated platforms like LIKE.TG Data to set this integration and handle the ETL process. It helps you directly transfer data from a source of your choice to a Data Warehouse, Business Intelligence tools, or any other desired destination in a fully automated and secure manner without having to write any code and will provide you with a hassle-free experience of connecting your SQL Server to BigQuery instance.
Want to try LIKE.TG ? Sign Up for a 14-day free trial and experience the feature-rich LIKE.TG suite first hand. Have a look at our unbeatable LIKE.TG Pricing, which will help you choose the right plan for you.
Share your experience of loading data from Microsoft SQL Server to BigQuery in the comment section below.
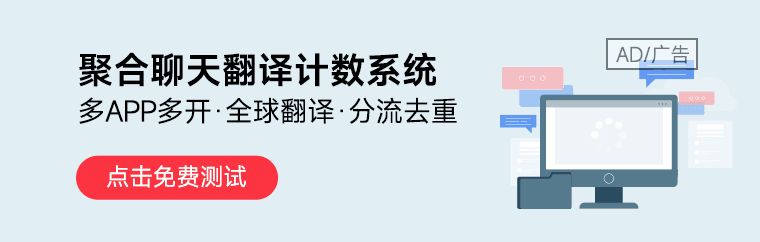
LIKE.TG 专注全球社交流量推广,致力于为全球出海企业提供有关的私域营销获客、国际电商、全球客服、金融支持等最新资讯和实用工具。免费领取【WhatsApp、LINE、Telegram、Twitter、ZALO】等云控系统试用;点击【联系客服】 ,或关注【LIKE.TG出海指南频道】、【LIKE.TG生态链-全球资源互联社区】了解更多最新资讯
本文由LIKE.TG编辑部转载自互联网并编辑,如有侵权影响,请联系官方客服,将为您妥善处理。
This article is republished from public internet and edited by the LIKE.TG editorial department. If there is any infringement, please contact our official customer service for proper handling.
效率工具客服坐席客服系统坐席多开