DynamoDB to Snowflake: 3 Easy Steps to Move Data
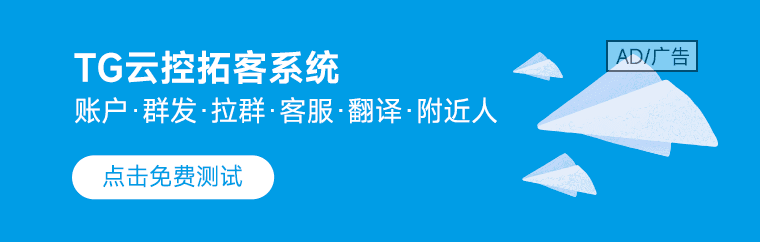
LIKE.TG 成立于2020年,总部位于马来西亚,是首家汇集全球互联网产品,提供一站式软件产品解决方案的综合性品牌。唯一官方网站:www.like.tg
If you’re looking for DynamoDB Snowflake migration, you’ve come to the right place. Initially, the article provides an overview of the two Database environments while briefly touching on a few of their nuances. Later on, it dives deep into what it takes to implement a solution on your own if you are to attempt the ETL process of setting up and managing a Data Pipeline that moves data from DynamoDB to Snowflake.
The article wraps up by pointing out some of the challenges associated with developing a custom ETL solution for loading data from DynamoDB to Snowflake and why it might be worth the investment in having an ETL Cloud service provider, LIKE.TG , implement and manage such a Data Pipeline for you.
Overview of DynamoDB and Snowflake
DynamoDB is a fully managed, NoSQL Database that stores data in the form of key-value pairs as well as documents. It is part of Amazon’s Data Warehousing suite of services called Amazon Web Services (AWS). DynamoDB is known for its super-fast data processing capabilities that boast the ability to process more than 20 million requests per second. In terms of backup management for Database tables, it has the option for On-Demand Backups, in addition to Periodic or Continuous Backups.
Snowflake is a fully managed, Cloud Data Warehousing solution available to customers in the form of Software-as-a-Service (SaaS) or Database-as-a-Service (DaaS). Snowflake follows the standard ANSI SQL protocol that supports fully Structured as well as Semi-Structured data like JSON, Parquet, XML, etc. It is highly scalable in terms of the number of users and computing power while offering pricing at per-second levels of resource usage.
There are two popular methods to perform Data Migration from DynamoDB to Snowflake:
Method 1: Build Custom ETL Scripts to move from DynamoDB data to Snowflake
Method 2: Implement an Official Snowflake ETL Partner such as Hevo Data.
This post covers the first approach in great detail. The blog also highlights the Challenges of Moving Data from DynamoDB to Snowflake using Custom ETL and discusses the means to overcome them.
So, read along to understand the steps to export data from DynamoDB to Snowflake in detail.
Moving Data from DynamoDB to Snowflake using Custom ETL
In this section, you understand the steps to create a Custom Data Pipeline to load data from DynamoDB to Snowflake.
A Data Pipeline that enables the flow of data from DynamoDB to Snowflake can be characterized through the following steps –
- Step 1: Set Up Amazon S3 to Receive Data from DynamoDB
- Step 2: Export Data from DynamoDB to Amazon S3
- Step 3: Copy Data from Amazon S3 to Snowflake Tables
Step 1: Set Up Amazon S3 to Receive Data from DynamoDB
Amazon S3 is a fully managed Cloud file storage, also part of AWS used to export to and import files from, for a variety of purposes. In this use case, S3 is required to temporarily store the data files coming out of DynamoDB before they are loaded into Snowflake tables. To store a data file on S3, one has to create an S3 bucket first. Buckets are placeholders for all objects that are to be stored on Amazon S3. Using the AWS command-line interface, the following is an example command that can be used to create an S3 bucket:
$aws s3api create-bucket --bucket dyn-sfl-bucket --region us-east-1
Name of the bucket – dyn-sfl-bucket
It is not necessary to create folders in a bucket before copying files over, however, it is a commonly adopted practice, as one bucket can hold a variety of information and folders help with better organization and reduce clutter. The following command can be used to create folders –
aws s3api put-object --bucket dyn-sfl-bucket --key dynsfl/
Folder name – dynsfl
Step 2: Export Data from DynamoDB to Amazon S3
Once an S3 bucket has been created with the appropriate permissions, you can now proceed to export data from DynamoDB. First, let’s look at an example of exporting a single DynamoDB table onto S3. It is a fairly quick process, as follows:
- First, you export the table data into a CSV file as shown below.
aws dynamodb scan --table-name YOURTABLE --output text > outputfile.txt
The above command would produce a tab-separated output file which can then be easily converted to a CSV file.
