Sentiment Analysis: An Essential Tool for Understanding Customers
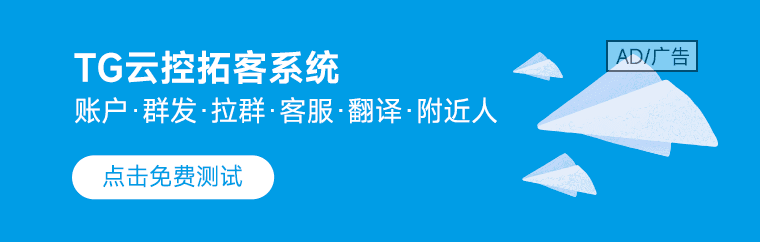
LIKE.TG 成立于2020年,总部位于马来西亚,是首家汇集全球互联网产品,提供一站式软件产品解决方案的综合性品牌。唯一官方网站:www.like.tg
Introduction
In today's digital age, businesses have access to more customer data than ever before. Social media platforms, review sites, surveys, and more provide a wealth of insights into how customers feel about brands, products, and services. However, sifting through all of this unstructured data manually is inefficient and time-consuming. This is where sentiment analysis comes in.
Sentiment analysis, also known as opinion mining, refers to the use of natural language processing and text analysis techniques to systematically identify, extract, and quantify subjective information in textual data. The overarching goal is to determine the attitude, opinions, or emotions within a text to gain insights into the broader sentiment toward a particular topic.
For businesses, sentiment analysis is invaluable for understanding the voice of the customer at scale. Whether analyzing customer service transcripts, social media conversations, product reviews or survey responses, sentiment analysis provides actionable insights to guide everything from reputation management to product development.
Types of Sentiment Analysis
There are three main approaches to sentiment analysis:
Machine Learning-Based Sentiment Analysis
This approach uses machine learning algorithms that are trained on large datasets of text that has been manually annotated for sentiment. The algorithms learn to associate certain words, phrases, and linguistic patterns with positive, negative or neutral sentiment. A key advantage of machine learning is its ability to understand sentiment in the context of how words appear together in a sentence, rather than just the presence of individual words. However, machine learning models require extensive training data to be accurate.
Rule-Based Sentiment Analysis
This approach relies on manually created lexicons of words classified as conveying positive, negative or neutral sentiment. To analyze a text, the system counts the number of positive and negative words based on the lexicon to determine an overall sentiment score. The main advantage of rule-based systems is interpretability - you can understand exactly why a text was classified as positive or negative. However, rule-based systems struggle to deal with ambiguities in language and require extensive lexicons to be effective.
Aspect-Based Sentiment Analysis
This approach seeks to identify the sentiment toward specific aspects of a product or service that is discussed in a text. For example, in a review of a smartphone, the battery life may be discussed positively while the screen quality is criticized. Aspect-based analysis provides more nuanced insights compared to document-level sentiment analysis. However, it requires more advanced natural language processing to extract product aspects accurately.
Challenges with Sentiment Analysis
While sentiment analysis has come a long way, several challenges remain:
Contextual Errors
Words can convey different sentiments depending on context. For example, "unpredictable ride" could be positive when describing a rollercoaster but negative for vehicle reviews. Sentiment analysis systems still struggle to fully grasp contextual meaning.
Sarcasm and Ambiguity
Language is often ambiguous. Sarcasm and irony are especially problematic for sentiment analysis systems to detect accurately. Creative linguistic expressions of sentiment also pose challenges.
Negation
The presence of negation words like "not" can flip the sentiment of an otherwise positive or negative phrase. Accurately handling negation remains an active area of research.
Inefficient Language Detection
Most sentiment analysis tools are focused on English, but multilingual analysis is critical for global brands. Detecting sentiment across different languages, dialects and local idioms presents difficulties.
Analyzing Emojis and Images
With visual content dominating social media, analyzing emojis, memes and images for sentiment is growing increasingly important. But this remains a technically difficult problem.
Potential Biases
Biases in training data and subjectivity in lexicon creation can lead to inconsistent or misleading sentiment analysis. Ongoing evaluations are needed to minimize bias.
Use Cases for Sentiment Analysis
Despite these challenges, sentiment analysis delivers immense value for businesses across many use cases:
Monitoring Brand Reputation and Health
Analyzing sentiment on social media, review sites and news provides invaluable insights into brand reputation. Sentiment analysis helps identify PR crises, negative publicity and opportunities for positive brand engagement. Tracking sentiment over time is a proxy for overall brand health.
Identifying Urgent Customer Queries
Sentiment analysis allows customer service teams to automatically prioritize and respond first to the most urgent or dissatisfied customer inquiries based on the sentiment detected in text.
Evaluating Marketing Campaigns
The performance of marketing campaigns, product launches and other initiatives can be evaluated by analyzing sentiment in social conversations, reviews and media coverage. Sudden shifts in sentiment may indicate a misguided campaign.
Getting Product/Service Feedback
Mining reviews and social media for fine-grained, aspect-based sentiment analysis provides detailed insights into how customers feel about specific product attributes and features. This fuels innovation.
Competitor Analysis
Performing comparative sentiment analysis on competitors helps identify their weaknesses, brand crises and areas where your brand has an opportunity to excel in consumers' eyes.
Tips for Effective Sentiment Analysis
To maximize the value gained from sentiment analysis, keep these tips in mind:
- Benchmark sentiment scores to establish a baseline for comparison over time. Set goals for ideal positivity levels.
- Monitor shifts in sentiment through real-time dashboards and set alerts for decreasing sentiment scores. Dig deeper into negative sentiment drivers.
- Resolve issues proactively by responding swiftly to negative feedback and mitigating reputational threats before they spiral.
- Compare competitor sentiment on an ongoing basis to find strategic advantages. Learn from their mistakes.
- Communicate insights effectively to leadership through clear reports and data visualizations illustrating the "voice of the customer."
In summary, sentiment analysis is an indispensable tool for extracting insights from customer conversations to guide brand strategy and growth. While some language complexities persist, the value generated by understanding sentiment at scale makes overcoming these challenges well worth the effort. Leveraging sentiment analysis effectively will only grow more vital for business success as consumer data expands.
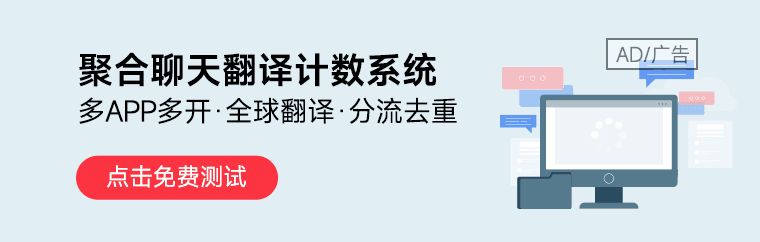
想要了解更多内容,可以关注【LIKE.TG】,获取最新的行业动态和策略。我们致力于为全球出海企业提供有关的私域营销获客、国际电商、全球客服、金融支持等最新资讯和实用工具。住宅静态/动态IP,3500w干净IP池提取,免费测试【IP质量、号段筛选】等资源!点击【联系客服】
本文由LIKE.TG编辑部转载自互联网并编辑,如有侵权影响,请联系官方客服,将为您妥善处理。
This article is republished from public internet and edited by the LIKE.TG editorial department. If there is any infringement, please contact our official customer service for proper handling.