- Later, this CSV file (testLIKE.TG .csv, let’s say) could then be uploaded to the previously created S3 bucket using the following command:
$aws s3 cp testLIKE.TG .csv s3://dyn-sfl-bucket/dynsfl/
In reality, however, one would need to export tens of tables, sequentially or parallelly, in a repetitive fashion at fixed intervals (ex: once in a 24 hour period). For this, Amazon provides an option to create Data Pipelines. Here is an outline of the steps involved in facilitating data movement from DynamoDB to S3 using a Data Pipeline:
- Create and validate the Pipeline. The following command can be used to create a Data Pipeline:
$aws datapipeline create-pipeline --name dyn-sfl-pipeline --unique-id token { "pipelineId": "ex-pipeline111" }
- The next step is to upload and validate the Pipeline using a pre-created Pipeline file in JSON format
$aws datapipeline put-pipeline-definition --pipeline-id ex-pipeline111 --pipeline-definition file://dyn-sfl-pipe-definition.json
- Activate the Pipeline. Once the above step is completed with no validation errors, this pipeline can be activated using the following –
$aws datapipeline activate-pipeline --pipeline-id ex-pipeline111
- Monitor the Pipeline run and verify the data export. The following command shows the execution status:
$aws datapipeline list-runs --pipeline-id ex-pipeline111
- Once the ‘Status Ended’ section indicates completion of the execution, go over to the S3 bucket s3://dyn-sfl-bucket/dynsfl/ and check to see if the required export files are available.
Defining the Pipeline file dyn-sfl-pipe-definition.json can be quite time consuming as there are many things to be defined. Here is a sample file indicating some of the objects and parameters that are to be defined:
{
"objects": [
{
"myComment": "Write a comment here to describe what this section is for and how things are defined",
"id": "dyn-to-sfl",
"failureAndRerunMode":"cascade",
"resourceRole": "DataPipelineDefaultResourceRole",
"role": "DataPipelineDefaultRole",
"pipelineLogUri": "s3://",
"schedule": {
"ref": "DefaultSchedule"
}
"scheduleType": "cron",
"name": "Default"
"id": "Default"
},
{
"type": "Schedule",
"id": "dyn-to-sfl",
"startDateTime" : "2019-06-10T03:00:01"
"occurrences": "1",
"period": "24 hours",
"maxActiveInstances" : "1"
}
],
"parameters": [
{
"description": "S3 Output Location",
"id": "DynSflS3Loc",
"type": "AWS::S3::ObjectKey"
},
{
"description": "Table Name",
"id": "LIKE.TG _dynamo",
"type": "String"
}
]
}
As you can see in the above file definition, it is possible to set the scheduling parameters for the Pipeline execution. In this case, the start date and time are set to June 1st, 2019 early morning and the execution frequency is set to once a day.
Step 3: Copy Data from Amazon S3 to Snowflake Tables
Once the DynamoDB export files are available on S3, they can be copied over to the appropriate Snowflake tables using a ‘COPY INTO’ command that looks similar to a copy command used in a command prompt. It has a ‘source’, a ‘destination’ and a set of parameters to further define the specific copy operation. A couple of ways to use the COPY command are as follows:
File format:
copy into LIKE.TG _sfl
from s3://dyn-sfl-bucket/dynsfl/testLIKE.TG .csv
credentials=(aws_key_id='ABC123' aws_secret_key='XYZabc)
file_format = (type = csv field_delimiter = ',');
Pattern Matching:
copy into LIKE.TG _sfl
from s3://dyn-sfl-bucket/dynsfl/
credentials=(aws_key_id='ABC123' aws_secret_key=''XYZabc)
pattern='*LIKE.TG *.csv';
Just like before, the above is an example of how to use individual COPY commands for quick Ad Hoc Data Migration, however, in reality, this process will be automated and has to be scalable. In that regard, Snowflake provides an option to automatically detect and ingest staged files when they become available in the S3 buckets. This feature is called Automatic Data Loading using Snowpipe.
Here are the main features of a Snowpipe:
- Snowpipe can be set up in a few different ways to look for newly staged files and load them based on a pre-defined COPY command. An example here is to create a Simple-Queue-Service notification that can trigger the Snowpipe data load.
- In the case of multiple files, Snowpipe appends these files into a loading queue. Generally, the older files are loaded first, however, this is not guaranteed to happen.
- Snowpipe keeps a log of all the S3 files that have already been loaded – this helps it identify a duplicate data load and ignore such a load when it is attempted.
Hurray!! You have successfully loaded data from DynamoDB to Snowflake using Custom ETL Data Pipeline.
Challenges of Moving Data from DynamoDB to Snowflake using Custom ETL
Now that you have an idea of what goes into developing a Custom ETL Pipeline to move DynamoDB data to Snowflake, it should be quite apparent that this is not a trivial task.
To further expand on that, here are a few things that highlight the intricacies and complexities of building and maintaining such a Data Pipeline:
- DynamoDB export is a heavily involved process, not least because of having to work with JSON files. Also, when it comes to regular operations and maintenance, the Data Pipeline should be robust enough to handle different types of data errors.
- Additional mechanisms need to be put in place to handle incremental data changes from DynamoDB to S3, as running full loads every time is very inefficient.
- Most of this process should be automated so that real-time data is available as soon as possible for analysis. Setting everything up with high confidence in the consistency and reliability of such a Data Pipeline can be a huge undertaking.
- Once everything is set up, the next thing a growing data infrastructure is going to face is scaling. Depending on the growth, things can scale up really quickly and if the existing mechanisms are not built to handle this scale, it can become a problem.
Using a No-Code automated Data Pipeline like LIKE.TG (Official Snowflake ETL Partner), you can move data from DynamoDB to Snowflake in real-time. Since LIKE.TG is fully managed, the setup and implementation time is next to nothing. You can replicate DynamoDB to Snowflake using LIKE.TG ’s visual interface in 3 simple steps:
- Connect to your DynamoDB database
- Select the replication mode: (i) Full dump (ii) Incremental load for append-only data (iii) Incremental load for mutable data
- Configure the Snowflake database and watch your data load in real-time
LIKE.TG will now move your data from DynamoDB to Snowflake in a consistent, secure, and reliable fashion. In addition to DynamoDB, LIKE.TG can load data from a multitude of other data sources including Databases, Cloud Applications, SDKs, and more. This allows you to scale up on demand and start moving data from all the applications important for your business.
SIGN UP HERE FOR A 14-DAY FREE TRIAL!Conclusion
In conclusion, this article offers a step-by-step description of creating Custom Data Pipelines to move data from DynamoDB to Snowflake. It highlights the challenges a Custom ETL solution brings along with it. In a real-life scenario, this would typically mean allocating a good number of human resources for both the development and maintenance of such Data Pipelines to ensure consistent, day-to-day operations. Knowing that it might be worth exploring and investing in a reliable cloud ETL service provider, LIKE.TG offers comprehensive solutions to use cases such as this one and many more.
VISIT OUR WEBSITE TO EXPLORE LIKE.TGLIKE.TG Data is a No-Code Data Pipeline that offers a faster way to move data from 150+ Data Sources including 50+ Free Sources, into your Data Warehouse like Snowflake to be visualized in a BI tool. LIKE.TG is fully automated and hence does not require you to code.
Want to take LIKE.TG for a spin?
SIGN UP and experience the feature-rich LIKE.TG suite first hand.
What are your thoughts about moving data from DynamoDB to Snowflake? Let us know in the comments.
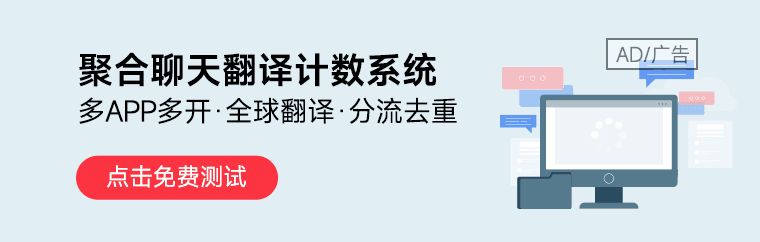
LIKE.TG 专注全球社交流量推广,致力于为全球出海企业提供有关的私域营销获客、国际电商、全球客服、金融支持等最新资讯和实用工具。免费领取【WhatsApp、LINE、Telegram、Twitter、ZALO】等云控系统试用;点击【联系客服】 ,或关注【LIKE.TG出海指南频道】、【LIKE.TG生态链-全球资源互联社区】了解更多最新资讯
本文由LIKE.TG编辑部转载自互联网并编辑,如有侵权影响,请联系官方客服,将为您妥善处理。
This article is republished from public internet and edited by the LIKE.TG editorial department. If there is any infringement, please contact our official customer service for proper handling.